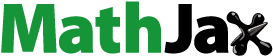
Abstract
Scientific journals may counter the misuse, misreporting, and misinterpretation of statistics by providing guidance to authors. We described the nature and prevalence of statistical guidance at 15 journals (top-ranked by Impact Factor) in each of 22 scientific disciplines across five high-level domains (N = 330 journals). The frequency of statistical guidance varied across domains (Health & Life Sciences: 122/165 journals, 74%; Multidisciplinary: 9/15 journals, 60%; Social Sciences: 8/30 journals, 27%; Physical Sciences: 21/90 journals, 23%; Formal Sciences: 0/30 journals, 0%). In one discipline (Clinical Medicine), statistical guidance was provided by all examined journals and in two disciplines (Mathematics and Computer Science) no examined journals provided statistical guidance. Of the 160 journals providing statistical guidance, 93 had a dedicated statistics section in their author instructions. The most frequently mentioned topics were confidence intervals (90 journals) and p-values (88 journals). For six “hotly debated” topics (statistical significance, p-values, Bayesian statistics, effect sizes, confidence intervals, and sample size planning/justification) journals typically offered implicit or explicit endorsement and rarely provided opposition. The heterogeneity of statistical guidance provided by top-ranked journals within and between disciplines highlights a need for further research and debate about the role journals can play in improving statistical practice.
1 Introduction
The validity of scientific claims often depends on the appropriate selection, implementation, reporting, and interpretation of statistical analyses. However, serious concerns have been raised about the frequent misuse, misreporting, and misinterpretation of statistical methods in several scientific disciplines (Altman Citation1982; Gigerenzer Citation2018; Nieuwenhuis, Forstmann, and Wagenmakers Citation2011; Sedlmeier and Gigerenzer Citation1989; Strasak et al. Citation2007; Wasserstein and Lazar Citation2016). Academic journals may attempt to counter these problems by providing guidance on statistical issues in their instructions to authors (Altman et al. Citation1983; Bailar and Mosteller Citation1988; Smith Citation2005).
It is unclear whether journal-based statistical guidance, advocacy, or regulation is helpful or detrimental, especially in the context of controversial statistical topics (Mayo Citation2021). There continues to be heated debate among statisticians and methodologists about fundamental statistical issues, as exemplified by the diverse commentaries in a 2019 special issue of The American Statistician (Wasserstein, Schirm, and Lazar Citation2019). p-values continue to be vigorously attacked (Wagenmakers Citation2007) and staunchly defended (Mayo Citation2018), and recent years have seen competing calls to either abandon (McShane et al. Citation2019), redefine (Benjamin et al. Citation2018), or justify (Lakens et al. Citation2018) statistical significance. Others have advocated for a so-called “New Statistics” centered on effect size estimation and confidence intervals (Cumming Citation2014) or promoted wider adoption of Bayesian statistics (Goodman Citation1999; Kruschke and Liddell Citation2018).
In some cases, these debates have influenced journal policy and controversial changes in policy have sometimes backfired. For example, in 2005, in an attempt to avoid the “pitfalls of traditional statistical inference,” the journal Psychological Science endorsed a novel statistic (Killeen Citation2005) which was subsequently adopted by the majority of publishing authors, and then abandoned under heavy criticism a few years later (Iverson et al. Citation2009). In 2015, the journal Basic and Applied Social Psychology introduced an outright ban on p-values and confidence intervals “because the state of the art remains uncertain” (Trafimow and Marks Citation2015). An assessment of articles published in the first year after the ban argued that the absence of statistical safeguards led many authors to overstate their findings (Fricker et al. Citation2019). Less controversial statistical guidance may help to reduce basic statistical errors; however, beyond case studies, we are not aware of any rigorous trials assessing the impact of journal-based statistical guidance on author behavior. Some evidence suggests that more general methodological reporting guidelines, such as Consolidated Standards of Reporting Trials (CONSORT), can be modestly effective (Turner et al. Citation2012). Understanding the current landscape of journal-based statistical guidance will help to inform the design of future trials that assess journal policies intended to improve statistical practice.
Currently there is limited empirical data documenting the prevalence and content of statistical guidance for authors issued by scientific journals. Such data will help to guide debates about the appropriate role of journal policy in addressing statistical issues (Mayo Citation2021) as well as identify gaps in journal-based statistical guidance within and across scientific disciplines. Some previous research has addressed this topic and suggested that there is probably large room for improvement. For example, Schriger, Arora, and Altman (Citation2006) reported that 39% of 166 leading medical journals provided statistical guidance to authors. Topics addressed included p-values (20%), confidence intervals (14%), statistical power (10%), multiple testing (5%), modeling (4%), sensitivity analysis (4%), and Bayesian methods (1%). Recently, Malički et al. (Citation2019) used a text-mining approach to search for a limited set of terms related to Bayes factors, confidence intervals, effect sizes, and sample size calculations in instructions to authors provided by 835 journals across a range of scientific disciplines. The prevalence of any statistical guidance, defined by these terms, was 6% across all journals. Another empirical evaluation (Malički et al. Citation2020) found that none of 57 preprint servers provided any statistical advice. These three studies provide some insight into the prevalence and content of statistical guidance offered to authors; however, Schriger, Arora, and Altman (Citation2006) was limited to medical journals, Malički et al. (Citation2019) focused on only a few statistical topics, and Malički et al. (Citation2020) was limited to preprint servers.
