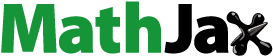
Abstract
Molecular docking and quantitative structure–activity relationship (QSAR) studies were carried out on 37 anticonvulsant compounds to develop a robust model for the prediction of anticonvulsant activities against Gama amino butyric acid aminotransferase (GABAAT) and to determine the dominant structural amino acid residues responsible for the binding affinity of the ligand-GABAAT complex. AutoDock Vina of PyRx virtual screening software was used to perform the molecular docking while Genetic function algorithm (GFA) was used to select the descriptors and to generate the correlation models that relate the structural features to the biological activities. The best binding affinity was found to be −11.9 Kcal/mol (compound 5a) while best QSAR model (model 1) was obtained with R2 of 0.970192, an R2adj value of 0.963095, Q2LOO value of 0.947995 and R2pred of 0.813. These confirms the stability, reliability, robustness and predictability of the model. Our research has shown that the binding affinity generated was found to be better than the one reported by another researcher. And the high correlation coefficient, (R2) shows that the model was reliable, robust and predictable. Our QSAR model and molecular docking results corroborate with each other (most especially in the area of binding affinity and atomic electronegativity of the inhibitors) and propose the directions for the design of new inhibitors with better activity against an enzyme that is responsible for epilepsy (GABAAT).
1 Introduction
Epilepsy is a well-documented neurological issue that influences roughly 65 to 75 million individuals around the world, of which 10.5 million are children [Citation1,Citation2] . However, the worldwide prevalence of epilepsy varies from 2.8 to 19.5 per 1000 of the general population [Citation3]. Epilepsy is basically a chronic brain disorder characterized by recurrent derangement of the nervous system due to the sudden excessive disorderly discharge of neurons that result in almost instantaneous disturbance of sensation and loss of consciousness [Citation4]. Seizures which is usually caused by epilepsy can cause a variety of symptoms depending on the areas of the brain affected. Symptoms may be the complete or partial loss of consciousness, loss of speech and uncontrollable motor behavior [Citation5].
The in silico approaches like Quantitative Gama amino butyric acid aminotransferase (GABAAT) catalyzes the conversion of GABA to succinylic semialdehyde. Convulsion is always triggered by reduced levels of GABA, while the high level of GABA in the brain has an anticonvulsant effect [Citation6Citation[7]Citation[8]–Citation9] . GABAAT is a receptor for most anti-epileptic drugs because of its selective deactivation raises GABA concentration in the brain [Citation10]. This understanding of GABA neurotransmitter paved the way for future research and some of the disorder's first effective treatments [Citation11Citation[12]–Citation13] .
Structure-Activity Relationships (QSAR) and molecular docking are widely used in the fields of structural molecular biology and structure-based drug design. Molecular docking is a computational procedure used in the field of structure-based rational drug design to identify correct conformations of small molecule and also to estimate the strength of the protein-ligand interaction [Citation14Citation[15]–Citation16] . Quantitative Structure-Activity Relationships (QSAR) models have gained an extensive recognition in the field of sciences [Citation17Citation[18]Citation[19]Citation[20]Citation[21]Citation[22]Citation[23]–Citation24] .
The aim of this research is to develop good and rational QSAR models that could predict the activities (pED50) values of quinoxaline and thiadiazoles derivatives (inhibitors) whose biological activities (ED50) against Gama amino butyric acid aminotransferase (GABAAT) and to predict the interactions energy between GABAAT and the inhibitors
2 Material and methods
2.1 Data sets used
Some quinoxaline and thiadiazoles derivatives were selected from the literature and used as anticonvulsant activity for this study [Citation25Citation[26]–Citation27] . The logarithm of measured ED50 against an anticonvulsant activity as pED50 (pED50 = log 1/ED50) was used as dependent variable, consequently correlating the data linearly to the independent variable/descriptors. The observed structures and the biological activities of these compounds are presented in .
Table 1 Biological activities of training and test set derivatives.
2.2 Docking study
2.2.1 Selection and refinement of receptors
Computer-aided drug design involves the identification and selection of the appropriate drug target [Citation28]. Gama amino butyric acid aminotransferase (GABAAT) was the target for the quinoxaline and thiadiazoles derivatives, and the three-dimensional structure of this protein was retrieved from Protein Data Bank (www.rcsb.org/pdb) using PDB ID: 10HV. The target protein was prepared by removing water molecules, adding Polar hydrogen atoms, minimizing energy, and the structure was saved as the pdbqt format. shows the prepared receptor (GABAAT).
