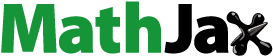
ABSTRACT
We investigate the impact of peer observation on consumption decisions using a lab-in-field experiment. Respondents make consumption decisions either alone or under peer observation. We find evidence for peer effects. We are able to study these further by looking into the mechanism and performing detailed heterogeneity analysis. Concerning the mechanisms, we find evidence for an information channel. Further, we show that the consumption choice is influenced by how many people made the same decision previously, but not by who those people are, hence finding evidence of a psychological channel. Respondents with higher cognitive ability are less susceptible to peer effects, while people living in small villages are more susceptible.
KEYWORDS:
I. Introduction
The feeling of buying something because someone else already has it, is a feeling familiar to many. Despite the familiarity of this feeling, there is still a lack of understanding on how peer effects in consumption work. So far most evidence comes from field experiments that study the effects of lottery wins (Kuhn et al. Citation2011), bankruptcy (Agrawal et al. Citation2016) or cash transfers (Angelucci and De Giorgi Citation2009) on the consumption level of people living close by. Less is known about the mechanisms behind peer effects, who is susceptible to peer effects or whether it matters who in a certain group makes a decision. A more detailed study of peer effects in consumption choices is not just crucial for further improving our understanding of individual decision-making, but it can also have an important effect on policy. Decision-makers interested in enhancing the uptake of certain goods, such as health services or innovative technology could use peer effects to increase general consumption thereof. For these reasons, it is important to further our understanding of peer effects and consumption choices.
Showing the existence of peer effects empirically and examining these further poses some challenges. Measuring the extent to which peers affect decision-making is not easy, because social group formation is usually endogenous, meaning that observed peer effects may be due to individuals in a group being more similar than other individuals, which complicates causal inference. At the same time, peers often experience the same events or shocks, causing them to behave in a similar way (Manski Citation1993). Hence, identifying peer effects based on most observational data is challenging.
We here perform a novel lab-in-the-field experiment to study peer effects in consumption choices in rural Thai villages. We perform this experiment with 552 individuals that live in 66 villages. The experiment is conducted in rural Thailand because of the prevalence of close knit communities. In other words, eventhough assignment to a group is random; groups are made up of people that are familiar with each other since they live in the same village, and often have done so for many generations (Mangyo and Park Citation2011). This allows us to study peer effects in a more natural environment, thus, enhancing external validity.
In addition, we are able to combine our lab-in-field experiment with a very extensive household survey that has been running for a number of years. This means that we have a large amount of information about our respondents, their household, and the village in which they live. Having this large amount of information about the respondents not only helps us to identify peer effects, but it also enables us to look deeper into the measured effects that often could not be examined in other studies. We are further able to answer some questions on the mechanism behind peer effects and look closer at who is susceptible to peer effects. To the best of our knowledge, no lab-in-the-field experiment of this form, studying the effect of peers on consumption, has been performed.
The design of our experiment is straightforward: we test consumption choices by simply offering respondents the choice between a combination of sweet and savory snacks, i.e. the temptation good (called the tasty treat or TT from here on) or money across seven rounds. Temptation goods are defined as goods that provide the current self with positive utility, but negative utility to any future selfFootnote1. The amount of money offered increases by ten Baht in every round, while the TT remains the same. In the control group, respondents have to make their consumption choices on their own, isolated from the rest of the respondents. In the treatment group, each respondent still makes his/her own decision, but all respondents observe each other and are able to communicate. Hence, the only difference between treatment and control group is the possibility of observing the other participants and so any difference in outcome can be attributed to peer observation.
We focus in particular on the effect of peer observation on temptation goods, since consumption choices for temptation goods are particularly susceptible to the influence of peer effects, as shown for alcohol consumption in social psychology (Gunter and Furnham Citation1998; Steinberg and Cauffman Citation1996).
In this paper, we aim to contribute to the literature on peer effects and consumption on the following way. (i) We identify the existence of peer effects in terms of consumption decisions using a novel experiment and a sample for which peer effects have not been studied previously; (ii) we investigate some of the mechanisms through which peer effects operate; and (iii) test whether there are differences in the susceptibility to peer effects.
Our results are the following: We find that observing groups – those that sit in close proximity to each other – have a higher group minimum and a lower group maximum. Consequentially, the standard deviation for observing groups is lower than for groups without peer observation. Hence, we show the presence of peer effects. Interestingly, there is no difference in the groups’ mean choices.
In further analyses, we confirm this finding by showing that the group average, excluding the individual him/herself, has a positive and significant influence on the decision made by the individual respondent. Most importantly, the effect is not significant when the experiment is performed in non-observing groups.
Next, we aim to explore the mechanisms behind this effect. There are two possible mechanism for this; either the respondents believes that others in the group have better information or they are gaining some kind of psychological benefit from conforming to others. Although definite answers are not possible, we find evidence that unfamiliarity with the tasty treat is counteracted by peer observations – indicating some evidence for the first mechanism. However, it has to be made clear that since neither familiarity with the tasty treat nor desire for conformity are controlled by the experimentor, this can not be seen as causal and only indicates towards a certain mechanism. We also show that the number of group members that previously switched away from the TT affect the likelihood of the individual also switching in the subsequent round. Interestingly, we find no evidence that socio-demographic characteristics of those that switched in the previous round affects individuals.
