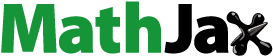
Highlights
• | There are differences in the experienced level of job satisfaction between AMS farmers and CMS farmers. | ||||
• | AMS farmers are more satisfied with their working day, work safety and working conditions. | ||||
• | These factors increase job satisfaction for all dairy farmers: Increased income, new cowshed, successor, continue farming. |
Abstract
Innovation and implementation of new technology in farming is considered important to meet challenges for agriculture to increase sustainability and improve efficiency in production. Less emphasise has been on how the farmers experience the new technology. This paper responds to this gap and explores how Automatic Milking Systems (AMS) influence farmers’ job satisfaction. The research questions are: Are there differences in the experienced level of job satisfaction between AMS farmers and farmers applying Conventional Milking Systems (CMS)? Which factors determine the level of job satisfaction in dairy farming? Do these factors vary on AMS farms compared to CMS farms? The empirical data is based on a survey to a sample of dairy farmers with AMS and CMS. The results show that the most important factors which influence job satisfaction positively are common for AMS and CMS; Increased income, new cowshed, there is a successor present, farmer wants to continue farming. Contrary, higher education and being a male reduces job satisfaction. Further, the results show that AMS farmers are more satisfied with their working day, their occupational safety and their working environment. Other factors which influence job satisfaction for dairy farmers are; Less paper work, working together, considering technological competence less important, being appreciated, considering economic competence less important, increasing milk quota, loneliness and health worries. However, these factors can to a varying degree be explained from an AMS/CMS perspective, even though they differ between the two technologies.
1 Introduction
To be a farmer is to combine a profession and a way of life. It is a complex existence that includes skills involving business management, agronomy, production etc, and a way of life related to issues like identity, personality, life style, gender, family household and part time work outside the farm (CitationStock and Forney, 2014; CitationMilone and Ventura, 2018; CitationBjørkhaug and Blekesaune, 2008). An important factor which integrates this complexity is job satisfaction, at least as important for farmers as for other professions. Whereas several studies have explored the relationship between farming and aspects of quality of life and well-being (CitationBesser and Mann, 2015; CitationHaugen and Blekesaune, 2005; CitationPeel et al., 2016; CitationBrew et al., 2016), less is done on job satisfaction, particularly in relation to specific technologies and production methods. Automatic Milking Systems (AMS) are gaining popularity on medium-sized or family-based dairy farms, where they are replacing Conventional Milking Systems (CMS). The implementation of technology in agriculture has a strong influence on the working conditions for farmers. However, how do the farmers experience the effect of new technology in their working day?
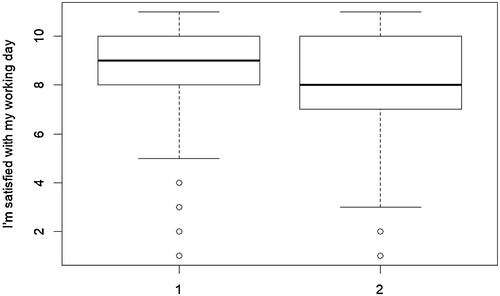
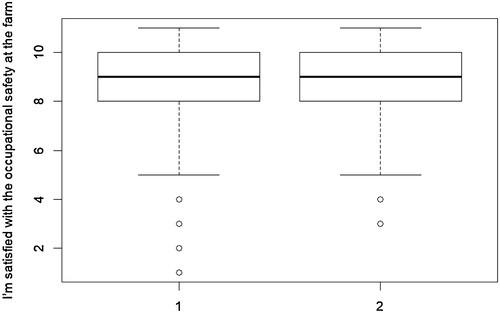
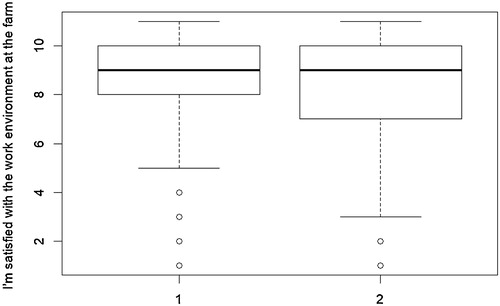
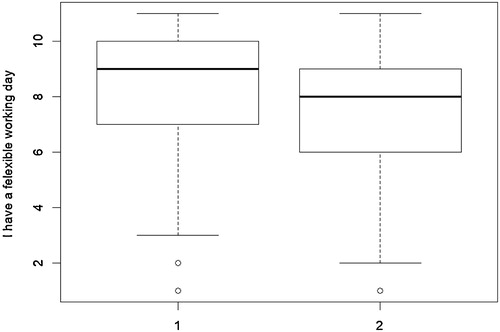
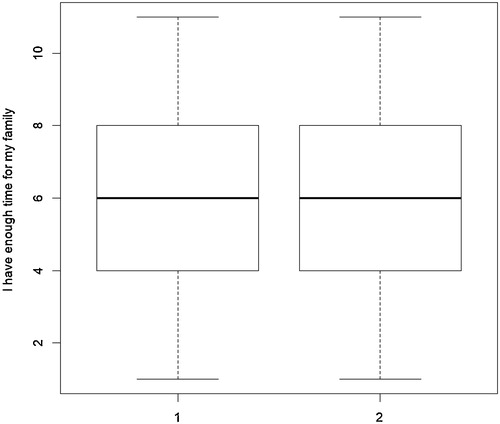
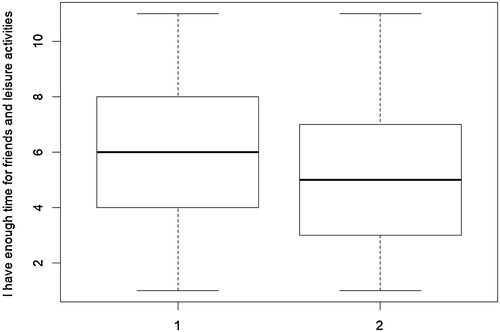
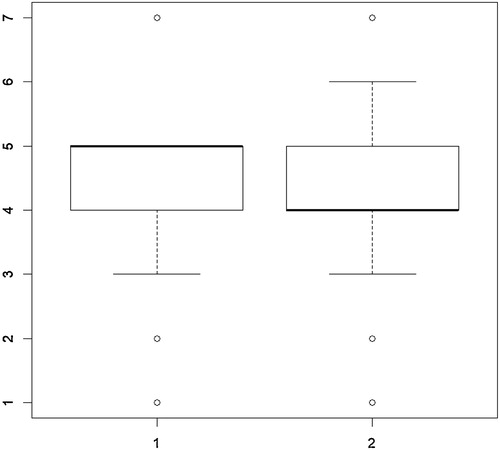
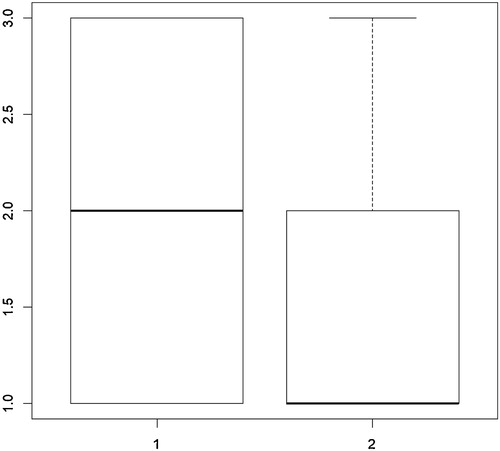
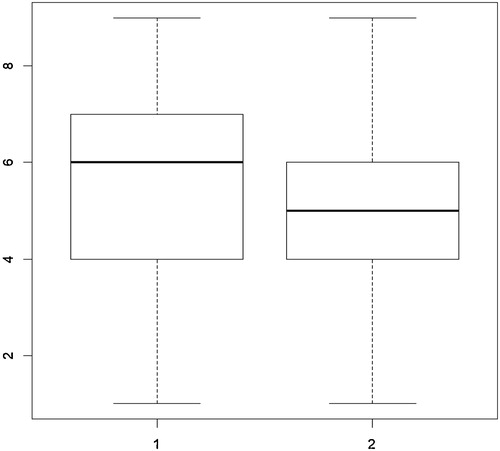
A main motivation for farmers to invest in AMS is to achieve a more flexible working day (CitationHansen, 2015; CitationStræte et al., 2017; CitationHårstad, 2019; CitationRodenburg, 2017). Less research is done on how AMS influences the job satisfaction when compared to farmers with other milking systems. This study attempts to fill this research gap. In this paper the aim is to explore and elucidate which factors determine farmers’ job satisfaction and whether these factors differ between farmers with AMS and without AMS. The remainder of the paper is organized as follows: First we present the Norwegian context and a theoretical approach to job satisfaction, and this is followed by a description of materials and methods, results, discussion and conclusions.
