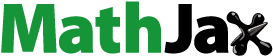
ABSTRACT
Migrants into European countries are often less educated than European natives. We analyse whether migrants’ children are more or less likely than natives’ children to achieve upward educational mobility across generations, and study differences in the factors, which contribute to differences in mobility for the two groups. We find that migrants’ descendants are more often upwardly mobile (and less often downwardly mobile) than their native peers in the majority of countries studied, and show that the main factor contributing to these patterns is the education level of parents. Although a lower parental education means that their children are less likely to access the same amount of human, social and financial capital as children of more highly educated parents, migrants’ descendants over the last two generations were able to make significant progress in reducing education gaps with natives.
I. Introduction
The tremendous influx of immigrants into Europe over the past several decades, particularly of people from poorer and politically less stable countries, has been the source of large social and demographic shifts. A particular challenge of these shifts is that migrants into western European countries are typically less educated than people in the native population. If immigrants’ children are unable to surpass their parents’ education levels and get closer to reducing the gap between their own and natives’ education levels, migrant communities will remain at a disadvantage and more likely be a burden for their destination countries’ public welfare systems (see Barrett and McCarthy Citation2007; Blume and Verner Citation2007; Boeri Citation2010; Pellizzari Citation2013; Huber and Oberdabernig Citation2016). In this article, we examine the probability that descendants of native-born versus foreign-born parents reach higher education levels than their parents in 11 European countries. Further, we identify the drivers of the differences in intergenerational mobility for the two groups.
The literature on migrants’ (and their descendants’) educational attainment shows that migrant academic success is often significantly lower than that of native-born persons (as shown in Schütz, Ursprung, and Wößmann Citation2008; Schneeweis Citation2011; Dustmann, Frattini, and Lanzara Citation2012; Aydemir, Chen, and Corak Citation2013; Schneebaum, Rumplmaier, and Altzinger Citation2016; for example). In this article, we investigate whether this gap in achievement narrows across generations by studying differences in intergenerational mobility for the two groups. We are interested in knowing if second-generation migrants or natives in the last generation were more likely to reach higher education than their parents, and in particular, in explaining which factors drive these patterns. If the children of lower educated migrants were more likely to surpass their parents’ education level or make greater strides than their native peers, migrants’ children are less likely to remain disadvantaged across generations as they narrow the gap in educational attainment with natives.
A challenge for catching up in terms of education is that school performance is strongly related to one’s social and economic background.Footnote1 Migrants’ worse educational outcomes in many countries are due at least in part to the socioeconomic conditions related to their families having come from another country, in particular lower levels of financial, social and human capital (e.g. Riphahn Citation2003; Colding Citation2006; Schneeweis Citation2011; Lüdemann and Schwerdt Citation2013) and what Lüdemann and Schwerdt (Citation2013) call ‘general inequalities’ which affect migrant students’ educational success. Educational upward mobility for migrants would thus come in spite of the circumstances around their migration background. Relatedly, though, much of the literature on the economics of immigration suggests that migrants might be more skilled than people who choose to stay in their country of birth, because more able and motivated people are the ones who self-select into migration (see Chiswick Citation1978, Citation1999; Borjas Citation1987, Citation1994).
The empirical literature on educational attainment shows that for the population as a whole, institutions such as preschool enrolment (the earlier, the better) (Schütz, Ursprung, and Wößmann Citation2008; Bauer and Riphahn Citation2009b), age of entry into school (again, the earlier the better) (Deming and Dynarski Citation2008; Bauer and Riphahn Citation2009a) and age of first tracking (later is better) (Dustmann Citation2004; Bauer and Riphahn Citation2006a; Hanushek and Wößmann Citation2006; Schütz, Ursprung, and Wößmann Citation2008; Wößmann Citation2009) all contribute to greater intergenerational mobility. Footnote2 Analyses which look instead more specifically at differences in educational mobility between immigrants and natives usually find that the educational outcome of migrants’ children is determined by their parents’ educational achievement to a lesser extent than it is for natives’ descendants, which is typically interpreted as greater educational mobility for migrants. Summary statistics in Gang and Zimmermann (Citation2000), Dustmann (Citation2008) and Yaman (Citation2014) show that children of the on-average less educated migrants in Germany are more mobile than their native peers. Similarly, Bauer and Riphahn (Citation2006b) find that in Switzerland, a greater percentage of children from low-educated migrants compared to low-educated natives are upwardly mobile, while Schneebaum, Rumplmaier, and Altzinger (Citation2016) show that in Austria, these results are gender specific: second-generation sons are more likely than natives’ sons to be upwardly mobile, but that daughters of migrants are less likely than daughters of natives to be mobile. Finally, the results in Van Ours and Veenman (Citation2003) suggest that controlling for the lower education of the immigrant parents renders the second-generation education gap statistically insignificant in the Netherlands. None of these papers go into a discussion of the drivers of the mobility differences, which is one of the contributions of this article.
Thus, while the existing body of literature has been very valuable for painting a picture of how intergenerational mobility differs by migration background and which institutions help mobility for the broader population, it has been quiet on the drivers of mobility differences between the migrant and native populations. An exception is a study by Bauer and Riphahn (Citation2013), which looks at the institutional characteristics that differently affect the probability of mobility for migrants and natives in Switzerland. Bauer and Riphahn (Citation2013) study the joint effects of institutional differences across Swiss cantons and find that it is early kindergarten entry which most positively affects the chances of intergenerational mobility for second-generation migrants, while later tracking and especially kindergarten attendance and early school entry positively affects the chances of upward mobility for natives. While the analysis in Bauer and Riphahn (Citation2013) is at the level of institutional differences, it would also be useful to have an understanding of mobility drivers at the individual level, which we provide here.
The contribution of this article to the literature is thus threefold. First, in contrast to the majority of previous studies, we explicitly distinguish between educational upward and downward mobility, rather than interpreting the strength of intergenerational correlations or elasticities as the key indicator of mobility. Weak elasticities and correlations imply more intergenerational mobility, but cannot speak to the direction of the mobility. In order to be able to say whether intergenerational mobility for migrants is such that it is also closing the gap in educational attainment with natives, migrants’ mobility must be more likely to be upward. We thus analyse the differing probabilities of upward and downward mobility for the two populations, separately. In addition, we analyse the magnitude of upward mobility by comparing individuals whose educational attainment is two classes higher than those of their parents.
Second, we address the fact that in much of the empirical literature, the intergenerational correlations or elasticities of the migrant and native populations are compared without accounting for the fact that migrants are a priori more likely to be upwardly mobile, because their parents often have less education than natives’ parents. The lower educational attainment of the migrants’ parents gives second-generation migrants a lower threshold to pass in order to be upwardly mobile. Ignoring this fact could easily lead to a misinterpretation of the greater mobility observed for migrants in the literature. In our analysis, we account for the fact that migrant parents are often less educated than natives, and test for mobility differences that may exist once eliminating the difference in the thresholds to pass across populations. To do so, we compare the mobility patterns of children of natives and migrants with the same education level.