Last but not least, we look at treatment heterogeneities to analyze whether there is a difference in peer effects for individuals with different characteristics. Hence we study susceptibility to peer effects. We show that those with the highest cognitive ability are less susceptible to peer effects. We further find that peer effects seem to be stronger for those living in small villages compared to those living in large villages.
This paper contributes to a large and growing literature on peer effects and consumption. Recent studies using data from field experiments find that individuals increase their consumption expenditure when their neighbors becomes exogenously wealthier (Angelucci and De Giorgi Citation2009; Kuhn et al. Citation2011; Roth Citation2014; Boneva Citation2014). Previous studies use exogenous group formation as an identification strategy to show that social interaction effects are also important in other domains. Sacerdote (Citation2001), for example, uses the random assignment of college students to their respective dorms to analyze peer effects in education. Bandiera, Barankay, and Rasul (Citation2010) and Mas and Moretti (Citation2009) find a positive and significant relation between social interaction and work performance. Chetty, Hendren, and Katz (Citation2015) show that moving into more affluent neighborhoods, has a positive influence on the well-being of children.
Other papers use the existence of partially overlapping groups of peers to solve issues related to both reflection and correlated effects. The intuition is that partially overlapping groups generate peers of peers (or excluded peers) who act as instruments in the simultaneous equation model of social interactions, thus, solving the reflection problem. De Giorgi, Pellizzari, and Redaelli (Citation2010), for instance, show that the choice of a major is influenced by peers using this method.
Another alternative is to use a lab experiment to causally measure peer effects. Using a lab experiment, Falk and Ichino (Citation2006) find positive peer effects in terms of labor productivity. Using a similar setting, Baecker and Mechtel (Citation2014) study the effect of peers on cheating behavior. The study coming closest to ours is the experimental study by Clingingsmith and Sheremeta (Citation2015). They investigate the effect of visibility and income on the demand for conspicuous goods. In a fully-controlled laboratory setting, they vary both whether the purchase of a physical product is publicly visible or kept private and whether the income used for purchase is linked to social status or randomly assigned. Similarly, Bougheas, Nieboer, and Sefton (Citation2013) find that risk taking is correlated between group members, when they consult, but in line with our results, do not find a difference in the average risk taking.
Our results also contribute to the literature on conformity which is defined as an intrinsic taste to follow others (Goeree and Yariv Citation2011), driven by factors such as popularity, observational learning, information, esteem and respect (Bernheim Citation1994), as well as inequality aversion (Fehr and Schmidt Citation1999; Bolton and Ockenfels Citation2000).
Evidence on the exact mechanism that underlies conformity is still rare. Bursztyn et al. (Citation2014) show, using a carefully designed field experiment, that both information transmission between friends and social network externalities play a role in peer effects regarding the demand for financial products. Delfino et al. (2016) show that information about peers lead respondents to imitate their investment decision. Cai, Chen, and Fang (Citation2009) look at an experiment with two treatments in a restaurant setting in order to distinguish the effect of social learning from the effect of salience. Hefferz (Citation2012) study to role of visibility of certain consumption goods and links this back to peer effects.
The remainder of this article is organized as follows. In Section 2, we present our data, experimental design and discuss descriptive statistics. Group level results are reported in Section 3. Section 4 provides individual level results. Section 5 concludes.
II. Data
Household survey data and sampling
Our peer experiment was part of the larger household survey of the research project ‘Impact of shocks on the vulnerability to poverty: Consequences for development of emerging Southeast Asian economies’ funded by the German Research Foundation. As part of this project surveys have been conducted in three northeastern provinces of Thailand since 2007. It is important to note that all villages as well as respondents were randomly sampled for the household survey. Details concerning sampling and the household survey can be found in Online Appendix A. In addition to the peer experiment, we also collected data on a number of variables to complement the household survey. Questions designed to measure cognitive ability and overconfidence were asked after the peer experiment (Details are reported in Online Appendix B).
Experimental design
The peer experiment was conducted in August 2013 with a total of 521 respondents from 66 villages in Ubon Ratchathani, the largest of the three Thai provinces, where the household survey takes place. The experiment consists of a very simple choice task that required no previous knowledge, that was easy to implement and to measure in the field with a rural sample (Details on the implementation and instructions of the experiment can be found in Online Appendix A). Each respondent has to choose between a tasty treat or a certain amount of money. The tasty treat consists of popular items that are widely known across the country – a can of Coca-Cola, a piece of custard cake, a small package of Lays Classic Potato Crisps, a bar of chocolate, and a small pack of candy. It has a value of 40 THB (approximately 1 Euro). We made sure that the tasty treat included both sweet and savory items so that it would appeal to a wide range of tastes.
These products are widely available throughout Thailand and are also available in small shops in remote villages. Although traditional markets are still common throughout rural Thailand, small shops that sell goods for every day needs and snacks exist in most villages. The prices of the products we used do not vary throughout the country. In the case of the chocolate bar the price is even printed on the packet. Hence, there should be no difference in actual costs or any other kind of transaction costs across different villages.