1.1 Norwegian agriculture
Norwegian agriculture has undergone major changes in recent years, and the number of farmers has decreased. Compared with the early 1960s, agriculture is now much more specialized and mechanized, and it is characterized by increased production, efficiency and workload (CitationAlmås, 2002). Most farms continue to be family owned and operated businesses, though some joint farming operations also exist. The average farm unit runs around 22 ha, and the average herd size is approximately 25 cows. Since 1983 Norwegian dairy farms have had milk quotas, and milk quotas can be traded among farmers. From their introduction in the early 2000s up to 2018, the installation of AMS has increased to involve 50 per cent of the milk production in Norway. The AMS technology has changed the working day and style of life for many farmers in several ways (CitationHansen, 2015; CitationHårstad, 2019; CitationVik et al., 2019). Norwegian AMS farms have significantly larger acreage and a higher share of joint farming operations compared to CMS farms, and the farms need 35–40 cows for AMS to be more profitable than CMS, i. e. a significant larger herd than the average. Adoption rates in Norway can i.e. be explained by human and social capital, socio-cultural factors, the agricultural knowledge system, a strong belief in technology and difficulties of getting skilled labor (CitationHansen, 2015). In this perspective, CMS is defined as the milking system a traditional and average dairy farm applies in a Norwegian context, i.e. pipeline milking or milking parlour.
1.2 Theory on job satisfaction
Job satisfaction refers to the positive attitudes or emotional dispositions people may gain from work or through aspects of work. Thus, CitationPrice (2001) defines job satisfaction as the affective orientation that an employee has towards his or her work. It can be considered as a global feeling about the job or as a related constellation of attitudes about various aspects or facets of the job. Job characteristics approach research has revealed that the nature of an individual’s job or the characteristics of the organization predominantly determines job satisfaction (CitationJex, 2002). A common premise in research of the effects of job circumstances on job satisfaction is that individuals assess job satisfaction by comparing the current receivables from the job with what they believe they should receive (CitationJex, 2002). Thus, Locke’s theory (CitationLocke, 1976) suggests that low job-satisfaction is caused by the difference between what one wants and values, and the experiences one receives from work. If the results of the workers’ efforts, e.g. economic compensation, are in line with their wished goals and values, workers will be satisfied. How important and valuable a wish or a value is for the worker also plays a role in determining the degree of satisfaction. In line with this, [CitationSteinhardt et al., 2003:1] define job satisfaction as “a pleasurable or positive emotional state resulting from appraisal of one’s job experiences as fulfilling important job values”. Based on a literature review of the most popular job satisfaction instruments, CitationSpector (1997) summarized the following facets of job satisfaction: appreciation, communication, co-workers, fringe benefits, job conditions, nature of the work itself, the nature of the organization itself, an organization’s policies and procedures, pay, personal growth, promotion opportunities, recognition, security and supervision.
Individual worker characteristics have also received attention in explaining job satisfaction (CitationJex, 2002). For example, some people are inclined to be satisfied or dissatisfied with their work irrespective of the nature of the job or the organizational environment (CitationJex, 2002). More simply put, some people are genetically positive in disposition, whereas others are innately negative in disposition. Further, individuals with a high degree of achievement motivation may become frustrated if the workplace offers little room for challenges and possibilities to unfold oneself. Finally, social information processing may also affect job satisfaction (CitationJex, 2002). According to this view employees look to co-workers to make sense of and develop attitudes about their work environment. In other words, if employees find their co-workers positive and satisfied then they will most likely be satisfied; however, if their co-workers are negative and dissatisfied then the employee will most likely become dissatisfied. In a similar vein CitationSalancik and Pfeffer (1978) claim that people tend to compare themselves with others in comparable jobs and judge how satisfied they are compared to their own degree of satisfaction. According to CitationKaufmann and Kaufmann (2009) this view has been backed by several studies. Taken together, job satisfaction is a multifaceted phenomenon consisting of interaction between the individual need, values and expectations, on one side, and what the job or working situation offers the individual on the other side. Thus, the working environment plays an important role in determining job-satisfaction. Following this perspective there is a need to have a holistic perspective to understand and consider job satisfaction at an individual level. Nevertheless, there is a need to emphasise elements and specific variables to increase knowledge on what factors influence job satisfaction or not at an aggregated level, and to find the hierarchy of the factors, to see how they contribute to job satisfaction as a whole.
1.3 Previous studies
Previous studies of job satisfaction have shown that self-employed women are more satisfied than employees, particularly the share of women who report high job-satisfaction (CitationSSB, 2009). The higher degree of freedom and self-determination at work as compared to employees may be one reason (CitationSSB, 2009). Low-educated workers thrive better at work than highly educated, particularly the share who report high job-satisfaction is larger (CitationSSB, 2009). Thus, highly educated workers, both men and women, more often report a medium score on job-satisfaction (CitationSSB, 2009). Accordingly, this finding most likely has little to do with the actual working conditions but relates to different demands and expectations. The more highly educated ones have higher demands and expectations of the working place (CitationSSB, 2009), an explanation in line with the process theories of job-satisfaction.
