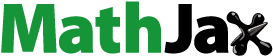
Abstract
Cancer is a cellular disease characterized by the progressive, persistent, abnormal, purposeless, and uncontrolled proliferation of tissues. 2D-QSAR was performed on a series of N,1,3-triphenyl-1H-pyrazole-4-carboxamides as anticancer agents to gain insights into the structural determinants and their structure–activity relationship. The 2D-QSAR model using 20 molecules in the training set gave an r2 value of 0.8059 and a pred_r2 value of 0.7861. In models, it is shown that bulky electron-withdrawing substituents at the R1 and R2 position of the chain are favoured for inhibitory activity. Hence the model proposed in this work provides important structural insights into designing novel pyrazole-4-carboxamide derivatives with specific Aurora A kinase inhibition.
1 Introduction
The mammalian Aurora kinases comprise a family of serine/threonine kinases that are essential for coordinated mitotic progression [Citation1]. As a potential target in anticancer therapy, these kinases have generated significant interest in the cancer research field due to their elevated expression profiles in many human cancers [Citation2]. Safe and effective anticancer agents are still one of the challenges in the field of drug discovery. Small molecule kinase inhibitors have been successfully introduced into the drug market as selective anticancer agents with lower side effects [Citation3]. The Aurora kinase family consists of highly homologous Ser/Thr protein kinases, which play a key role in regulating mitosis. Three members of the Aurora kinase family have been identified up to now in mammalian cells, namely Aurora A, B and C [Citation4–Citation6]. The biological roles of Aurora A and B have been identified, and the role of Aurora C is still unclear. Aurora kinases are strongly associated with cancer and are frequently detected to be over-expressed in a wide range of human tumours [Citation7]. The expression and activity of Aurora kinases are tightly associated with the cell cycle. The two major Aurora kinases, Aurora A and B, have distinct roles in mitosis. Along with its cellular binding partner TPX2 (target protein of Xenopus kinesin-like protein 2), Aurora A plays an essential role in mitotic spindle formation, centrosome maturation, and segregation; meanwhile, Aurora B is involved in chromosomal condensation, alignment and separation as well as kinetochore-microtubule attachment and cytokinesis with its INCENP binding partners [Citation8–Citation12]. Aurora C is required for spermatogenesis, and it may complement the functions of Aurora B; however, its function in cell cycle progression is still unknown [Citation13]. Aurora A kinase plays an important role in mitosis. It is associated with centrosome maturation and separation and thereby regulates spindle assembly and stability [Citation7]. Over-expression or deregulation of Aurora A has been associated with a high occurrence of cancer [Citation14]. Since its discovery, Aurora A kinase has been identified as a colon cancer-associated kinase that is over-expressed in a wide range of human tumours such as breast, colorectal, ovarian and glioma. [Citation15–Citation17] Thus, targeted inhibition of Aurora A kinase has become an attractive therapeutic strategy in cancer treatment, and more than 10 Aurora inhibitors have entered into early clinical assessment [Citation18]. Currently, cancer is the most dominant cause of death worldwide [Citation19,Citation20]. Apoptosis is a cellular process that organisms use for the digestion of excessive cells to control cell numbers. In recent years, a number of small-molecule inhibitors of Aurora kinase, with wide structural diversity, have been reported. Among these compounds, clinical trials have shown that ZM447439, VX-680, PHA-739358 and other potential inhibitors suppress the growth of tumour cells both in-vitro and in-vivo [Citation21]. Quantitative structure–activity relationship (QSAR) models have been demonstrated to be an effective computational tool in understanding the correlation between the structures of molecules and their activities [Citation22,Citation23]. In a QSAR study, one seeks to find a mathematical relationship between the activity and one or more descriptors. Using this approach, scientists could predict the activities of a series of newly designed drugs before making the final decision on whether or not to synthesize and assay them. The prediction is based on the structural descriptors of the molecular features that account for variations in the biological activity to the greatest degree [Citation22]. To investigate the key structural features required to design potential Aurora A inhibitor drug candidates from pyrazole-4-carboxamide derivatives [Citation24], in the current approach, an attempt has been made to understand the structural and physicochemical requirements of a set of Aurora A kinase inhibitors through the help of a regression QSAR method.