Finally, to our knowledge, we are the first to investigate which personal, parental and household-level characteristics are correlated with the mobility differences between natives’ and migrants’ children. In doing so, we provide some explanations of the mobility patterns that have been detected in many previous studies, but which have been left broadly unexplained. Knowing the characteristics which are related to the mobility differences between the two populations is important, because this information could be used to inform policy targeting educational advancement for one or the other groups specifically.
The results of our analysis confirm that in many European countries, migrants are more likely to be upwardly mobile (and less likely to be downwardly mobile) than natives.Footnote3 We find that differences in the socioeconomic background between natives and immigrants explain a large part of the difference in mobility patterns. In particular, the weaker educational attainment of migrants’ parents – and the lower threshold for upward mobility it implies – is a central factor in why second-generation migrants appear to be more upwardly mobile in many countries. Once the differences in parental education are taken into account, second-generation migrants remain significantly more likely to be upwardly mobile only in Switzerland and in Luxembourg (while in the Czech Republic the opposite applies). In these three countries, the mobility gap is mainly driven by the size of the household when the respondents were 14 years old, as well as the age structure of second-generation migrants. Focusing on the extent of upward mobility, we find that second-generation migrants are more likely than natives’ children to surpass the education level of their parents by two education classes only in the United Kingdom, while in all other countries the differences are statistically insignificant.
This article is structured as follows. Section II summarizes the data sources and provides descriptive statistics on education levels of educational mobility. Section III introduces the methodologies used in this article and summarizes the results of the multivariate analyses. Section IV concludes the article.
II. Data
We use data from the 2011 European Union Statistics on Income and Living Conditions (EU-SILC), which include information on each respondents’ parents, household circumstances when the respondents were 14, and standard demographic and socioeconomic information for respondents aged 25–59 in 2011.Footnote4 Upward (downward) mobility is a dummy variable equal to one if the respondent achieves a higher (lower) education level than their most highly educated parent, where education is measured in four categories (illiterate; ISCED 0–2; ISCED 3–4; ISCED 5–6).Footnote5 We study the sub-population of respondents who have completed their education and who were born in the country of residence and form two groups: natives (those with at least one parent born in the same country as the respondent) and migrants’ descendants, or second-generation migrants (those whose parents were born in another country).Footnote6
A summary of the educational attainment of respondents and their parents is provided in .Footnote7 Native-born parents are more likely to have tertiary education than the migrants in all countries but Austria, Switzerland, Estonia and the United Kingdom, and are less likely to be illiterate in all countries but Latvia and Germany.Footnote8 Comparing the educational attainment of migrants and natives over generations, we can observe a decrease in the achievement gap between the two groups. In the parental generation, migrants were more likely than natives to be illiterate in all but two countries, but the children of migrants and natives are about equally (un)likely to be illiterate. Further, in Switzerland, Croatia and the United Kingdom, migrants’ children are even more likely to have a tertiary education than natives’ children, and in more than half of the countries (6 of 11) migrants’ children are more likely to have completed upper secondary education (ISCED 3–4). Thus, we begin to see evidence of greater educational mobility for descendants of migrants in many of the countries in our sample.
Taking a closer look at upward mobility, shows the proportion of natives’ and migrants’ descendants, which is upwardly mobile for each country in our sample, given the highest education level of their parents. In all countries but Estonia and Latvia, a greater share of migrants’ children than natives’ children are in a higher education class than their parents, in total. Descendants of migrants with lower secondary education or less (ISCED 0–2) show higher mobility than native descendants with lower-secondary educated parents in all countries but Austria, the Czech Republic and Estonia. In most countries, children of low-educated migrants seem to be upwardly mobile more often than children of low-educated natives. In the former two countries where this is not the case (Austria and the Czech Republic), however, children of more highly educated parents with a migration background (with upper- or post-secondary education, ISCED 3–4) are more often upwardly mobile than those of natives. Indeed, children of migrants with upper- or post-secondary education are at least as mobile as descendants of similarly educated natives in half of the countries in our sample (Austria, Switzerland, the Czech Republic, France, Croatia and the United Kingdom).
Table 1. Education level by migration background.
Table 2. Upward mobility by parental education level and in total.
In what follows we examine how robust these differences are, once we compare descendants of migrants and natives from otherwise similar households, and study which characteristics drive the differences in mobility across migration background. To explore these issues, we turn to a multivariate analysis predicting mobility in section ‘Logit estimation’, and then decompose the detected mobility differences in section ‘Oaxaca–Blinder decomposition’.
III. Empirical analysis
Logit estimations
Taking the insights gained in the descriptive exploration of the data above, namely that children of the often lower educated group of migrants appear to be more mobile than their native counterparts in many countries, we move to a more structural analysis of these patterns and their causes. In a first step, using logit models, we investigate whether second-generation migrants are significantly more often mobile than natives once accounting for respondents’ socioeconomic backgrounds.
We use Bayesian estimation techniques throughout the article.Footnote9
Bayesian methods rely on Bayes’ rule, which is applied to learn about the parameters, , given the data,
where the posterior density is proportional to the likelihood function times the prior density.Footnote10
The posterior mean for variable , is calculated as
where S is the number of draws from the posterior. p-Values for each parameter, , can be derived either as
where is the distribution function of the Student’s t-distribution with
degrees of freedomFootnote11
and
or alternatively, we can obtain the p-values numerically as
where is an indicator function.Footnote12
Logit results
The results of the (Bayesian) logit estimations that explain educational upward mobility are reported in . In a first, naïve approach, we account for a respondent’s migration background by including a dummy variable that is equal to one if the respondent’s parents are born in another country as the single regressor. The results are reported in the upper panel of the table. The results suggest that migrants’ descendants have a significantly higher probability of being upwardly mobile than their native counterparts in seven countries (Belgium, Switzerland, Germany, France, Croatia, Luxembourg and the United Kingdom). Only in Latvia are migrants’ children less likely to be upwardly mobile than natives’ children. For Austria, the Czech Republic and Estonia, we do not detect a statistically significant difference between the mobility rates of the migrant and native samples.
Table 3. Logit results for pooled sample.
Next, in order to investigate whether the detected difference in upward mobility between the two groups is accounted for by a person’s socioeconomic background, we control for individual, parental and household characteristics, as well as potential cohort effects. In particular, we consider the respondents’ birth year and gender; their parents’ characteristics (parents’ age at childbirth and its square, parental age difference and an indicator of whether the mother was out of labour force when the respondent was 14); parental education (education levels of both parents and of the more highly educated parent)Footnote13 ; household characteristics at age 14 (number of adults and children in the household); and the household financial situation at age 14 (financial situation of the household and its interaction with the highest parental education level). We add two dummy variables equal to one if a respondent was born in the 50s or 60s, respectively, with the 70s and 80s being the base category.Footnote14 The results are reported in the lower panel of .