The goal of the present study was to describe the nature and prevalence of statistical guidance offered to authors by 15 journals (top-ranked by Impact Factor) in each of 22 scientific disciplines (N = 330 journals) across five high-level domains (Health & Life Sciences, Multidisciplinary, Social Sciences, Physical Sciences, Formal Sciences). We manually inspected journal websites to identify any author-facing guidance related to statistics. We noted how often guidance pertained to 20 prespecified statistical issues and described whether journals endorsed or opposed statistical methods related to six topics that we considered in advance to be “hotly debated” (p-values, statistical significance, effect sizes, confidence intervals, sample size planning/justification, and Bayesian statistics).
2 Materials and Methods
The study protocol (rationale, methods, and analysis plan) was preregistered on the Open Science Framework study registry on November 23rd, 2019 (https://osf.io/cz9g3/). All departures from that protocol are explicitly acknowledged in supplementary material A. All data exclusions and measures conducted during this study are reported in this manuscript. Our reporting adheres to The Strengthening the Reporting of Observational Studies in Epidemiology (STROBE) checklist (supplementary material B).
2.1 Design
The study had a cross-sectional design. We examined whether each journal provided any statistical guidance, included a dedicated statistical guidance section in its instructions to authors, or referred to any external statistical guidance (e.g., reporting guideline). For journals that provided their own statistical guidance, we recorded whether the guidance addressed each of 20 prespecified (https://osf.io/cz9g3/) topics (see ).
2.2 Sample
The sample consisted of the top 15 journals ranked by 2017 Journal Impact Factor (JIF) in each of 22 high-level scientific disciplines across five domains (N = 330 journals). Journals that only publish reviews were not included. This represents the entire population of interest. The sample size of 15 journals per discipline was based on our intuition that this would likely capture the most influential journals in each field. JIFs were obtained from Journal Citation Reports (https://jcr.clarivate.com) and scientific disciplines were defined by Essential Science Indicators (https://perma.cc/MD4V-A5X5; for details see supplementary material C). To help summarize the data, we assigned individual disciplines to higher level scientific domains (Health & Life Sciences, Multidisciplinary, Social Sciences, Physical Sciences, and Formal Sciences.
2.3 Procedure
Two investigators (T.B. & T.E.H.) manually searched each journal’s website as of November 2019 and made copies of any statistical guidance intended for authors (for details see supplementary material D). Our operational definition of statistical guidance was: any advice or instruction related to the appropriate selection, implementation, reporting, or interpretation of statistical analyses.
A group of investigators (M.M., D.S., M.S.H.) then manually examined and classified the extracted statistical guidance. All classifications were verified by a second investigator (T.E.H.) and any differences were resolved through discussion. Investigators were each randomly assigned (using the R function “sample”) to five ranks and coded journals at that rank in each of the 22 scientific disciplines (i.e., 110 journals per coder).
Investigators recorded whether journals provided a dedicated statistical guidance section in their author instructions and whether references were provided to external statistical guidance, such as reporting guidelines. We did not count general instructions to follow external guidelines (e.g., “follow EQUATOR reporting guidelines”) that did not refer to any specific guidelines.
For guidance provided directly by journals, investigators recorded whether each of 20 prespecified topics were mentioned and extracted all relevant verbatim guidance pertaining to those topics. For the topic “prespecification of analyses,” we did not count general instructions to register clinical trials unless they specifically referred to statistical analyses in some way, for example, a requirement to share the original statistical analysis plan.
For six topics that we considered in advance to be “hotly debated” in the statistical literature (p-values, statistical significance, confidence intervals, effect sizes, sample size planning/justification, and Bayesian statistics), one investigator (T.E.H.) examined the extracted verbatim guidance and classified the level of endorsement offered by the journal according to four categories:
Explicit endorsement—the journal advises or instructs authors to use this method whenever possible/appropriate (e.g., “Authors should report p-values”).
Implicit endorsement—the journal provides advice on the method, implying endorsement, but does not explicitly advise that the method should be used (e.g., “Articles that use significance testing should report the alpha level for all tests”).
Implicit opposition—the journal advises that they would prefer the method is not used, but does not explicitly rule it out (e.g., “articles should not usually contain statistical significance testing”).
Explicit opposition—the journal advises that the method should not be used (e.g., “Authors should replace p-values with effect size estimates and 95% confidence intervals”).
For guidance pertaining to p-values, statistical significance, and confidence intervals, one investigator (T.E.H.) recorded whether there were instructions to report exact p-values/alpha levels/confidence levels or use specific p-value thresholds/alpha/confidence levels (e.g., p < 0.05,
, 95% confidence interval).
2.4 Data Analysis
We report descriptive statistics (number and proportion of journals) stratified by domains and disciplines. Analyses were performed using custom code written in the programming language R (R Core Team Citation2021).
3 Results
3.1 Journal Impact Factors
Across all 330 journals, the median 2017 Journal Impact Factor was 7.54 (IQR = 6.56; range 2.45–79.26). Journal Impact Factors for each scientific discipline are available in supplementary Table E1.
3.2 Frequency of Statistical Guidance in General
Overall, we found that 160 journals offered some statistical guidance. This includes 32 journals that only referred to statistical guidance in external sources (reporting guidelines or academic papers) and 128 journals that provided their own guidance (of which 105 also referred to external sources). Of the 160 journals offering statistical guidance, 93 had a dedicated statistics section in their author instructions. Some journals shared the same publisher-level guidance, including 31 Nature journals, 12 Cell journals, and 2 Frontiers journals.