2.2.2 Ligand input file preparation and optimization
37 quinoxaline and thiadiazoles derivatives input structures were drawn using the graphic user interface of Spartan’14 version 1.1.2 software [Citation29]. The drawn structures were cleaned in 3D format and optimized using Spartan’14 version 1.1.2 [Citation29]. The resulting structures were then saved in pdb format for molecular docking studies.
2.2.3 Docking
The docking of the quinoxaline and thiadiazoles derivatives into the active site of GABAAT protein was carried out using AutoDock Vina of PyRx virtual screening software [Citation30]. Autodock vina has been reported to be an effective tool capable of quickly and accurately predicting bound conformations and binding energies of ligands with macromolecular targets [Citation31]. In the graphic user interface of PyRx virtual screening software, the grid box with a dimension of 60 × 60 × 60 points and 0.375 Å grid spacing was used to cover the entire protein binding site and accommodate ligand to move freely. After docking searches were completed, the best conformation was chosen from the most populated cluster with the minimum binding energy (highest binding score). The interaction of docked protein-ligand complex conformations, including hydrogen bond and hydrophobic interactions were analyzed using Discovery Studio Visualizer 4.1, Ligplot and PyMol visualization software [Citation32].
2.2.4 Geometry optimization and calculation of physiochemical properties
The Spartan’14 version 1.1.2 software [Citation29] running on Toshiba Satellite, Dual-core processor window eight (8) operating system was used to draw the molecular structures of the quinoxaline and thiadiazoles derivatives. All the structures of these compounds were geometrically optimized by minimizing energy (see ). The physicochemical properties of all the 37 compounds were calculated by means of Density functional theory (DFT) using the B3LYP methods and 6-31G∗ basis set. The lowest energy structure was used for each molecule to calculate their physicochemical properties. The optimized structures from the Spartan’14 version 1.1.2 [Citation29] Quantum chemistry package were saved in SDF format and transferred to PaDEL-Descriptor version 2.18 toolkit [Citation33] where the calculation of all the dimensional descriptors took place.
The 37 data sets descriptors generated from the PaDEL version 2.18 toolkit [Citation33] were divided into training and test sets (see ). The training sets were used to develop the model, while the test sets were used to test for the quality assurance of the model. The Material studio software version 8 was used to perform the correlation analysis between activity values of the molecules against GABAAT and the calculated descriptors. The Genetic Function Approximation (GFA) method in material studio software versions 8 was used to perform the regression analysis of the generated descriptors.
2.2.4 Quality assurance of the developed model
The reliability and predictive ability of the generated models were assessed by internal and external validation parameters. These validation parameters were compared with the minimum recommended value for the QSAR model standard [Citation34] showed in .
Table 2 Minimum recommended value of Validation Parameters for a generally acceptable QSAR model.
2.2.5 Determination of descriptors variance inflation factor (VIF)
The best regression model was generated by considering all the possible combination of descriptors. Variance inflation factor (VIF) [Citation35] was used to identifying the multi-collinearity among variables. The VIF for the regression coefficient is expressed as: represents the coefficient produced by regressing the descriptor xi against the other descriptors,
(j
) If VIF was greater than 10, it was not considered as a model
2.2.6 Calculation of physiochemical descriptors
Physicochemical descriptors are an expression of quantitative structure of a molecule, which are lipophilic, electronic and steric in nature. Physicochemical descriptors used in this study are presented in .
Table 3 List of physicochemical descriptors selected for this study.
3 Results and discussion
All the four developed QSAR models (1, 2, 3, and 4) were reported out of which model 1 was chosen as the best model for predicting the pED50 of anticonvulsant molecules due to its statistical significance. As shown in , the internal and external validation parameters of the model 1 conformed to the minimum standard for a stable, reliable, predictable and robust QSAR model. Furthermore, model 1 was chosen as the best model because the highest squared correlation coefficient (R2) of 0.970, adjusted squared correlation coefficient (R2adj) value of 0.963, Leave one out (LOO) cross-validation coefficient (Q2) value of 0.948 and the external validation (R2ext) of 0.813 were confirmed with the minimum recommended value () for a generally acceptable QSAR model [Citation34].
