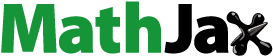
ABSTRACT
This paper analyzes spillover effects from previous matches against the future champion of a league. Using data from 78,264 European football games, the empirical results from a matching method show that favourites in the current match gain significantly fewer points after a match against the future league champion. By contrast, underdogs benefit from such a match, as they gain significantly more points. By considering shadow effects in the analysis, these results suggest that favourites put more effort into the match against the future champion and lower their effort level in the next match, which explains the negative spillover effects. As there are no significant shadow effects for underdogs, the positive spillover effects might be caused by positive ‘learning-by-doing’ effects. A subsequent logit regression using betting odds as control variables confirms the existence of the observed spillover effects.
I. Introduction
Tournaments and contests are important economic designs to find a ranking among all participants or to find the best player or team. For example, during a football season, each team usually plays twice against each other team in the league (double round-robin tournament) to determine a winner and a ranking among all teams at the end of the season. As the quality of the participating teams is usually heterogeneous, the scheduling of the matches might influence the ranking, because there are spillover and shadow effects involved. Spillover effects occur when the outcome of a match is influenced by a team’s last opponent. By contrast, shadow effects affect present results from the future opponent of a team.
The aim of this paper is to study the spillover effects in European football after a team has played against a high-quality team of its league, which I define as the future champion of the league. This definition ensures that the best team in a certain season is chosen. The spillover effect could then be negative if a team put more effort into the match against the future champion and is still exhausted, or if there are negative externalities involved. On the other hand, the spillover effect may even be positive if there are positive externalities of playing against a high-quality team, or if a team reduces its effort in the current match, in order to have a higher chance of winning its next one.
To test this hypothesis regarding spillover effects, I use data on 78,264 matches played in European football. Employing a matching method, I find that there are positive as well as negative spillover effects from having played against the future champion, and that the sign is dependent on a team’s own quality, as well as of the next opponent’s. If a team is favourite in its match after having played against the future league champion, it has a lower winning probability in the upcoming match, and an increased winning probability if it is the underdog. In addition, the results on the existence of spillover effects are confirmed in a logit regression using betting odds as control variables. To the best of my knowledge, this paper is the first to detect spillover effects in sports, dependent on the quality of opposition in the last match.
Furthermore, this paper extends the literature by differentiating between two types of spillover effect (effort level in the previous match and externalities) and attempting to distinguish between these effects in the data by considering additional shadow effects in the analysis. Here, I find that a high-quality team performs better against the future winner if it is the favourite in the next match, which explains the negative spillover effects, as it might still be exhausted or put less preparation into this match. As no shadow effect is found in the data for underdogs, this suggests that the positive spillover effects for underdogs are caused by a positive externality.
In addition, the paper also contributes to research on forward-looking of decision-makers in economic contexts, as (indirect) evidence is found that high-quality teams are capable of doing so, as they adjust their current level of effort to the strength of future opponents, among other factors. Dynamic strategic behaviour plays a large role in economic theory, for example, in promotion tournaments (e.g. Rosen Citation1986). One of the underlying assumptions of these theoretical models is the capability of economic agents to be forward-looking, which is, however, empirically unclear. Altmann, Falk, and Wibral (Citation2012), for example, show that participants in a laboratory experiment exert excess effort in early stages of a tournament, apparently caused by limited degrees of forward-looking. Here, I find that high-quality teams indeed adjust their dynamic behaviour to the strength of the present and future opponent by choosing a more appropriate and beneficial strategy.
This paper corresponds with the existing literature on spillover effects in tournaments with heterogeneous players. Brown and Minor (Citation2014), for example, develop a theoretical model that allows for positive and negative spillover effects on the outcome of the next match in elimination tournaments. In their empirical study, using data on professional tennis, they find that the spillover effect caused by exhaustion (measured by the number of tennis games played during the tournament) reduces the probability of a player winning the match, and that this effect is stronger for favourites than for underdogs. This situation is also observed in the corresponding betting odds.
In contrast to these findings, Hill (Citation2018) finds that there are positive exhaustion spillover effects for favourites in the NBA playoffs. Hence, a team that is favourite in the current game has a higher winning probability after having played more games in the playoffs so far. Hill (Citation2018) explains his results by fewer resting days in this case and a possible momentum effect carrying over into the next games. However, neither study controls for the playing ability of the last opponent, which also might have caused any spillover effects. Both studies also investigate possible shadow effects of a future opponent’s quality, and find that the likelihood of a favourite winning in the current stage increases, when the (expected) quality of the future opponent decreases.
