Figures & data
Table 1 Demographic Characteristics of BSDB Subjects with Respect to Individual Data Splits
Table 2 Occurrence of Different Classes of Sleep Disorders Among Conclusive Diagnoses of Subjects per Individual Data Splits of BSDB
Table 3 Measures Evaluating Prediction’s Uncertainty Using U-Sleep Softmax Output
Figure 1 Schematic overview of datasets used, their size, and purpose.
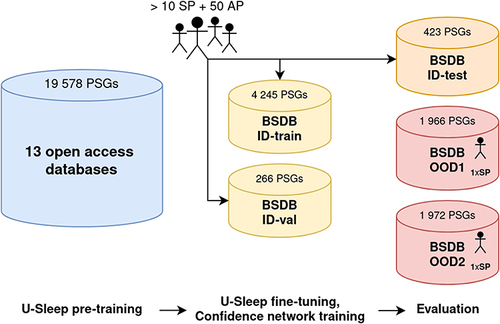
Table 4 Classification Performance of U-Sleep on Individual Data Splits
Table 5 Performance of Uncertainty Measures to Identify U-Sleep Predictions Discerning from Human Scoring on Individual Data Splits
Figure 2 Combined output of the predicted hypnogram (in white) and the associated confidence. TCP-scores (in the background), supplemented with the physician-scored hypnogram (in blue).
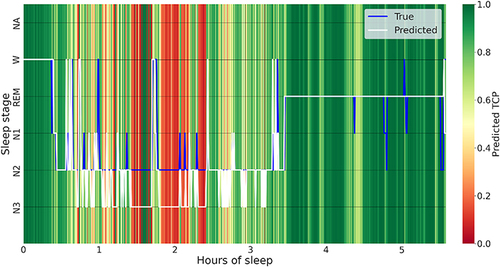
Figure 3 Schematic overview of the implemented pipeline.
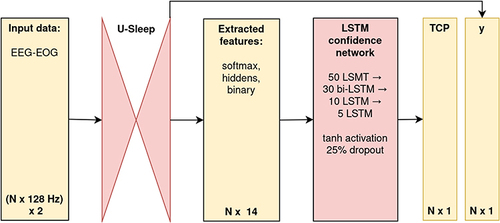
Table 6 Bootstrap Confidence Intervals for Difference of on-Subject Mean-Aggregated Confidence TCP-Scores of Aligning Vs Discordant Predictions
Table 7 Bootstrap Confidence Intervals for Correlation Between on-Subject Mean-Aggregated Confidence TCP-Scores and the Performance Metrics
Figure 4 Performance boost with physician’s review of epochs having confidence TCP-score lower than a given threshold.
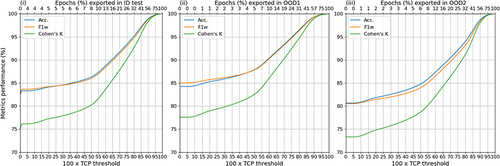
Table 8 Rescoring Amounts Needed to Achieve Desired Levels of Sleep-Scoring Performance