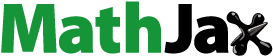
Abstract
The timing of seedling emergence greatly affects growth and yield of wheat (Triticum aestivum L.) and a good growth model should predict it accurately. The Cropping System Model of the Decision Support System for Agrotechnology Transfer (DSSAT-CSM) is used worldwide for many different applications, but its simulation of the timing of seedling emergence of wheat is not satisfactory under certain circumstances. In order to improve the prediction of seedling emergence, we incorporated a newly developed non-linear model, the Beta model, into DSSAT-CSM. Simulation performances were tested using observations in spring wheat (cv. Thatcher) from 24 sites across North America over the period 1930–1954, which totalled 244 site-years. Observed days from sowing to 50% seedling emergence (DSE) ranged from 5 to 39. The DSSAT-CSM model underestimated DSE in most cases. The Beta model using daily air temperature markedly improved prediction of seedling emergence. When using hourly air temperature, the Beta model generally resulted in predictions similar to when daily air temperature was used. However, calculated hourly temperature improved the simulation when the daily air temperature was near the base temperature for germination/emergence. When temperature was adjusted using a DSSAT-CSM-calculated soil moisture factor for germination/emergence (WFGE), the prediction was not improved, which could be related to the inaccurate simulation of near-surface soil moisture and the calculation of WFGE. The performance of the Beta model using soil temperature at sowing depth was not as good as simulations using air temperature, suggesting that the simulated soil temperature might not have been accurate. To further improve the prediction it is necessary to improve the simulation of near-surface soil moisture and temperature and the calculation of WFGE. Further work could also be done to simulate the dynamics of seedling emergence.
1 Introduction
The timing of seedling emergence is an important factor in determining phenological development, growth and grain yield of wheat (Triticum aestivum L.) [Citation1,Citation2]. It is important for farmers who manage large areas of land to arrange field operations, such as herbicide application. The accuracy of emergence prediction is also crucial for the performance of growth models. Although there are many biotic and environmental factors affecting the time of seedling emergence, temperature is the most important one [Citation3–Citation6]. The thermal time (TT; degree days – °C day) required for emergence is often considered to be constant and many researchers [Citation7–Citation10] think that the rate of emergence increases linearly with an increase in temperature between the base and optimum temperatures. However, some studies found that the response of the development rate of plants to temperature is non-linear [Citation11,Citation12]. Various non-linear functions such as power-law [Citation13], exponential [Citation14], quadratic [Citation15], logistic [Citation16], Weibull [Citation15,Citation17], Gompertz [Citation2], double Gompertz [Citation18], monomolecular [Citation19], and Chapman–Richards [Citation20] have been used to describe the environmental response of developmental processes in plants. However, none of these functions can describe the concave curvature of developmental processes near the base temperature or the decline of development rate at supra-optimal temperatures [Citation21]. Some authors have used the Beta model because of its ability to describe development rate over the full range of temperatures, its flexibility to take different shapes and its mathematical tractability [Citation21–Citation24]. Jame and Cutforth [Citation3] used a series of Beta functions to describe effects of temperature on germination, subcrown internode elongation and coleoptile elongation. The parameterized model with data from previous studies predicted the time required for seedling emergence reasonably well and agreed well with the observed times under several controlled environments and field conditions [Citation3].
The Decision Support System for Agrotechnology Transfer-Cropping System Model (DSSAT-CSM) is used successfully worldwide for many different applications [Citation25]. We are currently using DSSAT-CSM to predict impacts of climate change on the production of cereal crops in Western Canada. Our preliminary study showed that the simulation of the time of seedling emergence in wheat by DSSAT-CSM was not satisfactory. The objective of this study was to test the Beta model for its adequacy in predicting the time to 50% seedling emergence for spring wheat under various environments in North America. The Beta model was incorporated into DSSAT-CSM and was run using observed daily air temperature, calculated hourly temperature or simulated soil temperature at sowing depth, with or without including the effect of the DSSAT-CSM-simulated moisture stress factor. Simulation results were compared with those simulated by the seedling emergence module of DSSAT-CSM. If the Beta model can better predict the time to 50% seedling emergence in wheat, it will be used to replace the seedling emergence module of DSSAT-CSM to improve the model's performance.
2 Material and methods
2.1 Observations on seedling emergence
From 1930 to 1954, phenological development, including seedling emergence, heading and maturity of a hard red spring wheat was observed at 32 sites across North America [Citation26]. Among them were 24 sites where emergence was recorded for more than 1 year and weather data were available either on-site or within 100 km radius from the site. These 24 sites totalled 244 site-years of wheat emergence observations that were used for this study ().