Once we control for a person’s socioeconomic background, the mobility gap between natives’ and migrants’ descendants turns insignificant everywhere but in Latvia and the United Kingdom. Thus, we find that in most countries, individual, parental and household characteristics are important drivers of the observed mobility patterns. While the sign of the effects of birth year, cohort and gender on the probability of being upwardly mobile is country-specific, an older age of the mother at childbirth has a significantly positive effect on the probability of her children reaching a higher education than their parents, however with decreasing rate (as indicated by the negative effect of the squared term). A similar pattern is visible for the father’s age at childbirth, however in a smaller number of countries and to a quantitatively lesser extent. The effect of a larger age difference between the respondent’s parents is consistently negative, whenever it is statistically significant. The mother’s labour market status when the respondent was 14 years old plays a significant role in Belgium and the Czech Republic – being out of the labour force contributes to a lower probability of upward mobility in these countries. Very striking is the positive and statistically significant effect of a higher education level of both the mother and the father, which is in line with the findings of the large literature that finds that a higher parental education has a positive impact on the education level of children. The education level of the more highly educated parent, which picks up the education threshold effect, has a negative coefficient, as expected. Furthermore, a bigger household size when the respondent was 14 years old is significantly negatively related to educational upward mobility in most countries, as evidenced by the negative parameters for the number of children and the number of adults in the household. Finally, a better financial situation of the household when the respondent was young is connected to a higher educational upward mobility. This relationship becomes smaller, though, as the education level of the more highly educated parent increases.Footnote15
In order to obtain a more conclusive picture of why the observed pattern in emerges and the difference in upward mobility between migrants’ and natives’ children turns insignificant once controlling for their socioeconomic background, we go one step further and try to shed light on which factors are responsible for explaining the higher upward mobility of migrants’ descendants in most countries (and their lower mobility in Latvia, respectively).
Oaxaca–Blinder decomposition
A prominent means for finding out which characteristics explain the observed differences between two groups, which received great attention especially in the labour literature, is the use of Oaxaca–Blinder decompositions (Blinder Citation1973; Oaxaca Citation1973). The decomposition allows researchers to attribute that part of the mobility gap which can be explained by observable characteristics to individual variables (or variable groups) and provide a clearer picture of the mechanisms at work. It also allows for the identification of that part of the gap which stems from unobserved factors or differences in returns to characteristics between the two groups.
For performing the Oaxaca–Blinder decompositions of educational mobility, we first estimate country-specific logit models for migrants’ and natives’ descendants separately. Introducing notation, the logit model can be written as
Equation (5) links the probability of educational upward mobility with respect to the most highly educated parent (i.e. ) to the covariates
, where
is the vector of posterior means (from Equation (2)), and
is the distribution function of the logistic distribution.
Standard references for the Oaxaca–Blinder decomposition for nonlinear models are Yun (Citation2004) and Fairlie (Citation2005), who suggest decomposing the mobility gap between migrants, , and natives,
, (the base group) into
where and
is an individual. To find the contribution of covariate
to the difference in characteristics,
(i.e. differences in upward mobility that can be explained by differences in observable characteristics between natives’ and migrants’ descendants), we follow Kaiser (Citation2015), who shows that the detailed decomposition can be derived asFootnote16
To overcome the identification problem present in evaluating the effect of dummy variables in the detailed decomposition (see Yun Citation2005), we include dummy variables only in variable groups (e.g. we report the effect of all cohort dummies together rather than reporting the effect of each cohort separately).
Decomposition results
Upward mobility
shows the results of this decomposition analysis for the probability of respondents of obtaining a higher education level than their more highly educated parent. The upper panel of the table reports the upward mobility of second-generation migrants and natives and the difference in mobility between the two groups (mobility gap). It also shows how much of this gap can be explained by differences in observable characteristics between the groups (explained part) and which part remains unexplained. The lower panel of the table shows the contribution of individual variable groups to the part of the mobility gap that stems from observable characteristics.Footnote17 Specifically, the variable groups comprise birth cohort (including birth year and cohort dummies); gender; parents’ characteristics (parents’ age at birth, its square, parental age difference and the mother’s labour market status); education (education level of both parents and of the more highly educated parent); household characteristics (number of adults and children in the household when the respondent was 14); and finance (financial situation of the household when the respondent was 14 and its interaction with the highest parental education level). Apart from reporting the contribution of each variable group to the mobility gap and the associated level of statistical significance, the upper panel of the table also summarizes the share of second-generation migrants and natives whose more highly educated parent did not yet reach the highest education level (see the percentages in square brackets).
Table 4. Decomposition results for upward mobility.
Table 5. Decomposition results for downward mobility.
Table 6. Decomposition results for upward mobility conditioned on parental education.
Table 7. Decomposition results for upward mobility of two education levels conditioned on parental education.
The results of the decomposition indicate that the greater probability of migrants’ children of reaching a higher education class than their parents is statistically significant in seven of 11 countries (Belgium, Switzerland, Germany, France, Croatia, Luxembourg and the United Kingdom). Only in Latvia, migrants’ descendants are significantly less likely to be upwardly mobile than their native counterparts. The contribution of the difference in observable characteristics between immigrants’ and natives’ children (the explained part) is highly statistically significant in all of these countries, while the contribution of differences in returns to characteristics (the unexplained part) exhibits much lower significance levels or is even statistically insignificant (with the exception of Latvia and the United Kingdom, where significance levels are higher than 5%).Footnote18
In the majority of countries, the difference in mobility between immigrants’ and natives’ children can in large part be explained by the difference in parental education between the groups. Whenever migrants are more often (less often) upwardly mobile, their parents’ education significantly contributes to this higher (lower) mobility. To analyse these patterns in more detail, we can compare them with the results of the logit regressions for natives (which form the base group for the decomposition), reported in in Appendix B.Footnote19 Greater education for the mother and the father is related to higher upward mobility of their offspring (see the positive effects of the mother’s and the father’s education). A higher threshold set by the education level of the most highly educated parent, however, decreases the probability of a person being upwardly mobile (see the negative effects of the highest parental education). Quantitatively the negative effect of the education threshold is larger than the positive effect of parental education and, thus, it outweighs the latter. Because in most countries in our sample (except for the Baltic states) migrant parents are on average less highly educated than their native counterparts, the lower threshold that this implies for upward mobility is an important contributor to the higher mobility of migrants’ children as compared to those of natives in these countries, which is in line with other literature (Van Ours and Veenman Citation2003). For the Baltic states, in which native parents have on average lower education levels than migrants, the opposite applies (see descriptive statistics in in Appendix A). In most countries in our sample, parental education is also quantitatively the largest contributor to the mobility gap between natives’ and migrants’ children.