Satisfaction with various job characteristics is also related to the overall quality of life (CitationEvans et al., 1993). CitationPhillips (2006) defines it in this way: “Quality of life requires that people’s basic and social needs are met and that they have the autonomy to choose to enjoy life, to flourish and to participate as citizens in a society with high levels of civic integration, social connectivity, trust and other integrative norms including at least fairness and equity, all within a physically and socially sustainable global environment” [Phillips, 2006: 242]. Based on this and other definitions, a commission suggested a list of the basic components of quality of life consisting of 11 points. Among these are economic security, work and education, social community and care (CitationNorwegian Health Authority, 2016). We think these components are also relevant for farmers. The level of job satisfaction has also been related to quality of life and job stress; job dissatisfaction and mental distress may contribute to illness (CitationEvans et al., 1993; CitationMomose et al., 2008).
It has been noted that “Farmers are almost unique as a group whose work is so intimately tied with every aspect of their lives and the lives of their families, often across several generations” [- CitationGregoire, 2002: 472]. Farmers and their family members should be regarded as an occupational group at risk of mental distress (CitationZejda et al., 1993), and health promotion programs on job satisfaction are therefore important (CitationEvans et al., 1993). Farmers may work long hours, have physically demanding work, are often isolated socially and geographically from services, are less likely to take vacations and less likely to retire than people in other occupations (CitationThelin and Holmberg, 2010; CitationFragar et al., 2008). In addition, farming has undergone major changes over recent decades with regards to globalization, economic rationalization (CitationFjellhammer, 2013) diminishing rural populations and climate change. Therefore, farmers could be considered a vulnerable population and the association between work and health is particularly pertinent for their livelihood and well-being (CitationBrew et al., 2016).
In an automatic milking system (AMS), cows are enticed by concentrate feed to enter the milking stall, where the milking robot cleans the teats, attaches the teat cups, milks the udder on a quarter-basis and detaches the teat cups. Thus, conversion to an AMS radically changes the work routines of farmers as compared to conventional milking systems (Citationde Koning, 2010; CitationButler et al., 2012). Therefore, AMS have the potential to influence on dairy farmers’ job satisfaction. Thus, demonstrated benefits of AMS include reduced labor and more hours of sleep (CitationHansen, 2015; CitationBijl et al., 2007), a better social life and a more flexible lifestyle for dairy farmers and their families (Citationde Koning, 2010; CitationHansen, 2015). Other benefits include more interesting or less routine activities for the farmers (CitationJacobs and Siegford, 2012; CitationWoodford et al., 2015; CitationTse et al., 2017). However, an AMS has higher capital costs (CitationWade et al., 2004), and requires farmers to be on-call 24/7 (CitationHansen, 2015). AMS also makes management more data-based (CitationButler et al., 2012), potentially involving risk of technostress (CitationFuglseth and Sörebö, 2014). Further, a change to AMS involves a major restructuring of farm operations and a huge managerial challenge (CitationHansen and Jervell, 2014), with the potential to induce stress. Whereas some knowledge exists regarding job satisfaction in dairy farming, we know less about whether and how job satisfaction varies between AMS farmers and CMS farmers.
Our research questions are:
1) | Are there differences in the experienced level of job satisfaction between AMS farmers and CMS farmers? |
2) | Which factors determine the level of job satisfaction in dairy farming? |
3) | Do these factors vary on AMS farms compared to CMS farms? |
2 Materials and methods
2.1 Factor analysis
In this paper we apply factor analysis to examine the covariation among the set of observed variables to gather information on their underlying latent constructs or factors. We also use matching to make CMS farms more comparable with AMS farms. The basic idea of factor analysis is the following: For a given set of observed response variables ,…., one wants to find a set of underlying factors ,…, , being much fewer in number than the observed variables. These factors are supposed to account for the correlations of the response variables in the following way (CitationThurstone, 1947):
= +…….+ , , ,…, , where , the measurement error for , is uncorrelated with ,…., and with for (CitationJöreskog et al., 2016). Further, Var () = and E () = 0. Given the factor, the observed variables are independent of one another, cov(, ) = 0. This means that the ’ s are only related to each other through their common relationship with . Thus, the correlation between and , corr (, for a standardized , corr (, ) = .
The objective of factor analysis is to estimate the number of factors and the associated factor loadings ,…, . Factor loadings are equivalent to the correlation between factors and variables when only a single common factor is involved. If is N (0,1), then is equivalent to the correlation between and . Thus, to understand the structure and meaning of an unobserved or latent variable in the context of its’ manifest variables is the main goal of factor analysis.
A confirmatory factor analysis (CFA) begins by defining the latent variable one wants to measure (CitationJöreskog et al., 2016), based on both theory and previous knowledge. Thus, we constructed a set of observable variables or items to measure the latent variable job satisfaction. The CFA was statistically estimated and tested. Then we used a set of explanatory variables to explain the variation in the factor in a structural equation model (SEM) (CitationJöreskog et al., 2016). An SEM is an extension of the classical factor analysis where the goal is to use the factors themselves as predictors or outcome variables in further analyses. Thus, a SEM specifically expresses the effect of latent variables on each other and the effect of latent variables on observed variables. To analyse the data, we applied the Lavaan package in the statistical software R (CitationCRAN, 2018). Following the recommendations of (CitationCRAN, 2018), we applied polychoric correlations and diagonally weighted least squares (DWLS) for ordinal variables. Compared to maximum likelihood, DWLS often provides a model fit that is more robust to variable type and non-normality (CitationMîndrilă, 2010).
To compare our items across the two groups we use a linear-by-linear association test for ordered contingency tables (CitationAgresti, 2007) implemented in the ‘coin’ package in R.
2.2 Matching
To make CMS farms more comparable with AMS farms we chose the variable Construction year as the matching variable. This variable signals a group of CMS farmers who are willing to invest for the future, an assumption which also holds for most AMS farm. To match we use the ‘MatchIt’ package in R. We apply propensity score matching (see e.g. CitationRandolph et al., 2014), as this technique matches a treated unit (a CMS farm) with the control units (AMS farms) that are closest in terms of a distance measure, such as a logit. The matching process helps strengthen causal arguments in quasi-experimental studies like this by reducing selection bias (see e.g. CitationCook and Campbell, 1979). After testing different methods, we chose nearest-neighbour matching and three neighbours, because it resulted in the lowest mean differences between the two groups.
2.3 The empirical study
Participants in this study responded to a web-administered questionnaire to 3400 dairy farmers carried out in late autumn 2017. Data were collected for a larger study about AMS. The aim of the study was to explore how farmers perceive their quality of life, their working situation and mental health, the future of their farm, work division between family members, income etc. To compare farmers with and without AMS, the questionnaire was distributed to all 1700 farmers in Norway registered with an AMS autumn 2017, and to 1700 randomly selected dairy farmers with conventional milking systems. After two follow-up emails the overall response rate of the AMS-survey was 38 %, 43.5 % among AMS farmers and 32.2 % among CMS farmers. The relatively low response-rate among CMS farmers may be related to the fact that some sections of the questionnaire were related directly to the use of AMS, and this may have reduced CMS farmers’ interest in the survey.