There was considerable heterogeneity across disciplines and domains (; for equivalent tabular data, see https://osf.io/pkjhf/). The frequency of statistical guidance was highest in the Health & Life Sciences (122 of 165 journals, 74%), followed by Multidisciplinary journals (9 of 15 journals, 60%), Social Sciences (8 of 30 journals, 27%), Physical Sciences (21 of 90 journals, 23%), and the Formal Sciences (0 of 30 journals, 0%). In one discipline (Clinical Medicine) Statistical guidance was provided by all examined journals and in two disciplines (Mathematics and Computer Science) no examined journals provided statistical guidance.
Fig. 1 Frequency of journals offering some statistical guidance (circles) or having a dedicated statistical guidance section (diamonds) in each scientific discipline (bars not highlighted, n = 15 journals per discipline) and each higher-level scientific domain (bars highlighted; Health & Life Sciences: n = 165 journals; Multidisciplinary: n = 15; Social Sciences: n = 30; Physical Sciences: n = 90; Formal Sciences, n = 30). Data is presented in descending order of frequency first by domain, then by discipline within domains.
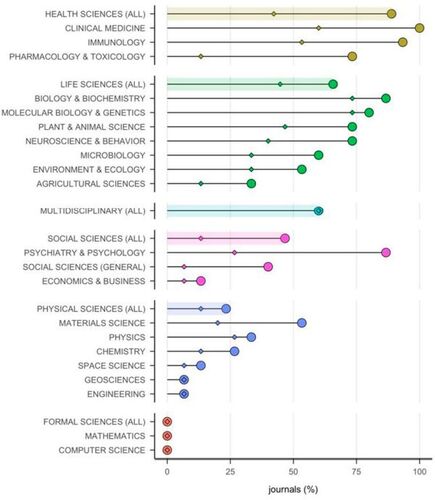
3.3 Frequency of Statistical Guidance on Specific Topics
Of the 128 journals that provided their own guidance, 117 mentioned at least one of the 20 prespecified statistical topics (supplementary material Figures F1–F22) and 11 did not mention any of these topics (but did provide guidance on other miscellaneous statistical issues). The maximum number of topics mentioned by an individual journal was 15. The median topics mentioned was 6 (IQR = 8; supplementary material Figure F23).
The frequency of guidance pertaining to each of 20 prespecified statistical topics is shown stratified by domain in and stratified by discipline in supplementary material Figures G1–G4. Overall, guidance was most common for confidence intervals (n = 90 journals), p-values (n = 88), sample size planning/justification (n = 72), effect sizes (n = 66), prespecification of analyses (n = 65), and data exclusions (n = 64). Guidance was less common for checking model assumptions (n = 61), multiple comparisons (n = 60), statistical significance (n = 53), one-tailed hypothesis tests (n = 51), Bayesian statistics (n = 39), null hypotheses (n = 33), subgroup analyses (n = 30), secondary outcomes (n = 25), missing data (n = 18), sensitivity analyses (n = 11), nonparametric tests (n = 10), baseline covariates (n = 9), outliers (n = 7), and categorization of continuous outcomes (n = 2). Guidance on the 20 topics tended to be more common in the Health & Life Sciences and Multidisciplinary journals compared to other domains () and was especially common in the Clinical Medicine journals compared to other disciplines (supplementary material Figures G1–G4). Examples of verbatim guidance are provided in supplementary Table H1. A sensitivity analyses excluding journals that shared publisher-level guidance is available in supplementary material I.
Fig. 2 Frequency of journals offering guidance on 20 prespecified statistical topics. Data is stratified by scientific domain (Health & Life Sciences: n = 165 journals; Multidisciplinary: n = 15; Social Sciences: n = 30; Physical Sciences: n = 90); the Formal Sciences are not shown because no journals provided statistical guidance. Data is presented in descending order of overall frequency across all domains. CCV = categorizing continuous variables.
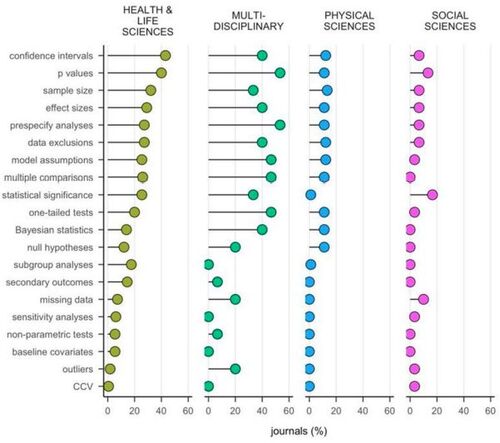
3.4 External Statistical Guidance
One-hundred and thirty-seven journals referred authors to statistical guidance in external sources (supplementary material J). Of these, 32 only referred to statistical guidance in external sources, whereas the other 105 also provided their own additional guidance. Seventy-nine different external sources were referred to, of which 49 were reporting guidelines and 30 were other types of documents, such as academic papers, textbooks, or society guidelines. Referring to external guidance was most common in the Health & Life Sciences (109 of 165 journals, 66%) followed by Multidisciplinary journals (8 of 15 journals, 53%), Physical Sciences (17 of 90 journals, 19%), Social Sciences (3 of 30 journals, 10%), and Formal Sciences (0 of 30 journals, 0%).