Table 1 Information on the Canada and USA sites used in this study.
The wheat cultivar grown was Thatcher, which is one of the first cultivars specifically bred for stem rust resistance [Citation27] and has been widely used as a resistant parent in subsequent breeding programmes in North America [Citation28–Citation30]. Other physiological characteristics of Thatcher are standard height [Citation31], vernalization insensitive [Citation32], photoperiod sensitive [Citation33] and relatively short green flag leaf duration [Citation34].
Because some important information related to modelling was not reported [Citation12], the following assumptions were made: (1) seedling emergence was defined to occur when 50% of the plant population had emerged. This criterion has often been used by others to determine the time of this phenological stage [Citation35–Citation38]; (2) the management was a fallow–wheat rotation under conventional tillage, where soil moisture was recharged during the fallow season to ensure adequate yields [Citation39]; (3) the sowing depth was the same on all sites; and (4) no fertilizer was applied as soil fertility was thought to be adequate at that time.
2.2 Weather and soil data
Temperatures and precipitation for the United States of America were downloaded from the United States Historical Climatic Network available on the National Climatic Data Centre website [Citation40] and Canadian meteorological data were downloaded from the Agriculture and Agri-Food Canada (AAFC) Daily Climate Information website [Citation41]. Daily solar radiation was calculated using the Mountain Climate Simulator [Citation42,Citation43]. Hourly air temperatures were calculated from maximum and minimum air temperatures and day length, using a model described by Parton and Logan [Citation44]. This model, which is implemented in the subroutine HTEMP of DSSAT-CSM, uses a truncated sine wave to predict daytime temperature changes and an exponential function to predict night temperatures. Comparison of this model with other models showed that it did a superior job of fitting the data with a smaller number of parameters [Citation44]. Soil physical and chemical properties required by DSSAT-CSM were downloaded from the United States Department of Agriculture Natural Resources Conservation Service soil survey characterization data website [Citation45] and the AAFC Soil Landscapes of Canada website [Citation46].
3 The DSSAT-CSM model
The DSSAT-CSM package (version 4.0) is a collection of independent programmes including 16 crop models and a set of modules for the simulation of soil temperature, water, carbon and nitrogen balances. It also has tools and utility programmes for managing data on soil, climate, genetics, crops, economics, and pests; and has application and analysis programmes. The plant life cycle in the wheat growth module of DSSAT-CSM is divided into several phases. Rate of development is governed by thermal time and day length. Daily plant growth was computed by converting daily intercepted photosynthetically active radiation into plant dry matter. Kernel numbers were computed based on the cultivar's genetic potential, growth and the environment. The grain growth rate was calculated based on the potential rate, assimilate availability and temperature.
The soil–plant–atmosphere module [Citation47] brings together soil, plant and atmospheric inputs and computes light interception by the canopy, potential evapotranspiration, actual soil evaporation and plant transpiration. The potential evapotranspiration can be calculated by three different methods: Priestley–Taylor [Citation48] modified by Ritchie [Citation47,Citation49], Penman–FAO24 [Citation50,Citation51] and the Penman–Monteith reference method (FAO56) [Citation52,Citation53]. The latter was recently recommended by FAO and International Commission on Irrigation and Drainage working groups [Citation54]. Many studies indicated that FAO56 was better than the other two modules for calculating evapotranspiration and resulted in better simulations on biomass and yield [Citation55–Citation61]. The soil moisture module is a one-dimensional model that computes the daily changes in soil moisture content by soil layer due to infiltration, drainage, unsaturated flow and evapotranspiration [Citation62,Citation63]. Drainage is first calculated based on a soil drainage parameter. The amount of water passing through any layer is then compared with the saturated hydraulic conductivity of that layer. If the saturated hydraulic conductivity of any layer is less than the computed vertical drainage through that layer, actual drainage is limited to the conductivity value, and water accumulates above that layer [Citation64]. More details of these modules were described by Jones et al. [Citation25].
The germination/emergence of wheat was simulated by the CROPSIM-CERES subroutine, using cumulative germination/emergence units (GEU, °C day), which is calculated by the following equation:where WFGE is the water factor for germination/emergence (0–1). The thermal time (TT – degree days) is calculated by comparing the mean daily air temperature (Ta) with the base (Tb), optimum (To) and maximum (Tm) temperatures for development:
The default values of Tb, To and Tm for wheat were 0, 26 and 50 °C, respectively. The water factor for germination/emergence is calculated as:
(3)
(3) where SWPSD is the soil moisture status at sowing depth and WFGEU is the upper limit of the water factor for germination/emergence (0.5 for wheat). The soil moisture status at sowing depth is calculated by:
(4)
(4) where W1 and W2 are the relative soil moisture contents for the soil layer in which the seeds are buried and the lower layer, respectively, and SDEPTH is sowing depth (cm). The relative soil moisture content is calculated by the following equation:
(5)
(5) where SW, LL and DUL are volumetric moisture content, lower limit and drained upper limit, respectively.