In total, 1091 of the 1288 responding farmers are men and 197 are women. Their age ranged from 22 to 78 years, with a mean of 47.1 for AMS farmers and 48.7 for CMS farmers. In total, 1112 farmers had a wife or live-in partner, while 176 were single. Altogether 248 farms were run as joint operations, which means that two or more farmers merge their milk quotas, herds and farmland, and deliver all milk from one farm only. Altogether 739 farmers had an AMS, and the average cowshed was refurbished for the last time in 1998. On AMS farms the average year of construction was 2008, and for CMS farms 1984. However, about half of the CMS farmers have renewed their cowshed in their period as farmers, and for those the average construction year was 2008. Of the CMS farms, 80.1 % have pipeline milking, and 19.9 % have a milking parlour. The main difference between the two systems is that with pipeline milking cows are stalled in stanchion barns, while with milking parlour the cows are in loose housing. While management practices in stanchion barns and loose housing are somewhat different, in both systems milking involves manual work to cleans the teats, attach the teat cups, milk the udder and detach the teat cups. However, both systems differ significantly from an AMS, where cows enter the milking system themselves and the system performs the whole milking operation. AMS also involves a complete management system which delivers much more detailed data on each cow and each milking as compared to pipeline milking or milking parlour. Therefore, introduction of an AMS represents a huge managerial change, in terms of removing routine contact between humans and animals, and of unsettling the usual ways in which farmers know and understand their cows. As such, conversion to a milking robot radically changes the work of the stockperson (CitationButler et al., 2012), and requires a transformation of the whole management process.
In our SEM-model, three items describe farmers’ job-satisfaction; I’m satisfied with my working day (Item 1), I’m satisfied with the occupational safety at the farm (Item 2) and I’m satisfied with the work environment at the farm (Item 3). From theory we know that the working environment is important in determining job-satisfaction. We think these three items together express positive attitudes or emotional dispositions farmers gain from their work. The items cover different facets of the job. The first and third item aim to reveal farmers attitudes towards their job at an overall level. While item one covers farmers’ feelings about the nature of the work itself, item three covers both physical and psycho-social aspects of the working environment. The second item covers job security, a more specific yet important facet of the job. We argue that a high score on all three items signals that farmers assess the receivables from the job in line with what they think they should receive. There is a balance between the individual needs and expectations, on one side, and what the job or working situation offers the individual on the other side.
Respondents were asked to mark on a Likert scale ranging from 1 to 11 whether they strongly disagreed or agreed with the three claims raised. The median value of Item 1 is 9 for AMS farmers and 8 for CMS farmers, for Item 2 and Item 3 the median values are 9 for both groups. Thus, in general the farmers are quite satisfied with their job, yet AMS farmers are somewhat more satisfied. In the Appendix we show boxplots of the three items. From the plots we can see that although the median values are similar for the two groups, the answers from CMS farmers vary more compared to the answers from the AMS farmers. We also use the following two variables as dependent variables in linear by linear association tests: “I have a flexible working day “, and “I have enough time for friends and leisure activities”. A similar Likert scale was used. For details we refer to the Appendix.
To explain the variation in factor job-satisfaction, we use the following independent or observed variables: Automatic milking system (AMS), size of milk quota (Quota), how likely it is that the farmers will continue farming the next five to ten years, ranging from very likely to very unlikely (Continue), how likely it is that family members will take over the farm, ranging from very likely to very unlikely (Successor), by how many percent they have increased their milk production the last ten years (Prod-increase), their total farm income (Income), whether they work most of the time alone or together with others during the working day (Work together), how much of their time was spent on farm economy and accounting (Paper work), their highest completed education level (Education), the last year when the cowshed was renewed or built (Cowshed year), how important they consider technical competence (Techn-comp.) or economic competence (Econ-comp.) during their workday, and finally whether they have been worried about their own health in relation to continuing farming (Health worries). For the variables Education, Income, Successor, Prod-increase, Work together, Continue, Techn-comp., Eco-comp., Health worries and the respondents were asked to mark their answer on a scale with a number of pre-specified alternatives. For Health worries a Likert scale ranging from 1 to 11 was used.
Most farmers, 842 or 65 % have high school as their highest education level, 228 have university or university college education of up to four years, and 106 beyond four years. In total 450, farmers or 35 % have no agricultural education. The median milk quota was 253.696 L, and the average 276.743 L, which is well beyond the national average in 2017 of 186.788 L (CitationNorwegian Agriculture Agency, 2019). AMS farmers had a quota more than twice the size of CMS farmers, 373 388 L versus 146 652 L. While most farmers (38 %) have increased production by less than 30 % over the last ten years, 373 or 29 % have increased production by more than 100 %. In total, 982 farmers or 76 % answered that they most likely will continue farming five to ten years ahead, 144 or 11 % felt sure they would quit dairy farming during the same period, while the rest were uncertain. Similarly, 611 farmers or 47 % answered that it was most likely that someone within the family will take over their farm, 149 considered it unlikely, while 528 or 41 % were uncertain. Here one should keep in mind that the alternative “uncertain” also includes farms where the children are currently so small that the question is of little relevance. In total 52 % of the farmers had an income between 200.000 and 499.000 NOK, while 17 % had an income below 199.999 NOK, and 30 % beyond 500.000 NOK. AMS and CMS farmers differ when it comes to total hours of work and how much of the time they work together with others. While 60 % of the AMS farmers worked more than 2500 h per year and 43.6 % of the hours alone, the corresponding figures for CMS farmers are 45 % and 58.8 %.
3 Results
A test of the items for skewness and kurtosis showed that all values are well within +/- 2, which is considered acceptable to prove normal univariate distribution (CitationGravetter and Wallnau, 2014). Similarly, tests of multivariate skewness and kurtosis (CitationMardia, 1970) revealed no signs of significant deviations from normality. The correlations are shown in .
Table 1 Spearman rank correlations among the items and variables in the study.
In we can see that Loneliness and Health worries are most strongly correlated with the three items. Further, Appreciate, Continue and Successor also show clear relationships with the items. We also notice the significant negative correlation between Eco-comp. and Techn-comp.
A linear-by linear test of the three items across the two groups show that AMS farmers score significantly higher on items 1,2 and 3 with Z= -5.999 (P < 0.001), Z= -2.178 (P < 0.005) and Z= -5.967 (P < 0.001), respectively. This shows that AMS farmers experience a higher job satisfaction compared to CMS farmers.
In we present our model of job satisfaction for all 1288 dairy farms. In and 3 the variables Continue and Successor are reverse- coded, so that negative values mean a high probability of continuing or having a successor.
Fig. 1 Path diagram for the SEM for all 1288 farms, with standardized factor loadings and measurement errors, and the regression coefficients between the explanatory variables and job satisfaction.