In studying spillover effects from the last opponent, round-robin tournaments have the advantage that information on future opponents is available at any time in the tournament. One of the first empirical studies to test for spillover effects in round-robin tournaments is that of Goossens and Spieksma (Citation2012a), who try to identify effects caused by the quality of opposition in the last match. More precisely, they analyse so-called ‘carry-over effects’ that measure the effect of strength of the opponent’s last opposition on the performance of a team. However, spillover and carry-over effects should impact in opposite ways, as a higher (lower) winning probability of a team lowers (improves) that of the opposition. The study of Goossens and Spieksma (Citation2012a) on carry-over effects also attempts to detect spillover effects. Using data from the highest football division in Belgium, they find no evidence of carry-over or spillover effects. Their study does not control for the playing strength of both teams in the observed match, as they assume the effect to be constant for all teams and therefore also for both favourites and underdogs.
Most recently, Deutscher et al. (Citation2019) show theoretically that in round-robin tournaments, underdog teams self-restrict their efforts in order to hold back resources for the next match, if the inequality of strength is sufficiently large in the current match. They test the prediction of their model by checking whether football players are suspended more frequently against high-competition teams, due to a rule declaring that players are automatically suspended for the next match after receiving a certain number of yellow cards in the current tournament. They argue that teams might use the suspension as a strategy, by provoking a yellow card prior to the contest against the high-competition team. By studying data on the first football league in Germany, they find evidence of such behaviour, if the heterogeneity of the two teams in the next match is low.
The work of Deutscher et al. (Citation2019) is strongly related to studies analysing the ‘discouragement effect’ and ‘contamination hypothesis’ that predict lower efforts of heterogeneous players competing against each other (e.g. Rosen Citation1986; Konrad Citation2009; Sunde Citation2009; Bach, Gürtler, and Prinz Citation2009). This phenomenon can be explained by high investment costs for underdogs in order to win against the favourite, costs they may be unwilling to pay. Instead, the underdog lowers his effort, and by anticipating this behaviour, the favourite does the same to save resources for future contests. According to this theory, low-quality teams might have a positive spillover effect in the next match, as they have self-restricted and saved resources for future contests.
Some football teams might be favoured or handicapped due to possible spillover effects, when they gain or lose points as a result. Therefore, this article is also related to effects caused by playing conditions, scheduling and crowd effects, as these effects also either benefit or handicap certain teams. For example, Casas and Fawaz (Citation2016) show that altitude is a handicap in football tournaments for teams that are not used to the air, so that they have a disadvantage playing under these conditions. In addition, there are several studies analysing the home-field advantage during the COVID-19 pandemic, when no fans at all were allowed to attend matches in stadiums, and which demonstrate that the advantage for the home team is reduced in empty stadiums (e.g. Dilger and Vischer Citation2020; Fischer and Haucap Citation2020). Essentially the same results were found by Pettersson-Lidbom and Priks (Citation2010), who additionally analyse the referee bias towards the home team in empty stadiums. Their results suggest that the behaviour of the referee is affected more by the lack of the crowd than the players, which explains the decreased home-field advantage.
The remainder of this paper is structured as follows. Section 2 describes the conceptual framework of spillover effects in tournaments. Section 3 presents the data and some relevant descriptive statistics, after which the estimation strategy is discussed in Section 4, before the empirical results are presented in Section 5. In Section 6, an additional robustness check with betting odds is conducted, and Section 7 concludes.
II. Conceptual framework of spillover effects
When studying spillover effects, it is important to distinguish between endogenous and exogenous spillovers. Endogenous spillovers occur when teams choose a different effort provision in the previous match, which impacts on the effort level in the present match. On the other hand, exogenous spillover effects appear when the effect is independent of the chosen effort level prior to the observed match. Spillover effects caused by a different effort level in the last match are then a strategic decision of a team, whereas exogenous spillovers are externalities that are only caused by the last opposition.
It is a challenge to determine the effort choice of economic agents empirically, as the effort level in a contest is usually unobservable. However, data in sports science often contain direct or indirect information on effort provision. Although there are some works that attempt to measure effort provision of teams in a match more directly (e.g. personal fouls committed (Lackner et al. Citation2015), the strategic use of suspensions (Deutscher et al. Citation2019) or the value of a starting line-up (Rohde and Breuer Citation2017)), in the remainder of this paper, the effort provision of teams is measured in terms of match outcomes. The application of match outcomes or scores as a proxy for effort is in line with the sports economics literature (see, e.g., Brown Citation2011; Pope and Schweitzer Citation2011; Brown and Minor Citation2014). A different effort provision of teams thus explains different outcomes or scores in matches (while controlling for team heterogeneity).
Shadow effects are also explained by a different level of effort in the present match, dependent on the quality of the next opponent (Hill Citation2018). A higher effort could lead to exhaustion and fatigue of the team and hence to higher marginal costs from effort in the next match and to a lower optimal effort level (Brown and Minor Citation2014). Fatigue from a previous match in a dynamic contest is, for example, theoretically analysed by Ryvkin (Citation2011), who shows that the effort level is lower in early stages of a contest in the presence of fatigue. These theoretical findings are confirmed in a laboratory experiment, in which participants indeed lower their effort at the beginning of a contest. Following this logic, one would expect negative (positive) spillover effects in the presence of positive (negative) shadow effects.