The most commonly referred to reporting guidelines were CONSORT (n = 95 journals), ARRIVE (n = 80), PRISMA (n = 47), REMARK (n = 40), and STARD (n = 36), with all other reporting guidelines being referred to by 21 or fewer journals. All other types of external guidance were referred to by 3 or fewer journals (supplementary material J).
3.5 Statistical Guidance on “Hotly Debated” Topics
shows how many of the examined journals offered different levels of endorsement for six statistical topics that we considered in advance to be “hotly debated” in the statistical literature (note that these only partly overlap with the most commonly mentioned statistical topics in journal guidance, as shown in ). Ninety journals provided guidance on confidence intervals, of which the vast majority (n = 85) offered explicit endorsement. Most of these journals (n = 69) did not provide any guidance on confidence levels, but 16 advised authors to use a 95% confidence level and 5 advised authors to report their chosen confidence level.
Table 1 Number of journals offering various levels of endorsement for six “hotly debated” statistical topics.
Eighty-eight journals provided guidance on p-values, of which the vast majority offered implicit endorsement (n = 77). One journal explicitly opposed the use of p-values, but only in the absence of prespecified multiplicity corrections. Fifty-two journals advised reporting exact p-values, 21 advised reporting of exact p-values unless they were very small (e.g., p < 0.001), 5 advised authors to use reporting thresholds (e.g., p < 0.05, p < 0.01, p < 0.001), and 10 offered no guidance on whether to report exact p-values or use thresholds.
Fifty-three journals provided guidance on statistical significance, of which the majority (n = 35) offered implicit endorsement. Three journals explicitly opposed the use of statistical significance, instructing authors to report exact p-values and/or standard errors/confidence intervals instead. Thirty journals advised authors to report the alpha level they used, 2 advised using an alpha level of 0.05, 1 advised using an alpha level of 0.01, and 20 offered no guidance on alpha levels. Seventy-two journals provided guidance on sample size planning/justification, of which the vast majority (n = 67) offered explicit endorsement. 66 journals offered guidance on effect sizes, of which the vast majority (n = 62) offered explicit endorsement. All 39 journals that provided guidance about Bayesian statistics provided implicit endorsement.
4 Discussion
Our investigation revealed considerable heterogeneity in the provision of statistical guidance by 330 top-ranked journals across scientific disciplines and domains. The vast majority of the examined Health & Life Sciences journals provided statistical guidance (including all Clinical Medicine journals), as did the majority of the examined Multidisciplinary journals. Less than half of the examined journals in the Social Sciences and Physical Sciences provided statistical guidance, and none of the examined journals in the Formal Sciences (Mathematics and Computer Science) did. Of the total 160 journals that offered some statistical guidance, 67 did not have a dedicated statistical guidance section in their author instructions. Statistical guidance addressed a range of topics, and was most common for hotly debated topics (p-values, confidence intervals, sample size planning/justification, and effect sizes) with the emphasis varying across disciplines. Few of the examined journals appeared to take particularly controversial positions, such as banning p-values or the use of statistical significance.
Our finding that 48% of the 330 examined journals across disciplines provided statistical guidance is substantially higher than observed in prior research on this topic. Malički et al. (Citation2019) found that statistical guidance was provided by only 6% of 835 journals across a similarly broad range of scientific disciplines; however, this estimate was based on a text-mining approach sensitive to only a narrow range of statistical topics (specifically, Bayes factors, confidence intervals, effect sizes, and power/sample size calculations). Additionally, our sample only included journals with high Impact Factors whereas Malički et al. examined a representative (randomly selected) sample of journals across a range of citation impact. It is possible that journals with lower citation impact are less likely to provide statistical guidance (as is the case for authorship and conflict of interest guidance according to a recent systematic review, Malički et al. Citation2021). Our methodology is more comparable to Schriger, Arora, and Altman (Citation2006) who reported that 39% of 166 major medical journals provided statistical guidance. By contrast, all of the medical journals we examined provided statistical guidance; however, we only examined the 15 journals with the highest Impact Factor. It is possible that there has been an increase in the provision of statistical guidance over time (as has been shown for guidance related to authorship, conflicts of interest, and data sharing, Malički et al. Citation2021), but empirical comparisons are not possible because journal-level information is not provided in Schriger et al. Our study contributes to this literature by establishing a benchmark against which future studies of journal-based statistical guidance can be compared.
Although there was substantial variation across disciplines in the provision of statistical guidance, our study was not designed to detect reasons for these differences. It is plausible that the examined Health & Life Sciences journals were more likely to provide statistical guidance because (a) it seems especially important to avoid errors with highly proximal repercussions for human health; (b) biomedical and health-related research is often subject to stricter regulatory oversight; (c) there is a plethora of empirical data highlighting the severity and prevalence of statistical misuse, misreporting, and misinterpretation in health research (Altman Citation1994; Strasak et al. Citation2007); (d) journals may feel they need to compensate for lower statistical competency among Health & Life Sciences researchers due to inadequate educational programs or statistical support services (Aiken, West, and Millsap Citation2008; Windish, Huot, and Green Citation2007). Differences in the use of statistics between disciplines is also likely to be a major factor; for example, statistics are likely to be less useful in the Formal Sciences relative to more applied sciences and may explain the absence of statistical guidance in the examined Computer Science and Mathematics journals. Cultural or historic factors related to the role that journals traditionally play in the oversight of research may also be important, though we are not aware of relevant empirical evidence that documents this.
