Abstract
Big data represent a pioneering development in the field of agriculture. By producing intuition, intelligence, and insights, these data have the potential to recast conventional process-driven agriculture, plotting the course for a smarter, data-driven farming. However, many open issues about the use of big data in agriculture remain unanswered. In this work, conceptualizing smart agricultural systems as cyber-physical-social systems, and building upon activity theory, we aim at highlighting some key questions that need to be addressed. To our view, big data constitute a tool reciprocally produced by all the actors involved in the agrifood supply chains. The constant flux of this tool and the intricate nature of the interactions among the actors who share it complicate the translation of big data into value. Moreover, farmers’ limited capacity to deal with data complexity, along with their dual role as producers and users of big data, impedes the institutionalization of this tool at the farm level. Although the approach used left us with more questions than answers, we suggest that unraveling the institutional arrangements that govern value co-creation, capturing the motivations of farmers and other actors, and detailing the direct and indirect effects that big data (and the technologies used to generate them) have in farms are important preconditions for setting forth rules that facilitate the extraction and equal exchange of value from big data.
1 Introduction
In the late 1990s, participants in a symposium on precision agriculture characterized smart farming as a high knowledge-intensive form of practicing agriculture, which uses insights from both basic and applied science to capture the effects of problems and actions in space and time, thus having the ability to enhance the productive potential of farm enterprises while, in parallel, reducing the environmental externalities of agriculture (CitationLake et al., 1997). Despite its vagueness, this definition indicates that smart farming is much more than the fine-tuning of technology and other inputs, which is the case in precision agriculture. More than two decades later, the interest in smart farming has grown apace, fueled by the huge opportunities new technological developments offer today. Using farm robotics, Internet of Things, and cloud computing capacities, and exploiting the interoperability of databases and the use of real-time data, smart farming can improve farm management, while ensuring the maintenance of natural environment (CitationRaja et al., 2018; CitationJayaraman et al., 2016; CitationWolfert et al., 2014).
Smart farming can be seen as the end of a continuum ranging from traditional, labor-intensive forms of agriculture, where past experience and intuition are used to inform farmers’ decisions, to technology-intensive, data-supported forms of precision agriculture, where field-specific data are used to help farmers make appropriate decisions on the production process (CitationKritikos, 2017), to a data-intensive form of farming, where smart devices and intelligent decision support systems can exploit the potential offered by big data, leading to data-driven decision making. Hence, smart farming can be conceived of as a data-driven form of agriculture, in which decision making processes are based on explicit information derived from big data collected through heterogeneous sources (CitationWolfert et al., 2017).
The use of big data for guiding farmers’ decision making is a real revolution which has the potential to transform current “process-driven agriculture” – where the emphasis is in the process of using scarce resources to produce crop and livestock products – to a new, data-driven agriculture. To better illustrate the difference between process- and data-driven (smart) farming let us take a look at an example. Summer 2017 in some Mediterranean regions was characterized by very high temperatures and high-precipitation events. Such a combination offers a favorable environment for grapevine diseases like downy mildew, which can significantly reduce both the quantity and the quality of the produced wine. Indeed, in Italy, Spain and France wine production dropped to a historic low (CitationOrganisation Internationale de la Vigne et du Vin, 2017). Being traditionally based on process-driven approaches, most Mediterranean viticulturists experienced wide departures from normal yields, since they followed the standard plant protection measures and agronomic strategies. On the contrary, having access to weather data, and constantly monitoring temperature, soil moisture, leave hydration and relative humidity data, or even having the potential to receive downy mildew alerts and advices – facilities offered by some smart systems (CitationEuropean Commission, 2017; CitationPérez-Expósito et al., 2017) – data-driven viticulturists might have the opportunity to adjust their phytosanitary treatments so as to increase the effectiveness of pesticide interventions, and to reduce both their spraying costs and the environmental impacts of fungicides. In other words, although in process-driven agriculture viticulturists failed to achieve high efficiency while maintaining product and ecosystem value, data-driven decisions helped farmers reducing the production losses, lessening the pesticide costs, and minimizing the environmental impact of plant protection.
Of course, the notion that data are important for informing farmers’ decisions is not new. Price- (CitationKruse, 1958) or production-related data (CitationAakhus, 1947) were always considered valuable inputs in the decision making process. As Brown noted in the mid-1950s, availability, timeliness, and accuracy of high volume datasets can secure the viability of agrifood supply chains (CitationBrown, 1955). Nevertheless, although “traditional” data – i.e. information that can be transferred between data originators, providers, users or third parties (CitationCopa-Cogeca et al., 2018) – have the potential to show past or present trends, big data, by taking advance of interconnectivity (CitationZikopoulos and Eaton, 2011), datafication (CitationWalker, 2014) and digitization (CitationLoebbecke and Picot, 2015), can combine complex and large datasets (CitationBajaj and Ramteke, 2014) thus offering entirely new sources of current and detailed information derived not only from structured but also semi-structured or even unstructured data (CitationFranks, 2012). This amalgamation of real-time multi-sourced information – derived not only from on- but also from off-farm data (CitationLiang et al., 2018) – offers farmers better opportunities to diagnose potential risks, to identify possible alternatives, and, more importantly, to predict future occurrences than traditional analytics which are based on past data. Consequently, big data can supply farmers with quick-witted intelligence, this way transforming traditional (process-driven) agricultural systems to smarter, data-driven systems.
Although the original definitions of big data emphasized their great volume, today the term encompasses not only the amount of data available but also the technologies and expertise used to generate, collect, store, analyze, present and use these data (CitationYang et al., 2017). Big data are characterized by their great volume (size of the datasets), their huge variety (heterogeneity of data types and sources) and their substantial velocity (speed of data generation) (CitationGandomi and Haider, 2015). These three characteristics (commonly referred to as “the three Vs” in the literature) permit the emergence of hidden value from big data (CitationHashem et al., 2015). Having the potential to glean important and applicable information from high-volume datasets (CitationSagiroglu and Sinanc, 2013), big data analytics can produce new forms of value, by helping actors make smarter, faster and impactful decisions (CitationAhmed et al., 2015) through informed intuition, intelligence, and insight (CitationAbdrabo et al., 2016). According to CitationPadgavankar and Gupta (2014):
- | informed intuition refers to the ability to predict future occurrences and identify appropriate actions, |
- | intelligence relates to the offering of solutions to real-time problems, |
- | insights emerge from the process of reviewing occurrences and actions. |
Moreover, big data can bring together different actors, through value-generating information. Farmers, processors, manufacturers, agrochemical companies, and technology suppliers (AgTech companies) are all involved in and affected by the use of big data in agriculture (CitationBronson and Knezevic, 2016), whereas researchers, practitioners and policymakers can draw important conclusions and design appropriate intervention schemes when needed (CitationChen et al., 2012). In this vein, big data have the potential to enhance collaboration among different nodes of the agrifood supply chain, facilitating the expression of what some scholars term connective action (CitationCieslik et al., 2018; CitationLeeuwis et al., 2018).
However, a crucial question is whether these data can really lead agriculture to a sustainable future. Despite the considerable amount of literature dealing with the issue, today our comprehension of how to use big data to ensure the sustainability of agrifood production is still at an embryonic stage. In the early discussions on smart farming, the ability of smart technologies to improve agricultural efficiency occupied a central position (CitationPorceddu and Rabbinge, 1997). Indeed, later works confirmed that smart (data-driven) farming has the potential not only to increase farm production and efficiency (CitationBorchers and Bewley, 2015), but also to help farmers minimizing microbiological (CitationOliver et al., 2017) or disaster-related (CitationŘezník et al., 2017) risks, to reduce fertilization costs (CitationBendre et al., 2016), to facilitate crop disease management (CitationGarg and Aggarwal, 2016), to minimize depletion of natural resources (CitationGupta et al., 2016), to mitigate climate change (CitationScherr et al., 2012) and to enhance food security (CitationRibarics, 2016). However, already from the very early stages of smart farming expansion, doubts about its potential impacts on ecological sustainability were raised (CitationAthanasiou, 1998). Today, many scholars express several concerns on a broad range of social and ethical issues associated with big data and smart farming (CitationBronson, 2018; CitationEastwood et al., 2019; CitationCarbonell, 2016). CitationKritikos (2017) provides a wide list of socio-ethical imperatives associated with the use of data in agriculture, including both data-related perils – such as dependency risks, potential lock-in effects, data concentration, and transformation of farmers into information tools – and sustainability challenges.
Notably, as a research topic, the issue of big data is characterized by diverse and, oftentimes contradictory arguments. Hype and confusion abound in the scientific debate (CitationMithas et al., 2013). Depending on the point of view, different features of big data are emphasized. Hence, although some claim that big data can provide answers to the most pressing problems generated by process-driven agriculture, others speak about a dark side of big data. Nevertheless, to better grasp the effects that big data can have on global farm architecture, it is important to clearly conceptualize not only how these tools are used, but also by whom they are used and what determines their ability to transform farming.
In this work, our purpose is to decipher the ways big data connect farmers, farms and agrifood systems, and to provide a list of questions that can open up new research agendas in the field of smart farming research. Building upon activity theory (CitationEngeström, 1999), and drawing from different fields of study, we attempt to sketch a new framework for understanding how farmers interact with big data with the aim to reach specific outcomes related to their farms. We also discuss the mediating role of the wider network of actors who share the work of production and use of big data in these interactions, and the ways formal and institutional rules catalyze the application of big data in agricultural systems. In doing so, this study moves beyond prior work in this area, by shifting the focus from the ways big data can be applied in farm settings to the interactions between the components of activity systems that big data mediate, and by setting the scene for identifying caveats and new research lines in the field of smart farming.
2 Smart farming as a cyber-physical-social system
In their highly cited work, CitationWolfert et al. (2017) conceptualize smart farming as a “cyber-physical system” in which smart devices (the “cyber” dimension of the system) are the locus of control for the farm (the “physical” dimension). Of course, in such a system humans still undertake different activities – like the analysis of data and the planning – but different appliances perform most of the tasks required to keep the system operating. However, in our view, this system also entails a social dimension, emerging from the fact that cyber-social information (social information exchanged within the cyberspace) is inextricably linked with big data (CitationBarnaghi et al., 2015). Actually, there is little – if any – doubt that the interactions between individuals and/or social groups constantly change the cyberspace through the production of new data (CitationZeng et al., 2019). Moreover, human action is a necessary condition for transforming big data and the farm, while, at the other end of the spectrum, humans continue to be in charge for the decision making processes – since some indications confirm that even sophisticated and highly intelligent decision support systems are differently approached and used by farmers (CitationLundström and Lindblom, 2018; CitationAlvarez and Nuthall, 2006) – which, in their turn, further alter the physical and the cyber dimensions of the system. Consequently, we argue that it is more precise to define smart agricultural systems as “cyber-physical-social” systems (CitationSheth et al., 2013).