However, it is theoretically unclear in which direction shadow effects work in matches against the future champion. Following the theoretical and empirical findings of Deutscher et al. (Citation2019) for example, teams will save resources and restrict their efforts, if the inequality of strength is large in the present match. This condition should be met, as I investigate matches with the best team of the league competing. Teams might put less effort into the match against the future champion in order to be well rested in the upcoming match. Teams are then expected to increase their effort level in the next match, resulting in better performances and positive spillover effects.
On the other hand, teams have a higher incentive to win a match against a team competing for the same prize within the same league (e.g. for the championship or avoidance of relegation) compared to a match against either a far weaker or stronger competition, as points are more valuable against a close rival, because additional points gained result automatically in fewer points for the opposition. Accordingly, high-quality teams, which are also competitors of the championship contest, might devote more effort to the present match against the future league champion, if they face weaker competition in their next match, or if they are the favourite. In this case, one would expect positive shadow effects due to higher effort provision in the match against the future. However, these favourite teams might be exhausted after this match and subsequently devote less effort to the next game, as the marginal cost of effort has increased due to the higher effort level in the previous match (Brown and Minor Citation2014). This could then lead to negative spillover effects for favourites in the next match, as the effort level in this match is lower.
According to this theory, one would only expect to observe these positive shadow effects, as long as both teams (the high-quality team and the future champion) are still in the same race for the championship. If the high-quality team drops out of this race at the end of the season, because the point differential is already too large between these two teams, there is no longer a higher incentive to win this match and no positive shadow effects are expected.
If spillover effects are observable without shadow effects in the previous match, then externalities explain the spillover effects. Negative externalities from a match against the future champion can occur, for example, when teams face different playing styles in the two matches and have problems adapting to the second match, leading to worse results. Furthermore, it may be that more players are suspended or injured after a match against the future champion (Goossens and Spieksma Citation2012a). This results in higher marginal costs from effort, so that the team chooses a lower optimal effort level.
On the other hand, possible positive externalities from a match against the future champion can appear, when the marginal effect from effort devoted to productivity increases. Rampini et al. (Citation2007), for example, show that the running intensities of football players increase (decrease) when playing against high-quality (low-quality) opposition. Similar results are shown in Lago, Casais, and Sampaio (Citation2010), who find that the distance covered by players is larger against strong competition. In addition, high-quality athletes usually have superior cognitive coordination like reaction time or decision-making. Opponents of high-quality teams will then also have to adapt cognitively to this higher playing tempo in order to be competitive in the game.
If we then regard each football match during a season not only as a competitive contest in the rank-order tournament, but also as practise time to improve future team performances, the above results suggest that playing against a high-quality team could also cause a positive externality for a team’s productivity. These positive spillover effects are described in Brown and Minor (Citation2014) as ‘learning-by-doing’ or ‘skill-building’. ‘Skill-building’ then appears, when the physical and cognitive abilities of a team improve from a match against the future champion, and ‘learning-by-doing’, when teams adapt to the playing style and speed of the future champion, which they apply to the next match. The difference between these two explanatory approaches, in this case, is that ‘skill-building’ is independent of a future opponent’s strength, whereas ‘learning-by-doing’ prepares one team to play against another team of strength similar to the future champion. In both cases, the marginal effect from effort devoted to productivity increases, so that teams are willing to put more effort into the next match (Clark and Nilssen Citation2013).
III. Data and descriptive statistics
Data base
In order to test for spillover effects from the future champion in the previous match, I use the results of 93,388 matches played in 22 leagues in Europe extending from the 2005/06 to the 2016/17 season. The leagues I used in this examination include the five leading English ones, the four leading Scottish leagues, the two leading ones in France, Germany, Italy and Spain, and the first leagues in Belgium, Greece, Netherlands, Portugal and Turkey. As I want to investigate spillover effects from the last match, games played on the first match day of a season are excluded from the analysis. To avoid additional spillover and shadow effects from international matches like the UEFA Champions League or Europe League games, matches just before and after these games are also excluded. Rohde and Breuer (Citation2017) show that teams indeed adjust their starting line-up in domestic league matches prior and after European Cup matches. Unfortunately, I do not have data on matches of national cup tournaments, so there might be some bias in the estimation of spillover effects due to these cup games. After these adjustments, there are 78,264 matches left in the study.