Household characteristics make the biggest difference in the probability of upward mobility for migrants’ and natives’ descendants in Estonia and France. Their effect is statistically significant also for most other countries in our sample. In particular, living in relatively large households at age 14 (especially with many other children) shrinks the higher probability of a person being upwardly mobile. The larger household sizes of migrant households as compared to natives in Belgium, the Czech Republic, France, Croatia and the United Kingdom make it more difficult for migrants’ descendants to be upwardly mobile (as in, for example, Lindahl Citation2008). The opposite is true in Austria, Switzerland and the Baltic countries, where in our sample household sizes of migrants are on average smaller than those of natives, which is related to greater mobility for the migrants.
In Latvia, it is differences in the gender composition between the groups that plays the biggest role in explaining the mobility gap observed in the data. In this country (like in Estonia and Belgium), women are more likely than men to have reached a higher education level than their parents. The lower proportion of women among second-generation migrants in comparison to the sample of native respondents contributes to the overall lower mobility of second-generation migrants in those countries. In all other countries in our sample, male respondents are more likely to be upwardly mobile. Thus, the higher proportion of men (women) among natives’ descendants in Austria, Switzerland, Czech Republic, Germany and Croatia (France and Luxembourg) explains the observed mobility patterns.
The birth cohort of the respondents and their age contributes to a higher upward mobility of migrants’ children in Belgium, France, Luxembourg, the United Kingdom and the Baltic countries, and to lower mobility in the Czech Republic. In the Czech Republic, a higher probability of being upwardly mobile of younger persons, together with the on average older age of second-generation migrants makes it more difficult for them to be upwardly mobile. By contrast, in Belgium, France, Luxembourg and the United Kingdom, migrants’ children are on average younger than their native peers (they are born in later birth-cohorts). The higher mobility of younger individuals in those countries adds to the higher probability of being upwardly mobile for migrants’ descendants.Footnote20 In the Baltic countries, individuals who are born in the 50s and 60s are more likely to be upwardly mobile than persons born in later cohorts. Thus, the on average older age of migrants’ descendants in those countries explains the positive contribution to them being upwardly mobile.
Parental characteristics contribute to the higher mobility of migrants’ descendants positively in Germany, Croatia and Latvia, and negatively in Belgium, the Czech Republic and Luxembourg. Which characteristics drive this pattern in each specific case can be analysed in more detail by consulting the results of the detailed decomposition reported in in Appendix B. Often the mother’s age, her labour market status and the parental age difference are important drivers of the negative effects, while for the positive effects a large part stems from the parental age difference and the father’s age. Finally, the financial situation of the household usually remains an insignificant contributor to the mobility gap (with the exception of the United Kingdom, where it contributes to a higher upward mobility of second-generation migrants).
Turning to the unexplained part of the mobility differences, we see that this part is statistically significant (although only at the 10% level) and positively relates to higher mobility for migrants in five countries in our sample (Belgium, Switzerland, France, Luxembourg and the United Kingdom). Chiswick (Citation1978) and Borjas (Citation1994) raise the idea that persons who are more motivated to succeed might select themselves into migration. Such selection, and the higher motivation of persons with a migration background that it implies, would be reflected in a positive unexplained component of the mobility gap. Only in Latvia is the unexplained part statistically significant and negative, which could be driven by return migration of more able second-generation migrants.Footnote21
All in all, parental education is the most influential factor in explaining mobility differences between natives’ and migrants’ children in most countries. Whenever migrants’ children are upwardly mobile more often than natives’, it is in large part because their parents started out with relatively low education levels. The literature shows that children’s success in school depends largely on their parents’ education levels, which are in turn related to the levels of human, social and financial capitals from which the children can benefit (Haveman and Wolfe Citation1995). Thus, although empirical studies often find that migrants’ academic success is lower than natives’ (Riphahn Citation2003; Colding Citation2006; Schneeweis Citation2011), we show that the children of migrants in the last generation, who grew up in homes with fewer resources than their native peers in many countries, were still able to narrow the education gap with natives’ children.
Downward mobility
In order to draw a more comprehensive picture of whether the patterns found so far imply that the education gap between natives and second-generation migrants is narrowing, we repeat our analysis from above focusing on the probability of migrants’ and natives’ descendants reaching a lower education level than their more highly educated parent. If migrants’ children are more likely to be downwardly mobile, the implication of a narrowing gap based on the fact that they are more likely to be upwardly mobile at the same time (as shown in section ‘Upward mobility’) would be nullified. presents the results of the Oaxaca–Blinder decomposition focusing on educational downward mobility.Footnote22
In Germany, France and Luxembourg, migrants’ descendants are significantly less likely to be downwardly mobile than their native peers, while in Estonia the opposite applies. Differences in observable characteristics between the children of natives and migrants are significant contributors to the mobility gap in all of these countries. Additionally to this explained part of the mobility gap, in Germany and Estonia also the unexplained part of the decomposition is statistically significant.
Concerning the contribution of different variable groups to the mobility gap explained by observable characteristics, we find that, as in the analysis of upward mobility, parental education is the largest contributor to this difference in most countries. In all countries in which the explained difference is negative (positive), the gap is driven in large part by differences in parental education between natives’ and migrants’ children. The more detailed decomposition results in in Appendix B show that this is again driven by the threshold effect of education, which suggests that the on average lower education level of migrant parents in most countries makes it less likely for their children to reach even lower education levels. For Switzerland and Latvia, the birth cohort of the respondent turns out to have the quantitatively largest impact on the explained part of the mobility difference. However, in these countries the overall difference in downward mobility between migrants’ children and their native peers is statistically insignificant.
Upward mobility conditioned on parental education
Our results thus far suggest that in most countries in which we detect a statistically significant difference in mobility, migrants’ children have an advantage: they are more often upwardly mobile and less often downwardly mobile than their native peers. The only exceptions are the Baltic countries where migrants are on average more highly educated than natives. We also uncovered that an important factor for explaining these mobility differences is the threshold implied by the education level of the more highly educated parent. This raises the question: are second-generation migrants upwardly mobile more often only because they have a lower threshold to surpass, or would they be more mobile also if their parents were equally educated as natives?
In order to perform this analysis, we restrict our sample to respondents whose more highly educated parents have the same highest education level (ISCED 0–2) and repeat the analysis from before on the restricted sample. In this way we compare mobility patterns of natives’ and migrants’ children that face the same education threshold to surpass.Footnote23 The results, which are reported in , suggest that once we restrict our sample to observations with the same parental education, migrants’ children are significantly less often upwardly mobile in the Czech Republic, and significantly more often upwardly mobile in Switzerland and Luxembourg. In all three countries, differences in observable characteristics between the groups are statistically significant contributors to the overall mobility gap. In the Czech Republic and Luxembourg also unexplained factors are marginally statistically significant.