Seedling emergence occurs when the cumulative GEU is greater thanwhere PEGD (10 °C day for wheat) and PECM (10 °C day per cm for wheat) are required GEU for germination and emergence, respectively.
4 The Beta model
Jame and Cutforth [Citation3] separated the period between sowing and emergence of wheat into three consecutive processes: (1) germination, (2) subcrown internode elongation if the sowing depth is more than 2.5 cm, and (3) coleoptile elongation. Rates of germination and coleoptile elongation (day−1) were described by a Beta function:where T is the temperature and μ, α and β are model parameters (). The parameters α and β determine the curvatures of the relationship for the temperature range between Tb and To and between To and Tm, respectively.
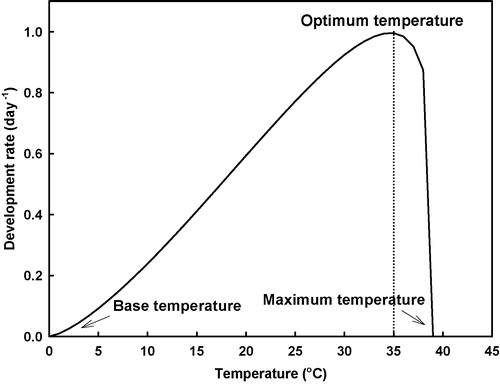
By examining the data derived by Jame and Cutforth [Citation3] and de Jong and Best [Citation5], we found that the Beta function described very well the relationship of the rate of subcrown internode elongation with temperature at each sowing depth. The shape parameters (α and β) were independent of sowing depth, whereas values of exp(μ) decreased linearly with increasing sowing depth. Therefore, we used the following equation to describe the effects of soil temperature and sowing depth on the rate of subcrown internode elongation:where a and b are parameters.
Jame and Cutforth [Citation3] used 0 and 42 °C as Tb and Tm, respectively. Based on a Petri plate study for the cultivar Neepawa (H. Wang and T. McCaig, unpublished data) we used 39 °C as Tm. Jame and Cutforth [Citation3] used data from studies under controlled environments to parameterize the equations describing the three processes [Citation5,Citation65,Citation66]. We used the same data excluding non-North American cultivars to parameterize μ, α, β, a, and b using non-linear regression analysis (PROC NLIN [Citation67]). The data of Neepawa were used to parameterize the germination equation [Citation66] and the data of the cultivar Canthatch [Citation69] were used to parameterize the equations describing coleoptile elongation and subcrown internode elongation [Citation5]. Both Neepawa [Citation68] and Canthatch [Citation69] are closely related to Thatcher [Citation70].
The algorithms of the Beta model were incorporated into the CSCER040 subroutine of DSSAT-CSM to simulate emergence time. The models were run in sequence mode. For each site there were two sequence files, odd year wheat and even year wheat, which allowed wheat to be simulated for each year under fallow–wheat rotations. Initial soil conditions were unknown in this study. Simulations using varying initial conditions of soil moisture and temperature showed that after 2 years of simulation, simulated soil temperature and moisture became independent of initial conditions. Therefore, simulations started 2 years prior to the years with emergence observations. The Beta model developed by Jame and Cutforth [Citation3] does not consider the effect of soil moisture. To test the effect of simulated soil moisture by DSSAT-CSM on seedling emergence, the Beta model was run using hourly or daily temperatures adjusted by WFGE:
To test the effect of simulated soil temperature, the model was also run using DSSAT-CSM-simulated soil temperature at sowing depth with or without the effect of the simulated soil moisture factor.
The sowing depth was unknown. It was assumed to be at 1–5 cm, as deeper than 5 cm is generally not recommended in North America [Citation71–Citation73]. Deep sowing may result in delayed and uneven emergence, may increase the risk of disease and weed infestation and may reduce seedling vigour. Simulation was done using depths from 1 to 5 cm with an increment of 1 cm.
5 Model evaluation
Comparisons of seedling emergence simulations among the various models mentioned above were assessed firstly by Pearson's correlation (r) [Citation74], which evaluates the association between simulated and measured values (precision). Then, the concordance correlation coefficient (CCC) and the bias correction factor (BCF = CCC/r) were calculated [Citation75]. Values of CCC close to 1 indicate that there is good agreement between measurements and simulations. The bias correction factor reflects simulation accuracy, i.e., the degree to which the regression line adheres to the 1:1 line through the origin (the concordance line). Thirdly, root mean square errors (RMSE) were used to estimate prediction errors (coincidences [Citation76]). Finally, the mean difference (M) was calculated to estimate the consistent error [Citation74]. All analyses were conducted with SAS [Citation67].