Model 1Model 2
Model 3
Model 4
3.1 Plot of experimental versus predicted ED50 of both training and test sets of model 1
gives the plot of predicted activities of both training and test sets against observed activities; the reliability of the model (best QSAR model) was confirmed as the GFA derived R2 value was in agreement with the R2 value of 0.970 recorded in this graph. The high Linearity of this plot indicates the high predictive power of the model.
3.2 Comparison of observed and predicted pED50 of model 1
The comparison of the predicted pED50 of the model 1 with its experimental values is presented in . The low residual values shown in the confirm the high predictive power of the model
Table 4 Comparison of Observed, Predicted and Residual of Model 1.
3.3 External validation of model
Test set compounds of external validation of model 1 revealed a good agreement between the actual and predicted pED50 of the test set molecules. The actual, predicted and residual pED50 values of the test set compounds are presented in the .
Table 5 External validation of Model 1.
3.4 Calculation of predictive R2 of model
The stability, reliability and robustness of the generated model 1 were confirmed by predictive R2 (). The calculated predictive R2 for model 1 were in conformity with the standard value shown in .
Table 6 Calculation of Predictive R2 of Model 4.10.
3.5 Variance inflation factor (VIF) Statistic for the descriptors in model 1
The corresponding VIF values of the five descriptors are presented in . As can be seen from this table, all the variables has VIF values of less than 10, indicating that the obtained model has statistical significance, and the descriptors were found to be reasonably orthogonal [Citation35].
Table 7 Variance Inflation Factor (VIF) Statistic for the Descriptors in Model 1.
3.6 Result of molecular docking studies of quinoxaline and thiadiazoles derivatives
Molecular docking studies were carried out between the targets (GABAAT) and its inhibitors (quinoxaline and thiadiazoles derivatives). In , all the compounds were found to strongly inhibit by completely occupying the active sites in the target protein (GABAAT). All the GABAAT inhibitors showed lower energy values (higher docking scores) than the binding energies of vigabatrin (−4.4 kcal/mol), the standard antiepileptic drug. For target protein, binding energy values range from −5.8 to −11.9 kcal/mol. Furthermore, shows the best first-three docking results
Fig. 4 3D and 2D of (A): GABAAT-Ligand 5a Complex. (B): GABAAT-Ligand 16a Complex. (C): GABAAT-Ligand 17a Complex. Ligand: H-bond interactions represented by green dashed lines: Hydrophobic interactions represented by red dashed line.
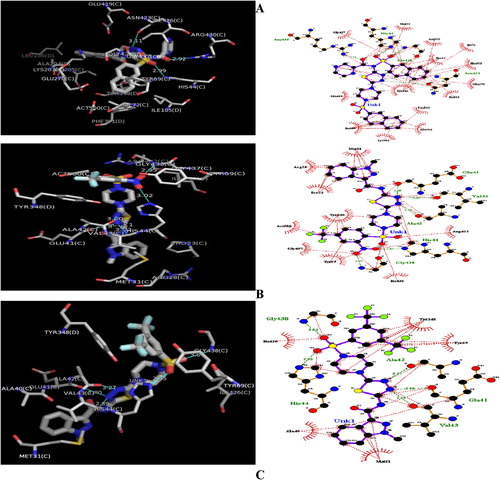
The positive coefficient in model 1 implies that increase in physiochemical parameters such as minHBint4 (Minimum E-State descriptors of strength for potential Hydrogen Bonds of path length 4), ETA_Alpha (Sum of alpha values of all non-hydrogen vertices of a molecule) and GRAV-5 (Square root of gravitational index of all pairs of atoms) will increases the anticonvulsant activities (pED50) of these quinoxaline and thiadiazoles derivatives against GABAAT enzyme, an enzyme responsible for epilepsy. More also, negative coefficient of DPSA-1 (Difference of PPSA-1 and PNSA-1) and WT.eneg (Non-directional WHIM, weighted by Mulliken atomic electronegativity) are inversely proportional to the inhibitory activities of quinoxaline and thiadiazoles derivatives.
