Abstract
Background
Large Language Models (LLMs) might offer a solution for the lack of trained health personnel, particularly in low- and middle-income countries. However, their strengths and weaknesses remain unclear.
Aims/objectives
Here we benchmark different LLMs (Bard 2023.07.13, Claude 2, ChatGPT 4) against six consultants in otorhinolaryngology (ORL).
Material and methods
Case-based questions were extracted from literature and German state examinations. Answers from Bard 2023.07.13, Claude 2, ChatGPT 4, and six ORL consultants were rated blindly on a 6-point Likert-scale for medical adequacy, comprehensibility, coherence, and conciseness. Given answers were compared to validated answers and evaluated for hazards. A modified Turing test was performed and character counts were compared.
Results
LLMs answers ranked inferior to consultants in all categories. Yet, the difference between consultants and LLMs was marginal, with the clearest disparity in conciseness and the smallest in comprehensibility. Among LLMs Claude 2 was rated best in medical adequacy and conciseness. Consultants’ answers matched the validated solution in 93% (228/246), ChatGPT 4 in 85% (35/41), Claude 2 in 78% (32/41), and Bard 2023.07.13 in 59% (24/41). Answers were rated as potentially hazardous in 10% (24/246) for ChatGPT 4, 14% (34/246) for Claude 2, 19% (46/264) for Bard 2023.07.13, and 6% (71/1230) for consultants.
Conclusions and significance
Despite consultants superior performance, LLMs show potential for clinical application in ORL. Future studies should assess their performance on larger scale.
Chinese Abstract
背景
大型语言模式(LLM)可能为训练有素的健康工作者的缺乏提供解决方案, 特别是在低收入和中等收入国家。 然而, 这种模式的优点和缺点仍不清楚。
目的
在这里, 我们将不同的大型语言模式(Bard2023.07.13、Claude2、ChatGPT4)与六位耳鼻喉科 (ORL) 顾问医生比较而进行基准化。
材料和方法
基于案例的问题摘自文献和德国政府考试。 采用 6 点李克特量表, 对Bard2023.07.13、Claude2、ChatGPT4、六位ORL顾问医生的回答随机评级, 来衡量医学充分性、可理解性、连贯性和简洁性。将给出的答案与经过验证的答案进行比较并评估风险。 进行了修改后的图灵测试并比较字符计数。
结果
在所有类别中, 大型语言模式的答案的等级均低于顾问医生们的答案。 顾问医生们和大型语言模式之间的区别很小, 在简洁性方面差异最明显, 在可理解性方面差异最小。在大型语言模式中, Claude2 在医疗充分性和简洁性方面被评为最佳。 顾问医生们的答案与经过验证的解决方案的匹配率为 93% (228/246), ChatGPT4 的匹配率为 85% (35/41), Claude2 的匹配率为 78% (32/41), Bard2023.07.13 的匹配率为59% (24/41)。 ChatGPT4 的答案被评为有风险性的为 10% (24/246), Claude2 为14% (34/246), Bard2023.07.13 为 19% (46/264), 顾问医生们为 6% (71/1230)。
结论和意义
尽管顾问医生们表现出色, 大型语言模式仍显示出耳鼻喉科临床应用的潜力, 未来的研究应该更大规模地评估它们的表现。
Introduction
The impact of Artificial Intelligence (AI) and Large Language Models (LLMs) on society is anticipated to be as groundbreaking as the industrial revolution [Citation1]. While the exact impact of AI is yet to become evident, the increasing influence of AI on many aspects of human society, in particular the delivery of healthcare, is already without question. Delivery of health care is currently facing huge challenges due to economic pressure and demographic change with widespread lack of access to adequate medical care in industrial as well as low- and middle-income countries commonplace [Citation2–4]. LLMs may have the potential to overcome some of the challenges of healthcare delivery especially in the areas of diagnosis, management, and referral [Citation5].