The social dimension of this system refers to the sum of entities which provide and use data, that is all the immediately involved actors in the supply chain of agrifood products – farmers, manufacturers, wholesalers, distributors, and consumers – but also intermediately connected actors who might be involved in the various upstream and downstream flows of goods, services, resources and capital, such as intermediate suppliers and customers, collaborative or competitive organizations, institutes or communities, as well as a cloud of “external” actors like governmental organizations, research and policy institutes, banks, etc. (CitationLioutas et al., 2018; CitationMentzer et al., 2001). Big data link all these actors through shared cognition – the ability to build institutional connections that help them interpret cues in a similar way (CitationWertsch, 1991; CitationCannon‐Bowers and Salas, 2001) – thus augmenting their ability to produce meaning necessary for sustaining systems performance. Hence, big data are used as glue, connecting the physical and the social dimension of the system.
3 A brief insight into activity theory
Activity theory (or cultural-historical activity theory) draws from thoughts developed by a group of thinkers belonging to what today is called the School of Russian Social-Psychology. The two main exponents of this school – CitationLeontyev (1978) and CitationVygotsky (1978) – aimed at elucidating and explaining the relationships between “subjects,” “objects,” and “tools” used to transform these objects. Subjects, objects and tools constitute what can be termed “activity system.” In plain words, every activity – which consists of a set of intentionally performed goal-directed actions (CitationRoth, 2007) – can be captured as an interaction between a subject and an object with the aim of transforming the object through the use of various tools (CitationRussell, 1998). This conceptualization is also evident in interactional psychology, where an individual’s actions are considered as the outcome of her/his interaction with the specific situations she or he encounters. In this vein, individuals have to be conceived of as active agents, who intentionally engage in an interaction process (CitationEndler and Magnusson, 1976).
CitationEngeström (1987) expanded upon Soviet scholars’ work, by designing a more complete theoretical framework of human activity. Instead of some related theoretical foundations, CitationEngeström’s (2005) view is based on a systems approach to conceptualize human (intentional) activities, without considering actors as “black boxes” lacking internal properties. This philosophy emphasizes the continuous process of transformation across time; a process that extends beyond individual levels, thus affecting and being affected by a wide array of actors (CitationEngeström, 1999). Following this line of thinking, current conceptualizations of activity theory have moved beyond the issue of mediation through specific tools, to incorporate diversity and interactivity between activity systems (CitationBakhurst, 2018).
According to activity theory’s basic premises, individual activities are “practical,” take place in social contexts, and can be mediated by various artifacts, i.e., technological developments, laws, methods, practices, etc. (CitationEngeström and Middleton, 1998; CitationKuutti, 1996). To produce any result or to transform an object to an outcome, a subject – after developing a level of motivation – uses the available toolsFootnote 1 to effectively interact with the object. However, the activity is also influenced by a community of actors who share a common interest in the process. A set of rules intermediates the subject-community relationship, whereas a certain division of labor regulates the interrelation between subject, object and the community (CitationEngeström, 2001; CitationNardi, 1996) ().
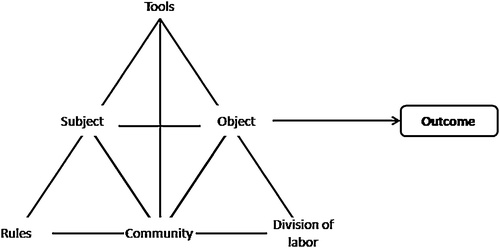
Activity theory has drawn much attention from theorists and researchers interested in explaining the ways technology can be exploited within activity systems in the field of education (CitationIsssroff and Scanlon, 2002), ergonomics (CitationEngeström, 2000), transportation (CitationEttema, 2018), and so on. Indeed, as CitationKaptelinin and Nardi (2018) explain, activity theory can frame the human-technology interaction with a meaningful context, offering opportunities to better capture the ways technology affects – and is affected by – individuals and groups, as well as illustrating the real meaning of technology for people. The application of activity theory in the study of human-technology interaction represents a shift in scientific focus from the technological inventions to the ways human actors interact with technology and with each other within a framework determined by specific requirements and constraints (CitationBannon, 1995). Therefore, activity theory, by depicting “the doing of the activity in a rich social matrix of people and artifacts” (CitationKaptelinin and Nardi, 2006, p. 9) can shed new light on the ways big data influence the interactions between farmers, farming, and agrifood systems.
4 Applying an activity theory approach to reframe smart farming
To frame our analysis we used the model developed by CitationMwanza and Engeström (2005), widely known as the eight-step model. According to this model, to operationalize the activity and sub-activity triangles (), one needs to define the activity under investigation (1 st step: Activity), to understand the “why” behind the activity (2nd step: Objective), to identify the actors who perform the activity (3rd step: Subjects) and the tools that mediate this activity (4th step: Tools), to determine the rules that constrain and regulate the activity (5th step: Rules and regulations), to understand how labor is divided among the actors who participate in the activity system (6th step: Division of labor), to define the community of actors involved in the activity (7th step: Community), and to identify the expected outcome of the activity (8th step: Outcome). Some brief descriptions of the terms used in the eight-step model for the case of smart farming are provided in .
Table 1 The eight-step model of activities (adapted from CitationMwanza and Engeström, 2005, p. 459) in the case of smart farming.
As CitationMwanza (2001) notes, defining the elements of an activity system helps us answering a series of questions that focus on the interrelations between systems’ components. These questions (presented in ) drove our analytic plan. The first of the two main sections that follow is dedicated to analyzing the subject-tools-object triangle (). Our analysis starts with a brief description of an activity system in the case of process-driven farming (Section 4.1.1), so as to help us better illuminate what differentiates big data from conventional technologies. Then, we present the special characteristics that account for the very nature of big data (Sections 4.1.2 and 4.1.3). In the next sections we shift our focus on the relation between tools and subjects, examining the ways big data affect farmers’ decision making process (Section 4.1.4) and analyzing the issue of data ownership (Section 4.1.5). The Section 4.1.6 aims at scrutinizing the issue of farmers’ motivation. Finally, we turn our attention to the relation between big data and the farm (Section 4.1.7). The second part of the analysis is devoted to identifying what community means in the case of smart farming (Section 4.2.1), to examining the ways the division of labor affects production of and access to value emerging from big data (Section 4.2.2) and to discussing the issue of rules that regulate (or should regulate) the activity (Section 4.2.3).
Table 2 Questions on the interrelations within the activity system.
4.1 Subjects, objects and tools
4.1.1 Activity systems in process-driven farming
In the case of process-driven farming, farmer – the subject of the activity – reciprocally interrelates with the object (the farm) to produce certain outcomes. It is this attempt to yield specific results that initiates every action. Traditional farm technologies (tractors, combines, fertilizers, pesticides, irrigation systems, etc.) are tools that mediate this process, facilitating outcome attainment. Nevertheless, apart from technological instruments, the term “tools” in activity theory also encompasses the subject’s knowledge, skills and competencies (CitationEngeström, 2000). These internal tools correlate with technologies affecting the mediation process, determining in this way the length of the interaction between subject and object.
4.1.2 Big data as tools: Inseparability of production and consumption
In the case of smart farming, the interrelations between farmers, farms and big data are quite more complex than in process-driven agriculture. Farmers occupy a double role in the activity system. First, they are users of data, since they utilize the results of data analysis to deal with real-farm problems (CitationEvans et al., 2017). Second, although farm-related data refer to issues pertaining to crop and/or livestock responses to various physical conditions, growth factors and climatic variables, farmer’s management practices heavily affect the information content of these data. In this vein, farmers are also co-producers of data. Hence, they simultaneously construct physically (through their decisions and actions) and socially (through the different meanings they ascribe to these data during use) big data. This peculiarity generates a different form of “duality.” As CitationOrlikowski (1992) explains in her seminal article, tangible technologies are created by some actors and then used by others, which reify and institutionalize them, through embedding them into specific organizational structures. In the case of big data, however, farmers’ dual role complicates the process of institutionalization, since every action made by a farmer influences both the quantity and the quality of big data. This raises an important question:
Q1. How does the duality of farmers (as data users and co-producers) influence the institutionalization of big data?
Remarkably, the inseparability of production and consumption is a key feature differentiating big data from any other technological development ever appeared in the field of agriculture. For instance, the way a farmer uses her/his tractor affects the tractor only in the long-term, so the quality of the tractor remains relatively stable when the analysis is restricted to a limited time frame. On the contrary, differently from other – tactile – tools, big data are in continuous evolution (CitationChen et al., 2014), in the sense that every action generates new data, thus altering the nature of the tool. So, farmers have to continuously adapt to the constantly evolving nature of big data and to develop new knowledge and skills (internal tools) so as to effectively transform big data (external tools) into usable decisions. Nevertheless, it is questionable whether the farmer, as an individual agent, has the ability to deal with the ever-increasing complexity of the big data (CitationMittelstadt and Floridi, 2016). Absorbing the meaning of the complex insights offered by big data analytics is not an easy task, and lack of understanding these insights significantly restricts an actor’s ability to act upon them (CitationLaValle et al., 2011). Moreover, even when information is rich and insightful, heuristics are often used to guide decision making processes (CitationKlein and Thompson, 1984). Therefore, a germane question is:
Q2. Do farmers have the ability to deal with the ever-increasing complexity of big data?
4.1.3 Big data, bigger data and more cooked data
The dramatic increase of data generated by sensors and computers, not only in agriculture but in every arena of economic and social life (CitationKhan et al., 2014), raises some new concerns about the quality of the information provided through data analytics. First of all, the size of data is not a synonym of data quality. As van den CitationHoven et al. (2012, p. 157) put it, “bigger data are not always better data.” Second, potential biases are more possible as the number of data increases. Although a variety of data cleaning applications emerged already from the early stages of big data explosion (CitationRahm, 2000), cleaning is in itself a subjective process which might lead to serious biases (CitationBoyd and Crawford, 2012). Apart from cleaning, all the data handling operations carried out lead to a different form of data. As CitationBowker (2013) notes, data are never “raw” – i.e., data “generated and collected without editing or any processing” (CitationCopa-Cogeca et al., 2018) – but rather “cooked,” in the sense that a degree of processing always exists. The term “processing” encompasses every operation performed on data or datasets, including, for example, data organization, structuring, adaptation, etc. (CitationCopa-Cogeca et al., 2018).