2 Experimental
2.1 Data set
A dataset comprised of 25 pyrazole-4-carboxamide derivatives was used in the present study. All compounds and associated data were taken from the literature [Citation24] for the development of the QSAR models. The Aurora A kinase inhibitory activity data were reported as IC50 values in the same biological assay conditions. The dataset consists of some highly active and inactive compounds along with some compounds with moderate activities. The IC50 (μM) values reported in the literature were converted to negative logarithmic values to get pIC50 values, which were used for the QSAR study. The structures of these inhibitors and their pIC50 values are given in .
Table 1 Structures and activity of pyrazole-4-carboxamide derivatives as anticancer agents..
The sphere exclusion method [Citation25] was divided into a training set and a prediction set. The training set was comprised of 20 compounds. The test set consists of five compounds, which are randomly selected and structurally diverse molecules possessing a wide range of activities. The IC50 values employed in this work were measured under the same experimental conditions, a fundamental requirement for QSAR studies. We have used some 2D-descriptors to demonstrate the relationship between the structural properties and activity.
2.2 Generation of structures
The three-dimensional structures of these compounds were built by using the Sketch Molecule module in the VLife science molecular modelling package [Citation26]. Structural energy minimization was performed using the standard Merck molecular force field (MMFF), and the maximum number of iterations for the minimization was set to 1000. The minimization was terminated when a distance-dependent dielectric constant of 1.0 and the convergence criterion of 0.01 kcal/mol Å were reached [Citation27].
2.3 2D-QSAR studies
A large number of theoretical 2D individual descriptors such as the molar refractivity (MR), molecular weight (Mol wt.), partition coefficient (LogP), volume, XlogP, and smr; physiochemical descriptors such as the estate numbers, element count, dipole moment, XlogP, and SlogP; and the topological type have been computed. The E-state descriptor is calculated for each atom (e.g., OH, N, and Cl) and also separately for each H-atom. Physicochemical descriptors including 239 physicochemical parameters, 700 alignment type parameters and 99 atoms type count descriptors were calculated. The QSAR worksheet was generated using biological activity as the dependent variable and various 2D descriptors as independent variables. The data set was divided into a training set and a test set. In this study, more than 300 calculated descriptors (2D) were subjected to partial least squares coupled with a stepwise forward–backward variable selection method in order to establish a correlation between the physicochemical parameters and activity. The QSAR model is considered to be predictive if the following conditions are satisfied: r2 > 0.6, q2 > 0.6 and pred_r2 > 0.5 [Citation25].
2.4 Cross validation
To calculate q2, each molecule in the training set was sequentially removed, the model was refit using the same descriptors, and the biological activity of the removed molecule was predicted using the refit model [Citation28]. The q2 was calculated using Eq. Equation(1)(1)
(1) .
(1)
(1) where yi and
are the actual and predicted activities of the ith molecule in the training set, respectively, and ymean is the average activity of all of the molecules in the training set. For external validation, the activity of each molecule in the test set was predicted using the model generated from the training set. The pred_r2 value is calculated as follows (Eq. Equation(2)
(2)
(2) ):
(2)
(2) where yi and
are the actual and predicted activities of the ith molecule in the test set, respectively, and ymean is the average activity of all of the molecules in the training set. Thus, the pred_r2 value is indicative of the predictive power of the current model based on the external test set.