Turning to the contribution of the different variable groups to the mobility gap explained by differences in observable characteristics, birth cohorts account for the largest part in the majority of countries. In Switzerland and the Czech Republic, it is household size (especially the number of children in a household, see detailed results in in Appendix B) that contribute the largest part to the mobility gaps, and in Estonia it is differences in the financial situation of households when the respondent was 14 years old.
Two classes upward mobility conditioned on parental education
Finally, we shortly compare the probability of migrants’ and natives’ descendants of surpassing their more highly educated parent by two education classes. The idea behind this analysis is that if migrants are also more likely to jump two classes, they are even more likely to narrow the education gap with natives.Footnote24 shows that in the United Kingdom, migrants’ children are significantly more likely than natives’ to surpass their more highly educated parent by two education classes.Footnote25 This mobility difference cannot be explained by observable characteristics but is driven by unobservable factors. In all other countries in our sample, the mobility gap between natives’ and migrants’ descendants is statistically insignificant. However, there is some indication that in Belgium and France observable characteristics (mainly larger household sizes) would explain a lower (two-class) upward mobility of second-generation migrants, while in Switzerland, Germany, Estonia and Luxembourg the opposite is true (mainly explained by household size in Switzerland and Estonia, parental characteristics in Germany and birth cohorts in Luxembourg).
All in all, our results suggest that the higher educational upward mobility of second-generation migrants that has been found in many European countries can be, to a large extent, explained by the lower threshold implied by the on average lower education level of their parents (as in Belgium, Switzerland, Germany, France, Croatia, Luxembourg and the United Kingdom). Parental education also explains the lower downward mobility of migrants’ children that is detected in Germany, France and Luxembourg. Once we restrict our sample to persons that face a similar education threshold, the significant difference in mobility patterns disappears in many countries. Second-generation migrants are then more often upwardly mobile only in Switzerland and Luxembourg, and surpass their parents by two education levels more often than natives only in the United Kingdom. On the other hand, we detect a higher upward mobility of natives in Latvia and a lower downward mobility of natives in Estonia, countries in which migrant parents are on average more highly educated than native parents. Thus, a similar catch-up process of natives seems to take place in those countries. Once we restrict our sample to individuals with similar parental education, a higher upward mobility of natives is only detected in the Czech Republic.
Although data limitations impede the analysis of the patterns found here in further detail, this would be a promising direction for future research, which could focus on one specific country and dig deeper into what drives differences in educational mobility after accounting for parental education. In any case, the results here challenge the idea of more highly motivated migrants. Instead, the research shows that the often lower threshold that migrants’ children need to surpass in order to be upwardly mobile is the main driver of mobility differences to natives in most countries. Beyond the lower education of the parents, we find that some personal and household-level characteristics, in particular household size at age 14, also matters (though less so). Regardless of the reason, though, the education gap appears to have been closing in most countries across the last two generations.
IV. Conclusion and discussion
The analysis in this article provides clear evidence of a narrowing gap in educational attainment levels between natives and immigrants across the two most recent generations. Migrant parents are less educated than native parents in many European countries, but their children are often able to surpass their parents’ education levels and get closer to the level of education achieved by their native peers. In most countries, the socioeconomic background of native and migrant parents is an important determinant of the mobility differences between their children. Apart from parental education, which is the quantitatively most important contributor to the mobility difference in most countries, the size of the household when the respondent was 14 turns out to be an important driver of differences in upward mobility between the two groups in most of the countries in our sample, while the financial situation of the household at that time is more important for explaining patterns of downward mobility. In many countries, the age structure and the gender composition of the two groups also contributes to the observed mobility differences, while parental characteristics are statistically insignificant drivers of mobility gaps in about half of the countries analysed.
In order to see the extent to which second-generation migrants’ greater educational mobility is driven by the lower threshold that they have to pass (because of the on average lower educational attainment levels of their parents in many countries), we provide additional information on the mobility difference between the children of similarly educated natives and migrants. Evidence points towards a higher educational upward mobility of migrants’ children in Switzerland and Luxembourg, whereas their mobility is significantly lower than that of their native peers in the Czech Republic.
The analysis presented in this article suggests that in most countries in our sample, the difference in educational attainment between natives and second-generation migrants has been narrowing across the two most recent generations. If this process persists over future generations, people with a migration background might soon have comparable education levels to the native population.
The findings in this article have important implications for the design of immigration policy, which often focuses on the on-average lower education level of immigrants into western European countries. Empirical studies often find a higher use of social welfare assistance of lower educated individuals (see Barrett and McCarthy Citation2007; Blume and Verner Citation2007; Boeri Citation2010; Pellizzari Citation2013; Huber and Oberdabernig Citation2016), which spurs arguments against the inflow of unskilled immigrants. Although the effects of education on welfare receipt are usually small and many studies find an only small fiscal impact of immigration as a share of gross domestic product in most countries, unskilled immigration remains a concern (see OECD Citation2013, and studies cited therein). Reinforcing the argument that descendants of immigrants are likely to provide a strong positive fiscal contribution to the state budget (see Lee and Miller Citation1997; cited by OECD Citation2013), our study shows that if the patterns detected for the last two generations continue, the potentially negative effect of migrants’ lower education on their net fiscal position eventually dies out in the long run, which would lead to similar conclusions.
Further, to the extent to which policy makers want to speed up the convergence of migrants’ to natives’ education levels over generations, they could consider policies which have been shown to reduce intergenerational persistence in educational outcomes, such as later tracking, early school entry and greater access to pre-school and kindergarten programmes (Bauer and Riphahn Citation2006a; Schütz, Ursprung, and Wößmann Citation2008; Bauer and Riphahn Citation2009a). These policies could be particularly helpful to migrants’ descendants, given that their parents are often less educated than native-born parents.
intergenerational_persistence_migrants_appendix_tables.pdf
Download PDF (419.5 KB)Acknowledgements
The authors are grateful for helpful comments from Octavio Fernández-Amador, Stefan Humer, participants of the XIX Applied Economics Meeting and two anonymous reviewers, and acknowledge support from the NCCR trade regulation, University of Bern. A. Schneebaum gratefully acknowledges funding for this research from the Austrian Science Fund (FWF) under grant number T 714-G11. Sophie Augustin provided excellent research assistance.
Disclosure statement
No potential conflict of interest was reported by the authors.
Supplementary data
Supplemental data for this article can be accessed here.