For a long time, Natural Language Processing (NLP) tasks like question answering, reading comprehension, and summarization were typically performed by sequential models processing tasks in a word-by-word approach. However, sequential computation is limited in parallelization and inaccurate for large input data due to a lack of prioritization. In 2017, Vaswani et al. introduced the transformer model, providing a solution to these architecture-dependent deficits [Citation6,Citation7]. Unlike sequential models, the transformer model provides a self-attention mechanism tracing global dependencies between words, enabling significantly more parallelization and thus accurate processing for large data input [Citation7]. Various well-known Natural Language Processing (NLP) tools including ChatGPT 4, Bard 2023.07.13, and Claude 2 rely on the transformer model.
Taking these developments into consideration and given the ubiquitous and low barrier access, patients and caregivers alike are likely to consult LLMs on medical queries, especially in scenarios with limited access to medical care. A recent study by our working group showed that the LLMs ChatGPT 3.5 and ChatGPT 4 were inferior to specialists in the specific medical field of Otorhinolaryngology (ORL) [Citation8]. Nevertheless, the performance of universal LLMs like ChatGPT 4 is respectable and promising, especially when considering the early stage of their development and that these universal models like ChatGPT 4 are not specially trained for medical purposes. Considering the current speed of development and improvement of universal LLMs, subsequent individual ‘specialization’ for certain fields or tasks as well as the economical commitment, the applicability of LLMs for health care services will certainly increase. Taking this into account, a specific evaluation of current LLMs is of high importance. ORL is a highly specialized field of medical care. However, typical pathologies in the field comprise a broad variety of diseases ranging from relatively ‘harmless’ conditions to life-threatening disease. Symptoms associated with severe disease and harmless conditions may often overlap highlighting the importance of a proper initial assessment. Moreover, owing to the high burden of disease, otolaryngology cases are the common focus of symptom-based internet searches by patients.
To assess the potential and limitations of LLM performance in the field of ORL [Citation8–15], we benchmarked the medical performance of different LLMs including ChatGPT 4, Bard 2023.07.13, and Claude 2 against six experienced consultants working in both clinical and outpatient care by evaluating both the semantic qualities as well as the medical content of responses to case-based questions.
Materials and methods
One thousand four hundred case-based questions were retrieved from the ORL literature and German state examination exams for doctors. Cases that did not correlate to equivalent realistic clinical scenarios in the University Medical Center of Mainz were excluded from our study. The questions covered the categories ear (n = 14), nose (n = 8), head and neck (n = 13) and tumor (n = 6). After assessment of all questions, 41 common and realistic ORL case-based clinical questions (same as used in our previous study) were posed to the LLMs ChatGPT 4 (Open AI, San Francisco, USA), Bard 2023.07.13, now known as Gemini (Google, Mountain View, USA) and Claude 2 (Anthropic, San Francisco, USA) in October 2023 [Citation8]. For each LLM the base model was used without any tuning, resembling the most likely scenario of patients investigating their own symptoms. The same questions were answered by six ORL consultants working in University Medical Centers and two consultants based in an outpatient practice. The consultants had at least 7.5 years of clinical experience in ORL.
The answers were then blindly rated by the consultants in the categories of coherence, comprehensibility, conciseness, and medical quality on a 6-point Likert-scale (1 = very poor and 6 = excellent). As a modified Turing Test consultants also recorded whether they felt the answer was generated by a human or a LLM [Citation16]. To evaluate possible hazards consultants also assessed each answer for potential jeopardy to patient well-being. Since rating poses possible bias, all answers given were also compared to the validated answers provided in the study books [Citation17,Citation18]. Finally, the character count for each answer was recorded.
For all queried data, normality distribution was tested with the D’Agostino and Pearson test. Since the data did not show a Gaussian distribution, comparisons between the two groups were conducted using the Mann-Whitney U test and multi-group comparisons with the Kruskal-Wallis test, respectively.