3 Results and discussion
A series of triphenyl-1H-pyrazole-4-carboxamide derivatives as anticancer agents was selected for QSAR studies. QSAR investigations of the triphenyl-1H-pyrazole-4-carboxamide derivative series resulted in several QSAR equations, considering the term selection criterion as r2, q2 and pred_r2. In the present study, training and test sets were generated using the sphere exclusion method followed by partial least squares regression coupled with a stepwise forward–backward variable selection method.pIC50 (HCT116-cell-lines) = 0.6457 (±0.2833); SsCH3E-index, +0.1137 (±0.0150); SsBrE-index, +0.0345 (±0.0016); T_2_Cl_1, −0.1743 (±0.0257); 2PathCount
N = 25, r2 = 0.8059, q2 = 0.7621, F test = 37.8701, r2_se = 0.3197, q2_se = 0.3738, pred_r2 = 0.7861
2D-QSAR model 1 shows a coefficient of determination (r2) of 0.8059 and a cross-validated correlation coefficient (q2) of 0.7621. shows the correlation matrix for the descriptors used in generating Eq. Equation(1)(1)
(1) . a shows the contribution chart representing the contributions of descriptors in the 2D-QSAR model developed by the PLS method. The descriptors contribute to the 2PathCount parameter, which can signify the total number of fragments with an order of two (two bond path). It may be inferred that increasing the branching of the compound is favourable for anticancer activity. The presence of the descriptor SsBrE-index [signifies the electrotopological indices and the number of bromine atoms connected with one single bond] showed that an increase in the value of this descriptor is beneficial for the activities of the pyrazole-4-carboxamide derivatives. Compounds 3, 8, 13, 18, and 23 bearing a relatively bromo-substituent at the R2 position were the potential derivatives. The next important descriptor is the SsCH3E-index indices for the number of eCH3 groups connected with one single bond, and it showed an increase in the activities of the pyrazole-4-carboxamide derivatives with the presence of a methyl group at the R1 position, such as in compounds 6, 7, 8, 9 and 10. The present QSAR model reveals that Baumann’s alignment independent topological descriptor T_2_Cl_1 has a major contribution, which means that the count of the number of double bonds separated from a chlorine atom by single bond distances in a molecule indicates that the activity will increase with the presence of a relative chloro-substituent at the R1 and R2 positions (compounds 2, 7, 12, 16, 17, 18, 19, 20 and 22). Further optimization of the phenyl ring of inhibitor compounds using common aromatic constants showed electron-withdrawing substituents at the ortho position to be essential for Aurora A kinase inhibition. A preliminary analysis with pyrazole-4-carboxamide derivatives identified certain structural features, such as Cl, OCH3, and CH3 groups around the pyrazole ring, as the most favourable substitution for Aurora A kinase inhibition. The plots of observed vs. predicted values of pIC50 are shown in b.pIC50 (HCT116-cell-lines) = 0.1200 (±0.0159); SssCH2count, +0.3605 (±0.0174); SsFcount, +0.0449 (±0.0077); hydrogen count −0.3918 (±0.0862) SlogP
Table 2 Correlation matrix between descriptors present in the best QSAR model-1(HCT116-cell-lines).
Fig. 1 (a) Contribution charts of the descriptors for the 2D model-1. (b) Plot of observed versus predicted activity by best 2D QSAR model 1. (c) Contribution charts of the descriptors for the 2D model-2. (d) Plot of observed versus predicted activity by best 2D QSAR model 2. (e) Contribution charts of the descriptors for the 2D model-3. (f) Plot of observed versus predicted activity by best 2D QSAR model 3. (g) Contribution charts of the descriptors for the 2D model-4. (h) Plot of observed versus predicted activity by best 2D QSAR model-4. (i) The designed scaffold new chemical entity.
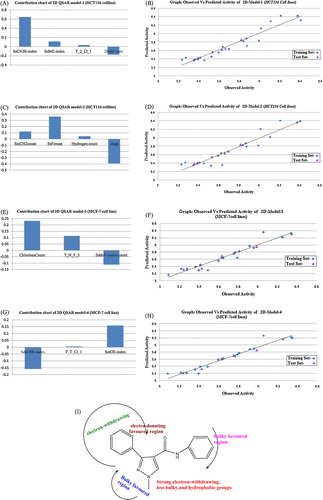
N = 25, r2 = 0.7759, q2 = 0.6784, F test = 13.1278, r2_se = 0.5314, q2_se = 0.5874, pred_r2 = 0.7061
Model 2 produced from the training set obtained from the sphere exclusion method shows r2, q2, and pred_r2 values much lower than model 1. The descriptors SlogP signify the log of the octanol/water partition coefficient compounds. In the model, the lipophilicity suggests that a bulky substituent would be favourable at the R1 position. The model reveals that the descriptor SssCH2count suggests that the activity of pyrazole-4-carboxamide derivatives may be increased by increasing the number of –CH2 groups present in the molecules. The model indicated the SsFcount of the total number of fluorine atoms connected with a single bond, suggesting that the activity of pyrazole-4-carboxamide derivatives may be increased by increasing the number of fluorine atoms present in the moiety at the R2 position. The hydrogen count descriptor suggests that an increased number of hydrogen atoms will increase the activity. The contribution chart of selected descriptors is represented in c, and plots of the observed vs. predicted values of pIC50 are shown in d. The observed and predicted activity values of the training and test sets of compounds selected for the model are given in .pIC50 (MCF-7-cell-lines) = 0.2323 (±0.0273); chlorine count, +0.1138 (±0.0173); T_N_F_3, −0.1118 (±0.0097), SddsN (nitro) count
Table 4 Comparative observed and predicted activities of carboxamide derivatives.