For each match, there is also information on the date, on the final results in the previous matches of both teams and on the betting odds. Furthermore, I collected the standings at the end of the season of both the home and away team, and those of their opponent in their last match. All data were extracted from www.football-data.co.uk and www.rsssf.com. To test for possible spillover effects from the future champion of the league, I employ three different variables: one in which the home team had played against the future champion (), a second one in which the away team did so (
) and the last one in which either the home or the away had played against the future champion in its last match (
).Footnote1
At this point, I choose the first place team at the end of the season instead of the current leader of a league to detect a spillover effect, because the season standing reflects the strength of a team better than an current standing. As lower leagues are also part of the investigation, it is not possible to examine spillover effects from a previous league champion, because teams from leagues below the first league get promoted to the next higher league. Furthermore, the choice of the future champion as a high-quality team guarantees that the best team during a season is being picked. In addition, I assume that all teams have knowledge about the strength of each team during the whole season.
Descriptive statistics
To detect possible spillover effects from the future champion, I use four different outcome variables. The first two are the points achieved by the home and by the away team in the specific match.Footnote2 The other two outcome variables are dummy variables which equal one if the home or, respectively, the away team won the match, and zero instead.
In addition, I classify all matches into three groups to control for the inequality of strength of the two teams: one in which the home team is a strong favourite (SHF), one in which the home team is a weak favourite (WHF) and finally, one in which the home team is an underdog or, respectively, the away team the favourite (HU). The classification is based on the betting odds, and assigns a match to the class , if the decimal betting odd on a home win is smaller than 1.7, to the class
if the betting odd is greater than 3.0 and to the class
in all other cases.Footnote3 presents the descriptive statistics of the home and away points, categorized by the three variables
,
and
and the heterogeneity of the two teams.Footnote4
Table 1. Descriptive statistics of selected variables
In the first two rows of , it can be seen that there is no general spillover effect from having played against the future champion, because the points attained by the home and away team are almost identical in all three settings. However, these findings do not control for the inequality of playing strength between the two teams. By controlling for the heterogeneity of the two teams, the results regarding the treatment effect change in all three settings. For example, rows 3–4 in contain matches with a strong home favourite and show that on average, a home team achieves 0.16 less points (2.05 points compared to 2.21 points) if it has played against the future champion team in its last match. It is then obvious that the effects on the away points are the opposite of the effect on the home points, as fewer points for the home team typically lead to more points for the away team. Interestingly, the effect is quite the opposite if the away team had the future champion as its last opponent. Being an underdog in this match, the away team attains 0.08 more points (0.67 to 0.59) after such a match. It is then not surprising that the effect on the group is similar to the other two ones, because the latter are subgroups that form the group
.
If we take a look at the matches in which the home team is the underdog, we can see that the effects on the home and away points are similar to those with the home team being a strong favourite, because the underdog (in this case the home team) gains 0.12 more points on average if it had played against the future winner in the match prior to the observed one. In contrast, we observe that the favourite away team achieves 0.09 less points after a match against the future champion. Again, the effects in the groups () and (
) on the home and on the away points are almost the same, so that the effect in the group
is similar.
Unlike matches with a strong home favourite or home underdog, there seems to be no effect from high-competition in matches with a weak home favourite, irrespective of whether the home or away team had played against the future champion. The descriptive statistics regarding the outcome variables of a home and away win in in the Appendix also show the same effects described in this section.
As the effects of the home or away team having played against the future champion seem to be similar in cases of being a favourite or underdog in the observed match, it is possible to create three new variables. equals one for matches in which the home team is a strong home favourite and the away team had played against the future champion, or if the home team is the current underdog and it has played against the future champion. The variable
is defined similarly, but with the difference that the team that has played against the future champion is either a strong favourite (home team) or a favourite (away team). Then,
equals one for all other matches in which either the home or away team had played against the future champion. shows the descriptive statistics regarding the circumstance of having played against the future champion, split by the new three variables.
Table 2. Descriptive statistics of three new variables
The results from the descriptive statistics above suggest that there are negative spillover effects for favourites from having played against the future champion, as these teams gain 0.13 less points on average in their current match. By contrast, underdogs seem to benefit from a match against the future champion, as they achieve 0.09 more points on average. In matches with teams of similar strength, there tend to be no spillover effects.
Control variables
Two different variables are employed in this analysis to control for the playing abilities of the home and the away team. First, I use the position of both teams at the end of the last season as a predictor of the strength of these teams. I do not take the standing at the end of the current season, because there could be an endogeneity problem, as the outcome of a specific match is correlated with the ranking at the end of a season. Teams that entered the league in the season are labelled as either relegated or promoted.
Second, I utilize betting odds as another way to control for the playing abilities of the teams in a match. Betting odds are one of the most accurate predictors of match results, as betting odds contain, among other things, information on the overall strength of both teams, the current form of both teams, the availability of players (some players might be injured or suspended) and home-field advantage (e.g. Forrest, Goddard, and Simmons Citation2005; Spann and Skiera Citation2009). Furthermore, betting odds also reflect information about incentives and the expected effort made by both teams.