In the first round the enumerator asks respondents whether they would like to choose the tasty treat or 10 Thai Baht (THB). Respondents have to express their choices to their assigned enumerator. Once the respondent has made the decision for that round, the enumerator moves to the next round. In the second round, the respondent has to choose between the tasty treat and 20 THB. This continues for a total of seven rounds, with the value of the cash increasing by 10 THB each round. In the last round, the respondent has to choose between the tasty treat and 70 THB. In order to make the experiment as easy as possible to follow, we use showcards that display the amount of money they can chose. The tasty treat is also placed directly in front of each respondent. In round four there is no price difference between the two choices. After round four, it becomes increasingly unreasonable to choose the tasty treat because of the significant price difference. The enumerator assigned to each respondent records the decision in each round. We allowed switching back and forth. There were 24 respondents who switched twice and were dropped from later analysis.
Before the experiment, respondents were asked to estimate the price of the tasty treat. After their prediction, each respondent was informed that the tasty treat costs 40 THB in order to avoid information asymmetries concerning the value of the product.
Another important component of our experiment was that the respondents would receive the tasty treat immediately after the experiment while they had to wait for the money until the end of the session, thus, enhancing temptation. Respondents were reminded that they had to stay after the experiment and answer further questions (i.e. risk attitude, financial literacy, overconfidence and cognitive ability). During the experiment, we made sure that the respondents did not receive any food or sweet beverages. Time-discounting factors can largely be ignored since the seven rounds of choosing between the tasty treat and money only took about ten minutes. The full session including post-experimental questions lasted on average one hour.
Once all seven choices were made, one decision was randomly played out by picking a number between 1 to 7 from a non-transparent bag. If the respondent picked number 3 and chose the tasty treat in round 3, he/she received the tasty treat immediately. If the respondent picked money in that round, the respondent would receive 30 THB at the end of the survey. All participants received 50 Baht as a show-up-fee at the end of the session. After the experiment, respondents were asked how much he/she would be willing to pay at most to receive the tasty treat.
Randomization between treatment and control group took place at the village level. This means that in treatment villages, the experiment was played in groups where individuals could observe the choices and actions of each other. In control villages, the experiment was played individually where respondents could not hear and see the choices of other participants as they were separated from each other. In detail, in the control villages, the tasty treat game was played individually and was conducted with 261 individuals in 66 groups. Groups refer to a number of people that where randomly selected to play in the village at the same time. In the control village, we refer to these groups as non-observing groups as participants cannot see the choices of others. It is also unlikely that the decision of one respondent affects other respondents in the control group because individuals respond at different speed levels. One tasty treat was always on display for each individual respondent (for details see Figure A.1 in Online Appendix A, hence there should be no confusion that each respondent in the control villages was separate of other participants.
The peer treatment which was conducted in the treatment villages was conducted with 260 individuals in 60 observing groups. The procedure of the treatment is the same as in the individual treatment, or non-observing group, with the sole exception that decisions were conducted with peers observing each other, hence respondents could see and hear each other. Each respondent is still responsible for their own choices, but they have to sit next to each other while completing the experiment. As in the control group, all the instructions were read out loud and showcards were used to demonstrate the possible choices between tasty treat and money in each round. The same number of tasty treats were on display as there were respondents. The principal enumerator read out the instructions. In each round, each respondent announces their choice out loud and the enumerator assigned to them would record their choice. After all respondents have made their choice, the group moves on to the next round (for details see Figure A.2 in the Online Appendix). There is no particular order in which respondents announce their choices within each round, thus creating a more dynamic and natural setting. In our analysis we use the respondents that played at the same time, but were not able to see or hear each other as a placebo group against the observing group where respondents were able to see each other.
Our experimental design does not allow us to observe who of the participants in the treatment groups answers first. Given our random assignment of individuals to play the game alone or in a group, we are able to create counterfactual groups out of individuals in the control villages that played the game at the same time as their peers, but without directly observing their peers. Thus, we have two types of groups – those that performed the experiment at the same time and same location directly observing each other in treatment villages and those that performed the experiment without observing each other in control villages. Hence, any difference in group outcomes can, therefore, be attributed to the only difference between groups – peer observation.
Descriptive statistics
shows summary statistics of individual and village characteristics broken down by treatment and control group as well as p-values for the t-test and Wilcoxon rank-sum test. Panel A displays results for individual characteristics. Our sample consists of around 50% women, average age is 54 years and 83% of our respondents are married. They have low levels of education (less than six years on average) with an average household size of more than four members. The vast majority of respondents name farming as their main occupation, with the remainder consisting of government officials, business owners, students, and housewives. As this study uses edible goods for consumption, we also look at the body mass index (BMI). The average in our sample has a BMI of 23, which is within the normal BMI range according to the WHO. Despite considerable growth in rural Thailand over the last decades, the North East is still relatively poor, which is reflected in the average rate of consumption and average household wealth.
Table 1. Summary statistics and tests of randomisation on individual and village characteristics.
In addition to standard socio-demographic variables, we also collect a number of variables designed to measure cognitive ability (details are in Appendix B). First, we asked respondents to answer six math-based questions. On average 3.6 out of 6 math questions were answered correctly. The numeracy score shows a near normal distribution with 1.99% answering all incorrectly and only 4.81% answering all six questions correctly. Second, we asked respondents to name as many animals as they can in 60 seconds. The average number of animals named is 17.2; however the standard deviation for this measure is rather large at 6.86. The correlation between the two cognitive ability measures, numeracy test and word fluency is 0.355 (Spearman; p-value0.001). Both tests capture similar, yet distinct, aspects of cognitive ability. Third, we follow the same procedure as Dohmen et al. (Citation2010) and use a single combined measure of cognitive ability.