*P ≤ 0.05, **P ≤ 0.01, ***P ≤ 0.001
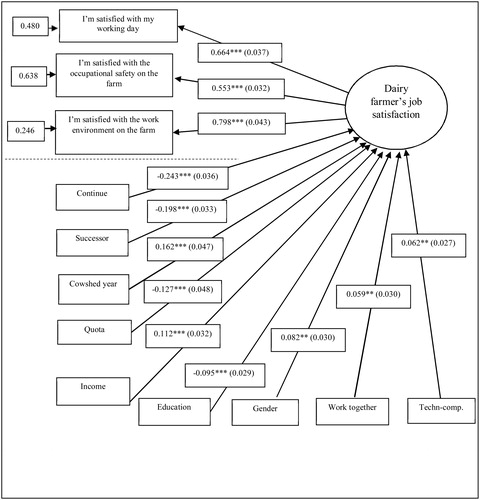
All factor loadings are significant and greater than 0.5. The theoretical model provides a good fit to the observed data with a Chi- square value of 26.696 (P = 0.093). Other indices (0.000 < RMSEA = 0.019 < 0.034, SRMR = 0.018, TLI = 0.984, CFI = 0.991) also point to a good model fit. The reliability composite measure is 0.749, and Chronbach’s alpha 0.769. Both measures indicate that the items included in the model are reliable measures of the construct.
In the level of job satisfaction increases with increasing income and construction year of the cowshed. Working together with others, having a successor, an intention to continue farming and considering technical competence as less important also contribute positively to job satisfaction. Job satisfaction reduces with increasing level of highest education, quota and being a male.
In we present the model for the 739 AMS farms.
Fig. 2 Path diagram for the SEM for the 739 AMS farms, with standardized factor loadings and measurement errors, and the regression coefficients between the explanatory variables and job satisfaction.
*P ≤ 0.05, **P ≤ 0.01, ***P ≤ 0.001
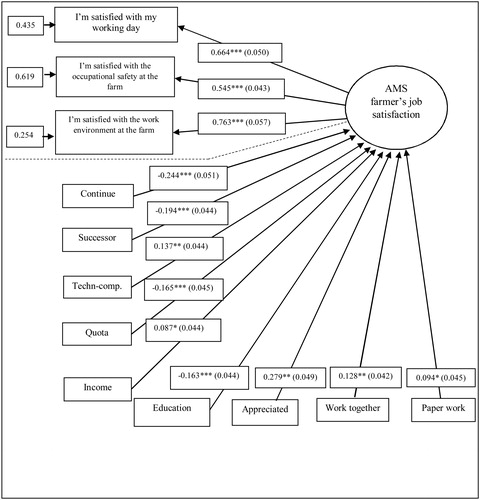
All factor loadings are significant and greater than 0.5. The theoretical model provides a good fit to the observed data with a Chi- square value of 14.941 (p = 0.666). Other indices (0.000 < RMSEA = 0.000 < 0.027), SRMR = 0.018, TLI = 1.010, CFI = 1.000) also point to a good model fit. The reliability composite measure is 0.751, and Chronbach’s alpha 0.783. Both measures indicate that the items included in the model are reliable measures of the construct.
In the level of job satisfaction for AMS farmers increases with increasing income, spending less time on paper work, working together with others, considering technical competence less important, a feeling of being appreciated as a farmer, having a successor and an intention to continue farming. Job satisfaction reduces with increasing quota and level of education. A linear-by-linear association test shows that AMS farmers work significantly more hours compared to CMS farmers, Z= - 4.930 (P < 0.001) and more often work together with others during their working day, Z= -5.357 (P < 0.001). However, despite the more hours worked, AMS farmers think they have a more flexible working day, Z= -6.461 (P < 0.001), more time for their family, Z= -2.420 (P < 0.05), and more time for friends and leisure activities, Z= -5.967 (P < 0.001) compared to CMS farmers.
The job-satisfaction model for the CMS farmers is in .
Fig. 3 Path diagram for the SEM for the 549 CMS farms, with standardized factor loadings and measurement errors, and the regression coefficients between the explanatory variables and job satisfaction.
*P ≤ 0.05, **P ≤ 0.01, ***P ≤ 0.001
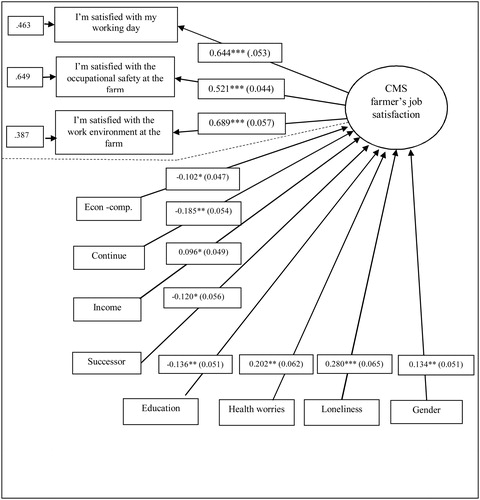
In we can see that all factor loadings are significant and greater than 0.5. The theoretical model provides a good fit to the observed data with a Chi- square value of 22.483 (P = 0.128). Other indices (0.000 < RMSEA = 0.027 < 0.051), SRMR = 0.028, TLI = 0.975, CFI = 0.985) also point to a good model fit. The reliability composite measure is 0.724, and Chronbach’s alpha 0.745. Both measures indicate that the items included in the model are reliable measures of the construct. In the level of job satisfaction for CMS farmers increases with income, considering economic competence less important, having a successor and an intention to continue farming. Job satisfaction reduces with an increasing level of education, a feeling of loneliness, health worries and being a male.
The results from the matching procedure used to make AMS farms and CMS farms comparable with respect to cowshed year are in . We matched all CMS farms with all AMS farms to obtain similar values of the variable Cowshed year.
Table 2 Results from matching AMS farms and CMS farms on the variable Cowshed year.
In we can see that without matching the CMS farms on average have cowsheds that are 24 years older compared to the AMS farms. However, after matching the cowsheds are on average equally old. This shows that matching worked well for this data, although we lost more than half of the CMS farms.
Linear-by-linear association tests comparing the AMS and the CMS farms after matching showed that AMS farmers score significantly higher on all three items with Z= - 4.691 (P < 0.001), Z= - 2.301 (P < 0.05) and Z= - 4.204 (P < 0.001), respectively. This shows that AMS has a positive effect on satisfaction with the working day, occupational safety and the working environment compared to CMS, in addition to the general positive effect of a new cowshed for all farms.
In we summarize our findings.
Table 3 Summary of how different factors affecting job satisfaction relate to milking system.
4 Discussion
Our results suggest that AMS farmers experience a higher satisfaction with the working day, the occupational safety and the working environment compared to CMS farmers. However, as shown in , construction year and not AMS enters the model for the whole group of farmers. The reason is that AMS are only available on 57 % of the farms in the whole sample, while construction year data is available on all farms. This means that a new cowshed increases job satisfaction for all dairy farmers, and there is an AMS-effect in addition. In the following we discuss what increases and decreases job satisfaction on AMS and CMS farms.
4.1 What increases job satisfaction?
In general, for the dairy farmers the level of job satisfaction increases with increasing income, and there is no difference in this between AMS farmers and CMS farmers. This finding is as expected, so even though several factors influence job satisfaction, income matters (CitationBesser and Mann, 2015).
