Figures & data
Table 1 Demographic Characteristics of the Patients
Table 2 Comparisons of Clinical Outcomes Between Survival and Non-Survival Groups
Table 3 Predicting Indicators Screened from LASSO Regression
Figure 1 The LASSO regression analysis identified variables correlated with ICU death.
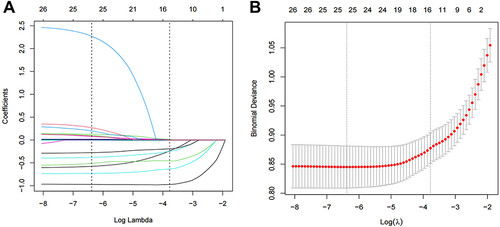
Figure 2 The predictive values of the model of machine learning algorithm.
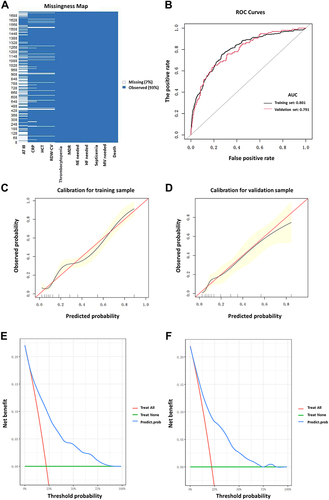