Figures & data
Table 1 Sample attrition table
Table 2 Demographic characteristics of the evaluated cohorts
Figure 1 The ten most important variables for predicting a diagnosis of fibromyalgia identified from random forest models.
Abbreviation: ER, emergency room.
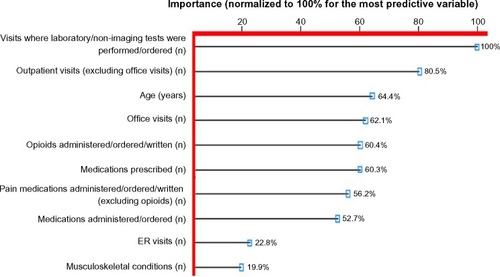
Figure 2 Receiver operating characteristic curve modeled using the test dataset.
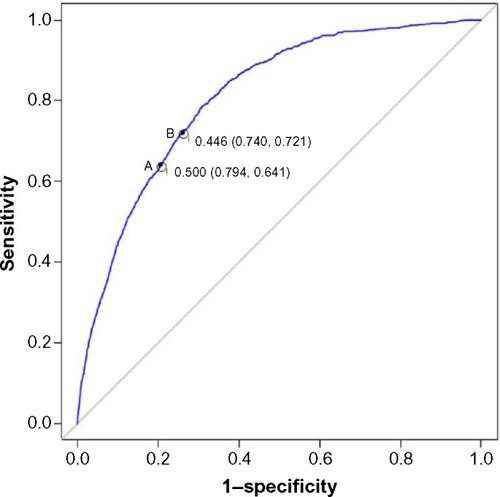
Figure 3 Cumulative distribution functions for the variables identified in the random forest model.
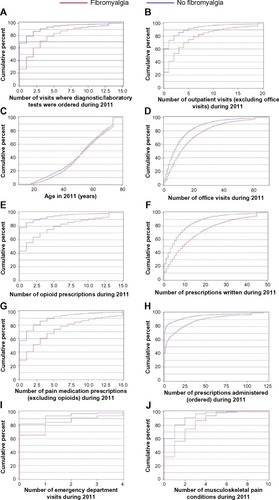
Table 3 Rules for identifying FM and no-FM subjects based on results of the predictive modeling using a technique known as C5.0 rules
Table S1 Variables put into random forest model