When viewed within an activity theory context, this transformation of tools further increases the complexity of the activity system (CitationPetersen et al., 2002; CitationBertelsen, 2000). Indeed, this transformation from a raw to a cooked form – owing to the conditions of collection, storage, and transmission – might heavily affect the ability to precisely interpret data and the capacity to draw usable conclusions, whereas it increases the complexity of the activity system and puts in doubt the accuracy of big data (CitationFan and Bifet, 2013). On the other hand, some scholars raise serious concerns about the power of various actors to hijack data during their processing so as to gain competitive advantages (CitationMoura and Serrão, 2015). Consequently, the quality of big data is an outcome not only of processes used during data acquisition, preprocessing and analysis, but also depends on the intentions, orientations, and motivations of different actors. Here, a new question comes to the fore:
Q3. How does transformation (quantitative and qualitative) of big data affect the quality of information provided to farmers?
4.1.4 Big data and farmers’ decision making
In the growing body of the relevant literature, big data are often viewed as a tool that “guides” decision making (CitationPhillips-Wren and Hoskisson, 2015; CitationGopalkrishnan et al., 2012). If that is true, then big data analytics provide specific opportunities of action, determining to a great extent farmers’ decisions. Indeed, studies converge to show that such tools can increase farmers’ decision making performance by offering specific solutions (CitationCapalbo et al., 2017; CitationZhang et al., 2012). In this vein, big data open-up specific pathways of action, which farmers can follow to solve problems of their farms. Nevertheless, as CitationMorozov (2013) states, this reliance on fixed norms of action might reduce actors’ creativity and experimentation, thus restricting their ability to discover and exploit – even by mistake – new paths. At the other side of the coin, as structurational models of technology suggest (CitationOrlikowski, 2008), the solutions provided by big data are differently experienced and used not only by different actors but also by the same actor at different times. In this view, the ways big data are interpreted and used depends on a wide array of factors, including personal variables (beliefs, attitudes), characteristics of the task at hand, and sets of priorities (CitationDeSanctis and Poole, 1994). So, a critical question is the following:
Q4. Do big data represent a tool that “guides” farmers’ decision making processes or do they offer abstract information that is differently elaborated and used by farmers?
To answer this question, one needs to shift focus from how big data impact a farm to how big data analytics are used within complex systems that entail social, technological and physical dimensions (CitationRose et al., 2018). Although, traditionally, technology is treated by researchers as an independent variable that affects, changes and transforms an object (CitationOrlikowski and Iacono, 2001), it is perhaps more important to understand how subjects simultaneously interact with big data and objects (their farms). As CitationFisher et al. (2012) argue, to capture the potential of big data, a more concise focus on the experience of user interaction with these data is needed.
4.1.5 A shortlist of “hot” issues: Data ownership and data privacy
Notably, another characteristic that distinguishes big data from technologies used in process-driven agriculture is the lack of ownership. Although a farmer can claim that a tractor is “her” or “his” tractor, no one can support that big data are her/his “property.” So, every farmer has to deal with a tool that is collaboratively developed and used, but its ownership is unclear. This peculiarity of big data generates two major issues. First, despite their contribution to the development of the tool, farmers lack control over the data exchange process in terms of data customization. CitationBronson and Knezevic (2016) also stress this issue by observing that farmers do not have a voice in shaping the context of data collection, analysis, and use.
Second, as we explain in a following section, by eliminating individuals’ opportunities to set-up clear zones where other actors do not have access to their “own” data (CitationMai, 2016), the lack of ownership rights is associated with the issue of privacy (CitationShepherd et al., 2019), thus complicating the relationships between farmers and other members of the community (CitationKempenaar et al., 2016; CitationSykuta, 2016). The practice of signing agreements with technology providers creates a sense of uncertainty and perceived risk about the potential uses of farmers’ data (CitationDesouza and Smith, 2014). Hence, despite the existence of regulatory frameworks and industry standards – at least in developed countries – on the use of big data (CitationKshetri, 2014), farmers are sometimes unwilling or even reluctant to share their data (CitationJakku et al., 2019). Although the issue of willingness traditionally receives less attention than the quality of the connection between different actors through data sharing practices, it is inextricably linked with the performance of the whole agrifood supply network (CitationFawcett et al., 2007). Consequently, a crucial question is:
Q5: How can we increase farmers’ active engagement in big data production and use?
4.1.6 Subjects and motives
To better capture the ways an activity is performed it is fundamental to understand what gives birth to subjects’ actions, by focusing on the motives that spur individuals to act upon an object with the aim of producing certain outcomes (CitationFoot, 2014). In other words, a challenging task is to delineate how farmers’ motivations, goals, and intentions determine the limits within which big data and smart technologies can be fully exploited at the farm level. Several indications confirm that farmers’ actions have different economic, managerial, social, and also psychological precursors (CitationFang et al., 2018; CitationLioutas and Charatsari, 2018; CitationBrown and Roper, 2017), while their motivational orientations guide their engagement in various activities (CitationCharatsari et al., 2018; CitationTriste et al., 2018; CitationCharatsari et al., 2017). A more concise focus on the motivation behind adoption and use of big data is expected to shed further light on the process of farm transformation, since it can help us put action into contexts that integrate environmental, personal, and procedural factors (CitationSzalma, 2009). As CitationEngeström (2000, p. 964) puts it, motives give to actions “their ultimate continuity, coherence, and meaning.”
From an activity theory perspective, different motives – sometimes conflicting (CitationSannino, 2011) – can drive subjects’ behaviors. This type of “poly-motivation” (CitationKaptelinin, 2005, p. 6) raises some new conundra concerning the ways multiple motives emerge, the processes by which motives are generated within and beyond the need to transform the object, and the ways motives are associated with certain activities instead of other sets of activities (CitationKaptelinin, 2005). Moreover, social contexts catalyze subjects’ motivation (CitationAllen et al., 2011), whereas oftentimes both personal and collective motives may operate in tandem (CitationHyysalo, 2005). Therefore, two questions emerge:
Q6. To what extent is the use of big data a self-determined and autonomous decision?
Q7. How is the motivation process reshaped and embedded within a community of actors?
4.1.7 Back to the basics: rethinking the farm
As the object of the activity, the farm drives while in parallel determines the length of any possible human action. Following Engeström’s objects-as-concerns view (Citation2009, p. 304), a farm can be viewed simultaneously as a generator and a focus of “attention, motivation, effort, and meaning.” In this vein, the farm serves as the basis for the development of motivations and the setting of goals to be attained by farmers, whereas it is in continuous transformation and reorganization. Big data (the tools) are used to gradually transform the farm, thus facilitating the achievement of the desired outcomes (higher production, better product quality, more efficient farm management, reduction of environmental impacts, etc.). Nevertheless, as in any activity, potential negative outcomes are always possible.
However, the interrelation between big data and the farm represents the less discussed aspect of the activity system in the literature. Quite surprisingly, there is broader speculation about the legitimacy of using technology in process-driven crop and especially animal production than in data-driven farming. The limited emphasis on the issue probably owes to the intangible nature of big data. Nevertheless, big data have both direct (referring to the new allocation of resources due to investments in smart technologies needed) and indirect effects on the farm (e.g., the use of wearable sensors for data collection purposes in productive animals). Hence, most of the concerns over the negative effects of technology on farms are multiplied in the case of smart farming. Among the few studies addressing this dimension of smart farming, issues like animals’ freedom of choice (CitationDriessen and Heutinck, 2015) or animals’ autonomy (CitationSchewe and Stuart, 2015) hold a predominant position. The emphasis of big data analytics on the outcome (i.e. higher performance, lower effort) leads to an over-commodification of farms (especially of farm animals) which creates new ethics. This adds a new question to the discussion on big data in farming:
Q8. Do (and, if so, how) the novel digital ethics affect the quality of natural resources used in farm production?
4.2 Community, division of labor, and rules
4.2.1 Defining community
Perhaps the most challenging task when applying activity theory in the field of smart farming is to define what community means. A simple approach would be to describe the community in terms of access to the object. In this sense, the community is the group of persons who “hold” the farm (and, thus, they share the labor). However, within an activity system, access to the tool is also a characteristic of the community. Consequently, the term community can be thought of as a group of individuals who engage in processes of collective action (CitationOstrom, 2010, Citation2007) driven by the common interests they share on the object and the tools (CitationEngeström, 2014; CitationBellamy, 1996).
In process-driven farming, the term “community” is liable to a wide array of interpretations, ranging from the farm family to farm enterprise (including the farm family and the personnel), to the actors that supply farmers with products or services aimed at helping them to effectively use the different tools so as to transform the object of the activity (the farm). These suppliers can be manufacturers of technologies, providers of repair services, advisors, extension providers or other actors involved in the field of agricultural production. Nevertheless, when the discussion turns to data-driven farming, the term is also used to denote the sum of players involved in the processes of producing, handling, and using big data. In other words, in the case of smart farming, it is not only the involvement in the production or translation of technology which defines the community. All the entities who provide, analyze and use data shape an informal community which is characterized by a remarkable heterogeneity, in that involved actors have significantly different aims and worldviews (CitationEastwood et al., 2019). Consequently, the term community encompasses the whole supply chain (i.e. the actors involved in the supply of inputs, products, and services, but also the entities which collect, store and process data) – also including consumers (CitationJay, 2007). Although the latter conceptualization of the community seems exaggerated, it is well known that nodes in a supply chain are firmly connected so that every change at a farm level generates fluctuations that increase in volume moving downstream the supply chain (CitationLioutas et al., 2018). In any case, the interactions between the community and the three nodes that constitute the upper triangle of activity theory’s model (CitationEngeström, 2001) shape the effectiveness of the transformation process, thus deserving intensive inquiry. To put in context these interactions, we should first find answers to the following questions:
Q9. Which actors affect (and are affected by) the use of big data in farming?
Q10. How do different actors interrelate with farmers and with each other?
