Figures & data
Table 1 Differences between demographic and clinical characteristics of adherent and nonadherent groups
Figure 1 Demographic and clinical feature selection using the LASSO binary logistic regression model.
Abbreviations: LASSO, least absolute shrinkage and selection operator; SE, standard error.
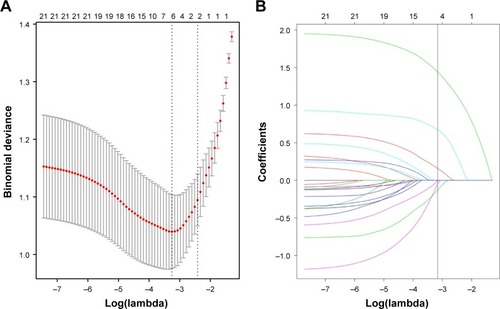
Table 2 Prediction factors for medication nonadherence in IRDs
Figure 2 Developed medication nonadherence nomogram.
Abbreviations: GC, glucocorticoid; NSAIDs, nonsteroidal anti-inflammatory drugs.
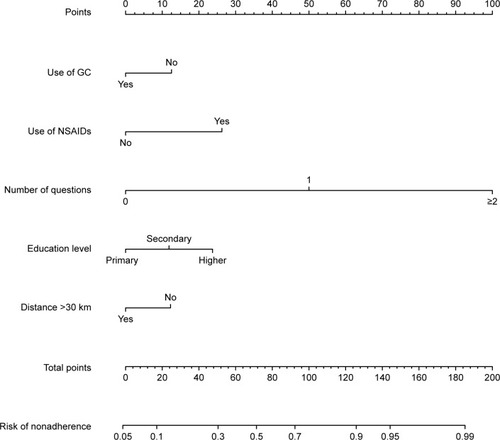
Figure 3 Calibration curves of the nonadherence nomogram prediction in the cohort.
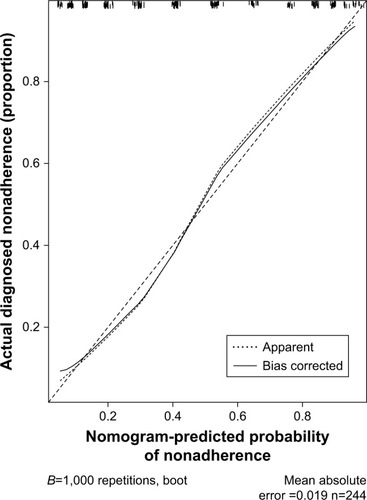
Figure 4 Decision curve analysis for the nonadherence nomogram.
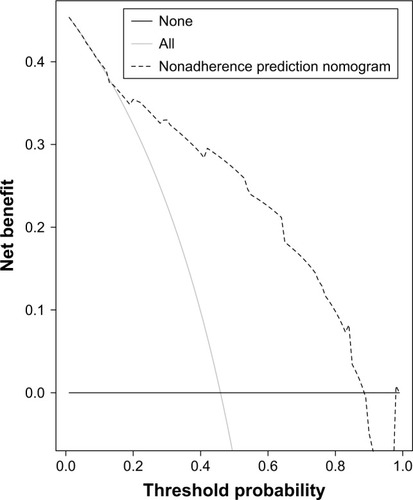