To evaluate correlations of the evaluated parameters to the character count, the nonparametric Spearman correlation test was performed. Data was collected in Microsoft Excel sheets (Microsoft, Redmond, WA, USA) and all statistical testing was conducted using Prism for Windows (version 9.5.1; GraphPad Software, La Jolla, CA, USA).
Results
Ratings for all answers for the categories of medical adequacy, comprehensibility, coherence, and conciseness are shown in . Ratings for the consultants were superior to the LLMs in all categories. The difference between the consultants and the LLMs was however small. On medical adequacy, the consultant’s answers were rated best (median 5, IQR 5–6), followed by Claude 2 (median 5, IQR 4–6), ChatGPT 4 (median 5, IQR 4–6), and lastly Bard 2023.07.13 (median 5, IQR 3–5). The rating for comprehensibility showed a small difference between the consultant’s (median 6, IQR 5–6) and the LLM answers. Again, Claude 2 (median 5, IQR 5–6) performed best among the LLMs, with ChatGPT 4 (median 5, IQR 5–6) closely behind and finally Bard 2023.07.13 (median 5, IQR 5–6). For coherence, ChatGPT 4 (median 5, IQR 5–5) was rated best among the LLMs, closely followed by Claude 2 (median 5, IQR 4–5) and Bard 2023.07.13 (median 5, IQR 4–5). The rating in the conciseness category showed the clearest disparity between ORL consultant (median 6, IQR 5–6) and LLM answers. Here, Claude 2 (median 4, IQR 4–5) performed best, and Bard 2023.07.13 (median 4, IQR 3–4) worst. Overall, Bard 2023.07.13 performed worst in all categories (), whereas ChatGPT 4 and Claude 2 achieved similar scores in all categories.
Figure 1. Comparison between ORL consultants and the different LLMs (ChatGPT4, Bard 2023.07.13, Claude 2) for all evaluated categories. Data shown as a scatter dot blot with points representing absolute values and bar width representing amount of individual values. Horizontal lines represent mean (95% CI). Normality distribution was tested with the D’Agostino and Pearson test. Multi-group comparisons were performed using the Kruskal-Wallis-Test. ns > .05, *p < .05, **p < .01, ***p < .001, ****p < .0001.
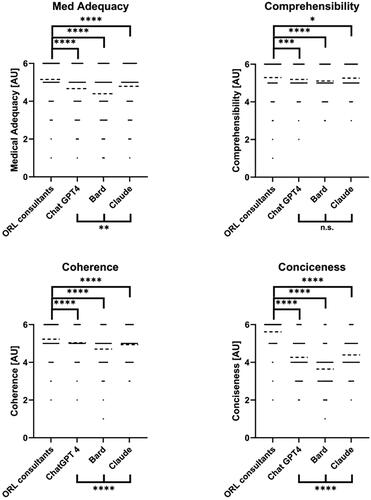
Only 1 of 246 of Bard 2023.07.13 ‘s answers passed the modified Turing (1/246 = 0.4%) with ChatGPT 4 in 3.7% (9/246 = 3.7%) and Claude 2 in 8.1% (20/246 = 8.1%) fairing slightly better.
Consultants’ answers included the validated answer in 92.7% (228/246 = 92.7%) of cases, ranging from 100% (41/41 = 100%) to 85.4% (35/41 = 85.4%) between the 6 different consultants. ChatGPT 4 performed best among the LLMs, with 85.4% of answers containing the validated solution (35/41 = 85.4%), followed by Claude 2 with 78.1% (32/41 = 78.1%) and Bard 2023.07.13 with 58.5% (24/41 = 58.5%).
Answers were rated as hazardous for the patient in 9.8% of cases for ChatGPT 4 (24/246 = 9.8%), 13.8% for Claude 2 (34/246 = 13.8%), 18.7% for Bard 2023.07.13 (46/264 = 18.7%), and 5.8% for the consultants (71/1230 = 5.8%).
