Figures & data
Table 1 Differences Between Demographic and Clinical Characteristics of T2DM and Non-T2DM Groups
Figure 1 Using the LASSO model of logistic regression to determine the connection between populations and clinical characteristics.
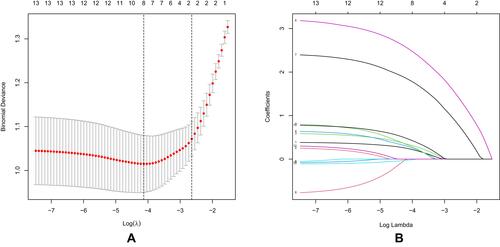
Table 2 Prediction Factors for HZ in T2DM Patients
Figure 2 Development of the HZ nomogram. The chart is made from the data based on gender, age, length of hospital stay, weight, 2 hour PG, creatinine, location of skin rash, and hypertension.
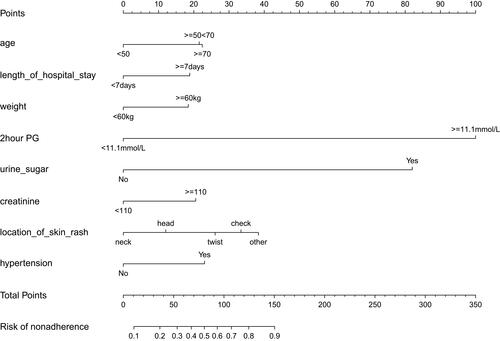
Figure 3 The calibration curves of the topic HZ nomogram prediction in the cohort.
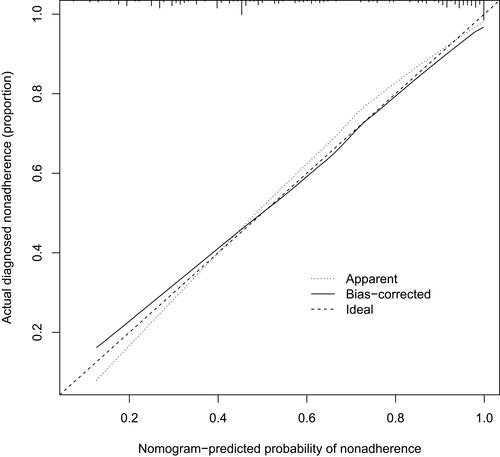
Figure 4 Decision curve analysis (DCA) for the HZ nomogram.
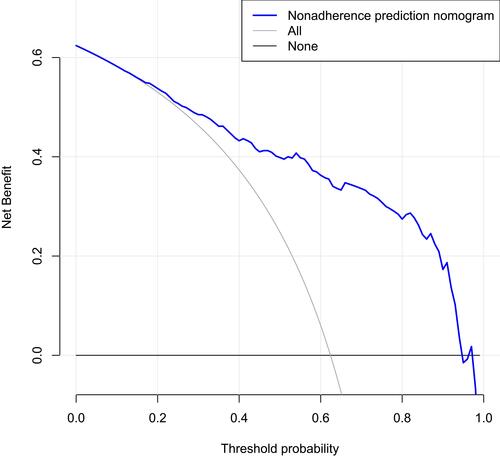