Additional information
Funding
Notes
1 A large literature finds educational attainment to be strongly related to the socioeconomic background of an individual’s parents (see Becker and Tomes Citation1979, Citation1986; for theoretical foundations, Haveman and Wolfe Citation1995; for a review of the foundational empirical literature, and Dustmann, Rajah, and Soest Citation2003; Bauer and Riphahn Citation2007; Chevalier et al. Citation2013; for some recent studies on European countries).
2 Also relevant in this literature are the findings from Fredriksson and Öckert (Citation2005) and Puhani and Weber (Citation2007), who show that school entry at an older age is correlated with greater academic achievement – although these findings may be related to the fact that older children are better performers because they are older and thus have had more time to accumulate skills. Elder and Lubotsky (Citation2009) also measure student achievement and point to the importance of family background in academic success.
3 An exception are the Baltic countries, where migrant parents are more highly educated on average than native parents.
4 We base our analysis on EU-SILC data rather than on data from alternative sources (e.g. the European Social Survey, ESS; the Generations and Gender Survey, GGS; the Survey of Adult Skills, PIAAC; and the European Values Survey, EVS), because of the availability of more detailed information on educational attainment (in comparison to PIAAC), the availability of information on a person’s socioeconomic background (especially parental characteristics and information on household composition when the respondent was 14 years old), which is not available to the same extent in some other databases (in ESS, PIAAC), the larger coverage of European countries (in comparison to GGS), or the larger sample size (in comparison to EVS). Still, our sample size for second-generation migrations is quite small in some countries. Thus, we rely on Bayesian estimation methods throughout the article (see section ‘Logit estimations’).
5 ISCED 0–2 corresponds to pre-primary through lower-secondary education, ISCED 3–4 to upper secondary through post-secondary non-tertiary education and ISCED 5–6 to tertiary education.
6 We compare persons with at least one parent born in their country of residence to persons for whom this is not the case, assuming that the native born parent is able to help their child at school in a similar way as parents who are both native born. We exclude persons who did not yet finish their education and persons with missing information on their parents or missing values for any explanatory variable from the sample. For the analysis of upward mobility, we exclude respondents whose more highly educated parent has reached the highest education class because these persons can per definition not be upwardly mobile. Similarly, we exclude persons whose parents have the lowest education class in the analysis of downward mobility. Information on which share of observations is dropped in each step is available in in Appendix A. The exclusion of observations due to missing information on parental characteristics or explanatory variables could lead to selection bias, which would arise if observations are non-randomly missing across the two groups. While we have no means to check the direction of potential bias for missing information on parental characteristics (such as education or country of birth), in Appendix A provides an overview of the mobility differences between natives and migrants for observations that have been excluded because of missing information on explanatory variables. (and Table E.2 in the online appendix) show the impact of different possibilities of observations being dropped due to missing observations.
7 Descriptive statistics for the control variables that are used in the multivariate analysis for the full sample can be found in in Appendix A. provides an overview of the definitions of the variables.
8 Treating education levels as a cardinal scale, native parents are more educated than migrant parents on average in all countries but Estonia and Latvia, as indicated by the column labelled ‘average education’. The Baltic countries are likely to be different from the other countries included in our analysis because they regained independence from the Soviet Union only in the early 1990s. Thus, immigration into these countries was to a big part internal.
9 We prefer Bayesian techniques to frequentist analyses because inference based on the latter is often complicated in small samples. For more information on Bayesian techniques see Koop (Citation2003).
10 For prior information we use three distinct specifications. One is an uninformative prior with mean and precision equal to 0. The other two prior specifications use information obtained from frequentist logit regressions. More specifically, we estimate logit regressions on the pooled samples of natives and second-generation migrants for each country, using the resulting parameter estimates as prior means and the inverse of the standard errors obtained from the frequentist estimations divided by and
, respectively, as prior precision. We rely on Gibbs sampling and run three Markov chains, one for each definition of the prior density, for which convergence is evaluated using Gelman and Rubin’s (Citation1992) convergence diagnostic (the diagnostics can be found in the supplementary material (online appendix). Inference is based on a combination of the three chains, leading to a random sample from the posterior of
draws. Our estimations are conducted in R using the package ‘BayesLogit’ (see Polson, Scott, and Windle Citation2013; Polson and Scott Citation2011). For each model we run three Markov chains with 20,000 iterations each, after a burn-in of 5000 and a thinning of 100, resulting in
.
11 We adjust for potential autocorrelation in the Markov chain.
12 That is, counting the share of draws for which is equally signed as its posterior mean
.
13 With including the education level of the more highly educated parent additionally, we account for the fact that if the parents already have a high education level it is harder for a person to surpass this level than if the parents have a very low education level. We expect the coefficient of this education threshold effect to be negative.
14 The respondents in our sample are all born between 1951 and 1986 (around 31% in the 50s, 34% in the 60s and 36% in the 70s or 80s).
15 This finding suggests that offspring of financially well-endowed parents with lower education levels are especially eager to obtain a higher education level, while this might not be true to the same extent for persons with relatively highly educated parents. Luxembourg is an exception, as especially children of highly educated parents with a good financial situation are more often upwardly mobile.
16 Yun (Citation2004) proposes an alternative methodology to isolate the contribution of each covariate to the aggregate decomposition, which is based on the evaluation of covariates at their means. We follow the methodology in Kaiser (Citation2015) because it allows us to take into account the nonlinear nature of logit models by accounting for differences also in higher order moments of X.
17 To keep the interpretation simple, we form groups of individual variables and report the contribution of each group to the part of the mobility gap that is based on the difference in observable characteristics. The results of the detailed decomposition, which summarizes the contribution of each individual variable, are reported in in Appendix B.
18 The results reported in the table should be interpreted as in the following examples. In Belgium, migrants’ children are 9.4 percentage points more likely than natives’ children to surpass their parents’ education level. Differences in characteristics between natives and second-generation migrants explain 3 percentage points (about 40%) of this mobility gap. Due to unobserved factors, which explain the remaining part of the gap, migrants’ children have a 6.3 percentage points higher probability of being upwardly mobile. In Latvia, by contrast, migrants’ children are 5.7 percentage points less likely than their native peers to surpass their parents in terms of education. While differences in characteristics between migrants’ and natives’ children account for a 1.2 percentage point lower probability of upward mobility, it is unobserved factors that drive the bigger part of the lower mobility of second-generation migrants (4.5 percentage points or about 80% of the mobility gap). The same interpretation applies to the results for individual variables or variable groups.
19 The results for second-generation migrants are reported in in Appendix B.
20 More specifically, it is the younger age of second-generation migrants in France and the United Kingdom that explains their higher mobility, while in Belgium cohort effects have a larger impact. In Luxembourg, it is a combination of both effects.