For both types of farming, the more recent the construction year of the cowshed, the higher the level of job satisfaction. This is as expected, a new cowshed means new and better working conditions; being easier, safer and more comfortable. It is also related to having an optimistic and long-term perspective for the future. Finally, the symbolic value of a new cowshed may also give a satisfaction. A new and up-to-date cowshed supports the image of being a good and dynamic farmer, showing for the neighbours that the farmer is doing well. However, this variable does not differ between AMS and CMS farmers.
Further, the results suggest that having a successor and an intention to continue farming increases job satisfaction for both AMS farmers and CMS farmers. This finding is as expected, since succession is an important factor to remain farming (CitationDeFrancesco et al., 2018), and an intention to exit farming is associated with a poorer sense of well-being (CitationHansen and Greve, 2015; CitationPeel et al., 2016). It is more likely that there is a successor when there is farm size increase, income increase, and when children take part in work at the farm (CitationZahl-Thanem et al., 2018). We also recognize that there are differences between AMS and CMS farmers concerning which variables increase the level of job satisfaction. For AMS farmers job satisfaction increases with:
- | Spending less time on paper work related to management of farm economy and accounting: This may suggest two alternative explanations. Firstly, AMS farmers may prefer to be more hands-on in the cowshed and spend less time on paper work. Secondly, AMS farmers are more competent and/or more efficient at paper work than CMS farmers. Other research indicates that the latter alternative is the most likely one, as AMS farmers often involve advisors and supporters in their network to solve an increasing number of tasks (CitationStræte et al., 2017). |
- | Working together with others, which is also positive for all dairy farmers: From this we can infer that AMS farmers are more satisfied when or as they work together with others. We don’t know if they are more social than CMS farmers. However, we know that they are more likely to work together with others regularly, which contributes to their need to be social. |
- | Considering technical competence less important (this is also true for all dairy farmers). That AMS farmers who consider technical competence less important experience higher job satisfaction seems to be a rather paradoxical finding at first glance, as AMS is more technical than CMS. However, this may not be so surprising considering that AMS and related technology is so high tech that most farmers get used to needing experts to solve problems. Hence, they are familiar with calling the experts when in trouble, and do not need to be an expert themselves. A supplementary explanation is that AMS farmers are more interested and skilled in working with technology. If so, they take their competence for granted and not something that needs to be emphasized. |
- | For AMS farmers, a feeling of being appreciated as a farmer by others in society increases the satisfaction of being a farmer. Recognition is a motivational factor that increases satisfaction. A reflection why CMS farmers are not in the same category here can be that the milking robot and AMS is a hot issue receiving the most recognition among those who discuss and care about dairy farming. CMS farmers may be the ignored majority – there being an attitude that CMS and tie-stalls belong to the past. Thus, CMS farmers do not experience the same appreciation as AMS farmers. |
- | The findings that AMS farmers feel they have more time for friends and leisure activities can explain why they are more satisfied with their job in general. |
The only variable where the level of job satisfaction increases only for CMS farmers, is considering economic competence less important. A reasonable explanation of this finding is that among CMS farmers we find a kind of farmers that are in a stable production with few changes and relatively low risk, i.e. being in a predictable economy that does not cause major worries.
4.2 What reduces job satisfaction?
The results suggest that education, quota, gender, feeling of loneliness, and health worries all have a negative influence on job satisfaction. However, the influence differs somewhat between AMS and CMS farmers. A high level of education reduces the level of job satisfaction both for AMS and CMS farmers. An explanation may be that a greater proportion of farmers with a higher level of education, not just agricultural education, are part time farmers. Particularly for dairy farmers, this may create a situation where they experience shortcoming in both farming and their other job. Further, more years of higher education involves being away from the farm for longer periods and experiencing other ways of living. Most likely the relationship can be explained by the process-theories on job-satisfaction, namely as a discrepancy between job expectations and demand on side, and actual experiences on the other. Our finding supports the findings reported by (CitationSSB, 2009).
Increasing milk quota is an indicator of the volume of production influencing farmers’ job satisfaction negatively. It reduces the satisfaction for AMS farmers, but not for CMS farmers. An explanation is that AMS farmers have, when investing in milking robot most often together with building a new cowshed, expanded their production significantly by buying a larger quota. Our study shows that AMS farmers increase their production by an average of 70 per cent by installing the AMS. The main driver of this expansion is the capacity of one milking robot (60–70 dairy cows), and the need to meet an increased financial burden. However, following this expansion there is also a need for fodder for the increased herd. Traditionally the main forage grass is produced on their own farms or rented land in the neighbourhood. Increasing forage production adds a considerable workload compared to the pre-AMS situation. In some regions there is also strong competition for farmland. All in all, this may result in a higher level of stress, i.e. a larger milk quota involves more stress for AMS farmers compared to CMS farmers.
From a gender perspective, we find that male farmers, both AMS farmers and CMS farmers, are less satisfied than females. This is in line with the findings among self-employed women in (CitationSSB, 2009). The gender issue in dairy farming is complex and relates to identity, roles, practice, off-farm work etc (CitationHaugen and Blekesaune, 2005; CitationCush and Macken-Walsh, 2018). Without further elaboration here, we recognize that the gender issue is more about dairy farming than about AMS or not. This may suggest that the introduction of AMS and degree of satisfaction may have fewer gender related aspects than other studies have indicated (CitationStræte et al., 2017).
For CMS farmers there are two variables which differ from AMS farmers; feelings of loneliness and health worries both reduces the job satisfaction. This is not surprising, but the difference from AMS needs explanation. We propose that the feeling of loneliness is related to the type of farmer, and not the AMS technology as such. Farmers with AMS are more frequently in contact with advisors and other farmers, while CMS farmers to some degree can run their dairy farm on their own. Several off-farm activities are offered to AMS farmers, both from robot suppliers and the advisory services. Among these are Lely-clubs and DeLaval-clubs, seminars, study visits, courses and so on. Further, milking robots still have a high profile in the media, the local press or TV. Thus, AMS farmers simply get more attention than CMS farmers. However, this issue also relates to household and personality, which is not elaborated on here.
The negative influence of health worries is most likely directly related to the technology. CMS requires a heavier physical load on arms, back and knees, and health problems may be more of an issue with CMS than with AMS, as indicated in a study based on interviews (CitationStræte et al., 2017).
4.3 Variables work together for job satisfaction
Taken together, based on the discussion we identified four different groups of variables and explanations on whether and how job satisfaction is influenced by milking system ().
Table 4 Factors which increase or decrease job satisfaction for AMS farmers and CMS farmers.
The variables increasing income, new cowshed, having a successor, and a clear intention to continue farming all increase job satisfaction. Further, there are no differences between AMS and CMS. These variables are all important to induce an optimistic and positive perspective on the future of being a dairy farmer. The second group includes variables that affect AMS farmers more than CMS farmers. There are various explanations as discussed above. The third group is related to the result that AMS farmers increase their production (increasing quota) when investing in AMS, and this increase has a negative effect on satisfaction. The fourth group of variables is related to a negative effect on satisfaction for CMS farmers. These various influences on satisfaction show that there is a need to be nuanced when considering how new technology influences job satisfaction. The facet-theory on job satisfaction is relevant for the complex situation for dairy farmers.
