Figures & data
Figure 4 Sample results of the Cervix ROI extraction model (A) input images (B) ROI extracted images.
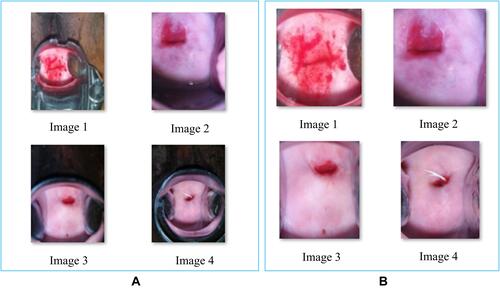
Table 1 Results of Models Trained Using ROI Extracted Data and Raw Data
Figure 6 Training and validation performance of EfficientNetB0 model for cervix type classification (A) Accuracy (B) Loss.
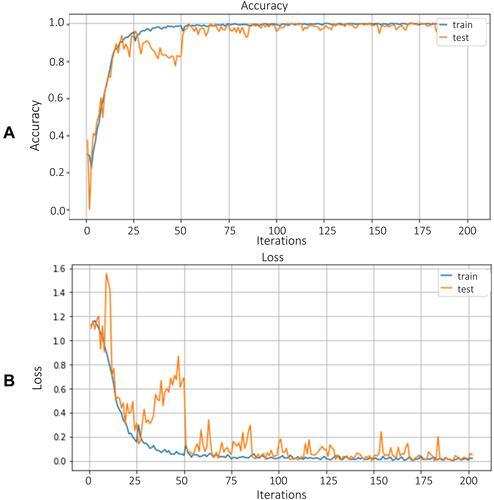
Table 2 Result of Cervix Type Classification with Different Metrics for the Final Model
Figure 7 Test results of EfficientNetB0 model for cervix type classification (A) Confusion matrix (B) ROC-AUC plot.
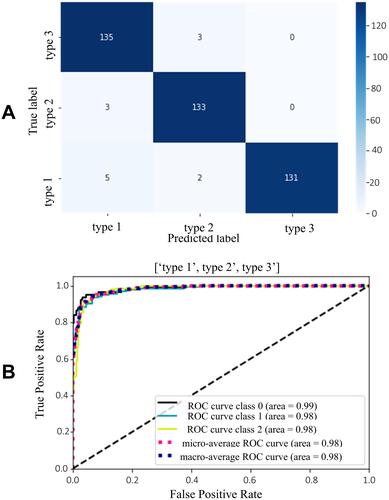
Figure 9 Effect of histogram matching on cervical histopathology sample images (A) Reference image (B) Source images (C) Histogram matched images.
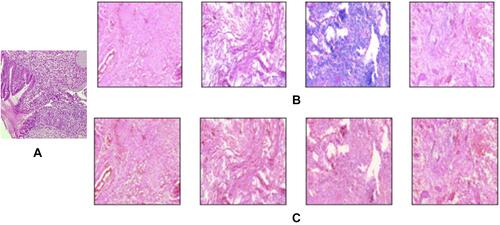
Figure 10 Training and validation performance of EfficientNetB0 model for cervical cancer type classification (A) Accuracy and (B) Loss.
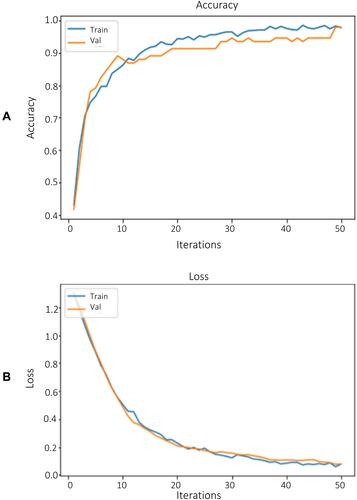
Table 3 Result of Cervical Cancer Classification with Different Metrics