21 Hazans, Trapeznikova, and Rastrigina (Citation2008) point out that between 1991 and 2002, 17% of the minority population in Latvia left the country. If ability selection lead to the emigration of more able persons, as suggested by Chiswick (Citation1978) and Borjas (Citation1994), this might explain the negative unexplained part for Latvia. An alternative explanation might be the transition from central planning to a market economy after the dissolution of the Soviet Union and its resulting effects on the education system. During the transition, the Latvian education system stopped offering courses in Russian for the large proportion of Russian-speaking minorities (42% in 2002) to replacing them with courses in the national language. At the same time, returns to education increased and access constraints to higher education were removed, resulting in higher demand for tertiary education among the native population. The two effects together have potentially contributed to a falling ratio of tertiary graduation rates between the minority and native population (see Hazans, Trapeznikova, and Rastrigina Citation2008, for details). We leave an analysis of which of the two potential explanations account for the observed pattern in Latvia to future research.
22 Because of the small number of downwardly mobile second-generation migrants in Belgium, the Czech Republic and the United Kingdom (3, 1 and 9, respectively) we had to exclude these countries from this analysis. Descriptive statistics for this subsample (and also for the following analyses) and a summary of the results from the logit regressions can be found in the online appendix.
23 We had to exclude Austria, Germany and the United Kingdom from the analysis because of the small sample size for migrants (45 in Austria) or small number of migrants’ children who are not upwardly mobile (four and three cases in Germany and the United Kingdom, respectively). We exclude all parental education variables and its interaction with the financial situation from the regressions because of the multicollinearity induced by conditioning on the same parental education level.
24 Here too we restrict our sample to persons whose more highly educated parent reached an education level corresponding to ISCED 0–2. Austria, the Czech Republic and Croatia had to be excluded from the analysis because of the small number of migrants’ descendants who surpass their more highly educated parent by two education levels (1, 7 and 8, respectively).
25 The results of the detailed decomposition are reported in in Appendix B.
References
- Aydemir, A., W.-H. Chen, and M. Corak. 2013. “Intergenerational Education Mobility among the Children of Canadian Immigrants.” Canadian Public Policy 39: S107–S122. doi:10.3138/CPP.39.Supplement1.S107.
- Barrett, A., and Y. McCarthy. 2007. “Immigrants in a Booming Economy: Analysing Their Earnings and Welfare Dependence.” Labour 21 (4–5): 789–808. doi:10.1111/labr.2007.21.issue-4-5.
- Bauer, P. C., and R. T. Riphahn. 2006a. “Timing of School Tracking as a Determinant of Intergenerational Transmission of Education.” Economics Letters 91: 90–97. doi:10.1016/j.econlet.2005.11.003.
- Bauer, P. C., and R. T. Riphahn. 2006b. “Education and Its Intergenerational Transmission: Country of Origin-Specific Evidence for Natives and Immigrants from Switzerland.” Portuguese Economic Journal 5: 89–110. doi:10.1007/s10258-006-0011-8.
- Bauer, P. C., and R. T. Riphahn. 2007. “Heterogeneity in the Intergenerational Transmission of Educational Attainment: Evidence from Switzerland on Natives and Second-Generation Immigrants.” Journal of Population Economics 20: 121–148. doi:10.1007/s00148-005-0056-5.
- Bauer, P. C., and R. T. Riphahn. 2009a. “Age at School Entry and Intergenerational Educational Mobility.” Economics Letters 103: 87–90. doi:10.1016/j.econlet.2009.01.032.
- Bauer, P. C., and R. T. Riphahn 2009b. “Kindergarten Enrollment and the Intergenerational Transmission of Education.” Technical Report No. 4466, IZA Working Paper.
- Bauer, P. C., and R. T. Riphahn. 2013. “Institutional Determinants of Intergenerational Education Transmission — Comparing Alternative Mechanisms for Natives and Immigrants.” Labour Economics 25: 110–122. doi:10.1016/j.labeco.2013.04.005.
- Becker, G. S., and N. Tomes. 1979. “An Equilibrium Theory of the Distribution of Income and Intergenerational Mobility.” Journal of Political Economy 87: 1153–1189. doi:10.1086/260831.
- Becker, G. S., and N. Tomes. 1986. “Human Capital and the Rise and Fall of Families.” Journal of Labor Economics 4 (3, Part 2): S1–S39. doi:10.1086/298118.
- Blinder, A. 1973. “Wage Discrimination: Reduced Form and Structural Estimates.” The Journal of Human Resources 8 (4): 436–455. doi:10.2307/144855.
- Blume, K., and M. Verner. 2007. “Welfare Dependency among Danish Immigrants.” European Journal of Political Economy 23: 453–471. doi:10.1016/j.ejpoleco.2006.08.005.
- Boeri, T. 2010. “Immigration to the Land of Redistribution.” Economica 77: 651–687. doi:10.1111/j.1468-0335.2010.00859.x.
- Borjas, G. 1994. “The Economics of Immigrations.” Journal of Economic Literature 32 (2): 1667–1717.
- Borjas, G. J. 1987. “Self-Selection and the Earnings of Immigrants.” American Economic Review 77 (4): 531–553.
- Chevalier, A., C. Harmon, V. O’Sullivan, and I. Walker. 2013. “The Impact of Parental Income and Education on the Schooling of Their Children.” IZA Journal of Labor Economics 2 (1): 1–22. doi:10.1186/2193-8997-2-8.
- Chiswick, B. R. 1978. “The Effect of Americanization on the Earnings of Foreign-Born Men.” Journal of Political Economy 86 (5): 897–921. doi:10.1086/260717.
- Chiswick, B. R. 1999. “Are Immigrants Favorably Self-Selected?” American Economic Review: Papers and Proceedings 89 (2): 181–185. doi:10.1257/aer.89.2.181.
- Colding, B. 2006. “A Dynamic Analysis of Educational Progression of Children of Immigrants.” Labour Economics 13: 479–492. doi:10.1016/j.labeco.2006.02.005.
- Deming, D., and S. Dynarski 2008. “The Lengthening of Childhood.” Technical Report, NBER Working Paper.
- Dustmann, C. 2004. “Parental Background, Secondary School Track Choice, and Wages.” Oxford Economic Papers 56 (2): 209–230. doi:10.1093/oep/gpf048.
- Dustmann, C. 2008. “Return Migration, Investment in Children, and Intergenerational Mobility: Comparing Sons of Foreign- and Native-Born Fathers.” The Journal of Human Resources 43 (2): 299–324. doi:10.3368/jhr.43.2.299.
- Dustmann, C., T. Frattini, and G. Lanzara. 2012. “Educational Achievement of Second-Generation Immigrants: An International Comparison.” Economic Policy 27 (69): 143–185. doi:10.1111/ecop.2012.27.issue-69.
- Dustmann, C., N. Rajah, and A. Soest. 2003. “Class Size, Education, and Wages.” Economic Journal 113 (485): F99–F120. doi:10.1111/ecoj.2003.113.issue-485.