MinHBint4 (Minimum E-State descriptors of strength for potential Hydrogen Bonds of path length 4) is an electrotopological state atom type descriptor. In model 1, positive coefficient of this descriptor implies that increase in this descriptor will directly increase the inhibitory activities of the inhibitors against GABAAT enzyme, an enzyme that causes epilepsy. Furthermore, ETA_Alpha (Sum of alpha values of all non-hydrogen vertices of a molecule) and GRAV-5 (Square root of the gravitational index of all pairs of atoms) are extended topochemical atom and the gravitational index descriptors. These two descriptors (model 1) are directly proportional to the inhibitory activities (pED50) of the quinoxaline and thiadiazoles derivatives (inhibitors). Therefore, increase the value of ETA_Alpha and GRAV-5 will lead to an increase in the inhibitory activities of quinoxaline and thiadiazoles derivatives against GABAAT enzyme.
DPSA-1 (Difference of PPSA-1 and PNSA-1), the charged partial surface area descriptor (CPSA) provides information that describes global and local electrophilicity in case of non-covalent molecular interactions [Citation36]. Moreover, DPSA-1 descriptor provides separation information about high binding affinity to estrogen receptor [Citation37]. Therefore, reduced level of DPSA-1 descriptor will increase the inhibitory activities of quinoxaline and thiadiazoles derivatives against an enzyme that causes epilepsy (GABAAT).
WT.eneg (Non-directional WHIM, weighted by Mulliken atomic electronegativites) which is 3D, is defined as WHIM descriptor [Citation38]. It encodes information about the weight of atomic electronegativites of the molecules (GABAAT inhibitors). The QSAR model (Model 1) pointed out that the pED50 (activities) of the inhibitors (quinoxaline and thiadiazoles derivatives) increases with the decrease in this descriptor. A critical look at this descriptor inferred that the atomic electronegativity in a molecule have to be reduced in order for the inhibitory activities of quinoxaline and thiadiazoles derivatives to be effective in inhibiting the activities of GABAAT enzyme, an enzyme responsible for epilepsy.
From the docking study, it was shown that quinoxaline and thiadiazoles ring of ligand number 5a () with the highest binding energy of −11.9 kcal/mol and RMSD of 0.00 are surrounded by hydrophobic residues. In A, quinoxaline and thiadiazoles ring is bounded by hydrophobic pockets consisting of amino residues such as Gly437, Ile426, Act500, Ile72, Tyr69, Phe351, Glu270, Ile105, Leu166, Ala204, Lys203, Glu419, Ile205, and four hydrogen bonding of Arg4309 (2.92 A°), His44 (2.87 A°), Gly438 (2.87 A°) and Asn423 (3.11 A°). This insilico study revealed that ligand number 5A showed good binding energy toward the GABAAT protein than other co-ligands ().
Table 8 GABAAT active site residues involved in docking interactions with the inhibitors and docking scores.
The docked models reveal that O-1, O-3, O-2 and N-2 of the quinoxaline and thiadiazoles ring forms hydrogen bonds with amino acids backbone of Arg4309 (2.92 A°), His44 (2.87 A°), Gly438 (2.87 A°) and Asn423 (3.11 A°) respectively. The hydrogen bonding distance for highly active compound 5a is shown in A. The hydrogen bonding distance for remaining compounds is shown in . The quinoxaline and thiadiazoles ring play a crucial role in producing biological activity by interacting with Arg4309, His44, Gly438 and Asn423, an important active residue for binding affinity of the inhibitor. These interactions underscore the importance of electronegative elements as such oxygen and nitrogen atoms for binding and subsequent inhibitory capacity.
The decrease in descriptors such as DPSA-1 and WT.eneg in particular will increase the binding affinity and atomic electronegativity of these inhibitors. A critical look at these two descriptors inferred that the binding affinity and atomic electronegativity have to be considered in order for the inhibitory activities of quinoxaline and thiadiazoles derivatives to be effective in inhibiting the activities of GABAAT enzyme, an enzyme responsible for epilepsy. This is in agreement with the result of molecular docking in which compounds 5a (A) with the best binding affinity of −11.9 kcal/mol is bounded by hydrogen bond to amino acid residues through the electronegative atoms such as oxygen (O-1, O-3, O-2) and nitrogen (N-2) of the ligand number 5A ring.
