Figures & data
Figure 1 Screening of CTD-ILD patients.
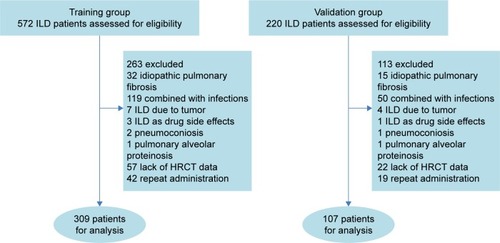
Figure 2 Feature selection.
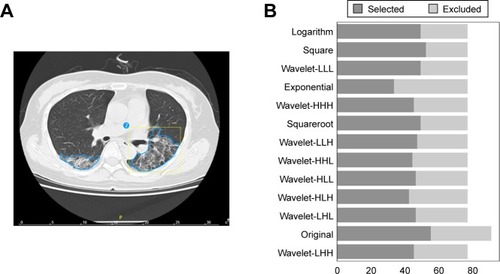
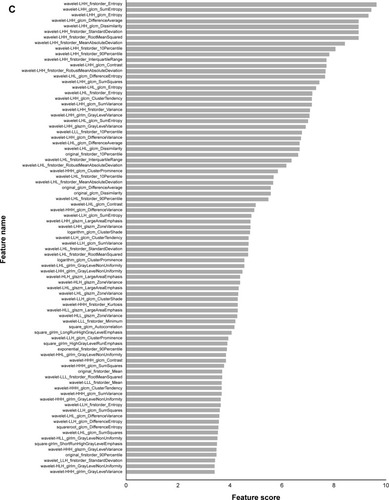
Table 1 Patient characteristics in the training and testing cohorts
Table 2 Characteristics of patients who responded or did not respond to treatment in the training and testing cohorts
Figure 3 Feature selection using the LASSO binary logistic model.
Abbreviation: LASSO, least absolute shrinkage and selection operator.
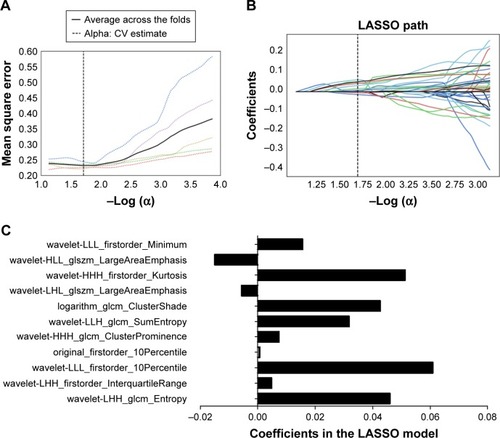
Table 3 LASSO coefficient profiles of the eleven features
Figure 4 ROC curves for machine learning of radiomics to predict treatment response.
Abbreviations: AUC, area under the curve; k-NN, k-nearest neighbors; RF, random forest; ROC, receiver-operating characteristic.
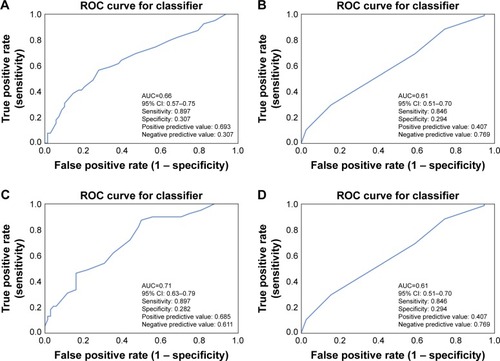
Figure 5 Developed radiomics nomogram.
Abbreviation: ICU, intensive care unit.
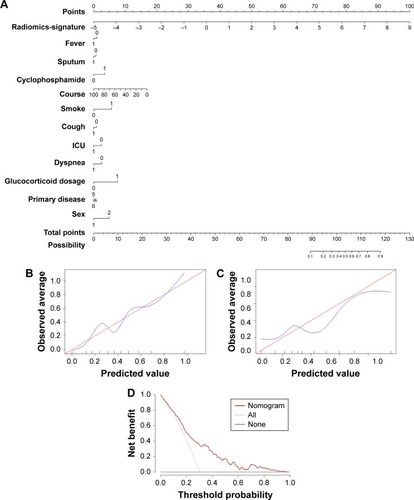