Finally, we also measure overconfidence of our respondents, by asking them to predict how many math questions they answered correctly. We define a respondent whose math prediction is higher than his/her actual score as overconfident, and a respondent whose prediction is below her actual score is called underconfident. Using this measure, 37% of our sample are overconfident while 33% are underconfident.
Panel B displays village characteristics and differences between villages that have been chosen for randomization. In terms of village characteristics, we find that the average distance to the next district capital and to the provincial capital, Ubon, is 16 km and 60 km respectively. The average number of shocksFootnote2 in our 66 villages was 1.45 ranging from 1 to 3 shocks in total. The number of households in a village varies significantly, ranging from 813 households in a village to only 55 households in a village.
Aside from villages characteristics and most importantly for our study, the table shows that villages do not differ significantly from each other. This holds for all village characteristics that we look at, especially the number of poor households (p-value0.18) and the exposure to shocks between villages (p-value
0.65). This indicates that average income levels as well as purchasing power between households in different villages is not significantly different between treatment and control villages. Hence, transaction costs between villages in terms of tasty treat should not differ and our results should not be driven by the price of the tasty treat or different opportunity costs of households between different villages.
also shows that randomization was successful and that there are no significant differences in observable factors between those that played the tasty treat game alone and those that played the game with peer observation. The only difference is that those who played in a group have, on average, more children which is statistically significant in the t-test and in the Wilcoxon rank-sum test. We control for this difference in further analysis.
As this study not only compares the behavior of individuals but also looks at the behavior of groups, it is important to check that group composition is the same between those that played in observing and non-observing groups. There are 126 groups in total with 60 observing groups and 66 non-observing groups. shows that group means and group standard deviations are the same when looking at measured observables, with the exception of the number of children. Hence observable and non-observable groups are the same on average in composition and are equally alike. However, we also control for possible confounding effects in later regressions.
Table 2. Comparing means and standard deviation for non-observing and observing groups.
III. Group level results
Comparing groups
We begin our analysis on the effects of peer observation by studying the difference between those groups that played the game observing each other and those that played the game at the same time and under the same conditions as others but without observation. T-tests and Wilcoxon-rank tests compare decisions between the two types of groups in . At first, it seems that there is no difference in the mean group choice of the last row that was chosen since the average last row chosen in both groups is the same.
Table 3. Comparing outcomes for non-observing and observing groups.
We observe, however, a difference in the standard deviation in the last row of the tasty treat chosen between observing and non-observing groups. The standard deviation in the observing group is significantly lower than in the non-observing groups. This is also reflected in the group minimum and the group maximum. The group minimum is the lowest switching point of anyone within the group, whereas the group maximum is the highest switching point within a group. We find that the group minimum is significantly higher and the group maximum is significantly lower when the experiment is performed with peers observing each other.
The finding is further supported in , where we control for both group means and group standard deviations of observables. Outcome variables stay the same as above (i.e. group mean, group minimum, group maximum, group standard deviation). The peer dummy is unity if the group played with peer observation. We confirm our finding from above – observing groups have lower standard deviation. The same can be seen when looking at the group minimum and maximum. The coefficient on the peer dummy is positive and significant in the regression estimating the group minimum and negative and significant in the regression estimating the group maximum. Interestingly, average group composition seems to have only a limited influence on the tasty treat choice. Groups with more women switch from tasty treat to money earlier. Similarly, there seems to be an effect of groups that are richer, i.e. that have higher average consumption.
Table 4. Group level treatment effect on PayTT.
In contrast, we find that the standard deviation of group observables seems to be linked to group outcomes. Specifically, we find that groups with higher standard deviations of cognitive ability seem to have higher mean switching rows and a higher group maximum. Interestingly, there seems to be a negative effect on the group maximum in groups with lower standard deviation in children. A negative and significant effect is also found for schooling, BMI, and overall well-being of the respondent in the column on the group minimum, but only at the 10% significance level for two of these cases. It should also be noted that group standard deviation of observables is not linked to standard deviation in choices. Hence our results in are not driven by similarity in groups. Both and show that there is a significant difference in consumption choices between observing and non-observing groups. We find that consumption choices converge in observing groups. Hence, there is evidence for conformity when respondents observe each other.
Performing the experiment in an observing group seems to affect those that would have chosen the money early or those who decided to keep taking the tasty treat until later rounds. In we again use t-test and Wilcoxon-rank tests to compare deciles between observing and non-observing groups. We find that the first and second decile in the observing group is higher than in the non-observing group. The opposite can be seen when looking at the eighth and ninth decile. This is significantly higher in non-observing groups than in observing groups. This effect is stronger for the higher deciles. To conclude, it seems that peer observation particularly affects those that would have made extreme choices.
Table 5. Comparing deciles between non-observing and observing groups.
Giving in to temptation?
We here briefly discuss our respondent’s propensity to give in to temptation. This experiment was deliberately performed using temptation goods, as evidence from social psychology (Gunter and Furnham Citation1998; Steinberg and Cauffman Citation1996), suggests that demand for these goods is more likely to be influenced by the presence of peers. At the same time, we believe that playing the game with temptation goods and hence non-essential goods gives cleaner evidence of the effect of peer observation. It is possible that playing the game with other goods that can be considered necessities would have had a confounding effect on the choices made by individuals.