Moreover, because statistical methods and their optimal use differ across scientific subfields it is difficult to score or rank journal-based statistical guidance. It may be useful to assess the accuracy, thoroughness, and consistency of statistical guidance at the level of individual journals; however, that was beyond the scope of our study. Future work may try to assess such performance by examining not only statistical guidance but also the use and misuse of statistics in specific papers published by each journal.
It is unclear whether journal-based statistical guidance is an effective means to address misuse, misreporting, and misinterpretation of statistics. Indeed, one may be skeptical about whether instructions to authors are even commonly read. Some evidence does suggest that, at least in particular scenarios, editorial advocacy for specific statistical practices has been effective. For example, several journals have successfully encouraged authors to abandon null-hypothesis significance testing in favor of an estimation approach centered on reporting effect sizes and confidence intervals (Fidler et al. Citation2004; Finch et al. Citation2004; Giofrè et al. Citation2017). However, these effects seem at least partly dependent on the active campaigning and oversight of specific editors, rather than written guidance to authors alone. A coordinated approach in which the journal’s expectations about statistical practice are made explicit in author instructions, then reinforced by editors and reviewers is likely important. Soliciting dedicated statistical reviewers may be more effective at addressing statistical issues beyond simple reporting and previous studies have found that the use of statistical review is rare in some fields, such as psychology (Hardwicke et al. Citation2019) and relatively common in other fields, such as biomedicine (Hardwicke and Goodman Citation2020).
Journals are only one interventional lever for improving statistical practice and an exclusive focus on journals is likely to be insufficient. In one study, Fidler et al. (Citation2004) observed that although authors tended to follow instructions to report confidence intervals, they often showed little evidence of using them to aid their interpretation of the research results. Ultimately, any journal-based intervention intended to improve statistical practice is likely to be limited by the knowledge, motivation, and understanding of authors (and reviewers), the improvement of which will require nontrivial changes to reward structures and educational practices deeply rooted in the scientific ecosystem (Goodman Citation2019).
As noted in the introduction, even well-intentioned statistical guidance offered by journals may be detrimental. This was clear in the case of the flawed statistic introduced at the journal Psychological Science (Iverson et al. Citation2009; Killeen Citation2005) and arguably the case with the ongoing p-value ban at the journal Basic and Applied Social Psychology, depending on one’s statistical philosophy (Fricker et al. Citation2019; Trafimow and Marks Citation2015). Mayo (Citation2021) has recently argued that journal editors should enforce proper use of statistical methods but avoid “taking sides” on particularly controversial matters, or advocating for specific statistical philosophies. However, the definition of “proper use” may be in the eye of the beholder and some experimentation with different approaches could be informative, if ethically appropriate and properly monitored with meta-research (Hardwicke et al. Citation2020). Nevertheless, we found that very few journals in our sample appeared to be outwardly and decisively “taking sides” in the sense of explicitly opposing the use of “hotly debated” statistical tools like p-values and statistical significance; however, the choice of wording often implicitly endorsed or opposed certain practices, which suggests journals may be “taking sides” albeit without clear language.
It was common for journals to provide their own idiosyncratic statistical guidance. However, some publishers had developed guidance that was applied across multiple journals, often in the form of checklists (e.g., the Nature Life Sciences Reporting Checklist). Moreover, many journals (especially in the Health & Life Sciences) referred to the same reporting guidelines, particularly the most established ones available on the EQUATOR website, like CONSORT, ARRIVE, and PRISMA. It is unclear whether authors refer to, and whether editors and reviewers enforce, external reporting guidelines comparably with internal ones. Increasing standardization could improve efficiency and consistency for authors, reviewers, and readers; but some degree of variability and experimentation may also be desirable. It is unclear where the balance lies.
Our study has some important limitations. (a) We focused only on statistical guidance provided on journal websites. We do not know how many authors actually read this information, or the extent to which journals enforce their use through editorial oversight or statistical review (Hardwicke et al. Citation2019; Hardwicke and Goodman Citation2020); (b) We deliberately based our sample on 22 broadly defined disciplines to ensure wide coverage across the sciences, but this does not necessarily imply that the findings will straightforwardly generalize to lower-level sub-fields within those disciplines. Moreover, we focused only on journals with the highest Impact Factor because they are likely to have the clearest potential to modify author behavior. Lower-ranked journals may differ in many important respects; for example, they may have fewer resources to dedicate to developing statistical guidance. It is unclear if they might be more or less willing than top-ranked journals to introduce policy changes that could cause controversy, such as p-value bans. (c) As noted, for the topic “preregistration of analyses” we did not count general instructions to register clinical trials unless they specifically referred to the prespecification of statistical analyses. It is possible that some of the examined journals assumed a general instruction to register trials also constituted a specific instruction to prespecify statistical analyses; however, we did not consider that to be sufficiently direct. Similarly, we did not consider a general instruction to authors that they should follow EQUATOR reporting guidelines (without specifically mentioning individual guidelines) to be sufficiently direct and did not count it as statistical guidance.