However, betting odds have the disadvantage that information on the quality of the last opponent might be already contained in the betting odd in itself, so that it would be not possible to detect a spillover effect. Therefore, I assume at this point that the bookmaker does not take into account the effect from high-quality opposition in the last match and therefore misvalues the betting odds. This assumption can be justified, because it is usually not known which team is going to win the championship at the end of the season, so that the bookmaker cannot use this information.
IV. Estimation strategy
In order to identify an effect of having played against the future champion (treatment effect) empirically, I choose an exact matching model. This method matches each treated game to all possible games in the control group, with exactly the same values of the covariates (Ho et al. Citation2011). A treatment effect can then be calculated by comparing the weighted mean of the outcome variables in the treatment and control groups (Ho et al. Citation2007). The means are weighted, because there are more observations in the control than in the treatment group.
As covariates in the matching model, I use the position of both teams at the end of the last season and the league in which the game is played. Basically, the matching algorithm in this model searches, for each treated game, other games from the same league (though typically played in another season), in which the positions at the end of the last season of the home and the away teams are the same.Footnote5 For example, in a league of six teams over a two-year span with a double round-robin structure, the place team of last season has played against the league champion in its last match and is now playing against last season’s
place team at home (treated match). Then, a match in the control group with this treated match is a game played in a different season in which last season’s
place team plays against last season’s
place team at home (both teams might be different), and the
place team has not played against the league champion immediately prior to this match.
At this point, I choose an exact matching model, rather than a propensity score matching model, because an exact matching model has the advantage that there is an automatic balance of covariates in the treated and control group. One of the disadvantages though, is that some treated games might be not be matched, as there is no game in the control group with the identical covariates (Ho et al. Citation2007). However, the rich dataset and especially having data on 12 different seasons enable me to find matches within the same league for almost each match in the treatment group, so that it is possible to apply this matching method. Note that the results from the exact matching method can also be replicated in regression adjustment, if the covariates are fully interacted (which is possible, as all covariates are discrete).
V. Empirical results
Existence of spillover effects
Applying the matching method presented in the previous section, shows the main results of this paper, namely the average effect of having played against the future champion in three different settings on two outcome variables (points gained and wins).Footnote6
Table 3. Spillover effects from the future champion with heterogeneous teams
The results from strongly support the existence of spillover effects from future champions with heterogeneous players. Favourite teams gain 0.11 less points after their match against the future champion, whereby the same situation leads to 0.10 more points for underdogs. Then, teams might gain (or lose) 0.21 points on average, if they are an underdog (favourite) instead of a favourite (underdog) in the current match. As the order of play in a double round-robin league with a balanced schedule is usually the same in the first and second half of a season, the spillover effect from the future champion can be up to 0.84 points on average over the course of a season, depending on if a team is the favourite or underdog in the matches after the one against the future champion.Footnote7 The effect may be even larger for some teams in a league with an unbalanced schedule, as these teams receive more indirect spillover effects (carry-over effects) from the league champion. By differentiating between home and away teams that had played against the future champion of the league, it seems that the results are driven by treated home teams, as the average treatment effect gets insignificant for away teams (see in the Appendix).
Origins of the spillover effects
As discussed in Section 2, several causes might explain the spillover effects. Favourite teams might be exhausted after their match, as they put more effort and preparation into their match against the future winner, and less into the next match in which it is a favourite (shadow effect), or there are negative externalities as the match against the future champion does not prepare the team quite so well for a match against a much weaker competition. In contrast, underdogs might benefit from positive externalities as the playing styles in both matches are similar, or they self-restricted their efforts in the match against the future number one team in order to save resources for the next match. In the remainder of this section, I will attempt to identify the origins of these spillover effects.
In contrast to the previous estimation, I will use new variables defining a team as a favourite or an underdog, so as to avoid selection distortions, as the classification based on the betting odds, calculated after the match against the future champion, are affected by the result of this match, among others. As a consequence, there might be an endogeneity problem between the points gained against the future champion and the variable or
. Instead, I split teams from each league into three groups, based on their positions at the end of the last season. A favourite is then a team from Group 1 (top third last season) competing at home against a team from Group 2 (middle third) or Group 3 (bottom third), or against a team from Group 3 away.Footnote8 An underdog is defined as a team from Group 3 competing against a Group 1 team (home or away).
presents the results regarding the new two variables and
. Again, the existence of spillover effects is confirmed. Furthermore, I split the season into two parts in order to test whether the spillover effects are present in all periods of a season. As a football season usually starts in July and ends in June, the periods (July till February) and (March till June) are chosen to check if the spillover effect still exists at the end of a season. The results show that the spillover effects are larger at the beginning and middle of a season and become insignificant at the end of a season. Given these results in this section, I will exclude matches played between March and July. The possible origins of this period effect are discussed at the end of this section.