Therefore, it can be inferred that the quinoxaline and thiadiazoles derivatives are good anticonvulsant compounds that possessed inhibiting potential against an enzyme that is responsible for epilepsy (GABAAT enzyme).
4 Conclusion
The approach used in this research was successful in finding novel GABAAT inhibitors from the data set developed by computational methods. The robustness and applicability of the QSAR models have been established by internal and external validation techniques. It has been revealed that the dominant structural features responsible for the inhibitory activity of quinoxaline and thiadiazoles derivatives against an enzyme responsible for epilepsy (GABAAT) were physiochemical parameters such as minHBint4, ETA_Alpha, DPSA-1, GRAV-5, and WT.eneg. In docking analysis, Compound 5a indicated higher binding affinity with docking score of −11.9 kcal/mol against GABAAT than other co-ligands. From the docking analysis, we realized that the binding scores generated were found to be better than the one proposed by another researcher [Citation39].
The physicochemical descriptors used in QSAR analysis (model 1) in this study were important parameters to consider in improving the potency of these substituted quinoxalines and thiadiazoles derivatives as inhibitors of GABAAT. Our QSAR model and molecular docking results corroborate with each other (most especially in the area of binding affinity and atomic electronegativity of the inhibitors) and propose the directions for the design of new inhibitors with better activity toward GABAAT an enzyme responsible for epilepsy.
Conflict of interest
No conflict of interest.
Funding
The authors received no direct funding for this research.
Compliance with ethics requirements
This article does not contain any studies with human or animal subjects.
References
- L.P.YemadjeD.HoinonatoF.QuentM.Druet-CabanacP.M.PreuxUnderstanding the differences in prevalence of epilepsy in tropical regionsEpilepsia52201113761381
- Guerrini R. Epilepsy in children. Seminar at Department of child Neurology and Psychiatry. University of Pisa and IRCCS Foundazionestella Maris; 2006:367;499–524.
- F.G.DantasG.A.CaririA.R.FilhoKnowledge and attitudes towards epilepsy among primary, secondary and tertiary level teachersNeurology-Psiquiatar592001712716
- M.KabirZ.IliyasuI.S.AbubakarZ.S.KabirA.U.FarinYaroKnowledge, attitude and belief about epilepsy among adults in a Northern Nigeria Urban communityAnn Africa Med42012107112
- L.L.AtshunlerDepression, anxiety and temporal lobe epilepsy. Laterality of focus and symptomsArch Neurol41990284288
- K.I.OsolodkinV.I.ChupakhinV.A.PalyulinN.S.ZefirovMolecular modeling of ligand-receptor interaction in GABAc receptorJ Mol Graphics Mod72009813821
- A.J.SmithP.B.SimpsonMethodological approaches for the study of GABAA receptor pharmacology and functional responsesAnal Bioanal Chem3772003843851
- A.KarlssonF.FonnumD.Malthe-SorenssenJ.Storm-MathisenEffect of the convulsive agent 3 mercaptopropionic acid on the levels of GABA, other amino acids and glutamate decarboxylase in different regions of the rat brainJ Biochem Pharmacol23197430533061
- P.Krogsgaard-LarsenGamma aminobutyric acid agonists, antagonists, and uptake inhibitors: design and therapeutic aspectsJ Med Chem24198113771383
- P.StoriciG.CapitaniD.D.BaiseM.MoserR.A.JohnJ.N.Jansoniuset al.Crystal structure of GABA aminotransferase, a target for antiepileptic drug therapyBiochemistry38199986288634
- T.XuS.M.BajjaliehSV2 modulates the size of the readily releasable pool of secretory vesiclesNat Cell Biol32002691698
- M.A.DichterM.J.BrodieNew antiepileptic drugsEnglish J Med336199615831590
- B.A.MalawskS.GobaillSynthesis, physicochemical and pharmacological properties N-substituted amides of α-piperazine-gamma-hydroxybutyric acidPhamarzie501995390
- E.YurievM.AgostinoP.A.RamslandChallenges and advances in computational docking: in reviewJ Mol Recognit242009149164
- C.MuraC.E.McAnanyAn introduction to biomolecular simulations and dockingMol Simul402014732764
- A.A.TantarS.ConilleauS.B.ParentN.MelabL.BrilletS.Royet al.Docking and biomolecular simulations on computer grids: Status and trendsCurr Comput-Aided Drug Des42008235249
- C.HanschA.LeoQ.S.A.R.ExploringFundamentals and applications in chemistry and biology1995American Chemical SocietyWashington, DC
- H.KubinyiDrug Discovery Today21997457467
- O.Ivanciuc3D QSAR ModelsM.V.DiudeaQSPR/QSAR Studies by Molecular Descriptors2001Nova ScienceHuntington, N.Y.