In conclusion, statistics are often a vital pillar in the edifice of scientific claims, but they are widely misused, misreported, and misinterpreted across several scientific disciplines (Altman Citation1982; Gigerenzer Citation2018; Nieuwenhuis, Forstmann, and Wagenmakers Citation2011; Sedlmeier and Gigerenzer Citation1989; Strasak et al. Citation2007; Wasserstein and Lazar Citation2016). Journals could play a key role in alleviating these statistical ailments before they plague the academic literature. However, our investigation shows that even at top-ranked journals, only a minority provide any statistical guidance. Moreover, there are inconsistencies in the guidance provided across journals. Our investigation provides a layer of empirical evidence upon which to inform ongoing research and debate about the role of journals in improving the statistical practice of scientists.
Supplementary Materials
The supplementary materials contain protocol amendments (supplementary materials A), a STROBE reporting checklist (supplementary materials B), definitions of scientific disciplines (supplementary materials C), procedures for preserving statistical guidance (supplementary materials D), journal Impact Factors stratified by discipline (supplementary materials E), additional data on twenty prespecified statistical topics (supplementary materials F), additional graphs for study one (supplementary materials G), examples of guidance for each of twenty statistical topics (supplementary materials H), the results of a sensitivity analysis on statistical topics without shared publisher-guidance (supplementary materials I) and external sources of statistical guidance (supplementary materials J).
Authors’ Contributions
Conceptualization: T.E.H., J.P.A.I., M.M., and D.S. Data curation: T.E.H. and M.S.H. Formal analysis: T.E.H. and M.S.H. Investigation: T.E.H., M.S.H., M.M., and T.B. Methodology: T.E.H., J.P.A.I., M.M., and D.S. Project administration: T.E.H. Resources: T.E.H. Software: T.E.H. Supervision: T.E.H. and J.P.A.I. Validation: T.E.H. Visualization: T.E.H. Writing – original draft: T.E.H. Writing – review & editing: T.E.H., J.P.A.I., M.S.H., M.M., D.S., and T.B.
Supplemental Material
Download PDF (5.2 MB)Data Availability Statement
All data, materials, and analysis scripts related to this study are publicly available on the Open Science Framework repository (https://dx.doi.org/10.17605/OSF.IO/JRX7D). To facilitate reproducibility this manuscript was written by interleaving regular prose and analysis code using knitr (Xie Citation2017) and papaja (Aust and Barth Citation2020), and is available in a Code Ocean container (https://doi.org/10.24433/CO.6131540.v1) which recreates the software environment in which the original analyses were performed.
Disclosure Statement
Mario Malički is a Co-Editor-in-Chief of the journal Research Integrity and Peer Review. All other authors declare no conflicts of interest.
Additional information
Funding
References
- Aiken, L. S., West, S. G., and Millsap, R. E. (2008), “Doctoral Training in Statistics, Measurement, and Methodology in Psychology: Replication and Extension of Aiken, West, Sechrest, and Reno’s (1990) survey of PhD programs in North America,” The American Psychologist, 63, 32–50. DOI: 10.1037/0003-066X.63.1.32.
- Altman, D. G. (1982), “Statistics in Medical Journals,” Statistics in Medicine, 1, 59–71. DOI: 10.1002/sim.4780010109.
- Altman, D. G. (1994), “The Scandal of Poor Medical Research,” British Medical Journal (Clinical Research ed.), 308, 283–284. DOI: 10.1136/bmj.308.6924.283..
- Altman, D. G., Gore, S. M., Gardner, M. J., and Pocock, S. J. (1983), “Statistical Guidelines for Contributors to Medical Journals,” British Medical Journal (Clinical Research ed.), 286, 1489–1493. DOI: 10.1136/bmj.286.6376.1489.
- Aust, F., and Barth, M. (2020), “Papaja: Create APA Manuscripts with rmarkdown,” available at https://github.com/crsh/papaja
- Bailar, J. C., and Mosteller, F. (1988), “Guidelines for Statistical Reporting in Articles for Medical Journals,” Annals of Internal Medicine, 108, 266–273. DOI: 10.7326/0003-4819-108-2-266.
- Benjamin, D. J., Berger, J. O., Johannesson, M., Nosek, B. A., Wagenmakers, E.-J., Berk, R., Bollen, K. A., Brembs, B., Brown, L., Camerer, C., Cesarini, D., Chambers, C. D., Clyde, M., Cook, T. D., De Boeck, P., Dienes, Z., Dreber, A., Easwaran, K., Efferson, C., Fehr, E., Fidler, F., Field, A. P., Forster, M., George, E. I., Gonzalez, R., Goodman, S., Green, E., Green, D. P., Greenwald, A. G., Hadfield, J. D., Hedges, L. V., Held, L., Hua Ho, T., Hoijtink, H., Hruschka, D. J., Imai, K., Imbens, G., Ioannidis, J. P. A., Jeon, M., Jones, J. H., Kirchler, M., Laibson, D., List, J., Little, R., Lupia, A., Machery, E., Maxwell, S. E., McCarthy, M., Moore, D. A., Morgan, S. L., Munafó, M., Nakagawa, S., Nyhan, B., Parker, T. H., Pericchi, L., Perugini, M., Rouder, J., Rousseau, J., Savalei, V., Schönbrodt, F. D., Sellke, T., Sinclair, B., Tingley, D., Van Zandt, T., Vazire, S., Watts, D. J., Winship, C., Wolpert, R. L., Xie, Y., Young, C., Zinman, J., and Johnson, V. E. (2018), “Redefine Statistical Significance,” Nature Human Behaviour, 2, 6–10. DOI: 10.1038/s41562-017-0189-z.