We here define giving in to temptations as choosing the tasty treat when the cash alternative is more than 40 THB, the TT’s inherent price. From the tables discussed above, it could be inferred that subject do not give in to temptation. First, it seems that peer observation seems to prevent subjects from giving in to temptation since the mean of the average group choice for both the treatment and control group is just below three. Thus, the average respondent in both treatments switches from the amount of money offered well below the purchasing value of the tasty treat. The same applies to median choice, which lies just below three for both the treatment and the control groups. On the other hand, peer observation not only lowers the switching point of those that would otherwise have given in to temptation, but it also raises the switching point of those that would have switched very early. In other words, peer observation induces conformity, but does not change the decision in any particular direction. Hence, we do not find evidence that peer observation acts as a self-control mechanism against individual temptation.
IV. Individual level results
Identification strategy
We are interested in identifying causal peer effects on individual decision-making and understanding whether consumption is affected by the observation of peers. The identification of peer effects, however, suffers from a number of econometric issues (Manski Citation1993; Moffit Citation2001) which can be summarized into three categories: (a) correlated effects; (b) contextual effects; and (c) endogenous effects. Our experimental design (discussed in Section 2) represents an attempt to surmount the challenge of identifying a causal peer effect. Much of the literature following Manski focuses on the econometric issue of separating the causal peer effect from that of correlated unobservables (Miguel and Kremer Citation2004; Bandiera and Rasul Citation2006; Conley and Udry Citation2010). Two ways of disentangling these effects are to (1) randomize the peers (Sacerdote Citation2001; Duflo and Saez Citation2003) or (2) randomize an intervention or new technology (Kremer and Miguel Citation2007; Oster and Thornton Citation2012; Godlonton and Thornton Citation2012). We follow the first approach.
The double randomization in our experimental design, that is, randomly selecting individuals to perform the experiment given the sampling procedure and also randomizing peer and control treatments according to villages, circumvents the problem of correlated effects. Since we chose randomly who plays in a peer group and who is part of that peer group, there are unlikely to be any unobservables that would systematically influence the choices made by individuals. At the same time, our experiment takes place in a relatively controlled environment and only takes a short period of time. It is, hence, unlikely that unobservable time-variant characteristics influence decisions made by respondents.
To identify endogenous peer effects we use the so called leave-out mean as the regressor in order to analyze the effect of the group average consumption on the individual consumption choice. While we are able to identify endogenous peer effects, we are not able to circumvent the reflection problem.
To identify the effect of peer observation, we will estimate the main regression model in the following form using least squares estimation:
In our framework, is the consumption choice of tasty treat for individual
who has group affiliation
(observing or non-observing group). In our main analysis
will be the last row in which they choose the tasty treat before switching to money. The coefficient of interest is
, the coefficient on the group mean. The leave-out mean is previously used by Townsend (Citation1994), Guryan, Kroft, and Notowidigdo (Citation2009), Duflo, Dupas, and Kremer (Citation2011), Carrell, Hoekstra, and West (Citation2011) and discussed by Angrist (Citation2013). In our analysis we split the sample between those performing the experiment in observing groups and those in non-observing groups. We expect
to be positive when the experiment is performed with peer observation. When the experiment is, on the other hand, performed without peer observation we expect
to be 0.
We also include the variable , which is the matrix average group socio-economic characteristics in group
, excluding the individual
.
is composed of a set of individual characteristics, such as female, age, schooling, log consumption, household size, dependency ratio, and BMI, that may affect consumption decisions. The error term
is clustered on the village level.
As we assign respondents randomly into peers groups, we assume , i.e., no correlated effects or self-selection into groups. Thus, if we observe a difference in outcomes between observing and non-observing groups we can attribute this directly to the (on average) only difference between these groups, namely peer observation.
Peer effects
As a next step, we look at peers and their effect on individual decisions, as described in the previous section. The outcome variable is the round when the individual switches from the TT to money. Robustness using other outcomes are shown in Online AppendixD. We find an effect of the group average on the individual consumption choice. We perform the regression using the above equation. Results are presented in . The first two columns cover the entire sample. We find that there is a significant and positive relationship between the average switching point in the group and individual’s switching point. As this covers the entire sample, this could be caused by correlated effects. Hence, in the next four columns, we split the sample into those performing the experiment in observing groups and those performing it in non-observing groups (denoted as Peer and Single). In columns 3 and 4 we observe that the positive and significant relationship found in column 1 and 2 remains and is even stronger for the observing group. Columns 5 and 6 show the same regression, but for respondents playing the game without peer observation. Here the effect of the average peer choice has no effect on the individual’s switching row. Similarly, in column 7 we introduce an interaction term between the group average and a dummy that takes the value one if the game was played in an observing group. The interaction term is positive and significant and so we can conclude that the relationship between the group average and the point of switching is not the same between observing and non-observing groups.
Table 6. Individual choice of tasty treat and group average.
These results in show that the peer effects observed in columns 3 and 4 above are not caused by unobserved correlated or contextual variables but rather by peers observing each other and making the same decision at the same time.
