Figures & data
Table 1. Mapping and CpG capture quality control matrices for different sequencing libraries.
(A) Number of CpGs captured at 5X from SAAP-BS and Bismark pipelines. Except for Swift-seq, all others have a higher number of CpGs at 5X from SAAP-BS pipeline. This is quite dramatic for QIAseq and is mostly related to mapping rate and duplication read identification and removal. (B) CpG methylation correlations among libraries by SAAP-BS. The highest correlation was between Swift-seq and EM-seq 25 ng across protocols while the lowest was between QIAseq and EM-seq 10 ng, which have the highest read duplication rates. (C). Pairwise correlations among libraries and processing pipelines. (D) Correlation heatmap among libraries and processing pipelines. Pipelines make little difference.
Bis: Bismark; Bsm: SAAP-BS with BSMAP; EM: NEBNext Enzymatic Methyl-Seq; QIAseq: QIAseq methyl-sequencing; SwiftSeq: Swift Accel-NGS-Methyl-Seq; SAAP-BS: Streamlined Analysis and Annotation Pipeline for Bisulfite Sequencing.
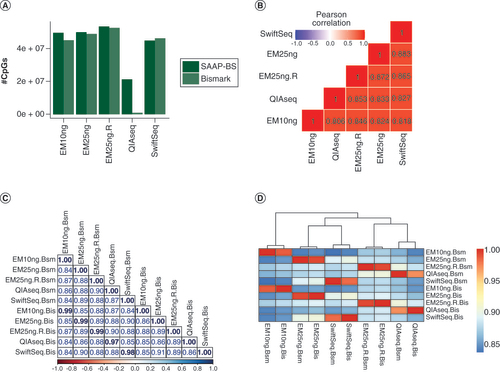
(A) GIAB SNP set as truth set. (B) Internal whole-genome sequencing variant set as truth set. In both cases, EM-seq leads to the best recall, precision and F1 score. QIAseq performs poorly for this purpose while it can be largely rescued using SAAP-BS pipeline and Biscuit caller. For genomic positions with 5 or 10X coverage, all callers make very accurate calls (high precision) although some variants may be missed.
EM: NEBNext Enzymatic Methyl-Seq; GIAB: Genome in a bottle; lib.aln.caller: Library preparation, aligner and caller combination; QIAseq: QIAseq methyl-sequencing; SwiftSeq: Swift Accel-NGS-Methyl-Seq; SAAP-BS: Streamlined Analysis and Annotation Pipeline for Bisulfite Sequencing; 1,5,10X: Coverage at 1, 5, 10 sequence reads.
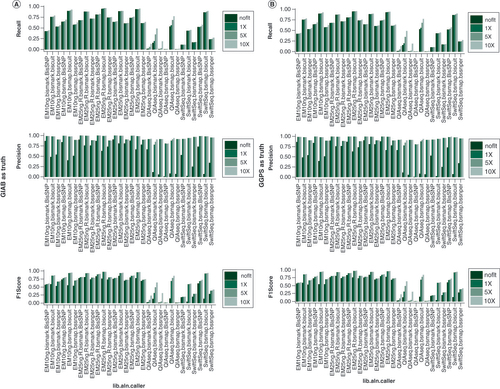
(A) Bin size of 1K without SNV usage. For comparisons with public CNV truth sets, performances of methylation sequencing libraries were similar to WGS although they all had low recall and F1 scores. Methylation sequencing gets about 75% of CNVs detected from WGS. (B) Bin size of 5K without SNVs. (C) Bin size of 5K with GIAB SNVs. (D) Bin size of 5K with SNPs called from each library itself. Improved performance can be seen with incorporating SNVs using CNVpytor, particularly for SNV set for GIAB, which has more complete and higher quality SNPs (versus SNVs from each library, which is more variable depending on libraries). Gold: Gold reference CNV set from 1000 genome project. 1K.cnvnator: CNV calls from 1000 genome project sample using CNVnator alone by Haraksing et al. [Citation17]. Lumpy: CNV calls using Lumpy along with orthogonal validations. i.cnvpytor: CNV calls from an internal WGS sample using CNVpytor, the new version of CNVnator with python implementation and extended functionality such as incorporation of SNVs to increase CNV call accuracy.
EM: NEBNext Enzymatic Methyl-Seq; QIAseq: QIAseq methyl-sequencing; SwiftSeq: Swift Accel-NGS-Methyl-Seq.
![Figure 3. CNV call accuracies (recall, precision and F1 score) with four reference sets from different methylation libraries at bin sizes of 1k and 5k. (A) Bin size of 1K without SNV usage. For comparisons with public CNV truth sets, performances of methylation sequencing libraries were similar to WGS although they all had low recall and F1 scores. Methylation sequencing gets about 75% of CNVs detected from WGS. (B) Bin size of 5K without SNVs. (C) Bin size of 5K with GIAB SNVs. (D) Bin size of 5K with SNPs called from each library itself. Improved performance can be seen with incorporating SNVs using CNVpytor, particularly for SNV set for GIAB, which has more complete and higher quality SNPs (versus SNVs from each library, which is more variable depending on libraries). Gold: Gold reference CNV set from 1000 genome project. 1K.cnvnator: CNV calls from 1000 genome project sample using CNVnator alone by Haraksing et al. [Citation17]. Lumpy: CNV calls using Lumpy along with orthogonal validations. i.cnvpytor: CNV calls from an internal WGS sample using CNVpytor, the new version of CNVnator with python implementation and extended functionality such as incorporation of SNVs to increase CNV call accuracy.EM: NEBNext Enzymatic Methyl-Seq; QIAseq: QIAseq methyl-sequencing; SwiftSeq: Swift Accel-NGS-Methyl-Seq.](/cms/asset/1f19e5e4-79d8-40f3-968c-df9946c440f0/iepi_a_12324449_f0003.jpg)