- Elder, T. E., and D. H. Lubotsky. 2009. “Kindergarten Entrance Age and Children’s Achievement: Impacts of State Policies, Family Background, and Peers.” Journal of Human Resources 44 (3): 641–683. doi:10.1353/jhr.2009.0015.
- Fairlie, R. 2005. “An Extension of the Blinder Oaxaca Decomposition to Logit and Tobit Models.” Journal of Economic and Social Measurement 30 (4): 305–316.
- Fredriksson, P., and B. Öckert 2005. “Is Early Learning Really More Productive? The Eff of School Starting Age on School and Labor Market Performance.” Technical Report.
- Gang, I. N., and K. F. Zimmermann. 2000. “Is Child like Parent? Educational Attainment and Ethnic Origin.” The Journal of Human Resources 35 (3): 550–569. doi:10.2307/146392.
- Gelman, A., and D. B. Rubin. 1992. “Inference from Iterative Simulation Using Multiple Sequences.” Statistical Science 7 (4): 457–472. doi:10.1214/ss/1177011136.
- Hanushek, E. A., and L. Wößmann. 2006. “Does Early Tracking Affect Educational In- Equality and Performance? Difference-In-Differences Evidence across Countries.” Economic Journal 116: C63–C76. doi:10.1111/j.1468-0297.2006.01076.x.
- Haveman, R., and B. Wolfe. 1995. “The Determinants of Children’s Attainments: A Review of Methods and Findings.” Journal of Economic Literature 33 (4): 1829–1878.
- Hazans, M., I. Trapeznikova, and O. Rastrigina. 2008. “Ethnic and Parental Effects on Schooling Outcomes before and during the Transition: Evidence from the Baltic Countries.” Journal of Population Economics 21: 719–749. doi:10.1007/s00148-007-0134-y.
- Huber, P., and D. A. Oberdabernig. 2016. “Decomposing Welfare Wedges: An Analysis of Welfare Dependence of Immigrants and Natives in Europe.” Kyklos 69 (1): 82–107. doi:10.1111/kykl.12104.
- Kaiser, B. 2015. “Detailed Decompositions in Nonlinear Models.” Applied Economics Letters 22 (1): 25–29. doi:10.1080/13504851.2014.907469.
- Koop, G. 2003. Bayesian Econometrics. West Sussex, England: John Wiley & Sons Ltd.
- Lee, R., and T. Miller. 1997. “The Lifetime Fiscal Impacts of Immigrants and Their Descendants”. In The New Americans - Economic, Demographic, and Fiscal Effects of Immigration, 297–362. Washington, DC: National Academy.
- Lindahl, L. 2008. “Do Birth Order and Family Size Matter for Intergenerational Income Mobility? Evidence from Sweden.” Applied Economics 40: 2239–2257. doi:10.1080/00036840600949421.
- Lüdemann, E., and G. Schwerdt. 2013. “Migration Background and Educational Tracking. Is There a Double Disadvantage for Second-Generation Immigrants?” Journal of Population Economics 26 (2): 455–481. doi:10.1007/s00148-012-0414-z.
- Oaxaca, R. 1973. “Male-Female Wage Differentials in Urban Labor Markets.” International Economic Review 14 (3): 693–709. doi:10.2307/2525981.
- OECD. 2013. International Migration Outlook 2013. Paris: OECD Publishing.
- Pellizzari, M. 2013. “The Use of Welfare by Migrants in Italy.” International Journal of Manpower 34 (2): 155–166. doi:10.1108/01437721311320672.
- Polson, N. G., and J. G. Scott 2011. “Default Bayesian Analysis for Multi-way Tables: A Data-augmentation Approach.” http://arxiv.org/pdf/1109.4180.pdf
- Polson, N. G., J. G. Scott, and J. Windle. 2013. “Bayesian Inference for Logistic Models Using Pólya–Gamma Latent Variables.” Journal of the American Statistical Association 108: 1339–1349. doi:10.1080/01621459.2013.829001.
- Puhani, P. A., and A. M. Weber. 2007. “Does the Early Bird Catch the Worm? Instrumental Variable Estimates of Early Educational Eff of Age of School Entry in Germany.” Empirical Economics 32: 359–386. doi:10.1007/s00181-006-0089-y.
- Riphahn, R. T. 2003. “Cohort Effects in the Educational Attainment of Second Generation Immigrants in Germany: An Analysis of Census Data.” Journal of Population Economics 16: 711–737. doi:10.1007/s00148-003-0146-1.
- Schneebaum, A., B. Rumplmaier, and W. Altzinger. 2016. “Gender and Migration Background in Intergenerational Educational Mobility.” Education Economics 24 (3): 239–260. doi:10.1080/09645292.2015.1006181.
- Schneeweis, N. 2011. “Educational Institutions and the Integration of Migrants.” Journal of Population Economics 24 (4): 1281–1308. doi:10.1007/s00148-009-0271-6.
- Schütz, G., H. W. Ursprung, and L. Wößmann. 2008. “Education Policy and Equality of Opportunity.” Kyklos 61 (2): 279–308. doi:10.1111/j.1467-6435.2008.00402.x.
- Van Ours, J. C., and J. Veenman. 2003. “The Educational Attainment of Second-Generation Immigrants in the Netherland.” Journal of Population Economics 16 (4): 739–753. doi:10.1007/s00148-003-0147-0.
- Wößmann, L. 2009. “International Evidence on School Tracking: A Review.” Technical Report, CESifo DICE Report.
- Yaman, F. 2014. “Ethnic Externalities in Education and Second-Generation Immigrants.” Applied Economics 46 (34): 4205–4217. doi:10.1080/00036846.2014.952893.
- Yun, M. 2005. “A Simple Solution to the Identification Problem in Detailed Wage Decompositions.” Economic Inquiry 43 (4): 766–772. doi:10.1093/ei/cbi053.
- Yun, M.-S. 2004. “Decomposing Differences in the First Moment.” Economics Letters 82: 275–280. doi:10.1016/j.econlet.2003.09.008.
Appendices
Appendix A. Data appendix
Descriptive statistics
Table A1. Descriptive statistics of all variables for the full sample.
Table A2. Descriptive statistics of all variables for the sample used for upward mobility.
Variable definitions
Table A3. Variable definitions.
Dropped observations
Table A4. Sample sizes.
Table A5. Mobility of dropped individuals due to missing values for explanatory variables.
Appendix B.
Estimation results
Table B1. Logit result for upward mobility of natives’ children.
Table B2. Logit results for upward mobility of migrants’ children.
Table B3. Detailed decomposition results for upward mobility.
Table B4. Detailed decomposition results for downward mobility.
Table B5. Detailed decomposition results for upward mobility conditioned on parental education.
Table B6. Detailed decomposition results for upward mobility of two education levels conditioned on parental education.