A comparison of the character count is shown in . Bard 2023.07.13 generated the longest answers with an average of 1911 characters (Range: 851–3709), followed by ChatGPT 1264 (Range: 475–2289), Claude 2 1078 (Range: 488–1529) and the consultants with statistically the least number of characters per answer (129–Range: 4–831).
Figure 2. The number of characters per answer used by ORL consultants and the different LLMs (ChatGPT 4, Bard 2023.07.13, Claude 2). Data shown as a scatter dot blot with each point resembling an absolute value. Horizontal lines represent the median. Normality distribution was tested with the D’Agostino and Pearson test. Multi-group comparisons were performed using the Kruskal-Wallis-Test. ****p < .001.
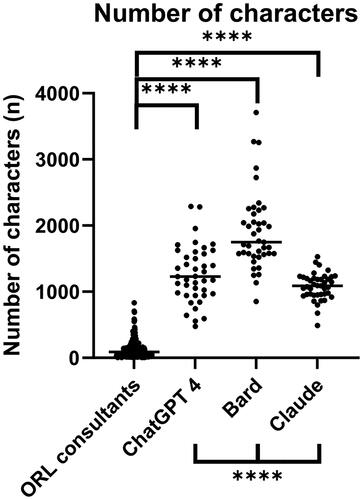
Correlations between the number of characters used and the specific qualities evaluated are described in . For ratings for Medical Adequacy, Comprehensibility, and Coherence a strong positive correlation to the number of characters used was determined, while the Conciseness showed a mild negative correlation for answers by the ORL consultants. In contrast, for answers by ChatGPT 4, a negative correlation between the number of characters and Conciseness was identified, while answers by Bard 2023.07.13 with a higher average character count correlated positively with Medical Adequacy and Coherence.
Table 1. Correlation Analysis for ratings for Medical Adequacy, Comprehensibility, and Coherence, Conciseness and the number of characters used. (ns >< .05, *p < .01, **p < .01, ****p < .001, ;****p < .0001).
Discussion
Previous studies have evaluated the potential and limitations of LLMs in the field of ORL [Citation8–15,Citation19–28]. Most of these studies however focused on the most cited LLM ChatGPT. Yet, the field of LLMs contains several widely used entities, each with differing architecture, training data, training process, generative performance, and interaction style. A study comparing the performance of different LLMs benchmarking their output against experienced consultants is therefore timely.
Except for comprehensibility, where all LLMs were rated comparably, statistically significant differences between the three tested LLMs were found. Of all tested LLMs Claude 2 was rated best in the categories of medical adequacy and conciseness and was only slightly surpassed by ChatGPT 4 for coherence. Bard 2023.07.13 on the other hand got the lowest ratings in every category. These results do in no way reflect the overall capabilities but solely the ratings in our specific field of analysis [Citation29].
In concordance with our previously published data, the consultants outperformed the LLMs in every rated category (Medical Adequacy, Comprehensibility, Coherence, and Conciseness) [Citation8]. While the ratings strongly suggest the superiority of ORL specialists over the LLMs in answering case based clinical questions, the high overall quality of answers must also be considered. The comprehensibility of answers received the highest overall ratings for all LLMs. In this category, differences between LLMs and ORL consultants, while still statistically significant, were least pronounced. Taking these findings into account and considering the high ratings for coherence, our results underline the very high quality of semantic output now being generated by LLMs. In contrast, the overall ratings for medical adequacy, arguably the most important qualitative asset evaluated, show a more obvious discrepancy between the ORL specialists and the LLMs. Noticeably, the ratings for all LLMs are still impressively high with Claude 2 providing the best and Bard 2023.07.13 the least medically adequate answers.