6 Results and discussion
Compared with the parameters derived by Jame and Cutforth [Citation3], the re-parameterized Beta model only slightly improved curve fitting for each of the three emergence processes in terms of the various indicators, except that the new model had a considerably higher CCC for coleoptile elongation simulation than the original model (), indicating improved agreement between measurements and simulations.
Table 2 Beta model parameters for germination, coleoptile elongation and subcrown internode elongation as derived by Jame and Cutforth [Citation3] and in this study, and model assessments using the Pearson correlation coefficient (r), concordance correlation coefficient (CCC), bias correction factor (BCF), root mean square error (RMSE), and mean difference (M).
Over all years and locations of this study, the number of days from sowing to 50% seedling emergence (DSE) ranged from 5 to 39 and averaged 12.2. Assuming sowing depth was 5 cm, the DSSAT-CSM model underestimated DSE in 94% of the site-years and averaged 4.8 days less than the observations ( and ). Although the correlation between measured and simulated DSE was statistically significant (r = 0.59, p < 0.001, n = 244) the CCC was quite low for DSSAT-CSM. Using daily air temperatures, the Beta model markedly improved simulations ( and ). Comparing the CCC, BCF and M indicators for the Beta model with those for the DSSAT-CSM model, the Beta model improved simulation in terms of both accuracy and precision.
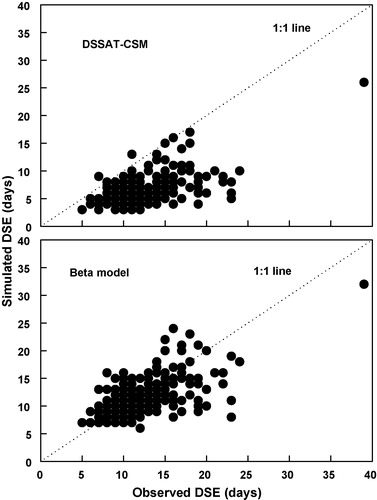
Table 3 Comparing observed days from sowing to 50% seedling emergence with those simulated by DSSAT-CSM or by Beta models using daily air, hourly air or daily soil temperatures, with or without including the soil moisture stress factor. Indicator variables used for comparison were: Pearson correlation coefficient (r), concordance correlation coefficient (CCC), bias correction factor (BCF), root mean square error (RMSE), and mean difference (M) (n = 244). The sowing depth was assumed to be 5 cm.
The model predicted the time to 50% seedling emergence for wheat reasonably well under different weather and soil conditions. Relatively poor simulations were found, however, at two sites: Indian Head, Saskatchewan and Fargo, ND; here temperature or soil moisture probably were not the main factors controlling DSE (). Other factors not considered by DSSAT-CSM or the Beta model may have affected seedling emergence. For example, the clay content at sowing depth was relatively high at those two sites (67% at Indian Head and 37% at Fargo), which may have caused soil compaction [Citation77] or crusting [Citation78] under certain conditions; and therefore, delayed seedling emergence.
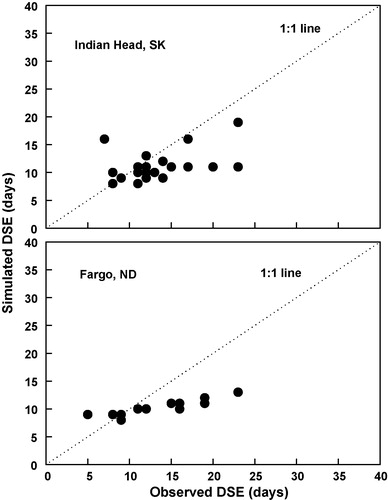
When the models were run using shallower sowing depths (1–4 cm), the averaged DSEs simulated by the Beta model were almost unchanged (−0.1 day) and all indicators (r, CCC, BCF, RMSE and M) remained unchanged or slightly improved, whereas DSSAT-CSM predicted shorter DSEs (1–3 days) and all indicators were poorer than when a sowing depth of 5 cm was used (data not shown).