- Cumming, G. (2014), “The New Statistics: Why and How,” Psychological Science, 25, 7–29. DOI: 10.1177/0956797613504966.
- Fidler, F., Thomason, N., Cumming, G., Finch, S., and Leeman, J. (2004), “Editors can Lead Researchers to Confidence Intervals, but can’t make them think: Statistical Reform Lessons from Medicine,” Psychological Science, 15, 119–126. DOI: 10.1111/j.0963-7214.2004.01502008.x.
- Finch, S., Cumming, G., Williams, J., Palmer, L., Griffith, E., Alders, C., Anderson, J., and Goodman, O. (2004), “Reform of Statistical Inference in Psychology: The Case of Memory & Cognition,” Behavior Research Methods, Instruments, & Computers, 36, 312–324. DOI: 10.3758/BF03195577.
- Fricker, R. D., Burke, K., Han, X., and Woodall, W. H. (2019), “Assessing the Statistical Analyses used in Basic and Applied Social Psychology after their p-value Ban,” The American Statistician, 73, 374–384. DOI: 10.1080/00031305.2018.1537892.
- Gigerenzer, G. (2018), “Statistical Rituals: The Replication Delusion and How We Got There,” Advances in Methods and Practices in Psychological Science, 1, 198–218. DOI: 10.1177/2515245918771329.
- Giofrè, D., Cumming, G., Fresc, L., Boedker, I., and Tressoldi, P. (2017), “The Influence of Journal Submission Guidelines on Authors’ Reporting of Statistics and Use of Open Research Practices,” PloS One, 12, e0175583. DOI: 10/f93jf8.
- Goodman, S. N. (1999), “Toward Evidence-based Medical Statistics. 2: The Bayes Factor,” Annals of Internal Medicine, 130, 1005–1013. DOI: 10.7326/0003-4819-130-12-199906150-00019.
- Goodman, S. N. (2019), “Why is Getting Rid of p-values so Hard? Musings on Science and Statistics,” The American Statistician, 73, 26–30. DOI: 10.1080/00031305.2018.1558111..
- Hardwicke, T. E., Frank, M. C., Vazire, S., and Goodman, S. N. (2019), “Should Psychology Journals Adopt Specialized Statistical Review?” Advances in Methods and Practices in Psychological Science, 2, 240–249. DOI: 10.1177/2515245919858428.
- Hardwicke, T. E., and Goodman, S. N. (2020), “How often do Leading Biomedical Journals Use Statistical Experts to Evaluate Statistical Methods? The Results of a Survey,” PloS One, 15, e0239598. DOI: 10.1371/journal.pone.0239598.
- Hardwicke, T. E., Serghiou, S., Janiaud, P., Danchev, V., Crüwell, S., Goodman, S. N., and Ioannidis, J. P. A. (2020), “Calibrating the Scientific Ecosystem through Meta-Research,” Annual Review of Statistics and Its Application, 7, 11–37. DOI: 10.1146/annurev-statistics-031219-041104.
- Iverson, G. J., Lee, M. D., Zhang, S., and Wagenmakers, E.-J. (2009), “prep: An Agony in Five Fits,” Journal of Mathematical Psychology, 53, 195–202. DOI: 10.1016/j.jmp.2008.09.004.
- Killeen, P. R. (2005), “An Alternative to Null-Hypothesis Significance Tests,” Psychological Science, 16, 345–353. DOI: 10.1111/j.0956-7976.2005.01538.x.
- Kruschke, J. K., and Liddell, T. M. (2018), “Bayesian Data Analysis for Newcomers,” Psychonomic Bulletin & Review, 25, 155–177. DOI: 10.3758/s13423-017-1272-1.
- Lakens, D., Adolfi, F. G., Albers, C. J., Anvari, F., Apps, M. A. J., Argamon, S. E., Baguley, T., Becker, R. B., Benning, S. D., Bradford, D. E., Buchanan, E. M., Caldwell, A. R., Van Calster, B., Carlsson, R., Chen, S.-C., Chung, B., Colling, L. J., Collins, G. S., Crook, Z., Cross, E. S., Daniels, S., Danielsson, H., DeBruine, L., Dunleavy, D. J., Earp, B. D., Feist, M. I., Ferrell, J. D., Field, J. G., Fox, N. W., Friesen, A., Gomes, C., Gonzalez-Marquez, M., Grange, J. A., Grieve, A. P., Guggenberger, R., Grist, J., van Harmelen, A.-L., Hasselman, F., Hochard, K. D., Hoffarth, M. R., Holmes, N. P., Ingre, M., Isager, P. M., Isotalus, H. K., Johansson, C., Juszczyk, K., Kenny, D. A., Khalil, A. A., Konat, B., Lao, J., Larsen, E. G., Lodder, G. M. A., Lukavský, J., Madan, C. R., Manheim, D., Martin, S. R., Martin, A. E., Mayo, D. G., McCarthy, R. J., McConway, K., McFarland, C., Nio, A. Q. X., Nilsonne, G., de Oliveira, C. L., de Xivry, J.-J. O., Parsons, S., Pfuhl, G., Quinn, K. A., Sakon, J. J., Saribay, S. A., Schneider, I. K., Selvaraju, M., Sjoerds, Z., Smith, S. G., Smits, T., Spies, J. R., Sreekumar, V., Steltenpohl, C. N., Stenhouse, N., Świątkowski, W., Vadillo, M. A., Van Assen, M. A. L. M., Williams, M. N., Williams, S. E., Williams, D. R., Yarkoni, T., Ziano, I., and Zwaan, R. A. (2018), “Justify your Alpha,” Nature Human Behaviour, 2, 168–171. DOI: 10.1038/s41562-018-0311-x.
