Figures & data
Figure 1 Mathematical descriptions of the same biology can vary in mechanistic complexity. Both panels here depict the same subprocess encountered in growth factor receptor signaling networks: receptor-mediated recruitment of guanine nucleotide exchange factor (GEF) activity, which promotes conversion of the small GTPase Ras to its GTP-bound form. (A) A minimal mass-action description requires explicit accounting of all molecular constituents (as described in the text), resulting in a large number of adjustable parameters. (B) A coarse-grained description instead considers only the association of the GEF with the receptor complex, limited by the availability of a certain, sparingly abundant component (the GEF itself or an intermediary adaptor). Other steps in the formation of the complex are effectively lumped.
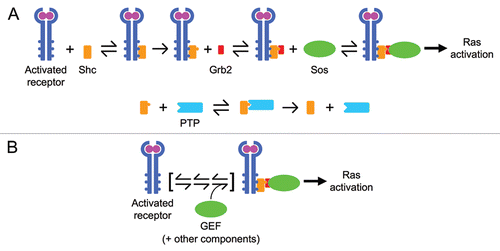
Figure 2 The number of model parameters needed should be much fewer than the number of distinct measurements to which the model is compared. (A) A signaling readout exhibiting partial adaptation has as few as four distinct features: the peak magnitude, the time at which it is reached, the characteristic time or rate of decay from the peak to the subsequent plateau, and the magnitude of the plateau. (B) We systematically measured both MEK and ERK phosphorylation for an array of stimulation and perturbation conditions; a portion (25%) of those data are shown here. The symbols and solid curves are, respectively, the means of the data and the model output aligned globally to all of the data, color-coded according to the dose of growth factor (adapted from Fig. 5 of ref. Citation3). Michaelis Menten-type equations coupling ERK phosphorylation to the active form of MEK are shown below; simplification of these equations to neglect enzyme saturation would reduce the number of adjustable parameters from 8 to 4.
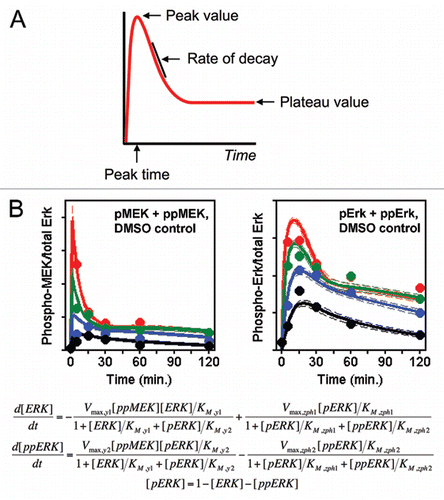