Table 4. Spillover effects on favourites and underdogs
I use the following estimation strategy to identify possible shadow effects which could explain the observed spillover effects in the next match. First, I only take a look at matches with teams from Group 1 competing against the future champion and use this sample to match games with teams which are the favourite in the next match (treatment group) to games in which the Group 1 team is not the favourite (control group). The matching variables are the position at the end of the last season of the observed team, the division and the location of the match (home or away). Then I compare the weighted means of points against the future champion in the two settings. If there are no shadow effects involved, there should be no significant differences.
In a second estimation, I use a new matching model to calculate the additional spillover effects of being a favourite. In order to do this, I match games in which a team from Group 1 had played against the future champion with games in which a team from Group 1 is involved that had not played against the future champion in its last match. Then, I include the two treatment variables and
in the regression model,Footnote9 in which the estimator of the variable
captures the effect on a team from Group 1 having played against the future champion and not being a favourite. In contrast to the estimation of , the estimator
measures the additional spillover effect on a team from Group 1 being a favourite, compared to a team from Group 1 which is not the favourite. This has the advantage that the variable
in both estimations identifies the effect of being a favourite, so that it is easier to compare and interpret them. The approach of detecting shadow effects of underdogs is similar.
The results from show that there are also shadow effects for favourites, because a team from Group 1 achieves 0.12 more points against the future champion if it is the favourite team in the next match. On the other hand, it achieves 0.11 fewer points in the upcoming match, compared to a team from the same group which had also played against the future champion, but is not a favourite in the current match. This is a strong indication that high-quality teams put more effort into the current match against the future champion if they have weaker competition in the next match.Footnote10 This could explain the negative spillover effects for favourites, because they might be still exhausted from their match against the future champion, and thus put less effort and preparation into the next match, as their marginal cost of effort increased. These findings are in line with those from Brown and Minor (Citation2014), who find that tennis players perform worse after increased exertion in the past. Note that these negative spillover effects are also only significant at a 10% level, if the time between these two matches is less than 15 days. In contrast to the estimation in , the addition of the variable increases the standard error of the variable
in this case.
Table 5. Shadow and spillover effects on favourites and underdogs
Furthermore, the results regarding shadow and spillover effects for favourites also show that teams from Group 1 (the high-quality teams of a league) are indeed forward-looking. By taking into account the strength of the next opponent, these teams exert excess effort in the match against the future champion if they are the favourite in the upcoming match. Although the sum of the shadow and spillover effect is almost zero, and assuming there are no further externalities for favourites, the strategy of distributing effort among the two matches is beneficial for a team from Group 1, as points gained against a potential competitor (like the future champion or other teams from Group 1) are more valuable than points against a team from Group 2 or 3 in the championship fight. One can also assume that teams are aware that a higher effort in the present match results in negative spillover effects in the next match, because otherwise, these teams would always choose a higher effort in the match against the future champion (regardless of the next opponent’s strength).
On the other hand, the results from show no significant shadow effect for underdogs from Group 3, so that teams from this group perform neither better nor worse against the future champion, if they are an underdog in their next match. However, it could also be the case that in general, teams from Group 3 self-restrict (‘discouragement effect’) in the match against the future champion in order to increase the probability of winning in the next match (independent of the future opponent’s strength). If this theory were true, all teams from Group 3 would then receive a positive spillover effect in their next match, but this is not supported by the data, as there is only a significant spillover effect for teams from Group 3 competing against Group 1 teams.
This also contradicts the theoretical findings of Deutscher et al. (Citation2019). As the inequality of strength is large in the match against the future champion, and comparatively low in the next match for teams from Group 3 which are not an underdog, their model predicts a lower effort level of these teams (negative shadow effects) and a higher effort in the next match with an opponent of more equal strength. This would lead to a positive estimator of the variable in estimation (1) of and also a positive one for
in estimation (2). However, both estimators are not significant, so that the estimations do not support their theory.
Then, these results regarding underdogs suggest that the positive spillovers are explained by externalizations from the match against the future champion. Playing twice in a row against high-quality teams seems to have a positive effect on the outcome in the second match, because teams can adapt to this playing style and learn from this match, what it takes to win in these games. The positive externalities are then ‘learning-by-doing’ effects rather than ‘skill-building’, because the effects are dependent of a future opponent’s strength. This theory is supported by results from another estimation in which an underdog is defined as a Group 3 team competing against a Group 1 team or against a Group 2 team away (the definition is similar to that of favourites). However, through this expansion of the definition of an underdog, the results change in comparison to those in , as all effects become insignificant (see in the Appendix).
Following the theory about positive externalities, lower-skilled teams should benefit more from a match against the future champion, as their playing ability is lower in general, so that their level of improvement is greater than that of higher-skilled teams. One way to test this theory is by comparing the effects in environments with different skill levels of teams, for example, teams in the first league compared to those in lower leagues. Then, the positive spillover effect should be greater in lower leagues, as the overall skill level is lower. By contrast, the negative spillover effect caused by greater effort in the last match is expected not to be dependent on the quality of a team, as the choice of distributing effort among different matches is assumed to be independent on the league affiliation. presents the corresponding average treatment effects on the points, separated by first and lower leagues. It can be seen that the positive spillover effects for underdogs are only significant in lower leagues, whereas the negative spillover effects for favourites are significant in first as well as in lower leagues. These results support the proposed explanations of different causes of spillover effects.