N = 25, r2 = 0.7601, q2 = 0.7188, F test = 39.7513, r2_se = 0.4921, q2_se = 0.4608, pred_r2 = 0.6821
Model 3 shows a good internal predictive power (q2 = 0.7188) of 72% and a predictivity for the external test set (pred_r2 = 0.6821) of approximately 68%. The descriptor chlorine count, which is the number of chlorine atoms connected with a single bond, showed that an increase in the values of this descriptor would be beneficial for the activity of the MCF-7 cell lines. SddsN (nitro) count suggests that the presence of a nitro group at the R1 position revealed that a bulky substituent at this site would increase the activity. The alignment independent descriptor T_N_F_3 (nitrogen atoms separated from any other fluorine atom by three bonds) showed that an increase in the value of this descriptor is beneficial for the activity. Compounds with an electron-withdrawing group at R1 and R2 exhibited significantly improved potencies compared to derivatives bearing an electron-donating methyl substituent at R1. shows the correlation matrix for the descriptors used in generating model-3. The contribution chart of selected descriptors is represented in e, and plots of the observed vs. predicted values of pIC50 are shown in f.pIC50 (MCF-7-cell-lines) = 0.1586 (±0.0373); SdsCHE-index, +0.0052 (±0.0017); T_T_Cl_1, +0.1586 (±0.0611); SssOE-index
Table 3 Correlation matrix between descriptors present in the QSAR model-3(MCF-7-cell-lines).
N = 25, r2 = 0.7669, q2 = 0.6933, F test = 10.0479, r2_se = 0.4658, q2_se = 0.5061, pred_r2 = 0.6217
The significant model 4 using the PLS method has 0.7669 as the coefficient of determination (r2), an internal predictive power (q2 = 0.6933) of 69% and a predictivity for the external test set of approximately 62%. From the above equation, the alignment independent descriptor T_T_Cl_1 contributes positively (approximately 14%) for the activity, which corresponds to the number of double bonds separated from a chlorine atom by a single bond distance. A pyrazole-4-carboxamide moiety at the R1 or R2 position suggests that a less bulky group at this position would benefit the potency of the compound. The SdsCHE-index descriptor indicates the presence of a number of –CH groups connected with one double and one single bond, showing an increase in the R1 position of the pyrazole-4-carboxamide nucleus and suggesting the presence of alkyl group at the R1 position. The descriptor SssOE-index indicated that the activity will increase with the presence of methoxy groups at the R1 position such as in compounds 11,12,13,14 and 15. The contribution chart of selected descriptors is represented in g, and plots of the observed vs. predicted values of pIC50 are shown in h.
4 Conclusion
In this paper, we propose a new method for the generation of 2D-QSAR models for the prediction of activity values of chemicals. In the above discussion, 2D-QSAR models identified the important structural requirements for triphenyl-1H-pyrazole-4-carboxamide derivatives exhibiting activity against Aurora A kinase. The detailed analysis of these four models enabled us to better understand the structure activity relationship of pyrazole-4-carboxamide derivatives. The QSAR models indicate that the descriptors of the SssCH2count, SsFcount, chlorines count, SddsN (nitro) count, T_T_Cl_1, T_N_F_3 and SssOE-index influenced the activity. The models indicated that a chlorine or methoxy substituent at the R1 site would benefit the activity of the compound. All of the derivatives involved in the present study possessed a halogen substituent (e.g., chloro-, bromo) at R1 (i). The results of the present study may be useful in the design of more potent Aurora A kinase inhibitors that may be used as potential anticancer agents.