Intriguingly, ratings for the conciseness of answers showed the biggest discrepancies between the ORL consultants and the LLMs, respectively. This aspect is especially interesting in relation to the character count. The LLMs utilized significantly more characters on each answer generated compared to the consultants, with Bard 2023.07.13 being the most verbose whilst achieving the lowest ratings in all evaluated categories. In contrast, Claude 2 made use of significantly less characters whilst getting the highest ratings for conciseness and medical adequacy. In the analysis with the Spearman rank test, a negative correlation for the number of characters was only detected in relation to the ratings for conciseness for ChatGPT 4 and coherence and medical adequacy for answers provided by Bard 2023.07.13, respectively.
Although Claude 2 received the highest rating for medical adequacy among the LLMs, ChatGPT 4 performed best in covering the validated answers. While the consultant answers were consistent with the validated solution in 92.68% of cases, ChatGPT 4 achieved 85.37%, Claude 2 78.05%, and Bard 2023.07.13 in 58.54%, respectively. We found ChatGPT 4 to perform significantly better compared to other studies, such as Hoch et al. who reported 57% correct answers from ChatGPT 4 on ORL board certification preparation questions, and Chee et al. who found 75% correct answers on vertigo scenarios, compared to 85.37% in our study [Citation10,Citation11].
Interestingly, all LLMs performed poorly interpreting the Weber test which can be considered a relatively simple ‘transfer task’. On the other hand, all consultants answered the two questions dealing with Weber test results correctly (12/12 = 100%). ChatGPT 4 was the only LLM that generated a correct answer for one of the two questions regarding the Weber test (1/6 = 16.67%). This example illustrates LLM’s potential to generate human-like responses but without the ability to ‘think’ like an experienced human counterpart. Possible explanations for the poor performance in the Weber test may originate in a lack of sufficient training data may result in ‘hallucination’. Alternatively, deficits of LLMs in the detection of context may be attributable. Ultimately, due to the closed architecture of the LLMs and training data, the LLMs decision making is a black box and a definite explanation for the wrong responses cannot be made.
In this regard, the capacity to prioritize certain symptoms in relation to the prevalence and likelihood of certain diseases is what currently sets the ORL consultants apart. While the ORL specialists usually provided the most likely diagnosis and added a focused and relevant differential, LLMs provided a much broader, less focused differential diagnosis mostly without any prioritization relevant to the clinical case [Citation15]. While this limitation can be addressed by using prompts asking for ranking and structured answers [Citation13], it is unlikely that patients would use this approach. However, for professional use in clinical practice prompts should be considered to generate more precise output. In the future, LLM services may provide specific options for medical consultancy or accessible user training on specific ORL topics. In time, more specialized training and narrowing down to a thematic field could result in more accurate and concise responses.
Case-based questions and Likert rating systems have limitations. On the one hand, case-based questions are advantageous due to their objective validated format and related answers and a broad range of ORL cases. Their validated wording reduces the risk of miscommunication but does not emulate the more likely questions posed by real patients. Further studies should therefore also evaluate questions originating from patients to feature in these factors. Moreover, a six point Likert scale, while suited for this type of study, has statistical limitations that must be taken into consideration when interpreting the results. While rating always poses possible bias, matching the answers given to validated answers can be considered objective. Since findings showed similar results to the ratings, the rating system seems valid although personal preferences may be featured in.
Accepting these limitations, this study still provides new important evidence for the diagnostic capability of this technology. While consultants are still superior to LLMs the gap between consultants and the LLMs is small. In a world suffering from a shortage of medical specialists and medical caregiving these results are promising especially in low resource settings, where internet access is often available but qualified medical personnel scarce. At present, some hazards to patients are still present in the responses from LLM based chatbots so they should still not be a substitute for a consultation with a trained professional. In a real-life consultation, much more can be achieved, such as non-verbal communication, physical examination, laboratory results, and imaging. These are crucial aspects of a consultation that LLMs simply cannot provide at present. Nevertheless, in the future, the combination of text and image analysis may enable LLMs to overcome some of these limitations. Although the upsides are obvious, critical aspects like the safety of personal data have to be carefully addressed [Citation30]. Moreover, reliability (reproducibility) is also an important factor in the comparative evaluation of LLM queries and consultants alike [Citation31]. Future studies should evaluate LLMs capabilities with real cases featuring aspects like miscommunication, machine-patient interaction, and reproducibility.