Table 6. Spillover effects on favourites and underdogs split by league affiliation
As described, the spillover effects after a match against high-competition are probably caused by ‘learning-by-doing’ for underdogs (positive) and by exhaustion and lack of preparation for favourites (negative). Accordingly, these explanations should only influence the outcome of games in the short term, so that there is no expectation of a long-term effect. To test whether the opposition effect is temporary or long term, I determine whether the days between the two matches influence the spillover effect. For games with a long break after the match against the future winner, the magnitude of the effect should be smaller. The results in support this hypothesis, as the effect size decreases with longer rest periods for both spillover effects. The effect for underdogs, for example, is only significant at a 10% level for matches with equal or less than 4 days of recovery time. The results in also explain why the shadow and spillover effects from are only significant at a critical maximum number of rest days, because the effects become insignificant after a certain time.
Table 7. Spillover effects on favourites and underdogs split by rest days
Finally, I discuss the results from , which show that the spillover effects are only significant in the period July – February, and not in the last third of a season. The results of Mohr, Krustrop, and Bangsbo (Citation2003) and Rampini et al. (Citation2007) indicate that soccer players’ physical shape is better at the end of a season than at the start and that their physical performance in a match is higher against better competition. If we additionally assume that the increase in physical and cognitive ability of a player, and consequently the ‘learning-by-doing’ effects decline over the duration of a season, this would suggest that the positive spillover effects are larger at the start of a season than at the end, which is confirmed in the data, as the effect becomes insignificant at the end of a season.
Favourites, on the other hand, seem to change their strategy regarding distributing effort among different periods at the end of a season. in the Appendix shows the results for additional shadow and spillover effects on favourites in the period . It can be seen that there are significant negative shadow effects on favourites in the last third of a season, which indicates a lower effort level in the match against the future champion, if the team is a favourite in the next match. Teams might believe at the end of a season that it is beneficial to save more resources for the match in which one is a favourite. As discussed in Section 2, this could occur, when teams already dropped out of the championship race prior to the match against the future champion. However, this strategy does not lead to significantly more points in the next match, as the estimator
is insignificant. As a consequence, teams should either change their strategy, as it is not profitable, or there are also additional negative externalizations involved in this period.
VI. Robustness check
The results presented in the previous section demonstrate the existence of spillover effects from future champions in European football. Following Fama (Citation1970), betting markets can be considered as information markets that yield to market prices which contain all available information at a certain time. If this statement were true, then betting odds should also reflect spillover effects. However, as a high-competition team is defined in this study as the future champion of a league, not all information is available to the betting company when the betting odds are calculated.
One advantage of betting odds though, is that they usually reflect more information than a standard econometric analysis. Among others, they contain information on players being injured or suspended, current winning or losing streaks or coach dismissals, which might all influence the outcome of a match, so that betting odds are good predictors of outcomes of a match. Therefore, I treat the empirical study in this section as a robustness check of the existence of spillover effects, and not as an additional analysis which explores whether spillover effects are incorporated into betting odds. To do so, I use a logit regression which estimates the following regression equation.
Basically, a logit regression estimates the impact of a set of exogenous variables on the logarithm of the odds that a certain event occurs. The regression in this paper utilizes a logit model, for which the endogenous variable
is binary and takes a value of one if the home team won the match
in league
in season
. In addition, I use the logit value of the inverse of the betting odd as a control variable, because the logit function gives the corresponding logarithm of the odds
. In this case
is the probability of the home team winning, according to the bookmaker, and therefore the inverse of the betting odd.
The variable is again a dummy variable that equals one if one of the two teams had played against the future champion of the league in its last match, and zero instead. Furthermore, there is an interaction term in this model to test for the simultaneous effect of having played against the future champion and the heterogeneity of the two teams. In contrast to the estimation models without betting odds in the previous section, it is easier to control for the inequality of strength or, respectively, a team being a favourite or an underdog, given that a high and positive value of the logit represents a strong home favourite and opposed to a large negative value for home underdog. Note that the value of the logit is zero if and only if the betting odd is two (or the corresponding winning probability according to the bookmaker is 0.5).
Following the results from the previous section, we would expect the estimator of the variable
to be zero (no expected spillover effect at a match with a weak home favourite) and the estimator
of the interaction term to be negative, as the situation of one of the two teams having played against the future champion in its last match is expected to decrease the winning probability of the home team, with it being a strong favourite (positive logit value) and to increase with the home team being the underdog (negative logit value).