4.2.2 Distribution of power, labor and value
Traditionally, in the relevant literature, the issue of power is tied to that of control over data management processes (CitationCarbonell, 2016; CitationAndrejevic, 2014). CitationHaire (2014) supports that today the distribution of value from big data is unequally allocated across agrifood systems, with farmers enjoying a limited share of it. In this perspective, the actors who collect and analyze data receive the lion’s share of value from big data. “Value” is the key-word here. To our view, what creates the power imbalance within a community is the uneven access not to big data but to the value emerging from them. CitationStubbs (2016) also argues that the central question in the discussion on data access is not who owns the data, but who owns the value of them. Besides, as CitationMittelstadt and Floridi (2016) note, although the notion of disempowered data subjects prevails, it is still unclear how data subjects – without having the skills and competencies needed – can exercise their access rights so as to independently exploit the potential of big data. On the other hand, albeit often portrayed by popular media as dark centers of power, big players in the field of smart farming are the only actors who seem to have the competence (both technological and operational) to handle and produce meaning from these data, thus having better access to their value.
Today, there is little doubt that the distribution of power within this community of actors follows the investments made by different actors in labor, technology, human resources and expertise (CitationAndrejevic, 2014). In other words, those actors who have the capacity to develop a high level of competence in dealing with big data are the most privileged (CitationBoyd and Crawford, 2012). Nevertheless, in this view, the value of big data is based on a neoclassical economics framework: farmers aim at maximizing the utility of big data, whereas AgTech companies, which process and analyze data, have as a central purpose the maximization of direct and indirect profits. Hence, these players “produce” value (and, consequently, enjoy the major share of it), whereas farmers “consume” this value or, as CitationVargo et al. (2008) put it, “destroy the value.” In other words, the value is considered as an asset that is to be delivered along with big data to their potential users.
However, within complex systems consisting of different actors, value is realized only when “consumers” (in our case the farmers) make use of a product or service (in this case, the big data) (CitationLusch and Vargo, 2006). In terms of current marketing thinking, all the actors participating in the community are linked with relationships aimed at translating big data into value. To achieve this purpose, actors integrate resources and work under the aim of providing value, not only for their own benefit but also for the other members of the community, thus engaging in “actor for actor relationships” (CitationPolese et al., 2017). Such a view acknowledges the importance of all the involved actors in the process of value creation. To use an example, in the BIGt&u project – a Dutch consortium consisting of horticultural companies, growers associations, governmental agencies, information technology providers, and knowledge institutes – big data referring to all aspects of horticultural products marketing (consumer data, production data, and supply data) represent the glue that connects actors with different roles, aims and interests (CitationVerwaart, 2015). Each one of these actors contributes data and/or work to the platform. In this sense, the labor is divided among all the involved members, although each actor undertakes different tasks. Nevertheless, this division of labor does not necessarily mean that actors cooperate with each other. It is well known that agrifood systems operate under conditions of coopetition (CitationWalley and Custance, 2010), which means that actors have to cooperate while at the same time they compete with one another. Although current work in the field emphasizes the competitive nature of the relationships among community members and farmers, to capture the multidimensional tenor of value it is essential to focus on the ways actors reciprocally co-create value.
Importantly, the collectively produced and used big data can pave the way for the development of an alternative, customer-dominant logic (CitationHeinonen et al., 2010), where actors who collect and handle data are viewed as “value facilitators” (to use the term of CitationGrönroos and Gummerus, 2014) and farmers create their own value, by embedding big data into their social and/or professional contexts, activities, and practices (CitationHeinonen et al., 2010). In addition, consumers of farm products can gain a more central position in the value ecosystem, since data on their preferences can be used to inform farmers’ crop choices. Going back to the BIGt&u example, insights from consumer data can help farmers adjust the allocation of their resources so as to correspond to the real market needs. However, the degree to which the value production process is consumer-driven, farmer-driven or controlled by other centers of power depends on the institutional arrangements that govern the activity system. Hence, irrespective of the actors involved in the activity system, there is a need to shift attention from the roles of individual players to the institutional factors that coordinate the community.
Therefore, researchers have the challenging task to define how value is co-created and what value means for each actor by moving beyond conceptualizations of value as the return of labor and invested capital. To borrow the terminology from systems ecology literature (CitationGren et al., 1994), the value of big data can be divided into primary and secondary. Primary value refers to the development and maintenance of the activity system; that is the ability of big data to connect the elements of the system, to ensure system’s productive capacity and to increase its resilience. For instance, helping farmers make sound and timely management decisions, big data can help them and other community members to avoid disturbances (weather hazards, market changes, etc.) by simultaneously reducing the economic waste associated with agricultural production. On the other hand, secondary value pertains to the outcomes that extend beyond the activity system. For example, by supporting the efficient use of natural resources (CitationLamrhari et al., 2016), big data can lead to more ecologically sustainable agriculture. The issue of value is quite multilayered and intricate, that makes it impossible to fully elaborate on it here. However, we offer a series of indicative questions:
Q11. What does value mean for farmers and other actors?
Q12. How is value (co-)created?
Q13. How do power dynamics and institutional factors shape the value (co-)creation frameworks?
4.2.3 Rules
The issue of rules is the most addressed in the literature on big data. As posited in the activity theory, the interrelations between the subject and the community are mediated through shared and agreed rules (CitationDissanayeke et al., 2014). Consequently, a fundamental element to be clarified refers to the norms, rules or other regulations which affect the activity under study (CitationMwanza and Engeström, 2005). Hence, an – old but always relevant – question is:
Q14. Which are the implicit and explicit rules affecting the way the activity takes place?
The creation of shared rules of thumb is as fundamental as complex in big data management. A huge amount of studies underlines several socio-ethical dilemmas associated with access to big data – but with a limited emphasis on big data value, as we discussed in the previous section. There is a consensus among researchers that if, on the one side, we are witnesses of a new era of agricultural production (where big data drive the evolution of farming), on the other side, privacy concerns occur (CitationWhitacre, 2014). The four main concerns that need to be considered in rule setting are the issues of ownership, privacy, security and openness (CitationJanssen et al., 2017; CitationStubbs, 2016; CitationSykuta, 2016). These questions are particularly urgent in supply networks dominated by big players like multinational corporations located upwards in the supply chain.
Nevertheless, another important task is to elucidate what rules are about. With the term “rule” we mean not only laws but also social, cultural and other patterns of interaction, as well as practices that influence the activity. CitationEngeström (1987) assimilates rules to procedures, norms, and conventions that frame behaviors of members within an organized social scheme. This definition of rules draws on the institutional tradition, both the original institutional economics (CitationHodgson, 2007) and the new institutional economics (CitationNorth, 1990), according to which institutions include both formal and informal conventions. Therefore, the definition of the rules needs to be contextualized on the basis of the three key “w-questions”: who, where, and when (CitationKébir, 2017):
Q15. Who defines the rules, where these rules are applied, and when (new) rules are needed?
The first strand of these questions refers to the key-actors involved in rule setting along the supply and data chain; that is the “stakeholder network” including big data users, companies specialized in data management, regulatory and policy actors (CitationWolfert et al., 2017, p. 71). What makes the setting of rules difficult is the growing number of players involved, both in data management (producers, data collectors, independent agricultural data banks, data cooperatives) and data uses (farmers and ranchers, retailers, industry groups, environmental interest groups) (CitationStubbs, 2016).
The second strand is related not only to the territorial dimension but also to the business context and to the institutional context. In the rich corpus of literature on the issue, the discussion on the very nature of agrifood supply chains holds an ascendant position. Supply chains in the agrifood sector are often dominated by the presence of large AgTech providers (actors that collect, aggregate, and process data), playing a monopolizing role with respect to the weak nodes of the chain, like farmers (CitationSykuta, 2016). The difficulty of rule design is drawn on the need for catching farmers’ trust in achieving agreements on rule identification and specification. This is particularly true in the family farm business, where the family sphere overlaps the farming one. Consequently, widening the horizons of knowledge access opportunities is a necessary step, through the involvement of all members of the activity system in processes of discussion, debate, and reflection (CitationAhonen et al., 2000; CitationEngeström, 2001).
Finally, the third strand refers to the ways current or past negative/positive experience affects the patterns of rule setting. Paying attention to the historical evolution of the contexts within which economic activities take place can help us better capture the future trajectories of any activity (CitationWelter, 2011). This dimension is linked with institutional arrangements, given that institutions – by determining the contexts of transactions and interactions (CitationVargo and Lusch, 2016) – shape the ways different actors experience their role in the activity system, thus offering the basis for the (re)designing of rules. When dysfunctional, these institutions impede the co-creation and sharing of value, whereas when instrumental facilitate the systemic attainment of common goals (CitationNelson and Nelson, 2002).
5 Discussion and conclusions
The discussion on the interrelation between big data and farming is shrouded by significant counterarguments. From the fear that an Orwellian future is near to the overenthusiasm for – and overconfidence to – the potential of big data in regenerating agriculture there is a wide variety of opinions and points of view. Our work, resting upon the premise that smart farming is a cyber-physical-social system, and applying an activity theory perspective, aimed at shedding new light on the ways the dimensions of data-driven agricultural systems intercorrelate. In doing so, the article provides a new series of questions, constituting not a new research agenda, but a map of areas in which new research agendas focusing on creating and answering different sets of sub-questions should be developed.
The fifteen questions emerged from our analysis () pertain to different relations within the activity system. Big data – the tools in our system – not only facilitate the transformation of the farm through their enormous potential, but also mediate between farmers and communities, and between communities and farms, whereas they prompt – and are subjects to – the development of new rules, and lead to a reorientation of the division of labor in the agrifood system. Nevertheless, big data radically differ from conventional technologies like the tractor repeatedly used as an example in the previous sections, because they are in continuous evolution and are characterized by ever-increasing size and complexity. As CitationKaranasios (2018) notes, these kinds of hybrid tools are produced by the concurrent action of subjects and communities, thus transgressing traditional notions of technology. The observation that farmers, although contributing to the development of the tool, don’t have full access to it seems paradox when we frame our discussion in terms of conventional technologies, but in the digital world it is a common ethos.
Table 3 Summary of the key questions on the use of big data in smart farming.