Supplemental Material
Download MS Word (26.6 KB)Disclosure statement
SK is the founder and shareholder of MED.digital.
References
- Cabrera J, Loyola MS, Magaña I, et al. Ethical dilemmas, mental health, artificial intelligence, and LLM-based chatbots. Bioinformatics and Biomedical Engineering. Lecture Notes in Computer Science; 2023. p. 313–326.
- Nicholas PK, Smith MF. Demographic challenges and health in Germany. Popul Res Policy Rev. 2007;25(5–6):479–487. doi: 10.1007/s11113-006-9009-2.
- Van Bokkelen G, Morsy M, Kobayashi T. Demographic transition, health care challenges, and the impact of emerging international regulatory trends with relevance to regenerative medicine. Curr Stem Cell Rep. 2015;1(2):102–109. doi: 10.1007/s40778-015-0013-5.
- Dawkins B, Renwick C, Ensor T, et al. What factors affect patients’ ability to access healthcare? An overview of systematic reviews. Trop Med Int Health. 2021;26(10):1177–1188. doi: 10.1111/tmi.13651.
- Tu T, Palepu A, Schaekermann M, et al. Towards conversational diagnostic AI. arXiv Preprint. 2024:arXiv:240105654.
- Radford A, Wu J, Child R, et al. editors. Language models are unsupervised multitask learners; Technical report, OpenAi, 2019.
- Vaswani A, Shazeer N, Parmar N, et al. Attention is all you need. In: Advances in neural information processing systems. Vol. 30; 2017. Computation and Language (cs.CL); Machine Learning (cs.LG)
- Buhr CR, Smith H, Huppertz T, et al. ChatGPT versus consultants: blinded evaluation on answering otorhinolaryngology case–based questions. JMIR Med Educ. 2023;9:e49183. doi: 10.2196/49183.
- Dallari V, Sacchetto A, Saetti R, et al. Is artificial intelligence ready to replace specialist doctors entirely? ENT specialists vs ChatGPT: 1-0, ball at the center. Eur Arch Otorhinolaryngol. 2023;281(2):995–1023. doi: 10.1007/s00405-023-08321-1.
- Chee J, Kwa ED, Goh X. “Vertigo, likely peripheral”: the dizzying rise of ChatGPT. Eur Arch Otorhinolaryngol. 2023;280(10):4687–4689. doi: 10.1007/s00405-023-08135-1.
- Hoch CC, Wollenberg B, Lüers J-C, et al. ChatGPT’s quiz skills in different otolaryngology subspecialties: an analysis of 2576 single-choice and multiple-choice board certification preparation questions. Eur Arch Otorhinolaryngol. 2023;280(9):4271–4278. doi: 10.1007/s00405-023-08051-4.
- Chiesa-Estomba CM, Lechien JR, Vaira LA, et al. Exploring the potential of Chat-GPT as a supportive tool for sialendoscopy clinical decision making and patient information support. Eur Arch Otorhinolaryngol. 2023;281(5):2777–2777. doi: 10.1007/s00405-023-08267-4.
- Qu RW, Qureshi U, Petersen G, et al. Diagnostic and management applications of ChatGPT in structured otolaryngology clinical scenarios. OTO Open. 2023;7(3):e67. doi: 10.1002/oto2.67.
- Nielsen JPS, von Buchwald C, Grønhøj C. Validity of the large language model ChatGPT (GPT4) as a patient information source in otolaryngology by a variety of doctors in a tertiary otorhinolaryngology department. Acta Otolaryngol. 2023;143(9):779–782. doi: 10.1080/00016489.2023.2254809.
