Figures & data
Figure 1. A visual of our analysis strategy applied to human in vitro, high dose, 8 h sampling time data. We begin in the top row (1-a), by conducting a Differentially Expressed Genes (DEGs) analysis on the gene expression matrix; columns represent the 93 samples (40 “most” and 53 “less or no” DILI concern), rows represent 1000 expressed genes. This returns a list of top DEGs (1-b); the genes that are most significantly associated with DILI concern. We then move to the middle row (2-a), using sparse PCA on the same gene expression matrix to obtain new sparse principal component (PC) variables (2-b) to work with; columns for this new data matrix again represent the 93 samples, but rows represent the 93 new sparse PC variables (we have reduced the dimension from 1000 to 93). Then, we conduct a Differentially Expressed PCs (DEPCs) analysis on the PC expression matrix to obtain a list of top DEPCs (2-c); the sparse PCs that are most significantly associated with DILI concern. At this point, we examine the genes that contribute to these DEPCs to makes sense of what the structures mean and make note of those genes in these structures that were also identified as differentially expressed in the DEGs analysis. As a final validation step (3-a), we apply sparse regression to the same 93 sparse PC variables to identify a concise list of sparse PCs that are potentially related to DILI concern (3-b).
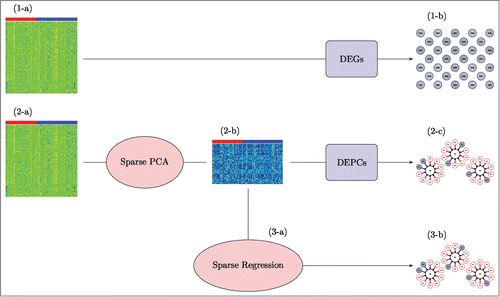
Table 1. Differentially expressed genes (DEGs; independently associated with DILI concern) from our analysis of the human in vitro, high dose, 8 h gene expression sampling time subset
Table 2. Counts of DEGs across all 16 subsets analyzed
Table 3. Differentially expressed PCs (DEPCs; associated with DILI concern) from our analysis of the human in vitro, high dose, 8 h gene expression sampling time subset
Figure 2. A visual display for the top 3 differentially expressed (most associated with DILI concern) sparse principal components (DEPCs) from our analysis of the human in vitro, high dose, 8 h gene expression sampling time subset. Larger central circles represent the principal components. Attached to each are the genes that form the linear combinations; probesets (gene names) and loading values are inside the outer circles. Shaded circles represent genes that were found to be independently associated with DILI concern (DEGs), whereas non-shaded circles contain genes that were not. PC15 might bring forth a network of trancriptomic material that is associated with DILI concern, not otherwise being found with more simple statistical tests. PC13 shows us that some marginally associated genes behave similarly.
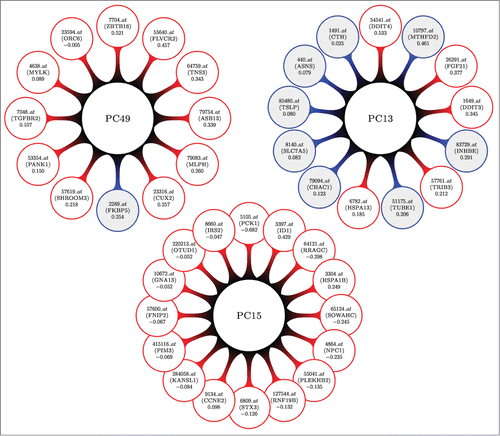
Table 4. Counts of DEPCs across all 16 subsets analyzed. The total number of DEPCs, along with the number of those which are upregulated (“most DILI concern” has larger PC cumulative expression values than “Less or No DILI concern”) in brackets and those which are downregulated in square brackets