However, access to big data – albeit associated with the creation of digital divides in the relevant literature (CitationCrawford, 2013; CitationNunan and Di Domenico, 2013) – is of less importance when compared with the question of access to the value derived from the use of big data. Surprisingly, the issue of value has attracted only a limited share of attention by scholars. Not rarely, in lieu of a more accurate way to defining it, value is conceived of as a synonym to farmers’ decision making performance. To our view, value can be distinguished into primary and secondary, where the former refers to the value enclosed within the activity system and the latter to the value extensions beyond the system. Such a differentiation could help researchers assess the value of big data not only within but also outside the zone of the activity system. Nonetheless, in networked systems, value takes different forms, as it is uniquely and phenomenologically experienced by the beneficiaries (CitationVargo and Lusch, 2017). So, a critical task for scholars is to clarify what value means for farmers and other community members, and then to examine how power dynamics affect access to this value. A first step to this end should be the identification of actors who collaboratively produce both the big data and the value extracted by them. A more concise focus on the community formed by these actors – and the institutions that it nests – can deepen our understanding of the ways interrelations and everyday practices lead to the co-creation of value.
Perhaps it goes without saying that these actors have different purposes, foci, and intentions. However, what is common within the activity system is the aim to produce value from big data. Nevertheless, the institutionalization of data is a provocative conundrum, especially for farmers who often lack the competence to deal with the labyrinthine nature of big data. To unravel the mechanisms with which farmers transform big data into value we need to illuminate the process of combining conventional wisdom with the insights offered by data analytics. The answer to this riddle cannot be simple, since each farmer adopts different patterns of interaction with big data, depending on her/his motivational orientations. The term motivation here refers not only to the goals set by individuals in relation to the object of the activity (CitationLocke and Latham, 1990) – which have received the most interest so far – but also to the intrinsic appeals that guide behavior (CitationRyan and Deci, 2000).
At the other end of the spectrum, the farm – as the object of activity – is in constant evolution. It consists of a sum of entities (including the land, the cultivated crops and livestock, farm machinery, buildings, production infrastructures, etc.) that, as CitationMiettinen (2005) argues, are embedded in different socio-economic activities. Hence, the value proposition within this system can be seen as the “meeting point of several interrelated activities” (CitationSpinuzzi, 2017, p. 268). Under this prism, the farm determines both the contexts of farmers’ actions and the tools used, this way also defining the community of actors that mediates the subject-object relation. The transition from process- to data-driven farming inevitably leads to the involvement of more actors in the system and requires the creation of new value propositions. Nevertheless, since such a shift can redefine the meaning of farming, we need to understand why farmers are doing farming under different situations.
Another interesting avenue for future research is to explore how big data affect not only the performance but also the structure of the farm and the quality of farm life. Both inanimate and – especially – animate elements (productive animals which are often transformed into data collection appliances) of a smart farm are variously affected by the technologies used for data collection purposes, as well as by the application of big data analytics in farm practice. Therefore, the reconceptualization of the interplay between farmers, animals, and big data should be brought to the attention of researchers and policy-makers (CitationHolloway et al., 2014). Other sets of rules, relating to the interrelation between subjects, objects, communities of involved actors, and tools are also necessary.
To sum up, our analysis instead of offering answers to the pressing dilemmas associated with data-driven agriculture provides fifteen questions and sets a foundation for future research. Activity theory affords researchers and policy makers an opportunity to taxonomize many challenging questions relevant to the potential benefits and the important risks associated with the use of big data in farming. This work represents a first attempt towards this direction, by depicting some intriguing interrelations among farmers, farms, big data, communities, and rules. Although other frameworks – like, for example, CitationLatour’s (1996) Actor Network Theory – that have been previously used to deal with the ways agricultural systems process inputs transforming them into outputs (CitationNoe and Alrøe, 2012, Citation2006) can also depict the ways big data produce value for the agrifood sector, activity theory differs in that it distinguishes between different entities within the system (CitationMiettinen, 2001), thus putting emphasis on the co-evolution of these entities in the framework of the activity (CitationMiettinen, 1999). Interestingly, this co-evolution generates conflicts, imbalances, and breakdowns, or – in activity theory vocabulary – contradictions.
Contradictions refer to misfits within elements of the activity systems (primary contradictions), between them (secondary contradictions), between different activities (tertiary contradictions), and between different developmental phases of a single activity (quaternary contradictions) (CitationFoot, 2014). As CitationFoot (2014) explains, these contradictions reflect the richness of a system and its ability to move forward, so, their careful consideration can open-up new outlooks for understanding the developmental potential of the system. Despite the fact that our aim was not to perform a contradiction analysis, our approach can throw some light on the secondary contradictions, which concern the conflicts between different components of the system, such as subjects and tools (farmers’ views on the ways big data reconstruct the farm), subjects and the community (since the community reconciles the subject-tools relationship but absorbs an important portion of the produced value), tools and the rules that frame the use of big data, etc. Our list of questions () might help to identify some of these contradictions.
Nevertheless, farming is by definition characterized by primary contradictions – emerged from the conflict between use value and exchange value (CitationFoot, 2001) – since the “doing” of farming has both an inherent and a commoditized value. On the other hand, smart faming is in itself a tertiary contradiction, since new tools – referring to both new data and their new uses – already used in other, more advanced activities (CitationEngeström, 1987) are continuously introduced in the activity system. Furthermore, given that both the practice of smart farming and the research in the field are in an early stage of development, the identification of quaternary contradictions – inter-activity conflicts (CitationFoot and Groleau, 2011) – is still largely undermined. Although it is important to understand how these contradictions affect the activity system, such an analysis is left for other researchers.
Moreover, it is worth mentioning that our analysis was concentrated to some universally shared features of data-driven farming systems, but it must be acknowledged that activity systems might be quite different depending on the type of farming they are referred to or on the available infrastructures. In supporting this argument, relevant work indicates that farms with higher levels of integration into the value chain can better exploit the opportunities offered by big data (CitationFleming et al., 2018), whereas the big data software capacity is concentrated in countries with advanced economies (CitationHilbert, 2016). Moreover, one can expect significant differences between developed and developing countries in the levels of big data availability or data literacy.
Obviously, much future work remains to be done on the modes activity systems operate, the ways in which value is produced, and the forces that govern the production and distribution of value. Hence, the present work must be viewed as what was designed to be: a first step towards a systemic analysis of activity systems in the case of smart, data-driven farming. To achieve a sound knowledge base on the topic, both theoretical and empirical research on specific activity systems are needed. Future researchers can expand our framework by adding and studying new concepts. Two central issues in activity theory – yet, not touched upon in this study – are historicity and expansive transformation. Historicity refers to the need to view the problems and potentials of activity systems against their own history (CitationEngeström, 2001; CitationFoot, 2001). Although it was beyond the scope of this article, the difficult task to put smart farming systems into a historical context – so as to understand how past activities, ideas, tools, and motives shape current trends of activities – can offer a wide range of new insights. Expansive transformation, on the other hand, refers to the ways the whole activity system is transformed as an outcome of the gradual resolution of contradictions (CitationEngeström, 2001; CitationAvis, 2009). We hope the questions posed here can provide a suitable jumping off point for those who will undertake the challenging task to study how data-driven farming is expansively transformed.
Acknowledgment
We would like to thank the two reviewers and the editor for their valuable comments and suggestions, which significantly improved the quality of this article.
Notes
1 Although Engeström also uses the words “mediating artifacts” (2001) or “instruments” (2000) in this article we adopted the term “tools” used in his later writings.