The Beta model using moisture-adjusted daily air temperature did not improve predictions except for the M value, which was slightly reduced (). Over the 244 site-years, calculated days with soil moisture stress (WFGE < 0) only occurred in 13% (379 days) of the total observed DSEs (2975 days). Obviously, the calculated WFGE did not affect the prediction of DSE very much. This is in contrast to many findings that soil moisture is an important factor in determining wheat emergence [Citation4,Citation79]. A possible explanation for this is that the simulation of soil moisture at sowing depth may not be very accurate. Casanova et al. [Citation80] pointed out that simulations of near-surface soil moisture and temperature (0–5 cm) using daily time steps (like DSSAT-CSM) were less realistic than modelling hydrological processes using much shorter time steps. Another reason could be related to the calculation of WFGE. It was not clear why WFGE was calculated using Eqs. (Equation(3)(3)
(3) Equation(5)
(5)
(5) ), and under certain circumstances the calculation is questionable. For example, if the sowing depth is 4 cm and W1 (0–5 cm) and W2 (5–15 cm) are 0.46 and 0.57, respectively, WFGE = 1, meaning no water stress for germination/emergence; if W1 is increased to 0.69, WFGE is reduced to 0.23, meaning there is water stress; if W1 is further increased to 0.92, WFGE becomes 0, meaning water stress completely inhibits germination/emergence. Clearly, the calculation of WFGE needs to be revised.
The Beta model using hourly air temperature with or without taking into account soil moisture stress resulted in predictions similar to the model using daily air temperature (), because most of the daily or hourly temperatures were in the near-linear portion of the temperature response curve between Tb and To (). When the daily temperatures are near Tb, however, the non-linear response at low temperature can result in overestimation of days to 50% seedling emergence compared with using hourly temperatures. For example, in 1936 the daily mean air temperature was relatively low after sowing at Havre, MT (). The number of simulated days for germination and coleoptile elongation were higher and resulted in increased DSE (15 days), which is 4 days more than the observed DSE. Using simulated hourly temperatures, the Beta model predicted a shorter DSE (12 days), which was only 1 day more than the observed one.
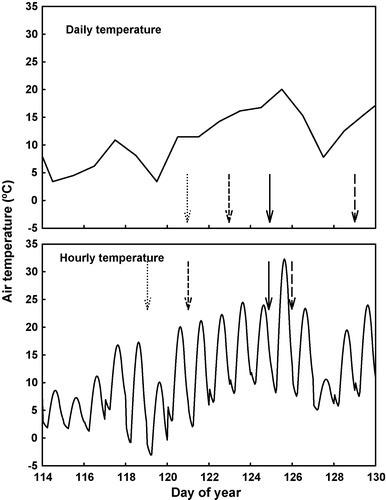
Estimations of the time to 50% seedling emergence using simulated daily soil temperature, with or without taking into account soil moisture stress were not as good as those using simulated using air temperatures (). This is surprising, considering that soil temperature should better reflect the seed environment than air temperature [Citation3,Citation4,Citation81]. The poorer results were likely caused by inaccurate simulation of near-surface soil temperatures [Citation80].
In conclusion, improved prediction of the time to 50% seedling emergence for wheat was achieved by incorporating the Beta model into DSSAT-CSM. Although using daily or calculated hourly air temperature generally resulted in similar predictions, hourly temperature could improve the simulation when the temperatures were near the base temperature. The predictions could be further improved if simulation of soil moisture and temperature at sowing depth were improved and the effect of soil water stress was properly addressed by the model. Other factors impacting seedling emergence such as soil surface properties could be incorporated into the model. Further work will also be done to simulate the dynamics of seedling emergence [Citation38,Citation55] because the population dynamics affect seedling uniformity and vigour and therefore the final yield.
Acknowledgements
The authors wish to thank Drs Gerrit Hoogenboom, Jeffrey White, Tony Hunt and Ken Boote for their help with the DSSAT-CSM. Alvin Anderson, Michael Bock and Wally Fraser from the Land Resource Unit with AAFC-AAC are gratefully acknowledged for their assistance with data collection. Special thanks go to our coop student Jae Hoon Saul for his help in data manipulation and model operation. We would also like to thank AAFC-AAC for providing the funding for outcome project 96 entitled ‘Impact of Climate Change on the Production of Energy Crops in Western Canada and Coping Strategies’.
References
- Y.GanE.H.StobeJ.MoesRelative date of wheat seedling emergence and its impact on grain yieldCrop Sci.32199212751281
- F.ForcellaR.L.B.ArnoldR.SanchezC.M.GhersaModeling seedling emergenceField Crops Res.672000123139
- Y.W.JameH.W.CutforthSimulating the effects of temperature and seeding depth on germination and emergence of spring wheatAgric. For. Meteorol.1242004207218
- M.J.LindstromR.I.PapendickF.E.KoehlerA model to predict winter wheat emergence as affected by soil temperature, water potential, and depth of plantingAgron. J.681976137141
- R.de JongK.F.BestThe effect of soil water potential, temperature and seedling depth on seedling emergence of wheatCan. J. Soil Sci.591979259264
- G.P.LafondB.D.FowlerSoil temperature and water content, seeding depth, and simulated rainfall effects on winter wheat emergenceAgron. J.811989609614
- E.M.KhahR.H.EllisE.H.RobertsEffects of laboratory germination, soil temperature and moisture content on the emergence of spring wheatJ. Agric. Sci. (Camb.)1071986431438
- J.T.RitchieWheat phasic developmentR.J.HanksJ.T.RitchieModeling Plant and Soil Systems, Agron. Monogr. vol. 31 (1991) ASA. Madison, WI. 31–54.