Mechanisms
So far, we find strong evidence for peer effects affecting consumption choices, thus causing conformity within a group. At the same time, we are able to show that individual decision-making is clearly influenced by the groups decision. However, it is not clear what the source of this group conformity is (information on the conceptual framework regarding conformity can be found in Online Appendix C).
The dynamic setting of our experiment allows us to gain an insight into what may be the mechanism behind the observed peer effects. In the literature, a number of reasons behind peer effects are discussed (Bikhchandani, Hirshleifer, and Welch Citation1998; Cai, Chen, and Fang Citation2009). Here we look at two factors: Firstly, peer effects are argued to be caused by respondent’s belief that others have better information (Foster and Rosenzweig Citation1995; Miguel and Kremer Citation2004; Conley and Udry Citation2010; Oster and Thornton Citation2012). Secondly, individuals could simply follow their peers because they are gaining some kind of network externality or benefit from doing what other people are doing (Moretti Citation2011). Due to the design of our experiment, we are unable to provide definite answers. Nonetheless, the results provide some interesting insights.
Firstly, we discuss the role of information on peer effects. As described in Section 2, we asked respondent to estimate how much the tasty treat costs in a shop. We use this response as a proxy for how familiar the respondents are with the tasty treat. We construct a variable using the difference between the actual and estimated price. Then, we use this variable and create an interaction term with the leave-out-mean and add both to the regressions shown in . Results are demonstrated in . Interestingly, unfamiliarity with the tasty treat makes the respondent less likely to choose it, but only in the treatment group. Not knowing the price of the product has no effect on the likelihood of choosing the tasty treat in the peer treatment. Hence, it seems possible that the effect of not knowing the product is counteracted by being able to observe one’s peers and so information transfer seems to play a role in explaining peer effects.
Table 7. Unfamiliarity with the tasty treat.
In order to look into the other possible mechanism, we study the decision of respondents to switch in a certain round, depending on the proportion of people in his/her group that has switched in a previous round. We here try to find out whether the number of people that have switched previously has an effect on the likelihood of an individual switching. shows results. The dependent variable is a dummy that is one if the respondent switches from the tasty treat to the money in a given round. The right hand variable is the proportion of people choosing the money i.e. had switched in previous rounds. shows these regressions for both the single and the peer treatments, for the decision taken in rounds two through round four. Since most people only switch once, the regressions only include people that had not switched in previous rounds. We find that the proportion of people that had switched in the previous rounds has a positive effect on the likelihood that a respondent will switch from TT to money in a given round. However, this relationship breaks down after round three – possibly because the number of people that had not switched beforehand is very small.
Table 8. Likelihood of switching on those that switched in previous round.
In the next step, we investigate whether individual decisions are affected by not just the number of group members who have previously switched but who among the group members have switched. We test whether the likelihood of switching is increased if group members with certain characteristics (i.e. age, wealth, over-confidence, cognitive aptitude) have switched in the previous round. We do not find any effects (details upon request). So it appears that the likelihood of switching is positively correlated with how many people have switched previously, but not with who has switched previously. It has to be noted here that due to our experimental design, it is possible that certain individuals within the group exert influence over other members, but we are not able to identify this through the choices that individuals make in each round. Instead this influence may be more subtle, occurring between rounds. Similarly, it is possible that unobserved characteristics make some people follow others.
In this section we present evidence that both information and network externalities are behind peer effects. Unfamiliarity with a product seems to be counteracted by peer effects and at the same time the number of people in a group who have switched previously affects the likelihood of an individual switching in a given round. These results only provide hints and not definite answers.
Treatment heterogeneity
Next, we test whether certain people are more likely to succumb to peer effects. We here look at both behavioral types and the social environment in which people live to see if some individuals are more likely to conform than others. There is a growing literature linking cognitive ability and financial literacy to improved financial behaviours and outcomes (see for instance Bertrand and Morse Citation2011; Agrawal and Mazumder, Citation2013). We hypothesize that high cognitive ability individuals should be less prone to peer pressure, while the opposite should be true for low skilled respondents.
Using the cognitive ability question, we create a dummy for those with the highest and one dummy for those individuals that have the lowest cognitive ability score compared to their peers within the group to test the effect of relative cognitive ability in a group. To test for the effect of absolute cognitive ability, we generate a dummy for those that scored in the highest 10% of the distribution. We include these dummies together with an interaction term between the dummy and the leave-out-mean into our regressions above using only the peer treatment. shows results. We find that the high cognitive ability (both in relative and in absolute terms) individuals are less likely to succumb to peer effects (column 1 and 3). This effect is weakened when controlling for individual and group characteristics, but the signs remain the same. We, however, do not find any statistically significant results for low cognitive ability people. We also use this method to test whether overconfident and wealthy individuals are more prone to succumb to peer effects. We do not find any significant results.
Table 9. Treatment heterogeneity: high cognitive ability and living in small villages.
Another interesting question is whether people who live in closer knit communities are more susceptible to peer effects. The cost of not doing what everyone else in the group is doing may be higher for those that live in communities with stronger social ties. We test whether the peer effect is stronger for those living in smaller villages. For this, we create a dummy that is unity for those respondents that live in villages that are smaller than the median size. Results are shown in in columns five and six. We can see that the small village dummy as well as the interaction term are significant. This shows us that those that live in smaller villages are more likely to conform to the group. This indicates that stronger social ties may lead to stronger conforming behavior. We also test whether women are more susceptible to peer effects. It is often argued that women are more social than men and, therefore, it is possible that they are more likely to be affected by peer effects. However, we find no evidence of this here. Further heterogeneity analysis regarding session times is shown in the Online Appendix D.
