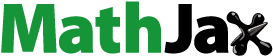
Abstract
In an attempt to find a potent inhibitor of antileukaemic activity, we performed two-dimensional quantitative structure–activity relationship studies. The QSAR models of the 1-(4-methoxyphenethyl)-1H-benzimidazole derivatives were developed by means of utilizing simulated annealing for the purpose of identifying an effective inhibitor. In the present study, the best 2D QSAR model developed had a cross-validated value of (q2) = 0.7584 and a coefficient of determination of (r2) = 0.8477 with partial least squares analysis. The models also suggest that the inclusion of fluorine, chlorine, methyl, and nitro substituents would enhance the antileukaemic activity. The resulting descriptors produced by the QSAR models were used to identify the physico-chemical features relevant to antileukaemic agents.
1 Introduction
Recently, multi-target drugs, which are designed as single molecules to modulate multiple physiological targets simultaneously, have increasingly attracted the attention of medicinal chemists [Citation1], and they represent a very promising way to enhance the efficacy and decrease the adverse effects of drugs, especially in the treatment of complex diseases such as cancers, cardiovascular diseases, and neurodegenerative diseases [Citation2,Citation3]. The leukaemias of childhood are common cancers of the hematopoietic system, primarily involving the malignant transformation of lymphoid progenitor cells and, less commonly, the transformation of myeloid progenitor cells [Citation4]. Cancer is now believed to result from the unlimited growth of a given cell [Citation5]. Cancer has been recognized as a disease of aberrant cellular proliferation, with traditional cancer therapies aiming to exploit the proliferation machinery. Cells die in two ways: necrosis and apoptosis. The regulation of apoptosis is crucial for development and sustained health [Citation6]. The dysregulation of apoptosis will result in a variety of clinical disorders, including cancer. The benzimidazole ring displays an important heterocyclic pharmacophore in drug discovery. Compounds carrying different substituents in the benzimidazole structure are associated with a wide range of biological activities, including anticancer [Citation7], antiviral [Citation8], antibacterial [Citation9], antifungal [Citation10], anti-inflammatory [Citation11], proton pump inhibitor [Citation12], antioxidant [Citation13], antihypertensive [Citation14] and anticoagulant [Citation15] properties.
Quantitative structure–activity relationship (QSAR) studies are mathematical equations that quantitatively correlate chemical structures with biological activity. These relationship models have been helpful in understanding the influence of molecular properties on the biological activity of different compounds, ultimately providing rational clues for the development of new compounds with desirable biological properties. Because they provide valuable information for molecular design and medicinal chemistry, QSAR studies have been widely used in drug design and discovery [Citation16].
To gain insight into the structural and molecular requirement influencing antileukaemic activity, we herein describe the QSAR analysis of the novel compound 1-(4-methoxyphenethyl)-1H-benzimidazole, focusing on the development of a QSAR models for antileukaemic activity. These results should serve as a guideline in designing compounds with more potent antileukaemic activity.
2 Materials and methods
2.1 Computational details
The computational studies were performed on a computer using HP Windows 7 Home Basic with an Intel® core processor. The molecular structures of the compounds in the data set were sketched using V-life MDS (Molecular Design Suite)™ 3.5 software, supplied by V-life Sciences Technologies Pvt. Ltd., Pune, India [Citation17].
2.2 Obtaining biological data and training and test sets
The QSAR studies were performed using a series of substituted 1-(4-methoxyphenethyl)-1H-benzimidazole analogues reported in the literature [Citation18]. Out of 22 molecules, four molecules were discarded for which the precise data were not available. The biological activities were represented by the IC50 divided by the mean IC50 in the two tumour cell lines K562 and CEM. The IC50 values were converted into the negative logarithm of pIC50 values and used as dependent variables in the QSAR analysis. The test compounds were selected considering the structural diversity and wide range of activity within data set. The chemical structures and their biological activities are represented in . The sphere exclusion method [Citation19] was adopted for division of the training and test data sets comprising 13 and five molecules, respectively, with a dissimilarity value of 1.0, where the dissimilarity value gives the sphere exclusion radius. The compounds in the test set allowed us to use one test compound over four training compounds, thus resulting in a more rigorous validation of the training model. Five compounds, namely, 3, 7, 11, 16 and 20, were used as the test set, while the remaining molecules were used as the training set.
Table 1 The structures of 1-(4-methoxyphenethyl)-1H benzimidazole-5-carboxylic acid derivatives with their K562 and CEM cells. .
2.3 Structure generation
The molecular structures of all of the molecules were sketched using the 2D builder module within VLife MDS 3.5 software, and the structures were then converted into the 3D space for further analysis. All of the compounds were batch optimized for the minimization of energies and optimization of geometry using Merck Molecular Force Field, followed by adjusting the parameters to include a distance-dependent dielectric constant of 1.0, convergence criterion or root mean square (RMS) gradient at 0.01 kcal/mol Å and an iteration limit of 10,000 [Citation20].