- G.S.McMasterW.W.WilhelmIs soil temperature better than air temperature for predicting winter wheat phenology?Agron. J.901998602607
- P.WilkensU.SinghA code-level analysis for temperature effects in the CERES modelsJ.WhiteModeling Temperature Response in Wheat and Maize2001CIMMYTEl Batan, Mexico17
- C.F.ShaykewichAn appraisal of cereal crop phenology modelingCan. J. Plant Sci.751995329341
- G.A.SlaferH.M.RawsonBase and optimum temperatures vary with genotype and stage of development in wheatPlant Cell Environ.181995671679
- W.E.Finch-SavageThe use of population-based threshold models to describe and predict the effects of seedbed environment on germination and seedling emergence of cropsR.Benech-ArnoldR.A.SánchezHandbook of Seed Physiology. Applications to Agriculture2004Food Products PressNew York5195
- R.WangY.BaiK.TaninoSeedling emergence of Winterfat (Krascheninnikovia lanata (Pursh) A.D.J. Meeuse & Smit) in the field and its prediction using the hydrothermal time modelJ. Arid Environ.6420063753
- B.G.H.TimmermansJ.VosJ.van NieuwburgT.J.StomphP.E.L.van der PuttenGermination rates of Solanum sisymbriifolium: temperature response models, effects of temperature fluctuations and soil water potentialSeed Sci. Res.172007221231
- D.J.SchimpfS.D.FlintI.G.PalmbladRepresentation of germination curves with the logistic functionAnn. Bot.41197713571360
- E.S.LeguizamónC.Fernandez-QuintanillaJ.BarrosoJ.L.Gonzalez-AndujarUsing thermal and hydrothermal time to model seedling emergenceWeed Res.452005149156
- E.KremerL.A.LotzGermination and emergence characteristics of triazine-susceptible and triazine-resistant biotypes of Solanum nigramJ. Appl. Ecol.351998302310
- M.MohantyD.K.PainuliModeling rice seedling emergence and growth under tillage and residue management in a rice–wheat system on a Vertisol in Central IndiaSoil Till. Res.762004167174
- J.QiuY.BaiB.CoulmanJ.T.RomoUsing thermal time models to predict seedling emergence of orchardgrass (Dactylis glomerata L.) under alternating temperature regimesSeed Sci. Res.162006261271
- X.YinM.J.KropffG.McLarenR.M.VisperasA nonlinear model for crop development as a function of temperatureAgric. For. Meteorol.771995116
- Y.W.JameH.W.CutforthJ.T.RitchieTemperature response function for leaf appearance rate in wheat and cornCan. J. Plant Sci.791999110
- W.YanL.A.HuntAn equation for modelling the temperature response of plants using the cardinal temperaturesAnn. Bot.841999607614
- A.SoltaniG.L.HammerB.TorabiM.J.RobertsonE.ZeinaliModeling chickpea growth and development: phenological developmentField Crops Res.9912006113
- J.W.JonesG.HoogenboomC.H.PorterK.J.BooteW.D.BatchelorL.A.HuntP.W.WilkensU.SinghA.J.GijsmanJ.L.RitchieThe DSSAT cropping system modelEur. J. Agron.182003235265
- M.Y.NuttonsonWheat–Climatic Relationships and the Use of Phenology in Ascertaining the Thermal and Photothermal Requirements of Wheat1955American Institute of Crop EcologyWashington, DC
- H.K. Hayes, E.R. Ausemus, E.C. Stakman, C.H. Bailey, H.K. Wilson, R.H. Bamberg, M.C. Markley, R.F. Crim, M.N. Levine, Thatcher wheat, Univ. of Minn. Agric. Experiment Station, Bull. No. 325 (1936).