- C.HanschA.LeoD.E.HoekmanQ.S.A.R.ExploringFundamentals and application in chemistry and biology1995Am Chem SocWashington, DC, USA
- S.MaroneI.RozasD.F.WeaverTheoretical structural analyses of tricyclic neuroactive drugs: quantum pharmacologic descriptors for clustering anticonvulsant, antidepressant, and antipsychotic activitiesJ Mol Struct (Theochem)46719992530
- M.MarderM.G.EstiuL.B.BlanchH.ViolaC.WasowskiJ.H.MedinaMolecular modeling and QSAR analysis of the interaction of flavones derivatives with the benzodiazepine site of GABAA receptor complexBioorg Med Chem92001323335
- H.VerliM.G.AlbuquerqueR.Bicca de AlencastroE.J.BarreiroLocal intersection volume: a new 3D descriptor applied to develop a 3D-QSAR pharmacophore model for benzodiazepine receptor ligandsEur J Med Chem372002219229
- A.J.JinH.KohnC.BéguinS.V.AndurkarJ.P.StablesD.E.WeaverA quantitative structure activity relationship study for Alpha_-substituted acetamido-N-benzylacetamide derivatives – A novel anticonvulsant drug classCan J Chem8320053745
- Kikkeri P. Harish A. Kikkeri N. Mohana N. Lingappa M. Synthesis of indazole substituted-1,3,4-thiadiazoles and their anticonvulsant activity; drug invention today 2013;5:9 2–9.
- Kikkeri P. Harish KN. Mohana A, et al. Synthesis of pyrazine substituted 1,3,4-thiadiazole derivatives and their anticonvulsant activity. Hindawi Publishing Corporation Organic Chemistry International; 2013. p. 8.
- Mohamed A. Adel G. Ahmed EL. Lingappa M. Synthesis and biological evaluation of some [1,2,4]Triazolo[4,3-a]quinoxaline derivatives as novel anticonvulsant agents. Hindawi Publishing Corporation Organic Chemistry; 2013. p. 7.
- S.RubaM.ArooG.NazIOSR J Pharm Biol Sci920141523
- Anonymous. Wavefunction. Inc., Spartan’14, version 1.1.2. Irvine, California, USA; 2013.
- O.TrottA.J.OlsonAutoDock Vina: improving the speed and accuracy of docking with a new scoring function, efficient optimization, and multithreadingJ Comput Chem312010455461
- G.M.MorrisR.HueyW.LindstromM.F.SannerR.K.BelewD.S.Goodsellet al.J Comput Chem3200927852791
- Accelrys Software Inc., Discovery Studio Visualizer 4.1, Accelrys Software Inc., San -Diego, CA, USA; 2013.
- Yap Chun Wei; Inc., PaDEL-Descriptor,version 2.18; 2011.
- V.RavinchandranH.RajakA.JainS.SivadasanC.P.VargheseR.Kishore-AgrawalValidation of QSAR models-strategies and importanceInt J Drug Des Discov22011511519
- R.H.MyersClassical and modern regression application2nd ed.1990Duxbury PressCA
- D.T.StantonP.C.JursDevelopment and use of charged partial surface area structural descriptors in computer assisted quantitative structure property relationship studiesAnal Chem62199023232329
- D.T.StantonS.DimitrovV.GrancharovO.G.MekenyanCharged partial surface area (CPSA) descriptors QSAR applicationsSAR and QSAR Environ Res1322002341351
- R.TodeschiniP.GramaticaNew 3D molecular descriptors: the WHIM theory and QSAR applicationsPerspect Drug Discovery Des1998355380
- H.IftikharS.BatoolA.DeepN.BalasubramanianC.S.PrabodhM.ManavIn silico analysis of the inhibitory activities of GABA derivatives on 4-aminobutyrate transaminaseArabian J Chem032013007