Figures & data
Figure 1 Dermoscopic image data preprocessing. Original dermoscopic image (left side) obtained from dermatology repository International Skin Imaging Collaboration (ISIC) archive (www.isic-archive.com) was cropped and removed the ruler, sticker, or hair (right side) for further analysis.
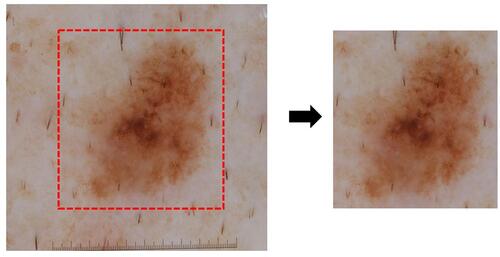
Figure 2 Schematic representation of experimental methodology. Initially input data is preprocessed to generate the dataset to be employed to further establish a CNN model. In parallel, patient metadata variable is also preprocessed to generate dataset for establishing the ANN model. These independent models were further combined to assess the impact on accuracy of the prediction between image only data (CNN model) and image plus patient’s metadata (CNN+ANN model).
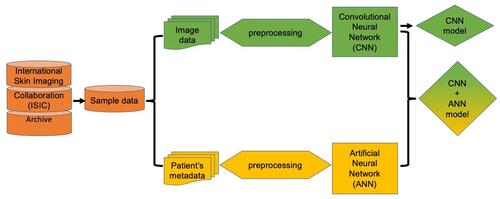
Figure 3 Model architecture. CNN model architecture (A) and CNN+ANN model architecture (B) showed the flow inside the model (printed from TensorFlow 1.14).
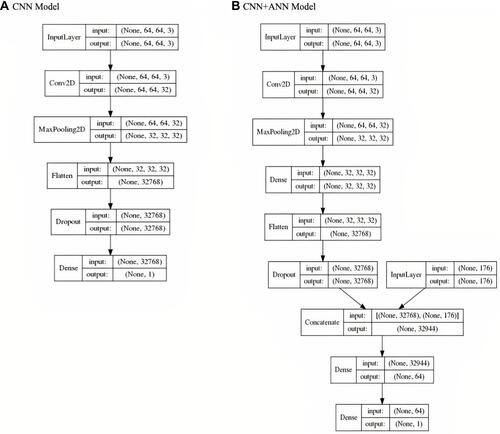
Table 1 Distribution of Patient’s Metadata
Figure 4 Mode learning curve. Based on loss and accuracy curve of training and validation vs epoch in each model, the learning optimization and performance of the CNN+ANN model (B) has improved more than the CNN model (A). The loss and accuracy of the CNN+ANN model (B) shows that the model is more representative and less overfitting than the CNN model (A).
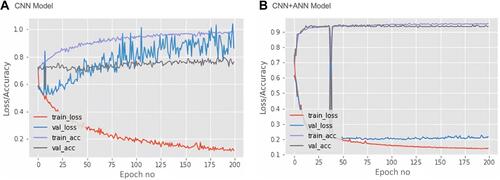
Figure 5 Area under the receiver operating characteristic curve (AUROC). The CNN+ANN model (red line) outperforms the CNN model (blue line).
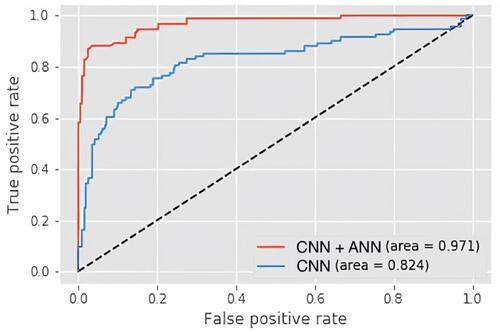
Figure 6 T-distributed stochastic neighbor embedding (t-SNE) plot to describe label distribution of malignant melanoma in the CNN model (A) and the CNN+ ANN model (B). The malignant melanoma tends to be classified better in the CNN+ANN model (B) than in the CNN model (A). One datum with malignant melanoma represented by one orange dot, one datum with nonmalignant melanoma represented by one blue dot.
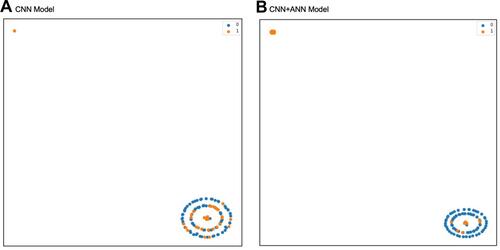
Table 2 Confusion Matrix CNN
Table 3 Confusion Matrix CNN+ANN
Table 4 Malignant Melanomas Detection Result Using CNN Model and CNN+ANN Model