- J.A.KolmerP.L.DyckA.P.RoelfsAn appraisal of stem and leaf rust resistance in North American hard red spring wheats and the probability of multiple mutations to virulence in populations of cereal rust fungiPhytopathology811991237239
- E.R.KerberT.AungLeaf rust resistance gene Lr34 associated with nonsuppression of stem rust resistance in the wheat cultivar CanthatchPhytopathology891999518521
- D.G.HumphreysT.F.Townley-SmithE.CzarneckiO.M.LukowB.D.McCallumT.G.FetchJ.A.GilbertJ.G.MenziesSnowbird hard white spring wheatCan. J. Plant Sci.872007301305
- A.H.RashidG.M.HalloranInfluence of photoperiod on culm elongation and apical development in semi-dwarf and standard-height wheatsAnn. Bot.541984375382
- R.G.FloodG.M.HalloranGenetics and physiology of vernalization response in wheatAdv. Agron.39198687125
- Y.Y.KlaimiC.O.QualsetGenetics of heading time in wheat (Triticum aestivum L.). I. The inheritance of photoperiodic responseGenetics741973139156
- N.K.BlakeS.P.LanningJ.M.MartinJ.D.ShermanL.E.TalbertRelationship of flag leaf characteristics to economically important traits in two spring wheat crossesCrop Sci.472007491494
- F.EwertSpikelet and floret initiation on tillers of winter triticale and winter wheat in different years and sowing datesField Crops Res.471996155166
- R.P.RajputM.R.DeshmukhV.K.ParadkarAccumulated heat units and phenology relationships in wheat as influenced by planting dates under late sown conditionsJ. Agron. Crop Sci.1591987345348
- A.H.Del PozzoJ.Garcia-HuidobroR.NovoaS.VillasecaRelationship of base temperature to development of spring wheatExp. Agric.2319872130
- G.S.McMasterW.W.WilhelmJ.A.MorganSimulating winter wheat shoot apex phenologyJ. Agric. Sci. (Camb.)1191992112
- Saskatchewan Agricultural Services Co-ordinating CommitteeGuide to Farm Practices in Saskatchewan1987Modern PressSaskatoon, SK
- National Climatic Data Centre, 2008. USHCN Daily Weather Data [Online]. Available: http://ftp://ftp.ncdc.noaa.gov/pub/data/ushcn/daily [2008 January 8].
- Agriculture and Agri-Food Canada, 2006. Daily Climate Information [Online]. Available: http://sisintrpd.agr.gc.ca/cansis/nsdb/climate/wthr_query.html [2008 January 4].
- R.D. Hungerford, S.W. Running, R.R Nemani, J.C. Coughlan, MTCLIM: a mountain microclimate extrapolation model, U.S. Forest Service Res. Paper INT-414, 1989, 52 pp.
- P.E.ThorntonH.HasenauerM.A.WhiteSimultaneous estimation of daily solar radiation and humidity from observed temperature and precipitation: an application over complex terrain in AustriaAgric. For. Meteorol.1042000255271
- W.J.PartonJ.A.LoganA model for diurnal variation in soil and air temperatureAgric. Meteorol.231981205216
- United States Department of Agriculture – Natural Resources Conservation Service, 2008. National soil survey characterization data [Online]. Available: http://ssldata.nrcs.usda.gov/querypage.asp [2008 February 4].
- Agriculture and Agri-Food Canada, 2007. Soil Landscapes of Canada Version 3.1.1 [Online]. Available: http://sis.agr.gc.ca/cansis/nsdb/slc/v3.1.1/intro.html [2008 February 14].
- J.T.RitchieModel for predicting evaporation from a row crop with incomplete coverWater Resour. Res.8197212041213
- C.H.B.PriestleyR.J.TaylorOn the assessment of surface heat and evaporation using large scale parametersMon. Weather Rev.10019728192
- J.T.RitchieA user-oriented model of the soil water balance in wheatE.FryT.K.AtkinWheat Growth and Modelling, NATO-ASI Series1985Plenum PressNew York293305
- H.L.PenmanNatural evaporation from open water, bare soil and grassProc. R. Soc. London, Ser. A1931948120146
- M.E.JensenR.D.BurmaanR.G.AllenEvapotranspiration and Irrigation Water Requirements: A Manual1990ASCENew York
- J.L. Monteith, Evaporation and environment, in: G.E. Fogg (Ed.), The State and Movement of Water in Living Organism, Soc. Exp. Biol. Symp. 19 (1965) 205–234.
- J.L.MonteithPrinciples of Environmental Physics1973Edward ArnoldLondon291 pp
- R.G. Allen, L.S. Pereira, D. Raes, M. Smith, Crop evapotranspiration. Guidelines for computing crop water requirements. FAO Irrig. and Drain. Paper 56, FAO, Rome (1988).