Acknowledgement
The author wishes to express gratitude to V-life Science Technologies, Pvt. Ltd. for providing the software for the study.
Notes
Peer review under responsibility of Taibah University.
References
- M.LiA.JungU.GanswindtP.MariniA.FriedlP.T.DanielK.LauberV.JendrossekC.BelkaAurora kinase inhibitor ZM447439 induces apoptosis via mitochondrial pathwaysBiochem. Pharmcol.792010122129
- G.MountziosE.TerposM.A.DimopoulosAurora kinases as targets for cancer therapyCancer Treat. Rev.342008175182
- J.ZhangP.L.YangN.S.GrayTargeting cancer with small molecule kinase inhibitorsNat. Rev. Cancer920092839
- J.FuM.BianQ.JiangC.ZhangRoles of Aurora kinases in mitosis and tumorigenesisMol. Cancer Res.52007110
- P.MeraldiR.HondaE.A.NiggAurora kinases link chromosome segregation and cell division to cancer susceptibilityCurr. Opin. Genet. Dev.1420042936
- H.KatayamaS.SenAurora kinase inhibitors as anticancer moleculesBiochim. Biophys. Acta17992010829839
- H.KatayamaW.R.BrinkleyS.SenThe Aurora kinases: role in cell transformation and tumorigenesisCancer Metast. Rev.222003451464
- A.H.KishoreB.M.VedamurthyK.MantelinguS.AgrawalB.A.A.ReddyS.RoyK.S.RangappaT.K.KunduSpecific small-molecule activator of Aurora kinase A induces autophosphorylation in a cell-free systemJ. Med. Chem.512008792797
- T.E.RawsonM.RüthE.BlackwoodD.BurdickL.CorsonJ.DotsonJ.DrummondC.FieldsG.J.GeorgesB.GollerJ.HalladayT.HunsakerT.KleinheinzH.W.KrellJ.LiJ.LiangA.LimbergA.McNuttJ.MoffatG.PhillipsY.RanB.SafinaM.UltschL.WalkerC.WiesmannB.ZhanA.ZhouB.Y.ZhuP.RügerA.G.CochranA pentacyclic aurora kinase inhibitor (AKI-001) with high in vivo potency and oral bioavailabilityJ. Med. Chem.51200844654475
- S.HeS.YangG.DengM.LiuH.ZhuW.ZhangS.YanL.QuanJ.BaiN.XuAurora kinase A induces miR-17-92 cluster through regulation of E2F1 transcription factorCell. Mol. Life Sci.67201020692076
- F.H.JungG.PasquetC.Lambert-van der BremptJ.J.LohmannN.WarinF.RenaudH.GermainC.De SaviN.RobertsT.JohnsonC.DoussonG.B.HillA.A.MortlockN.HeronR.W.WilkinsonS.R.WedgeS.P.HeatonR.OdedraN.J.KeenS.GreenE.BrownK.ThompsonS.BrightwellDiscovery of novel and potent thiazoloquinazolines as selective Aurora A and B kinase inhibitorsJ. Med. Chem.492006955970
- D.FancelliJ.MollM.VarasiR.BravoR.ArticoD.BertaS.BindiA.CameronI.CandianiP.CappellaP.CarpinelliW.CrociB.ForteM.L.GiorginiJ.KlapwijkA.MarsiglioE.PesentiM.RocchettiF.RolettoD.SeverinoC.SonciniP.StoriciR.TonaniP.ZugnoniP.Vianello1,4,5,6-tetrahydropyrrolo[3,4-c]pyrazoles: identification of a potent Aurora kinase inhibitor with a favorable antitumor kinase inhibition profileJ. Med. Chem.49200672477251
- S.WangC.A.MidgleyF.ScaërouJ.B.GrabarekG.GriffithsW.JacksonG.KontopidisS.J.McClueC.McInnesC.MeadesM.MeznaA.PlaterI.StuartM.P.ThomasG.WoodR.G.ClarkeD.G.BlakeD.I.ZhelevaD.P.LaneR.C.JacksonD.M.GloverP.M.FischerDiscovery of N-phenyl-4-(thiazol-5-yl)pyrimidin-2-amine aurora kinase inhibitorsJ. Med. Chem.53201043674378