Our results show there are differences between AMS farmers’ and CMS farmers’ self-appraisal of their job satisfaction. However, the differences are not related to the technology and milking system alone. Moving from facet-theory towards a holistic and aggregated perspective and taking the context of the dairy farmer into account, we argue that a new cowshed, with AMS or CMS, is very important also for the other variables that may increase job satisfaction. A new cowshed involves better working conditions and opens for new technological solutions that all together stimulate job satisfaction. For older farmers a new cowshed may be related to if they have successors or not. If a son or daughter are ready to become the next farmer in near future, this may motivate for investment in a new cowshed, but not necessarily with AMS technology. We argue that the variables in group 1 are of higher importance for job satisfaction than AMS technology itself, as these variables are probably more basic than which milking technology is chosen.
With a new cowshed follow other changes that play out differently for AMS- and CMS-farmers, like increased production and reduced satisfaction for AMS-farmers (group 3). Our explanation for this is that the AMS technology has a higher capacity than CMS, approximately 70 cows per milking robot. To utilize the capacity, farmers have an incentive to increase the production by increasing the number of cows, which requires more land to produce feed and spread manure (CitationVik et al., 2019). According to our results, in the next step this increased production tends to reduce job satisfaction. Other studies also show that some AMS farmers experience too long hours after farm expansion (CitationHansen, 2015; CitationVik et al., 2019).
4.4 Implications and further research
A weakness of this study is that we focus only on job satisfaction related to the working day, occupational safety and working environment. The farmers’ relation to the animals also affects job satisfaction and represents an element in what is considered being a good farmer. As CitationBurton et al. (2020) put it: “the good cow embodies the good farmer’s identity and secures it over time”. From this follows aspects like sense of achievement and acknowledgment. Future studies could explore other aspects of satisfaction with being a farmer, such as e.g. relation to the animals, life outside farming activities, including family and household issues, and how AMS relates to these factors. Another weakness is that variables which affects adoption rates of AMS not included in this study might also play a role. Finally, relationships to advisors and colleagues, degree of farm specialization and location of the farm might also have an impact on the degree of job satisfaction.
Despite these potential shortcomings of this study, we point to the need for mixed methods when a complex topic like job satisfaction is studied. The topic is multifaceted. Quantitative studies are needed to find what factors or elements are important for whom at an aggregated level. However, qualitative studies are needed to explore how the elements relate to each other and how they cause a certain development. As indicated in the abovementioned weaknesses, we propose both quantitative studies to include more elements and qualitive to improve the explanations on why some variables influence job satisfaction more than others.
5 Conclusion
In this paper we have examined whether and how new technology like AMS influences job satisfaction for farmers. Our research questions are answered as follows: Firstly, are there differences in experienced level of job satisfaction between AMS farmers and CMS farmers? Our answer is yes, AMS farmers are more satisfied with their working day, their occupational safety, and their working environment. Secondly, which factors determine the level of job satisfaction in dairy farming? We have identified the following factors that increase job satisfaction: Increased income, new cowshed, successor, continue farming. The following factors reduce job satisfaction: higher education, being a male. Thirdly, do these factors vary on AMS farms compared to CMS farms? Our results show that the following factors differ: Less paper work, working together, less tech competence, being appreciated, less economic competence, increasing quota, loneliness and health worries. However, they can to a various degree be explained from an AMS/CMS perspective. According to our findings, the most important factors which influence job satisfaction for dairy farmers are common for AMS and CMS; increased income, new cowshed, successor and continue farming. These factors also contribute positively to job satisfaction. Our explanation is that renewing the cowshed opens opportunities to invest, e.g. in AMS or CMS, and subsequently these choices may imply that other variables get more important for the farmers consideration of their job satisfaction.
Declaration of interest
None.
Conflict of interest statement
The authors declare that we have no conflict of interest.
Funding
This study is part of the Norwegian R&D project “New approaches for management and breeding of dairy cows, in automatic milking systems (AMS)” coordinated by the Norwegian University of Life Sciences and funded by Norwegian Research Funding for Agriculture and Food Industry [352030 / 1202027322], and the dairy cooperative Tine. The funders did not influence on the design and execution of the research or influence the analysis and conclusions drawn, and views expressed in this article are those of the authors only.
References
- A.AgrestiAn Introduction to Categorical Data Analysis2nd edition2007Wiley-InterscienceHoboken
- R.AlmåsNorwegian agricultural historyFrom Farmer-society to Bio-industry 42002Det Norske SamlagetOslo
- T.BesserS.MannWhich farm characteristics influence work satisfaction? An analysis of two agricultural systemsAgric. Syst.1412015107112doi: https://doi.org/10.1016/j.agsy.2015.10.003 (accessed November 5, 2018).