- Ayoub NF, Lee YJ, Grimm D, et al. Head-to-head comparison of ChatGPT versus google search for medical knowledge acquisition. Otolaryngol Head Neck Surg. 2023;1–8. doi: 10.1002/ohn.465.
- Turing AM. I.—Computing machinery and intelligence. Mind. 1950;LIX(236):433–460. doi: 10.1093/mind/LIX.236.433.
- Reineke U, Riemann R. Facharztprüfung Hals-Nasen-Ohrenheilkunde: 1000 kommentierte prüfungsfragen. Stuttgart, Germany: Thieme; 2007.
- Thomas Lenarz H-GB. Hals-Nasen-Ohren-Heilkunde. Germany, Neu-Isenburg: Springer Medizin Verlag GmbH; 2012.
- Warrier A, Singh R, Haleem A, et al. The comparative diagnostic capability of large language models in otolaryngology. Laryngoscope. 2024;1–6. doi: 10.1002/lary.31434.
- Saibene AM, Allevi F, Calvo-Henriquez C, et al. Reliability of large language models in managing odontogenic sinusitis clinical scenarios: a preliminary multidisciplinary evaluation. Eur Arch Otorhinolaryngol. 2024;281(4):1835–1841. doi: 10.1007/s00405-023-08372-4.
- Pugliese G, Maccari A, Felisati E, et al. Are artificial intelligence large language models a reliable tool for difficult differential diagnosis? An a posteriori analysis of a peculiar case of necrotizing otitis externa. Clin Case Rep. 2023;11(9):e7933. doi: 10.1002/ccr3.7933.
- Zalzal HG, Abraham A, Cheng J, et al. Can ChatGPT help patients answer their otolaryngology questions? Laryngoscope Investig Otolaryngol. 2023;9(1):e1193. doi: 10.1002/lio2.1193.
- Zalzal HG, Cheng J, Shah RK. Evaluating the current ability of ChatGPT to assist in professional otolaryngology education. OTO Open. 2023;7(4):e94. doi: 10.1002/oto2.94.
- Liu J, Wang C, Liu S. Utility of ChatGPT in clinical practice. J Med Internet Res. 2023;25:e48568. doi: 10.2196/48568.
- Long C, Lowe K, Zhang J, et al. A novel evaluation model for assessing ChatGPT on otolaryngology–head and neck surgery certification examinations: performance study. JMIR Med Educ. 2024;10:e49970. doi: 10.2196/49970.
- Noda M, Ueno T, Koshu R, et al. Performance of GPT-4V in answering the Japanese otolaryngology board certification examination questions: evaluation study. JMIR Med Educ. 2024;10:e57054. doi: 10.2196/57054.
- Shen SA, Perez-Heydrich CA, Xie DX, et al. ChatGPT vs. web search for patient questions: what does ChatGPT do better? Eur Arch Otorhinolaryngol. 2024;281(6):3219–3225. doi: 10.1007/s00405-024-08524-0.
- Dhar S, Kothari D, Vasquez M, et al. The utility and accuracy of ChatGPT in providing post-operative instructions following tonsillectomy: a pilot study. Int J Pediatr Otorhinolaryngol. 2024;179:111901. doi: 10.1016/j.ijporl.2024.111901.
- Eriksen AV, Möller S, Ryg J. Use of GPT-4 to diagnose complex clinical cases. NEJM AI. 2023;1(1). doi: 10.1056/AIp2300031.
- Meskó B, Topol EJ. The imperative for regulatory oversight of large language models (or generative AI) in healthcare. NPJ Digit Med. 2023;6(1):120. doi: 10.1038/s41746-023-00873-0.
- Kuşcu O, Pamuk AE, Sütay Süslü N, et al. Is ChatGPT accurate and reliable in answering questions regarding head and neck cancer? Front Oncol. 2023;13:1256459. doi: 10.3389/fonc.2023.1256459.