- Malički, M., Aalbersberg, I. J., Bouter, L., and ter Riet, G. (2019), “Journals’ Instructions to Authors: A Cross-sectional Study across Scientific Disciplines,” PloS One, 14, e0222157. DOI: 10.1371/journal.pone.0222157.
- Malički, M., Jerončić, A., Aalbersberg, I. J., Bouter, L., and ter Riet, G. (2021), “Systematic Review and Meta-Analyses of Studies Analysing Instructions to Authors from 1987 to 2017,” Nature Communications, 12, 5840. DOI: 10.1038/s41467-021-26027-y.
- Malički, M., Jerončić, A., ter Riet, G., Bouter, L. M., Ioannidis, J. P. A., Goodman, S. N., and Aalbersberg, I. J. (2020), “Preprint Servers’ Policies, Submission Requirements, and Transparency in Reporting and Research Integrity Recommendations,” JAMA, 324, 1901–1903. DOI: 10.1001/jama.2020.17195.
- Mayo, D. G. (2018), Statistical Inference as Severe Testing: How to Get beyond the Statistics Wars (1st ed.), Cambridge: Cambridge University Press. https://www.cambridge.org/core/product/identifier/9781107286184/type/book
- Mayo, D. G. (2021), “The Statistics Wars and Intellectual Conflicts of Interest,” Conservation Biology, 36, e13861. DOI: 10.1111/cobi.13861..
- McShane, B. B., Gal, D., Gelman, A., Robert, C., and Tackett, J. L. (2019), “Abandon Statistical Significance,” The American Statistician, 73, 235–245. DOI: 10.1080/00031305.2018.1527253.
- Nieuwenhuis, S., Forstmann, B. U., and Wagenmakers, E.-J. (2011), “Erroneous Analyses of Interactions in Neuroscience: A Problem of Significance,” Nature Neuroscience, 14, 1105–1107. DOI: 10.1038/nn.2886.
- R Core Team (2021), R: A Language and Environment for Statistical Computing, Vienna, Austria: R Foundation for Statistical Computing, Available at https://www.R-project.org/.
- Schriger, D. L., Arora, S., and Altman, D. G. (2006), “The Content of Medical Journal Instructions for Authors,” Annals of Emergency Medicine, 48, 743–749.e4. DOI: 10.1016/j.annemergmed.2006.03.028.
- Sedlmeier, P., and Gigerenzer, G. (1989), “Do Studies of Statistical Power have an Effect on the Power of Studies?” Psychological Bulletin, 105, 309–316. DOI: 10.1037/0033-2909.105.2.309.
- Smith, R. (2005), “Statistical Review for Medical Journals, Journal’s Perspective,” in Encyclopedia of Biostatistics, eds. P. Armitage and T. Colton, New Jersey: Wiley. DOI: 10.1002/0470011815.b2a17141..
- Strasak, A. M., Zaman, Q., Marinell, G., Pfeiffer, K. P., and Ulmer, H. (2007), “The Use of Statistics in Medical Eesearch: A Comparison of the New England Journal of Medicine and Nature Medicine,” The American Statistician, 61, 47–55. DOI: 10.1198/000313007X170242.
- Trafimow, D., and Marks, M. (2015), “Editorial,” Basic and Applied Social Psychology, 37, 1–2. DOI: 10.1080/01973533.2015.1012991.
- Turner, L., Shamseer, L., Altman, D. G., Weeks, L., Peters, J., Kober, T., Dias, S., Schulz, K. F., Plint, A. C., and Moher, D. (2012), “Consolidated Standards of Reporting Trials (CONSORT) and the Completeness of Reporting of Randomised Controlled Trials (RCTs) Published in Medical Journals,” The Cochrane Database of Systematic Reviews, 11, MR000030. DOI: 10.1002/14651858.MR000030.pub2.
- Wagenmakers, E.-J. (2007), “A Practical Solution to the Pervasive Problems of p values,” Psychonomic Bulletin & Review, 14, 779–804. DOI: 10.3758/BF03194105.
- Wasserstein, R. L., and Lazar, N. A. (2016), “The ASA’s Statement on p-values: Context, Process, and Purpose,” The American Statistician, 70, 129–133. DOI: 10.1080/00031305.2016.1154108.
- Wasserstein, R. L., Schirm, A. L., and Lazar, N. A. (2019), “Moving to a World Beyond “p < 0.05”,” The American Statistician, 73, 1–19. DOI: 10.1080/00031305.2019.1583913..
- Windish, D. M., Huot, S. J., and Green, M. L. (2007), “Medicine Residents’ understanding of the Biostatistics and Results in the Medical Literature,” JAMA, 298, 1010–1022. DOI: 10.1001/jama.298.9.1010.
- Xie, Y. (2017), Dynamic Documents with R and Knitr, Boca Raton, FL: CRC Press.