- P.D.JamiesonJ.R.PorterJ.GoudriaanJ.T.RitchieH.van KeulenW.StolA comparison of the models AFRCWHEAT2, CERES-Wheat, SIRIUS, SUCROS2 and SWHEAT with measurements from wheat grown under droughtField Crops Res.5519982344
- A.V.M. Ines, P. Droogers, I.W. Makin, A.D. Gupta, Crop growth and soil water balance modeling to explore water management options, IWMI Working Paper 22, International Water Management Institute, Colombo, Sri Lanka (2001).
- S.IrmakR.G.AllenE.B.WhittyDaily grass and alfalfa-reference evapotranspiration estimates and alfalfa-to-grass evapotranspiration ratios in FloridaJ. Irrig. Drain. Eng.1292003360370
- S.IrmakA.IrmakR.G.AllenJ.W.JonesSolar and net radiation-based equations to estimate reference evapotranspiration in humid climatesJ. Irrig. Drain. Eng.1292003336347
- F.SauK.J.BooteW.M.BostickJ.W.JonesM.I.MínguezTesting and improving evapotranspiration and soil water balance of the DSSAT crop modelsAgron. J.96200412431257
- F.X.López-CedrónK.J.BooteJ.PiñeiroF.SauImproving the CERES-maize model ability to simulate water deficit impact on maize production and yield componentsAgron. J.1002008296307
- Y.YinS.WuD.ZhengQ.YangRadiation calibration of FAO56 Penman–Monteith model to estimate reference crop evapotranspiration in ChinaAgric. Water Manage.9520087784
- J.W.JonesJ.T.RitchieCrop growth modelsG.J.HoffmanT.A.HowellK.H.SolomonManagement of Farm Irrigation Systems1991American Society for Agricultural Engineering6389
- J.T.RitchieSoil water balance and plant stressG.Y.TsujiG.HoogenboomP.K.ThorntonUnderstanding Options for Agricultural Production1998Kluwer Academic PublishersDordrecht, The Netherlands4154
- J.R.WilliamsC.A.JonesP.T.DykeA modeling approach to determining the relationships between erosion and soil productivityTrans. ASAE271984129144
- P.C.AddaeC.J.PearsonThermal requirements for germination and seedling growth of wheatAust. J. Agric. Res.431992585594
- G.P.LafondR.J.BakerEffects of temperature, moisture stress, and seed size on germination of nine spring wheat cultivarsCrop Sci.261986563567
- SAS Institute Inc.SAS Procedures Guide, Version 81999SAS Institute Inc.Cary, NC, USA
- A.B.CampbellNeepawa hard red spring wheatCan. J. Plant Sci.501970752753
- A.B.CampbellRegistration of Pembina and Canthatch wheats (Re. Nos. 422 and 423)Crop Sci.31963457458
- N.R.DraperH.SmithApplied Regression Analysis1966Wiley and SonsNew York, NY
- J. Fyksen, 2008. Things to consider about spring wheat in Wisconsin [Online]. Available: http://www.agriview.com/articles/2008/03/27/crop_news/crops01.txt [2009 June 18].
- J.L. Helm, L.A. Spilde, 1990. Selecting quality seed of cereal grains [Online]. Available: http://www.ag.ndsu.edu/pubs/plantsci/smgrains/a500w.htm [2009 June 18].
- J. Wiersma, 2009. Coleoptile lengths of current HRSW [Online]. Available: http://minnesotafarmguide.com/blog/%3Fp=325 [2009 June 18].
- K.LoagueR.E.GreenStatistical and graphical methods for evaluating solute transport models: overview and applicationJ. Contam. Hydrol.719915173
- L.LinA note on the concordance correlation coefficientBiometrics562000324325
- T.M.AddiscottA.P.WhitmoreComputer simulation of changes in soil mineral nitrogen and crop nitrogen during autumn, winter and springJ. Agric. Sci. (Camb.)1091987141157
- D.McGarryThe effects of soils water content during land preparation on soil physical condition and cotton growthSoil Till. Res.91987287302
- J.C.SheldonThe behaviour of seeds in soil. III. The influence of seed morphology and the behavior of seedlings on the establishment of plants from surface-lying seedsJ. Ecol.6219744766
- R.J.HanksF.C.ThorpSeedling emergence of wheat, grain sorghum, and soybeans as influenced by soil crust strength and moisture contentSoil Sci. Soc. Am. J.211957357359
- J.J.CasanovaJ.JudgeJ.W.JonesCalibration of the CERES-maize model for linkage with a microwave remote sensing modelTrans. ASABE492006783792
- G.S.McMasterW.W.WilhelmD.B.PalicJ.R.PorterP.D.JamiesonSpring wheat leaf appearance and temperature: extending the paradigm?Ann. Bot.912003697705