2.4 Descriptor calculation
A large number of theoretical 2D individual descriptors such as Chi, Path-cluster, Element Count, Estate number, Polar surface area, and alignment-independent topological descriptors have been computed from the chemical structures with an aim of identifying the structure/activity relationship of 1-(4-methoxyphenethyl)-1H-benzimidazole-5-carboxylic acid compounds which would, in turn, predict their biological activity.
The preprocessing of the independent variables (i.e., descriptors) was performed by removing invariable descriptors (constant column), which resulted in a total of 230 descriptors to be used for QSAR analysis. The descriptors having the same or almost the same value, or those that were highly correlated with other descriptors, were removed initially. The various alignment-independent (AI) descriptors [Citation21] were also calculated.
2.5 Cross validation
Models generated by 2D-QSAR studies were cross validated using a standard LOO procedure. The cross validated r2 (q2) value was calculated using Eq. Equation(1)(1)
(1) , where yi and ŷi are the actual and predicted activities of the ith molecule, respectively, and ymean is the average activity of all of the molecules in the training set [Citation22]. Because the calculation of the pairwise molecular similarities, and hence the predictions, were based upon the current trial solution, the q2 obtained is indicative of the predictive power of the current model. The q2 was calculated using Eq. Equation(1)
(1)
(1) .
(1)
(1)
The external predictive power of the model is assessed by predicting the pIC50 value of five test set molecules, which are not included in the QSAR model development. The predictive ability of the selected model is also confirmed by pred_r2.(2)
(2)
External validation of the generated models was carried out by predicting the activity of the test set of compounds. The predicted r2 (pred_r2) value was calculated using Eq. Equation(2)(2)
(2) , where yi and ŷi are the actual and predicted activities of the ith molecule in the test set, respectively, and ymean is the average activity of all of the molecules in the training set.
3 Results and discussion
For the development of QSAR models for 1-(4-methoxyphenethyl)-1H-benzimidazole-5-carboxylic acid derivatives analogues, PLS methodology was used based on the simulated annealing (K562 and CEM cell) feature selection method. The best 2D-QSAR models were chosen for discussion.
pIC50 (K562-cell line) = +0.9643 (±0.247) StNE-index +0.2487 (±0.0085) SddsN (nitro) count −0.3521 (±0.0483) T_N_O_1 + 0.7453 (±0.1574) SsNH2-index
Ntraining = 13, Ntest = 5, r2 = 0.8477, q2 = 0.7584, F test = 47.945, r2_se = 0.3965, q2_se = 0.3485, pred_r2 = 0.7855
The model-1 simulated annealing-PLS (SA-PLS) method can explain the coefficient of determination (r2), 84.7%, of the variance in the observed activity values. The cross-validated squared correlation coefficient (q2 = 0.7584) was 75%, and the predictivity for the external test set (pred_r2 = 0.7855) was approximately 78%. The model is validated by the values α_ran_r2 = 0.00369, α_ran_q2 = 0.001, α_ran_pred_r2 = 0.0001, best_ran_r2 = 0.015, best_ran_q2 = 0.02471, Zscore_ran_r2 = 0.0176 and Zscore_ran_q2 = 0.5234. The correlation matrix is shown in . Model-1 showed that evaluation of the descriptor SddsN (nitro) count suggests that the total number of nitro groups connected with one single and two double bonds at the R position will lead to improved activity. The presence of electron-withdrawing groups in compounds 1, 3, 12 and 14 resulted in highly active antileukaemic activity. The descriptors StNE-index and SsNH2Count (the total number of –NH2 groups connected with one single bond) suggest that the presence of cyano group and amino atoms (∼30) result in an increase in the activity at the R position in compounds 2, 4, 13 and 15, respectively. The contributing descriptor T_N_O_1 indicates that the presence of methoxy (compounds 5 and 16) at the R position of the ring would also affect the activity. The contribution chart for the QSAR model is shown in a and plots of the observed vs. predicted values of pIC50 are shown in b.
Table 2 Correlation matrix between descriptors present in the best QSAR model 1.
Fig. 1 (a) Contribution charts of the descriptors for the 2D model-1. (b) Graphs of observed vs. predicted activity of 2D model-1. (c) Contribution charts of the descriptors for the 2D model-2. (d) Graphs of observed vs. predicted activity of 2D model-2. (e) Contribution charts of the descriptors for the 2D model-3. (f) Graphs of observed vs. predicted activity of 2D model-3. (g) Contribution charts of the descriptors for the 2D model-3. (h) Graphs of observed vs. predicted activity of 2D model-4.