- R.BijlH.HogeveenS.R.KooistraThe profitability of automatic milking on Dutch dairy farmsJ. Dairy Sci.902007239248
- H.BjørkhaugA.BlekesauneGender and work in Norwegian family farm businessesSociol. Ruralis482008152165
- B.BrewK.InderJ.AllenM.ThomasB.KellyThe health and wellbeing of Australian farmers: a longitudinal cohort studyBMC Public Health16201698810.1186/s12889-016-3664-y
- R.BurtonJ.ForneyP.StockL.A.SutherlandThe Good Farmer: Culture and Identity in Food and Agriculture. ISBN 9781138727960. Routledge2020
- D.ButlerL.HollowayC.BearThe impact of technological change in dairy farming: robotic milking systems and the changing role of the stockpersonJournal of the Royal Agricultural Society of England1732012
- T.D.CookD.T.CampbellQuasi-experimentation: Design & Analysis Issues for Field Settings1979Houghton Mifflin CompanyBoston
- CRANThe Comprehensive R Archive Networkhttps://cran.r-project.org/ (accessed 29 October 2018)2018
- P.CushA.Macken-WalshReconstituting male identities through joint farming ventures in IrelandSociol. Ruralis582018726744
- K.de KoningAutomatic Milking – Common Practice on Dairy Farms. The First North American Conference on Precision Dairy Management. June 2 2017Available at: http://www.precisiondairy.com/proceedings/s3dekoning.pdf (accessed October 10, 2018)2010
- E.DeFrancescoP.GattoD.MozzatoTo leave or not to leave? Understanding determinants of farmer’s choices to remain in or abandon agri-environmental schemesLand Use Policy762018460470
- D.R.EvansJ.R.PellizarriB.J.CulbertPersonality, marital, and occupationalfactors associated with quality of lifeJ. Clin. Psychol.491993477485
- E.FjellhammerThe Development of Milk Production up Until 2017. Note No 32013Agri AnalyseOslo
- L.FragarA.J.HendersonC.MortonK.PollockFacts and Figures on Farm Health and Safety Series #12. Australia: Australian Centre for Agricultural Health and Safety. The Mental Health of People on Australian Farms- the Facts2008
- A.M.FuglsethÖSöreböThe effects of technostress within the context of employee use of ICTComput. Human Behav.402014161170
- F.J.GravetterL.B.WallnauStatistics for the Behavioral Sciences9th edition2014Cengage LearningWadsworth
- A.GregoireThe mental health of farmersOccup. Med. (Chic Ill)52200247147610.1093/occmed/52.8.471
- B.G.HansenRobotic milking - farmer experiences and adoption rate in JærenNorway. Journal of Rural Studies412015109117
- B.G.HansenA.GreveThe role of human and social capital in dairy farmingRural. Soc.242015154176
- B.G.HansenA.M.JervellChange management in dairy farmingInternational Journal of Sociology of Agriculture and Food2220142340
- R.B.HårstadThe Farmer, the Family and the Milking Robot- a New Everyday Life With Techno-driven Livestock. Unpublished Report. Ruralis- Institute for Rural End Regional Research, Trondheim2019
- M.S.HaugenA.BlekesauneFarm and Off-farm Work and Life Satisfaction Among Norwegian Farm Women452005718510.1111/j.1467-9523.2005.00291.x
- J.A.JacobsJ.M.SiegfordInvited review: the impact of automatic milking systems on dairy cow management, behavior, health, and welfareJ. Dairy Sci.95201222272247
- S.M.JexOrganizational Psychology: a Scientist-practitioner Approach2002John Wiley & Sons, IncNew York, NY
- K.G.JöreskogU.H.OlssonF.WallentinMultivariate Analysis With Lisrel2016SpringerNew York, NY
- G.KaufmannA.KaufmannPsychology in Organization and Leadership2009FagbokforlagetBergen
- E.A.LockeThe nature and causes of job satisfactionM.D.DunnetteHandbook of Industrial and Organizational Psychology1976Rand McNallyChicago, IL12971350
- K.V.MardiaMeasures of multivariate skewness and kurtosis with applicationsBiometrika571970519530file:///C:/Users/hanbjo01/Downloads/R2014%20KU%20-%20Disponibel%20kvote%202017,%20strukturfordeling.pdf (accessed April 30, 2019).
- P.MiloneF.VenturaNew generation farmers: rediscovering the peasantryJ. Rural Stud.6520184352doi: https://doi.org/10.1016/j.jrurstud.2018.12.009 (accessed January 20, 2019).
- D.MîndrilăMaximum likelihood (ML) and diagonally weighted least squares (DWLS) estimation procedures: a comparison of estimation Bias with ordinal and multivariate non-normal dataInt. J. Digit. Soc.120106066
- Y.MomoseT.SuenagaH.UneJob satisfaction and mental distress among Japanese farmersJ. Rural. Med.320082933
- Norwegian Agriculture AgencyQuotas 2017, Structural Distribution2019
- Norwegian Health AuthorityGood Life in Norway. Measuring the Populations’ Quality of LifeAvailable at: https://helsedirektoratet.no/publikasjoner/gode-liv-i-norge-utredning-om-maling-av-befolkningens-livskvalitet (accessed December 15, 2018)2016
- D.PeelH.L.BerryJ.SchirmerFarm exit intention and wellbeing: a study of Australian farmersJ. Rural Stud.472016415110.1016/j.jrurstud.2016.07.006(accessed November 24, 2018)
- D.PhillipsQuality of Life. Concept, Policy and Practive2006RoutledgeLondon
- J.L.PriceReflections on the determinants of voluntary turnoverInt. J. Manpow.222001600624
- J.J.RandolphK.FalbeA.Kureethara ManuelJ.L.BallounA Step-by Step Guide to Propensity Score Matching in R. Practical Assessment, Research & Evaluation 19, No 182014
- J.RodenburgRobotic milking: technology, farm design, and effects on work flowJ. Dairy Sci.10020177729773810.3168/jds.2016-11715(accessed November 24, 2018)
- G.SalancikJ.PfefferA social information processing approach to job attitudes and task designAdm. Sci. Q.231978224253
- P.E.SpectorJob Satisfaction: Application, Assessment, Causes, and Consequences1997SAGE PublicationsLondon
- SSBStatistics Norway. Probability of Satisfaction in Current JobAvailable at: https://www.ssb.no/a/samfunnsspeilet/utg/200901/06/tab-2009-03-09-02.html(accessed November 24, 2018)2009
- M.A.SteinhardtC.L.DolbierN.H.GottliebThe Relationship between hardiness, supervisor support, group cohesion, and job stress as predictors of job satisfactionAm. J. Health Promot.172003382389
- P.V.StockJ.ForneyFarmer autonomy and the farming selfJ. Rural Stud.362014160171doi: https://doi.org/10.1016/j.jrurstud.2014.07.004 (accessed April 20, 2018)
- E.P.StræteJ.VikB.G.HansenThe Social Milking Robot: a Study of the Social Aspects of Milking Robots in Dairy Farming. Paper at the 11th “International European Forum on System Dynamics and Innovation in Food Networks,” Innsbruck. 13-17 FebruaryPages 220–233 in Proceedings: http://centmapress.ilb.uni-bonn.de/ojs/index.php/proceedings/index (accessed April 20, 2018)2017
- A.ThelinS.HolmbergFarmers and retirement: a longitudinal cohort studyJ. Agromedicine152010384610.1080/10599240903389623
- L.L.ThurstoneMultiple Factor Analysis: a Development and Expansion of Vectors of the Mind1947University of Chicago PressChicago
- C.TseH.W.BarkemaT.J.de VriesJ.RushenE.A.PajorEffect of transitioning to automatic milking systems on producers’ perceptions of farm management and cow health in the Canadian dairy industryJ. Dairy Sci.1002017111
- J.VikE.StræteB.G.HansenT.NærlandThe political robot – the structural consequences of automated milking systems (AMS) in Norway NJAS - WageningenJ. Life Sci.201910.1016/j.njas.2019.100305
- K.M.WadeM.A.P.M.van AsseldonkP.B.M.BerentsenW.OuweltjesH.HogeveenEconomic efficiency of automatic milking systems with specific emphasis on increases in milk productionA.MeijeringH.HogeveenC.J.A.M.de KoningAutomatic Milking—A Better Understanding. Wageningen2004Academic PublishersWageningen, the Netherlands
- K.B.WoodfordM.H.BrakenrigM.C.PangbornNew Zealand Case Studies of Automatic-milking-systems Adoption. in: Proc. N. Z. Soc. Anim. Prod., Dunedin, New Zealand. New Zealand Society of Animal Production Inc., Dunedin, New Zealand2015
- A.Zahl-ThanemE.M.FuglestadJ.VikTrends in Norwegian Agriculture 2018: a Changing Agriculture. Report Nr 7. Trondheim: Ruralis2018
- J.E.ZejdaH.H.McDuffieJ.A.DosmanEpidemiology of health and safety risks in agriculture and related industriesWest. J. Med.15819935663
Appendix A
Differences between AMS and CMS farmers