- R.CraneB.GadeaL.LittlepageH.WuJ.V.RudermanAurora A, meiosis and mitosisBiol. Cell962003215229
- M.S.CoumarJ.S.LeouP.ShuklaJ.S.WuA.K.DixitW.H.LinC.Y.ChangT.W.LienU.K.TanC.H.ChenJ.T.HsuY.S.ChaoS.Y.WuH.P.HsiehStructure-based drug design of novel Aurora kinase A inhibitors: structural basis for potency and specificityJ. Med. Chem.5220091050
- M.ZhongM.BuiW.ShenS.BaskaranD.A.AllenR.A.EllingW.M.FlanaganA.D.FungE.J.HananS.O.HarrisS.A.HeumannU.HochS.N.IvyJ.W.JacobsS.LamH.LeeR.S.McDowellJ.D.OslobH.E.PurkeyM.J.RomanowskiJ.A.SilvermanB.T.TangonanP.TavernaW.YangJ.C.YoburnC.H.YuK.M.ZimmermanT.O’BrienW.Lew2-Aminobenzimidazoles as potent Aurora kinase inhibitorsBioorg. Med. Chem. Lett.17200951585161
- K.J.MoriartyH.K.KoblishT.GarrabrantJ.MaisuriaE.KhalilF.AliI.P.PetrouniaC.S.CryslerA.C.MaroneyD.L.JohnsonR.A.GalemmoJr.The synthesis and SAR of 2-amino-pyrrolo[2,3-d]pyrimidines: a new class of Aurora-A kinase inhibitorsBioorg. Med. Chem. Lett.16200657785783
- S.HowardV.BerdiniJ.A.BoulstridgeM.G.CarrD.M.CrossJ.CurryL.A.DevineT.R.EarlyL.FazalA.L.GillM.HeathcoteS.MamanJ.E.MatthewsR.L.McMenaminE.F.NavarroM.A.O’BrienM.O’ReillyD.C.ReesM.ReuleD.TisiG.WilliamsM.VinkovićP.G.WyattFragment-based discovery of the pyrazol-4-yl urea (AT9283), a multitargeted kinase inhibitor with potent aurora kinase activityJ. Med. Chem.522009379388
- H.VarmusThe new era in cancer researchScience312200611621165
- D.G.KingstonD.J.NewmanTaxoids: cancer-fighting compounds from natureCurr. Opin. Drug Discov. Dev.102007130144
- C.DitchfieldV.L.JohnsonA.TigheR.EllstonC.HaworthT.JohnsonA.MortlockN.KeenS.S.TaylorAurora B couples chromosome alignment with anaphase by targeting BubR1, Mad2, and Cenp-E to kinetochoresJ. Cell Biol.1612003267280
- H.DuZ.HuA.BazzoliY.ZhangPrediction of inhibitory activity of epidermal growth factor receptor inhibitors using grid search-projection pursuit regression methodPLoS ONE67201122367
- N.FrimayantiL.V.SanghiranS.M.ZainH.A.WahabN.A.Rahman2D, 3D-QSAR, and pharmacophore studies on thiazolidine-4-carboxylic acid derivatives as neuraminidase inhibitors in H3N2 influenza virusMed. Chem. Res.23201414471453
- X.LiX.LuM.XingX.H.YangT.T.ZhaoH.B.GongH.L.ZhuSynthesis, biological evaluation, and molecular docking studies of N,1,3-triphenyl-1H-pyrazole-4-carboxamide derivatives as anticancer agentsBioorg. Med. Chem. Lett.2211201235893593
- A.GolbraikhA.TropshaPredictive QSAR modeling based on diversity sampling of experimental datasets for the training and test set selectionJ. Comput. Aided Mol. Des.162002357369
- VLife MDS 3.52008Vlife Sciences Technologies, Pvt. Ltd.Pune, India
- T.A.HalgrenMerck molecular force field. III. Molecular geometries and vibrational frequencies for MMFF94J. Comput. Chem.171996553586
- R.D.CramerIIIJ.D.BunceD.E.PattersonCross validation, bootstrapping, and partial least squares compared with multiple regression in conventional QSAR studiesQuant. Struct. Act. Relat.719881825