pIC50 (K562 cell line) = 0.6588 (±0.0531) SsOHcount +0.4985 (±0.0587) T_N_F_1 − 0.8753 (±0.1498) HOMO energy
Ntraining = 13, Ntest = 5, r2 = 0.7953, q2 = 0.7336, F test = 38.095, r2_se = 0.3487, q2_se = 0.3127, pred_r2 = 0.7069
Equation 2 explains 79% of the variance in activity with low standard error estimation. It also has an internal (q2) and external (pred_r2) predictive ability of ∼73 and ∼70%, respectively. The contribution chart of the selected descriptors is represented in c and plots of the predicted vs. observed values of pIC50 are shown in d. The QSAR model showed a positive contribution of the SsOHcount indices for the total number of -OH groups connected with one single bond and indicated that the activity was increased with the presence of hydroxy groups in 2nd to 4th position in the benzimidazole moiety. T_N_F_1 (∼27%) indicated that the presence of a fluorine atom (i.e., -F) at position site R on the nucleus (as in compounds 1, 2, 9, 12, 13, 18 and 20) would increase the antileukaemic activity. The descriptor of HOMO energy contributes negatively to the activity and substituent hydroxy and methoxy groups on the ring.
pIC50 (CEM cell line) = 0.3436 (±0.0195) smr +0.5985 (±0.0968) SsCH3Count −0.4874 (±0.0854) Mol. Wt
Ntraining = 13, Ntest = 5, r2 = 0.7487, q2 = 0.6853, F test = 28.5973, r2_se = 0.3653, q2_se = 0.3218, pred_r2 = 0.6542
The same data set subjected to the SA-PLS method resulted in a coefficient of correlation of 0.7487 and an internal predictive power of 68%, with an external predictivity of 65%. The descriptor smr (∼20%) suggests that an increase in the R position may lead to an increase in the activity. The descriptor SsCH3Count implies that a bulky hydrophobic group at the R position is favourable for the antileukaemic activity of the compound. The descriptor of mol. wt. indicates that the presence of a bulky group at the R position is detrimental for the antileukaemic activity. The contribution chart of the selected descriptors is represented in e and plots of the predicted vs. observed values of pIC50 are shown in f.
pIC50 (CEM) = 0.7453 (±0.0431) SssOE-index −0.5421 (±0.0665) SsBr-index + 0.4313 (±0.0321) T_2_C_2
Ntraining = 13, Ntest = 5, r2 = 0.7232, q2 = 0.6976, F test = 21.786, r2_se = 0.3198, q2_se = 0.3068, pred_r2 = 0.6637
SA-PLS model-4 reveals that the descriptor SsBr-index reveals that the number of bromines connected with one single bond (i.e., -Br) at the R position on the ring is detrimental for the antileukaemic activity (compounds 10 and 21, with substituents at the R positions producing good activity). SssOE-index, describing the number of oxygen atoms connected with two single bonds, indicated that the activity was increased with the presence of electron donating groups, and T_2_C_2 indicates that presence of substituents with direct attachment of carbon on the aromatic ring (i.e., -COOH) is favourable for the antileukaemic activity. The contribution chart of the selected descriptors is represented in g, and plots of the predicted vs. observed values of pIC50 are shown in h. The overall model is validated by predicting the biological activities of the test molecules, as indicated in .
Table 3 Observed and predicted activities (pIC50) for the training and test set compounds.
4 Conclusion
The present study describes the successful application of 2D-computational approaches to identify the essential structural requirements in 3D chemical space for the modulation of antileukaemic agents of substituted benzimidazole derivatives. The QSAR models suggest that -OCH3 and amine groups are beneficial, indicating that the presence of a bulky substituent at these positions should improve biological activity. The results of analyses for the descriptors SsOHcount and SssOE-index suggest that the hydroxy and methoxy properties of the molecules are responsible for the determination of their activities. At the R position, atoms such as H, F, Cl, Br, alkyl groups and aromatic rings were added. The contribution of each descriptor specifies the properties that should be present in the drug lead to enhance its activity. Further QSAR studies, along with these findings, will helpful for the design of 1-(4-methoxyphenethyl)-1H-benzimidazole-5-carboxylic acid derivatives as antileukaemic agents.
Conflict of interests
The authors declare that there is no conflict of interests regarding the publication of this paper.
Acknowledgements
The author wishes to express gratitude to V-life Science Technologies Pvt., Ltd. for providing the trial version software for the study.