V. Conclusion
We conduct a lab-in-field experiment which can be linked to a very detailed household survey and find that peer observation leads to conformity. Due to the experimental design and the large number of control variables, we can circumvent the identification problems normally found in studies on peer effects. We find that standard deviations of those groups that observe each other are lower than for those groups that do not observe each other. At the same time, we show that individual choices are influenced by the group choice (measured as the leave-out group mean). Most importantly, we only observe this when the experiment is performed with peer observations. Hence, we provide clear evidence of peer effects and conclude that peer observation leads to conformity. This finding is in line with other studies on peer effects that look at various outcome variables (Falk and Ichino Citation2006; Bandiera, Barankay, and Rasul Citation2010; Bursztyn et al. Citation2014, De Giorgi, Pellizzari, and Redaelli Citation2010)
Our study adds to the literature by looking into the possible mechanism behind this conformity. We study the effect of familiarity with the product and find that peer observation can counteract the effect of a lack of knowledge of the product. There is also evidence that the number of people that switched previously increases the likelihood of an individual switching in the next round. We find no evidence that the characteristics of those that switched in the previous round is important. Hence we find evidence for both, information and psychological channel underlying peer effects.
Finally, we investigate treatment heterogeneities in order to see if the tendency to conform is stronger for some behavioral traits or environments. We find that individuals with high cognitive ability are less likely to succumb to peer effects. We also find evidence that those who live in closer knit communities are more likely to succumb to peer effects.
Despite these findings, many open questions remain, thus, further research into peer effects and its effect on consumption choices is needed. More research looking into the mechanisms behind peer effects and what leads to conformity is needed. While our research provides a first insight into this mechanism, additional research should be done into the effect of key individuals within a group – investigating who leads a group and who follows. Another open question is whether peer effects, as found in this experiment, persist beyond the time of observation.
Supplemental Material
Download PDF (2.1 MB)Disclosure statement
No potential conflict of interest was reported by the authors.
Supplementary material
Supplemental data for this article can be accessed here.
Additional information
Funding
Notes
1 These are, for example, alcohol, cigarettes or as in our case unhealthy foods (Banerjee and Mullainathan Citation2010).
2 A shock is an adverse event that affects the village, such as flooding or changes to the infrastructure serving the village. Village data was reported by the village head.
References
- Agarwal, S., and B. Mazumder. 2013. “Cognitive Abilities and Household Financial Decision Making.” American Economic Journal: Applied Economics 5 (1): 193–207.
- Agarwal, S., W. Qian, and X. Zou. 2016. Thy Neighbor’s. misfortune: Peer effect on consumption.
- Angelucci, M., and G. De Giorgi. 2009. “Indirect Effects of an Aid Program: How Do Cash Transfers Affect Ineligibles Consumption?.” American Economic Review 99 (1): 497–516.
- Angrist, J. 2013. “The Perils of Peer Effects.” Labour Economics 30 (1): 98–108.
- Baecker, A., and M. Mechtel (2014). “Peer Effects in Cheating on Task Performance.” Unpublished Manuscript.
- Bandiera, O., I. Barankay, and I. Rasul. 2010. “Social Incentives in the Work- Place.” Review of Economic Studies 77 (2): 417–458.
- Bandiera, O., and I. Rasul. 2006. “Social Networks and Technology Adoption in Northern Mozambique.” Economic Journal 116 (514): 869–902.
- Banerjee, A., and S. Mullainathan (2010). “The Shape of Temptation: Implications for the Economic Lives of the Poor.” Working Paper No. 257.
- Bernheim, B. D. 1994. “A Theory of Conformity.” Journal of Political Economy 102 (5): 841.
- Bertrand, M., and A. Morse. 2011. “Information Disclosure, Cognitive Biases, and Payday Borrowing.” Journal of Finance 66 (6): 1865–1893.
- Bikhchandani, S., D. Hirshleifer, and I. Welch. 1998. “Learning from the Behavior of Others: Conformity, Fads, and Informational Cascades.” Journal of Economic Perspectives 12 (3): 151–170.
- Bolton, G. E., and A. Ockenfels. 2000. “ERC: A Theory of Equity, Reciprocity, and Competition.” American Economic Review 90 (1): 166–193.
- Boneva, T. (2014). “Neighbourhood Effects in Consumption: Evidence from Disaggregated Consumption Data.” Cambridge Working Paper 1328.
- Bougheas, S., J. Nieboer, and M. Sefton. 2013. “Risk-taking in Social Settings: Group and Peer Effects.” Journal of Economic Behavior and Organization 92 (100): 273–283.
- Bursztyn, L., F. Ederer, B. Ferman, and N. Yuchtman. 2014. “Understanding Mechanisms Underlying Peer Effects: Evidence from a Field Experiment on Financial Decisions.” Econometrica 82 (4): 1273–1301.