References
- J.E. Aakhus Hog production in relation to corn-hog ratio and feed production in North Dakota Aidos News IX 1947 131 133
- M. Abdrabo M. Elmogy G. Eltaweel S. Barakat Enhancing big data value using knowledge discovery techniques Int. J. Inf. Technol. Comput. Sci. 8 2016 1 12 https://doi.org/10.5815/ijitcs.2016.08.01
- F.D. Ahmed A.N. Jaber M.B.A. Majid M.S. Ahmad Agent-based big data analytics in retailing: a case study 4th International Conference on Software Engineering and Computer Systems 2015 67 72 https://doi.org/10.1109/ICSECS.2015.7333085
- H. Ahonen Y. Engeström J. Virkkunen Knowledge management - the second generation: creating competencies within and between work communities in the competence laboratory Y. Malhotra Knowledge Management and Virtual Organizations 2000 Idea Group Publishing Hershey 282 305
- D. Allen S. Karanasios M. Slavova Working with activity theory: context, technology, and information behavior J. Am. Soc. Inf. Sci. Technol. 62 2011 776 788 https://doi.org/10.1002/asi.21441
- J. Alvarez P. Nuthall Adoption of computer based information systems: the case of dairy farmers in Canterbury, NZ, and Florida, Uruguay Comput. Electron. Agric. 50 2006 48 60 https://doi.org/10.1016/j.compag.2005.08.013
- M. Andrejevic Big data, big questions| the big data divide Int. J. Commun. 8 2014 1673 1689
- T. Athanasiou Divided Planet: The Ecology of Rich and Poor 1998 University of Georgia Press Athens (USA)
- J. Avis Transformation or transformism: Engeström’s version of activity theory? Educ. Rev. 61 2009 151 165 https://doi.org/10.1080/00131910902844754
- R.H. Bajaj P. Ramteke Big data–the new era of data Int. J. Comput. Sci. Inf. Technol. 5 2014 1875 1885
- D. Bakhurst Activity, action and self-consciousness Educ. Rev. 70 2018 91 99 https://doi.org/10.1080/00131911.2018.1388618
- L.J. Bannon From human factors to human actors: the role of psychology and human-computer interaction studies in system design R.M. Baecker J. Grudin W.A.S. Buxton S. Greenberg Readings in Human–Computer Interaction 1995 Morgan Kaufmann Burlington 205 214
- P. Barnaghi A. Sheth V. Singh M. Hauswirth Physical-cyber-social computing: looking back, looking forward IEEE Internet Comput. 3 2015 7 11 https://doi.org/10.1109/MIC.2015.65
- R.K.E. Bellamy Designing educational technology: computer-mediated change B.A. Nardi Context and Consciousness: Activity Theory and Human-Computer Interaction 1996 MIT Press Cambridge 123 146
- M.R. Bendre R.C. Thool V.R. Thool Big data in precision agriculture through ICT: rainfall prediction using neural network approach S. Satapathy Y. Bhatt A. Joshi D. Mishra Proceedings of the International Congress on Information and Communication Technology 2016 Springer Singapore 165 175
- O.W. Bertelsen Design artefacts: towards a design-oriented epistemology Scandinavian Journal of Information Systems 12 2000 2Available athttp://https://aisel.aisnet.org/cgi/viewcontent.cgi?article=1099%26context=sjis
- M.R. Borchers J.M. Bewley An assessment of producer precision dairy farming technology use, prepurchase considerations, and usefulness J. Dairy Scienc. 98 2015 4198 4205 https://doi.org/10.3168/jds.2014-8963
- G.C. Bowker Data flakes: an afterword to “Raw data is an oxymoron” L. Gitelman Raw Data is an Oxymoron 2013 MIT Press Cambridge 167 172
- D. Boyd K. Crawford Critical questions for big data: provocations for a cultural, technological, and scholarly phenomenon Inf. Commun. Soc. 15 2012 662 679 https://doi.org/10.1080/1369118X.2012.678878
- K. Bronson Smart farming: including rights holders for responsible agricultural innovation Technol. Innov. Manag. Rev. 8 2018 7 14 https://doi.org/10.22215/timreview/1135
- K. Bronson I. Knezevic Big data in food and agriculture Big Data Soc. 3 2016 1 5 https://doi.org/10.1177/2053951716648174
- D.D. Brown Local data wanted by business firms J. Farm Econ. 37 1955 1047 1049 https://doi.org/10.2307/1233988
- P. Brown S. Roper Innovation and networks in New Zealand farming Aust. J. Agric. Resour. Econ. 61 2017 422 442 https://doi.org/10.1111/1467-8489.12211
- J.A. Cannon‐Bowers E. Salas Reflections on shared cognition J. Organ. Behav. 22 2001 195 202 https://doi.org/10.1002/job.82
- S.M. Capalbo J.M. Antle C. Seavert Next generation data systems and knowledge products to support agricultural producers and science-based policy decision making Agric. Syst. 155 2017 191 199 https://doi.org/10.1016/j.agsy.2016.10.009
- I.M. Carbonell The ethics of big data in big agriculture Internet Policy Rev. 5 2016 https://doi.org/10.14763/2016.1.405Available athttp://https://policyreview.info/articles/analysis/ethics-big-data-big-agriculture
- C. Charatsari E.D. Lioutas A. Koutsouris Farmers’ motivational orientation toward participation in competence development projects: a self-determination theory perspective J. Agric. Educ. Ext. 23 2017 105 120 https://doi.org/10.1080/1389224X.2016.1261717
- C. Charatsari A. Koutsouris E.D. Lioutas A. Kalivas E. Tsaliki Promoting lifelong learning and satisfying farmers’ social and psychological needs through farmer field schools: views from rural Greece J. Agric. Food Inf. 19 2018 66 74 https://doi.org/10.1080/10496505.2017.1325742
- H. Chen R.H. Chiang V.C. Storey Business intelligence and analytics: from big data to big impact MIS Q. 36 2012 1165 1188 http://https://www.jstor.org/stable/41703503
- M. Chen S. Mao Y. Liu Big data: a survey Mob. Netw. Appl. 19 2014 171 209 https://doi.org/10.1007/s11036-013-0489-0
- K.J. Cieslik C. Leeuwis A.R.P.J. Dewulf R. Lie S.E. Werners M. van Wessel P. Feindt P.C. Struik Addressing socio-ecological development challenges in the digital age: exploring the potential of Environmental Virtual Observatories for Connective Action (EVOCA) NJAS-Wagening. J. Life Sci. 86–87 2018 2 11 https://doi.org/10.1016/j.njas.2018.07.006
- Copa-Cogeca EU Code of Conduct on Agricultural Data Sharing by Contractual Agreement 2018 Brussels
- K. Crawford The Hidden Biases of Big Data. Harvard Business ReviewAvailable at2013 http://https://hbr.org/2013/04/the-hidden-biases-in-big-data
- G. DeSanctis M.S. Poole Capturing the complexity in advanced technology use: adaptive structuration theory Organ. Sci. 5 1994 121 147 https://doi.org/10.1287/orsc.5.2.121
- K.C. Desouza K.L. Smith Big data for social innovation Stanford Soc. Innov. Rev. Summer 2014 39 43
- U. Dissanayeke K.P. Hewagamage R. Ramberg G. Wikramanayake Study to initiate mobile learning among a group of young farmers from Kandy District: an activity theory based approach Trop. Agric. Res. 26 2014 26 38 https://doi.org/10.4038/tar.v26i1.8069
- C. Driessen L.F. Heutinck Cows desiring to be milked? Milking robots and the co-evolution of ethics and technology on Dutch dairy farms Agric. Hum. Values 32 2015 3 20 https://doi.org/10.1007/s10460-014-9515-5
- C. Eastwood L. Klerkx M. Ayre B.D. Rue Managing socio-ethical challenges in the development of smart farming: from a fragmented to a comprehensive approach for responsible research and innovation J. Agric. Environ. ETH 2019 https://doi.org/10.1007/s10806-017-9704-5In press
- N.S. Endler D.E. Magnusson Interactional Psychology and Personality 1976 Hemisphere Oxford
- Y. Engeström Learning by Expanding: An Activity‐Theoretical Approach to Developmental Research 1987 Orienta‐Konsultit Helsinki
- Y. Engeström D. Middleton Introduction: studying work as mindful practice Y. Engeström D. Middleton Cognition and Communication at Work 1998 Cambridge University Press Cambridge 1 14
- Y. Engeström Activity theory and individual and social transformation Y. Engeström R. Miettinen R.L. Punamäki Perspectives on Activity Theory 1999 Cambridge University Press Cambridge 19 38
- Y. Engeström Activity theory as a framework for analyzing and redesigning work Ergon 43 2000 960 974 https://doi.org/10.1080/001401300409143
- Y. Engeström Expansive learning at work: toward an activity theoretical reconceptualization J. Educ. Work 14 2001 133 156 https://doi.org/10.1080/13639080020028747
- Y. Engeström Developmental Work Research: Expanding Activity Theory in Practice 2005 Lehmanns Media Berlin
- Y. Engeström The future of activity theory: a rough draft A. Sannino H. Daniels K. Gutiérrez Learning and Expanding with Activity Theory 2009 Cambridge University Press Cambridge 303 328
- Y. Engeström Activity theory and learning at work U. Deinet C. Reutlinger Tätigkeit-Aneignung-Bildung 2014 Springer Wiesbaden 67 96
- D. Ettema Apps, activities and travel: an conceptual exploration based on activity theory Transportation 45 2018 273 290 https://doi.org/10.1007/s11116-017-9844-5
- European Commission Smart Vineyard: Management and Decision Making Support for Wine Producers, Internal Market, Industry, Entrepreneurship and SMEs Digital Transformation Monitor 2017 European Commission Brussels
- K.J. Evans A. Terhorst B.H. Kang From data to decisions: helping crop producers build their actionable knowledge Crit. Rev. Plant Sci. 36 2017 71 88 https://doi.org/10.1080/07352689.2017.1336047
- W. Fan A. Bifet Mining big data: current status, and forecast to the future ACM SIGKDD Explor. Newsl. 14 2013 1 5 https://doi.org/10.1145/2481244.2481246
- W. Fang L. Tang P. Cheng N. Ahmad Evolution decision, drivers and green innovation performance for collaborative innovation center of ecological building materials and environmental protection equipment in Jiangsu Province of China Int. J. Environ. Res. Public Health 15 2018 2365 https://doi.org/10.3390/ijerph15112365
- S.E. Fawcett P. Osterhaus G.M. Magnan J.C. Brau M.W. McCarter Information sharing and supply chain performance: the role of connectivity and willingness Supply Chain Manag. Int. J. 12 2007 358 368 https://doi.org/10.1108/13598540710776935
- D. Fisher R. DeLine M. Czerwinski S. Drucker Interactions with big data analytics Interact 19 2012 50 59 https://doi.org/10.1145/2168931.2168943
- A. Fleming E. Jakku L. Lim-Camacho B. Taylor P. Thorburn Is big data for big farming or for everyone? Perceptions in the Australian grains industry Agron. Sustain. Dev. 38 2018 24 https://doi.org/10.1007/s13593-018-0501-y
- K.A. Foot Cultural-historical activity theory as practice theory: illuminating the development of conflict-monitoring network Commun. Theory 11 2001 56 83 https://doi.org/10.1111/j.1468-2885.2001.tb00233.x