Notes
Peer review under responsibility of Taibah University
References
- G.AlbertoB.DonatellaC.KonstantinC.ClaraD.S.AntonellaF.RobertaL.LorettaR.BarbaraT.PaoloMultitarget drugs: focus on the NO-donor hybrid drugsPure Appl. Chem.8020081693
- A.CavalliM.L.BolognesiA.MinariniM.RosiniV.TumiattiM.RecanatiniC.MelchiorreMulti-target-directed ligands to combat neurodegenerative diseasesJ. Med. Chem.5132008347372
- R.MorphyC.KayZ.RankovicFrom magic bullets to designed multiple ligandsDrug Discovery Today9152004641651
- D.S.PrasannaC.V.KavithaK.VinayaS.R.RanganathaS.C.RaghavanK.S.RangappaSynthesis and Identification of a new class of antileukemic agents containing 2-(arylcarboxamide)-(S)-6-amino-4,5,6,7-tetrahydrobenzo[d]thiazoleEur. J. Med. Chem.45201053315336
- P.NathalieL.J.NathalieD.DelphineP.NathalieF.DuboisReview of the contribution of radiolabelled tracers for tumour cell status imagingCurr. Med. Imaging Rev.22006193203
- V.NesterenkoK.J.PuttP.J.HergenrotherIdentification from a combinatorial library of a small molecule that selectively induces apoptosis in cancer cellsJ. Am. Chem. Soc.1254820031467214673
- J.MannA.BaronY.Opoku-BoahenE.JohanssonG.ParkinsonL.R.KellandS.NeidleA new class of symmetric bisbenzimidazole-based DNA minor groove-binding agents showing antitumor activityJ. Med. Chem.4422001138144
- T.IshidaT.SuzukiS.HirashimaK.MizutaniA.YoshidaI.AndoS.IkedaT.AdachiH.HashimotoBenzimidazole inhibitors of hepatitis C virus NS5B polymerase: identification of 2-[(4-diarylmethoxy) phenyl]-benzimidazoleBioorg. Med. Chem. Lett.167200618591863
- M.TunçbilekH.GökerR.ErtanR.EryigitE.KendiN.AltanlarSynthesis and antimicrobial activity of some new anilino benzimidazolesArch. Pharm. (Weinheim)330121997372376
- P.VenkatesanAlbendazoleJ. Antimicrob. Chemother.411998145147
- L.K.LabanauskasA.B.BrukstusP.G.GaidelisV.A.BuchinskaiteE.B.UdrenaiteV.K.DauksasSynthesis and anti-inflammatory activity of some new 1-acyl derivatives of 2-methylthio-5,6-diethoxybenzimidazolePharm. Chem. J.342004353355
- J.HornThe proton-pump inhibitors: similarities and differencesClin. Ther.222000266280
- G.Ayhan-KilcigilC.KuşT.CobanB.Can-EkeS.OzbeyM.IscanSynthesis, antioxidant and radical scavenging activities of novel benzimidazolesJ. Enzyme Inhib. Med. Chem.202005503514
- K.KuboY.KoharaY.YoshimuraY.InadaY.ShiboutaY.FurukawaT.KatoK.NishikawaT.NakaNonpeptide angiotensin II receptor antagonists. Synthesis and biological activity of potential prodrugs of benzimidazole-7-carboxylic acidsJ. Med. Chem.3616199323432349
- W.W.MederskiD.DorschS.AnzaliJ.GleitzB.CezanneC.TsaklakidisHalothiophene benzimidazoles as P1 surrogates of inhibitors of blood coagulation factor XaBioorg. Med. Chem. Lett.1414200437633769
- A.NargotraS.KoulS.SharmaI.A.KhanA.KumarN.ThotaJ.L.KoulS.C.TanejaG.N.QaziQuantitative structure–activity relationship (QSAR) of aryl alkenyl amides/imines for bacterial efflux pump inhibitorsEur. J. Med. Chem.442009229238
- VLIFE MDSSoftware package, version 3.5, supplied by V life science technologies Pvt. Ltd, Pune2008
- N.R.Thimme GowdaC.V.KavithaK.K.ChiruvellaO.JoyK.S.RangappaS.C.RaghavanSynthesis and biological evaluation of novel 1-(4-methoxyphenethyl)-1H-benzimidazole-5-carboxylic acid derivatives and their precursors as antileukemic agentsBioorg. Med. Chem. Lett.19200945944600
- A.GolbraikhA.TropshaPredictive QSAR modeling based on diversity sampling of experimental datasets for the training and test set selectionJ. Comput. Aided Mol. Des.162002357369
- T.A.HalgrenMerck molecular force field. II. MMFF94 van der Waals and electrostatic parameters for intermolecular interactionsJ. Comput. Chem.171996520552
- K.BaumannAn alignment-independent versatile structure descriptor for QSAR and QSPR based on the distribution of molecular featuresJ. Chem. Inf. Model.4220022635
- R.D.CramerD.E.PattersonJ.D.BunceComparative molecular field analysis (CoMFA) 1. Effect of shape on binding of steroids to carrier proteinsJ. Am. Chem. Soc.110199859595967