- Cai, H., Y. Chen, and H. Fang. 2009. “Observational Learning: Evidence from a Randomized Natural Field Experiment.” American Economic Review 99 (3): 864–882.
- Carrell, S. E., M. Hoekstra, and J. E. West. 2011. “Is Poor Fitness Contagious?.” Journal of Public Economics 95 (7–8): 657–663.
- Chetty, R., N. Hendren, and L. F. Katz. 2015. “The Effects Of Exposure to Better Neighborhoods on Children: New Evidence from The Moving to Opportunity Experiment.” American Economic Review 106 (4): 855-902.
- Chlingingsmith, D., and R. Sheremeta (2015). “Status and the Demand for Visible Goods: Experimental Evidence on Conspicuous Consumption.” MPRA Working Paper No. 68202.
- Conley, T. G., and C. R. Udry. 2010. “Learning about a New Technology: Pineapple in Ghana.” American Economic Review 100 (1): 35–69.
- Dohmen, T., A. Falk, D. Huffman, and U. Sunde. 2010. “Are Risk Aversion and Impatience Related to Cognitive Ability?” American Economic Review 100 (3): 1238–1260.
- Duflo, E., and E. Saez. 2003. “The Role of Information and Social Interactions in Retirement Plan Decisions: Evidence from a Randomized Experiment.” Quarterly Journal of Economics 118 (3): 815–842.
- Duflo, E., P. Dupas, and M. Kremer. 2011. “Peer Effects, Teacher Incentives, and the Impact of Tracking: Evidence from a Randomized Evaluation in Kenya.” American Economic Review 101 (5): 1739–1774.
- Falk, A., and A. Ichino. 2006. “Clean Evidence on Peer Effects.” Journal of Labor Economics 24 (1): 39–57.
- Fehr, E., and K. Schmidt. 1999. “A Theory of Fairness, Competition, and Cooperation.” Quarterly Journal of Economics 114 (3): 817–868.
- Foster, A. D., and M. R. Rosenzweig. 1995. “Learning by Doing and Learning from Others: Human Capital and Technical Change in Agriculture.” Journal of Political Economy 103 (6): 1176–1209.
- Giorgi, G. D., M. Pellizzari, and S. Redaelli. 2010. “Identification of Social Interactions through Partially Overlapping Peer Groups.” American Economic Journal: Applied Economics 2 (2): 241–275.
- Godlonton, S., and R. Thornton. 2012. “Peer Effects in Learning HIV Results.” Journal of Development Economics 97 (1): 118–129.
- Goeree, J. K., and L. Yariv. 2011. “An Experimental Study of Collective Deliberation.” Econometrica 79 (3): 893–921.
- Gunter, B., and A. Furnham. 1998. Children as Consumers: A Psychological Analysis of the Young People’s Market. London: Routledge.
- Guryan, J., K. Kroft, and M. J. Notowidigdo. 2009. “Peer Effects in the Work-place: Evidence from Random Groupings in Professional Golf Tournaments.” American Economic Journal: Applied Economics 1 (4): 34–68.
- Heffetz, O. 2012. “Who Sees What? Demographics and the Visibility of Consumer Expenditures.” Journal of Economic Psychology 33 (4): 801–818.
- Kremer, M., and E. Miguel. 2007. “The Illusion of Sustainability.” Quarterly Journal of Economics 122 (3): 1007–1065.
- Kuhn, P., P. Kooreman, A. Soetevent, and A. Kapteyn. 2011. “The Effects of Lottery Prizes on Winners and Their Neighbours: Evidence from the Dutch Postcode Lottery.” American Economic Review 101 (1): 2226–2247.
- Mangyo, E., and A. Park. 2011. “Relative Deprivation and Health: Which Reference Groups Matter?” Journal of Human Resources 46 (3): 459–481.
- Manski, C. F. 1993. “Identification of Endogenous Social Effects: The Reflection Problem.” Review of Economic Studies 60 (3): 531.
- Mas, A., and E. Moretti. 2009. “Peers at Work.” American Economic Review 99 (1): 112–145.
- Miguel, E., and M. Kremer. 2004. “Worms: Identifying Impacts on Education and Health in the Presence of Treatment Externalities.” Econometrica 72 (1): 159–217.
- Moffit, R. 2001. Policy Interventions, Low-Level Equilibria, and Social Interactions. Washington DC: Brookings Institution Press.
- Moretti, E. 2011. “Social Learning and Peer Effects in Consumption: Evidence from Movie Sales.” Review of Economic Studies 78 (1): 356–393.
- Oster, E., and R. Thornton. 2012. “Determinants of Technology Adoption: Peer Effects in Menstrual Cup Take-up.” Journal of the European Economic Association 10 (6): 1263–1293.
- Roth, C. (2014). “Conspicuous Consumption and Peer Effects among the Poor: Evidence from a Field Experiment.” CSAE Working Paper Series, 29.
- Sacerdote, B. 2001. “Peer Effects with Random Assignment: Results for Dart-mouth Roommates.” Quarterly Journal of Economics 116 (2): 681–704.
- Steinberg, L., and E. Cauffman. 1996. “Maturity of Judgment in Adolescence: Psychosocial Factors in Adolescent Decision Making.” Law and Human Behavior 20 (3): 249–272.
- Townsend, R. M. 1994. “Risk and Insurance in Village India.” Econometrica 62 (3): 539.