- K.A. Foot Cultural-historical activity theory: exploring a theory to inform practice and research J. Hum. Behav. Soc. Environ. 24 2014 329 347 https://doi.org/10.1080/10911359.2013.831011
- K. Foot C. Groleau Contradictions, transitions, and materiality in organizing processes: an activity theory perspective First Monday 2011 16 https://doi.org/10.5210/fm.v16i6.3479
- B. Franks Taming the Big Data Tidal Wave: Finding Opportunities in Huge Data Streams With Advanced Analytics 2012 Wiley Hoboken (NJ)
- A. Gandomi M. Haider Beyond the hype: big data concepts, methods, and analytics Int. J. Inf. Manag. 35 2015 13 144 https://doi.org/10.1016/j.ijinfomgt.2014.10.007
- R. Garg H. Aggarwal Big data analytics recommendation solutions for crop disease using Hive and Hadoop Platform Indian J. Sci. Technol. 9 2016 https://doi.org/10.17485/ijst/2016/v9i32/100728
- V. Gopalkrishnan D. Steier H. Lewis J. Guszcza Big data, big business: bridging the gap W. Fan A. Bifet Q. Yang P. Yu Proceedings of the 1st International Workshop on Big Data, Streams and Heterogeneous Source Mining: Algorithms, Systems, Programming Models and Applications 2012 ACM New York 7 11
- M. Gren C. Folke K. Turner I. Batemen Primary and secondary values of wetland ecosystems Environ. Resour. Econ. 4 1994 55 74 https://doi.org/10.1007/BF00691932
- C. Grönroos J. Gummerus The service revolution and its marketing implications: service logic vs service-dominant logic Manag. Serv. Qual. 24 2014 206 229 https://doi.org/10.1108/MSQ-03-2014-0042
- A. Gupta S. Mishra N. Bokde K. Kulat Need of smart water systems in India Int. J. Appl. Eng. Res. 11 2016 2216 2223
- B. Haire Ag Data: Its Value, Who Owns It and Where’s It Going? Southeast Farm PressAvailable at2014 http://https://www.southeastfarmpress.com/cotton/ag-data-its-value-who-owns-it-and-where-s-it-going
- I.A.T. Hashem I. Yaqoob N.B. Anuar S. Mokhtar A. Gani S.U. Khan The rise of “big data” on cloud computing: review and open research issues Inf. Syst. 47 2015 98 115 https://doi.org/10.1016/j.is.2014.07.006
- K. Heinonen T. Strandvik K.J. Mickelsson B. Edvardsson E. Sundström P. Andersson A customer-dominant logic of service J. Serv. Manag. 21 2010 531 548 https://doi.org/10.1108/09564231011066088
- M. Hilbert Big data for development: a review of promises and challenges Dev. Policy Rev. 34 2016 135 174 https://doi.org/10.1111/dpr.12142
- G.M. Hodgson What are institutions? J. Econ. Issues XL 2007 1 25
- L. Holloway C. Bear K. Wilkinson Robotic milking technologies and renegotiating situated ethical relationships on UK dairy farms Agric. Hum. Values 31 2014 185 199 https://doi.org/10.1007/s10460-013-9473-3
- S. Hyysalo Objects and motives in a product design process Mind Cult. Activity 12 2005 19 36 https://doi.org/10.1207/s15327884mca1201_3
- K. Isssroff E. Scanlon Using technology in higher education: an activity theory perspective J. Comput. Assist. Learn. 18 2002 77 83 https://doi.org/10.1046/j.0266-4909.2001.00213.x
- E. Jakku B. Taylor A. Fleming C. Mason S. Fielke C. Sounness P. Thorburn “If they don’t tell us what they do with it, why would we trust them?” Trust, transparency and benefit-sharing in Smart Farming NJAS-Wagening. J. Life Sci. 2019 https://doi.org/10.1016/j.njas.2018.11.002In press
- S.J.C. Janssen C.H. Porter A. Moore N. Athanasiadis I. Foster J.W. Jones J.M. Antle Towards a new generation of agricultural system data, models and knowledge products: information and communication technology Agric. Syst. 155 2017 200 212 https://doi.org/10.1016/j.agsy.2016.09.017
- M. Jay The political economy of a productivist agriculture: New Zealand dairy discourses Food Policy 32 2007 266 279 https://doi.org/10.1016/j.foodpol.2006.09.002
- P.P. Jayaraman A. Yavari D. Georgakopoulos A. Morshed A. Zaslavsky Internet of things platform for smart farming: experiences and lessons learnt Sensors 16 2016 1884 https://doi.org/10.3390/s16111884
- V. Kaptelinin The object of activity: making sense of the sense-maker Mind Cult. Act. 12 2005 4 18 https://doi.org/10.1207/s15327884mca1201_2
- V. Kaptelinin B.A. Nardi . Acting With Technology: Activity Theory and Interaction Design 2006 MIT press Cambridge
- V. Kaptelinin B. Nardi Activity theory as a framework for human-technology interaction research Mind Cult. Act. 25 2018 3 5 https://doi.org/10.1080/10749039.2017.1393089
- S. Karanasios Toward a unified view of technology and activity: the contribution of activity theory to information systems research Inf. Technol. People. 31 2018 134 155 https://doi.org/10.1108/ITP-04-2016-0074
- L. Kébir V. Peyrache-Gadeau O. Crevoisier P. Costa Introduction: sustainability, innovative milieus and territorial development L. Kébir V. Peyrache-Gadeau O. Crevoisier P. Costa Sustainable Innovation and Regional Development. Rethinking Innovative Milieus 2017 Edward Elgar Cheltenham 1 24
- C. Kempenaar C. Lokhorst E.J.B. Bleumer R.F. Veerkamp T. Been F.K. van Evert Big Data Analysis for Smart Farming 2016 Wageningen University and Research WageningenWageningen Plant Research report
- N. Khan I. Yaqoob I.A.T. Hashem Z. Inayat M. Ali W. Kamaleldin Big data: survey, technologies, opportunities, and challenges Transfus. Apher. Sci. 2014712826https://doi.org/10.1155/2014/712826
- E. Klein A.C. Thompson Theory of Correspondences, Including Applications to Mathematical Economics 1984 Wiley New York
- M. Kritikos Precision agriculture in Europe: legal, social and ethical considerations Science and Technology Options Assessment 2017 Scientific Foresight Unit (STOA) of the European Parliament BrusselsPE 603.207
- R.H. Kruse Acreage Response of South Dakota Wheat Producers to Expected Price Changes, 1955 1958 South Dakota State University
- N. Kshetri The emerging role of Big Data in key development issues: opportunities, challenges, and concerns Big Data Soc. 1 2014 1 20 https://doi.org/10.1177/2053951714564227
- K. Kuutti Activity theory as a potential framework for human-computer interaction research B.A. Nardi Context and Consciousness: Activity Theory and Human-Computer Interaction 1996 MIT Press Cambridge 17 44
- J.V. Lake G.R. Bock J.A. Goode Precision Agriculture: Spatial and Temporal Variability of Environmental Quality 1997 John Wiley & Sons Chichester
- S. Lamrhari H. Elghazi T. Sadiki A. El Faker A profile-based Big data architecture for agricultural context M. Essaaidi S. El Hani Proceedings of 2016 International Conference on Electrical and Information Technologies (ICEIT 2016) 2016 22 27
- B. Latour On actor-network theory: a few clarifications Soz. Welt 47 1996 369 381
- S. LaValle E. Lesser R. Shockley M.S. Hopkins N. Kruschwitz Big data, analytics and the path from insights to value MIT Sloan Manag. Rev. 52 2011 21 31
- C. Leeuwis K.J. Cieslik M.N.C. Aarts A.R.P.J. Dewulf F. Ludwig S.E. Werners P.C. Struik Reflections on the potential of virtual citizen science platforms to address collective action challenges: Lessons and implications for future research NJAS-Wagening. J. Life Sci. 86–87 2018 146 157 https://doi.org/10.1016/j.njas.2018.07.008
- A.N. Leontyev Activity, Consciousness, and Personality 1978 Prentice‐Hall Englewood Cliffs
- D. Liang H. Delgado V. Cabrera A virtual dairy farm brain 13th European International Farming Systems Association Symposium 2018Available athttp://ifsa.boku.ac.at/cms/fileadmin/Proceeding2018/Theme4_Liang.pdf
- E.D. Lioutas C. Charatsari Green innovativeness in farm enterprises: what makes farmers think green? Sustain. Dev. 26 2018 337 349 https://doi.org/10.1002/sd.1709
- E.D. Lioutas C. Charatsari M. De Rosa G. La Roca Knowledge and innovation in the agrifood supply chain: Old metaphors and new research directions 13th International Farming Systems Association Symposium 2018Available athttp://ifsa.boku.ac.at/cms/fileadmin/Proceeding2018/1_Lioutas.pdf
- E.A. Locke G.P. Latham A Theory of Goal Setting and Task Performance 1990 Prentice-Hall Englewood Cliffs
- C. Loebbecke A. Picot Reflections on societal and business model transformation arising from digitization and big data analytics: a research agenda J. Strateg. Inf. Syst. 24 2015 149 157 https://doi.org/10.1016/j.jsis.2015.08.002
- C. Lundström J. Lindblom Considering farmers’ situated knowledge of using agricultural decision support systems (AgriDSS) to Foster farming practices: the case of CropSAT Agric. Syst. 259 2018 9 20 https://doi.org/10.1016/j.agsy.2017.10.004
- R.F. Lusch S.L. Vargo Service-dominant logic: reactions, reflections and refinements Mark. Theory 6 2006 281 288 https://doi.org/10.1177/1470593106066781
- J.E. Mai Big data privacy: the datafication of personal information Inf. Soc. 32 2016 192 199 https://doi.org/10.1080/01972243.2016.1153010
- J.T. Mentzer W. DeWitt J.S. Keebler S. Min N.W. Nix C.D. Smith Z.G. Zacharia Defining supply chain management J. Bus. Logist. 22 2001 1 25 https://doi.org/10.1002/j.2158-1592.2001.tb00001.x
- R. Miettinen The riddle of things: activity theory and actor‐network theory as approaches to studying innovations Mind Cult. Act. 6 1999 170 195 https://doi.org/10.1080/10749039909524725
- R. Miettinen Artifact mediation in Dewey and in cultural-historical activity theory Mind Cult. Act. 8 2001 297 308 https://doi.org/10.1207/S15327884MCA0804_03
- R. Miettinen Object of activity and individual motivation Mind, Cult. Act. 12 2005 52 69 https://doi.org/10.1207/s15327884mca1201_5
- S. Mithas M.R. Lee S. Earley S. Murugesan R. Djavanshir Leveraging big data and business analytics IT Prof. 15 2013 18 20 https://doi.org/10.1109/MITP.2013.95
- B.D. Mittelstadt L. Floridi The ethics of big data: current and foreseeable issues in biomedical contexts Sci. Eng. Ethics 22 2016 302 341 https://doi.org/10.1007/s11948-015-9652-2
- E. Morozov To Save Everything, Click Here: The Folly of Technological Solutionism 2013 Public Affairs New York
- J. Moura C. Serrão Security and privacy issues of big data N. Zaman M.E. Seliaman M.F. Hassan F.P.G. Marquez Handbook of Research on Trends and Future Directions in Big Data and Web Intelligence 2015 IGI Global Hershey 20 52
- D. Mwanza Where theory meets practice: a case for an activity theory based methodology to guide computer system design M. Hirose Proceedings of INTERACT’ 2001: Eighth IFIP TC 13 Conference on Human-Computer Interaction 2001 IOS Press Oxford
- D. Mwanza Y. Engeström Managing content in e-learning environments Br. J. Educ. Technol. 36 2005 453 463 https://doi.org/10.1111/j.1467-8535.2005.00479.x
- B.A. Nardi Activity theory and human-computer interaction B.A. Nardi Context and Consciousness: Activity Theory and Human-Computer Interaction 1996 MIT Press Cambridge 7 16
- R.R. Nelson K. Nelson Technology, institutions, and innovation systems Res. Policy 31 2002 265 272 https://doi.org/10.1016/S0048-7333(01)00140-8
- E. Noe H.F. Alroe Combining Luhmann and actor-network theory to see farm enterprises as self-organizing systems Cybern. Hum. Know. 13 2006 34 48 https://doi.org/10.1007/978-94-007-4503-2_17
- E. Noe H.F. Alrøe Observing farming systems: insights from social systems theory I. Darnhofer D. Gibbon B. Dedieu Farming Systems Research into the 21st Century: The New Dynamic 2012 Springer Dordrecht 387 403
- D.C. North Institutions, Institutional Change, and Economic Performance 1990 Cambridge University Press New York
- D. Nunan M. Di Domenico Market research and the ethics of big data Int. J. Sales Mark. Manag. Res. Dev. 55 2013 505 520 https://doi.org/10.2501/IJMR-2013-015
- D.M. Oliver P.J. Bartie A.L. Heathwaite L. Pschetz R.S. Quilliam Design of a decision support tool for visualising E. coli risk on agricultural land using a stakeholder-driven approach Land Use Policy 66 2017 227 234 https://doi.org/10.1016/j.landusepol.2017.05.005
- Organisation Internationale de la Vigne et du Vin Global Economic Vitiviculture Data. Press Release 2017(Accessed 10 January 2019)http://www.oiv.int/en/databases-and-statistics
- W.J. Orlikowski The duality of technology: rethinking the concept of technology in organizations Organ. Sci. 3 1992 398 427 https://doi.org/10.1287/orsc.3.3.398
- W.J. Orlikowski Using technology and constituting structures: a practice lens for studying technology in organization M.S. Ackerman C.A. Halverson T. Erickson W.E. Kellog Resources, Co-Evolution and Artifacts 2008 Springer London 255 305
- W.J. Orlikowski C.S. Iacono Research commentary: desperately seeking the “IT” in IT research - a call to theorizing the IT artifact Inf. Syst. Res. 12 2001 121 134 https://doi.org/10.1287/isre.12.2.121.9700
- E. Ostrom Institutional rational choice: an assessment of the institutional analysis and development framework P.A. Sabatier Theories of Policy Process 2007 Westview, Cambridge Press 21 64
- E. Ostrom Analyzing collective action Agric. Econ. 41 2010 155 166 https://doi.org/10.1111/j.1574-0862.2010.00497.x
- M.H. Padgavankar S.R. Gupta Big data storage and challenges Int. J. Comput. Scienc. Inf. Technol. 5 2014 2218 2223
- J.P. Pérez-Expósito T.M. Fernández-Caramés P. Fraga-Lamas L. Castedo VineSens: an eco-smart decision-support viticulture system Sensors 17 2017 465 https://doi.org/10.3390/s17030465
- M.G. Petersen K.H. Madsen A. Kjær The usability of everyday technology: emerging and fading opportunities ACM Trans. Comput. Hum. Trans. Comput.-Hum. Interact. 9 2002 74 105 https://doi.org/10.1145/513665.513667
- G. Phillips-Wren A. Hoskisson An analytical journey towards big data J. Desic. Syst. 24 2015 87 102 https://doi.org/10.1080/12460125.2015.994333
- F. Polese J. Pels B. Tronvoll R. Bruni L. Carrubbo A4A relationships J. Serv. Theory Pract. 27 2017 1040 1056 https://doi.org/10.1108/JSTP-05-2017-0085
- E. Porceddu R. Rabbinge Role of research and education in the development of agriculture in Europe Eur. J. Agron. 7 1997 1 13 https://doi.org/10.1016/S1161-0301(97)00031-2
- E. Rahm H.H. Do Data cleaning: problems and current approaches IEEE Data Eng. Bull. 23 2000 3 13
- L. Raja S. Vyas The study of technological development in the field of smart farming R.C. Poonia X.Z. Gao L. Raja S. Sharma S. Yyas Smart Farming Technologies for Sustainable Agricultural Development 2018 IGI Global Hershey 1 24
- T. Řezník V. Lukas K. Charvát Z. Křivánek M. Kepka L. Herman H. Řezníková Disaster risk reduction in agriculture through geospatial (Big) data processing ISPRS Int. J. Geoinf. 6 2017 238 https://doi.org/10.3390/ijgi6080238
- P. Ribarics Big Data and its impact on agriculture Ecocycles 2 2016 33 34 https://doi.org/10.19040/ecocycles.v2i1.54
- D.C. Rose C. Morris M. Lobley M. Winter W.J. Sutherland L.V. Dicks Exploring the spatialities of technological and user re-scripting: the case of decision support tools in UK agriculture Geoforum 89 2018 11 18 https://doi.org/10.1016/j.geoforum.2017.12.006
- W.M. Roth Emotion at work: a contribution to third-generation cultural-historical activity theory Mind, Cult. Act. 14 2007 40 63 https://doi.org/10.1080/10749030701307705
- D.R. Russell Russian activity theory M.L. Kennedy Theorizing Composition: A Critical Sourcebook of Theory and Scholarship in Contemporary Composition Studies 1998 Greenwood Press Westport 265 268
- R.M. Ryan E.L. Deci Self-determination theory and the facilitation of intrinsic motivation, social development, and well-being Am. Psychol. 55 2000 68 78https://doi.org/10.1037110003-066X.55.1.68
- S. Sagiroglu D. Sinanc Big data: a review International Conference on Collaboration Technologies and Systems 2013 42 47 https://doi.org/10.1109/CTS.2013.6567202
- A. Sannino Activity theory as an activist and interventionist theory Group Dyn. 21 2011 571 597 https://doi.org/10.1177/0959354311417485
- S.J. Scherr S. Shames R. Friedman From climate-smart agriculture to climate-smart landscapes Agric. Food Secur. 1 2012 19 https://doi.org/10.1186/2048-7010-1-12
- R.L. Schewe D. Stuart Diversity in agricultural technology adoption: how are automatic milking systems used and to what end? Agric. Rep. Inst. Hum. Values 32 2015 199 213 https://doi.org/10.1007/s10460-014-9542-2
- M. Shepherd J.A. Turner B. Small D. Wheeler Priorities for science to overcome hurdles thwarting the full promise of the ‘digital agriculture’ revolution J. Sci. Food Agric. 2019 https://doi.org/10.1002/jsfa.9346In press
- A. Sheth P. Anantharam C. Henson Physical-cyber-social computing: an early 21st century approach IEEE Intell. Syst. 28 2013 78 82 https://doi.org/10.1109/MIS.2013.20IEEE Intelligent Systems, 28(1), 78-82
- C. Spinuzzi “I think you should explore the kinky market”: how entrepreneurs develop value propositions as emergent objects of activity networks Mind, Cult. Act. 24 2017 258 272 https://doi.org/10.1080/10749039.2017.1294606
- M. Stubbs Big Data in US Agriculture, Report, 44331 2016 Congressional Research Service Washington DC
- M.E. Sykuta Big data in agriculture: property rights, privacy and competition in ag data services Int. Food Agribus. Manag. Rev. 19 2016 57 74
- J.L. Szalma Individual differences in human–technology interaction: incorporating variation in human characteristics into human factors and ergonomics research and design Theor. Issues Ergon. Sci. 10 2009 2009 381 397 https://doi.org/10.1080/14639220902893613
- L. Triste J. Vandenabeele F. Van Winsen L. Debruyne L. Lauwers F. Marchand Exploring participation in a sustainable farming initiative with self-determination theory Int. J. Agric. Sustain. 16 2018 106 123 https://doi.org/10.1080/14735903.2018.1424305
- J. van den Hoven D. Helbing D. Pedreschi J. Domingo-Ferrer F. Gianotti M. Christen FuturICT - the road towards ethical ICT Eur. Phys. J. Spec. Top. 214 2012 153 181 https://doi.org/10.1140/epjst/e2012-01691-2
- S.L. Vargo R.F. Lusch Institutions and axioms: an extension and update of service-dominant logic J. Acad. Market. Sci. 44 2016 5 23 https://doi.org/10.1007/s11747-015-0456-3
- S.L. Vargo R.F. Lusch Service-dominant logic 2025 Int. J. Res. Market. 34 2017 46 67 https://doi.org/10.1016/j.ijresmar.2016.11.001
- S.L. Vargo P.P. Maglio M.A. Akaka On value and value co-creation: a service systems and service logic perspective Eur. Manag. J. 26 2008 145 152 https://doi.org/10.1016/j.emj.2008.04.003
- T. Verwaart Big Data Opportunities for Marketing of Horticultural Products 2015(Accessed 12 January 2019)http://bigtu.nl/wp-content/uploads/2016/01/Big-Data-opportunities-for-marketing-of-horticultural-products.pdf
- L.S. Vygotsky Mind in Society. The Development of the Higher Psychological Processes 1978 Harvard University Press Cambridge
- J.S. Walker Big data: a revolution that will transform how we live, work, and think Int. J. Advert. 33 2014 181 183 https://doi.org/10.2501/IJA-33-1-18183
- K. Walley P. Custance Coopetition: insights from the agri-food supply chain J. Chain Netw. Sci. 10 2010 185 192 https://doi.org/10.3920/JCNS2010.x187
- F. Welter Contextualizing entrepreneurship—conceptual challenges and ways forward Entrep. Theory Pract. 35 2011 165 184 https://doi.org/10.1111/j.1540-6520.2010.00427.x
- J.V. Wertsch A sociocultural approach to socially shared cognition L.B. Resnick J.M. Levine S.D. Teasley Perspectives on Socially Shared Cognition 1991 American Psychological Association 85 100
- B.E. Whitacre T.B. Mark T.W. Griffin How connected are our farms? Choices 29 2014 1 9
- S. Wolfert C.A.G. Sorensen D. Goense A future internet collaboration platform for safe and healthy food from farm to fork. Global Conference (SRII) 2014 Annual SRII, IEEE 2014 266 273 https://doi.org/10.1109/SRII.2014.47
- S. Wolfert L. Ge C. Verdouwa M.-J. Bogaardt Big data in smart farming – a review Agric. Syst. 153 2017 69 80 https://doi.org/10.1016/j.agsy.2017.01.023
- C. Yang Q. Huang Z. Li K. Liu F. Hu Big Data and cloud computing: innovation opportunities and challenges Int. J. Digit. Earth 10 2017 13 53 https://doi.org/10.1080/17538947.2016.1239771
- J. Zeng L.T. Yang M. Lin H. Ning J. Ma A survey: cyber-physical-social systems and their system-level design methodology Future Gener. Comput. Syst. 2019 https://doi.org/10.1016/j.future.2016.06.034In press
- Y. Zhang A.L. Collins R.D. Gooday Application of the FARMSCOPER tool for assessing agricultural diffuse pollution mitigation methods across the Hampshire Avon Demonstration Test Catchment, UK Environ. Sci. Policy 24 2012 120 131 https://doi.org/10.1016/j.envsci.2012.08.003
- P. Zikopoulos C. Eaton Understanding Big Data: Analytics for Enterprise Class Hadoop and Streaming Data first ed. 2011 McGraw-Hill Osborne Media New York