The following shows the estimates of the regression presented in EquationEquation (2)(2)
(2) . Regression (1) only estimates the effect of the log odds and of the variable
as explanatory variables on the probability of a home win, whereas regression (2) includes the interaction term of the log odds with the variable
. Regression (3) also controls for season and league fixed effects.
Table 8. Regression results of spillover effects on home wins
The results in confirm the findings from the previous section on the existence of spillover effects. Although there is no general significant spillover effect, because the estimator of the variable is insignificant as predicted, but there are significant spillover effects for favourites (negative) and underdogs (positive). If the home team, for example, had played against the future winner and is a favourite, the logit value is positive, so that the effect becomes negative, due to the negative estimator of the interaction term. On the other hand, if the away team is the favourite after having played against the future champion, the logit value is negative, and combined with the negative estimator of the interaction term, this leads to a positive effect on the probability of a home win and consequently, in a negative effect on the away team. The argumentation on the effect on underdogs is similar and results in positive spillover effects. The results from this regression also indicate that the spillover effects become stronger with an increasing heterogeneity of the two teams (higher negative or positive values of the logit). Two robustness checks, in which the first regression uses the away win as an endogenous variable and the second one additional season-league effects, also confirm the conclusions on spillover effects in this paper (see and in the Appendix).
VII. Conclusion
The main aim of this paper is to detect possible spillover effects from high-competition in a previous match. Using data from 78,264 matches in professional European football, I find that favourite teams perform significantly worse after having played against the future champion of their league. The results of an additional analysis on shadow effects in the match against the champion show that teams perform significantly better in these games if they are the favourite in the next match. This suggests that the negative spillover effects can be explained by a higher effort in the previous match and hence by exhaustion and less preparation in the current match.
On the other hand, the performance of underdogs is significantly better after a match against high-competition. As no significant shadow effects are found in the data, the positive spillover effects might be explained by ‘learning-by-doing’ effects, as teams are better prepared by competing against a high-quality team. This suggestion is reinforced by results from another estimation that lower-skilled underdogs benefit more from such a match, as their potential for improvement is greater.
One of the shortcomings of this paper is that it only focuses on spillover effects from national league matches, and not from national or international cup tournaments. Future research could investigate whether shadow and spillover effects are also observable from matches played outside the domestic league on the outcome of matches in the national league. Furthermore, future work could use a different variable to define the best team of a league, such as the champion from the previous season or the team with the highest market value before the season, to test whether the observed spillover effects also occur with these definitions.
Given the findings of this paper and assuming there is valid information as to which team will win the league title, coaches of teams that had played previously against the future champion and are the favourite in the present match should change their starting line up in the upcoming match, so as to avoid negative spillover effects caused by exhaustion or lack of preparation. On the other hand, coaches should keep their starting line-up from the match against the future champion to exploit the full potential of ‘learning-by-doing’ effects, if they are an underdog in the current match.
In addition, decision-makers of scheduling should focus on a balanced schedule in such a way that indirect spillover effects (carry-over effects) from the same team are minimized for each team, so that teams do not acquire either an advantage or disadvantage through spillover effects caused by the opposition. Goossens and Spieksma (Citation2012b), however, show that quite a few leagues in Europe still employ a canonical schedule which basically maximizes carry-over effects received from one team, instead of minimizing it.Footnote11 Some researchers try to find schedules that balance carry-over effects in round-robin tournaments (e.g. Russel Citation1980; Anderson Citation1999). Applying these scheduling methods would then improve the fairness of a competition for all teams participating. Furthermore, a league could also eliminate matches on midweek, as the spillover effects become stronger with fewer rest days for the teams.
Disclosure statement
No potential conflict of interest was reported by the author.
Notes
1 There are 29 matches, in which the home team and the away team both had played against the future winner in their respective league. To avoid a second spillover effect, these games are dropped from the group First Place.
2 A team obtains three points for a win, one for a draw and zero for a loss.
3 These frontier values were chosen, because the average betting odd with one team having played against the future winner is 2.35. Then, all matches within 0.6 of the standard deviation (1.04) from the mean were categorized into the class and the other ones to
or respectively
.
4 The descriptive statistics regarding wins, draws and losses are in in the appendix.
5 Four leagues changed their league size during the examined time period. However, as this increase or decrease was only of two teams in each league, this should not affect the estimation.
6 I used R statistical software to run all matching and regressions, using the packages MatchIt (Ho et al. Citation2011) and stargazer (Hlavac Citation2018).
7 In a balanced schedule, each team also receives two times indirect spillover effects (carry-over effects) from the future champion. Due to the small size of the Scottish League, clubs play each other more than twice a year, so that the overall spillover effect (direct and indirect) might be even greater there.
8 Teams that got relegated last season are in Group 1 and promoted teams in Group 3.
9 is a dummy variable that equals one, if a team from Group 1 had played against the future champion in its last match, and zero instead.
10 Unfortunately, 131 games out of 989 were dropped in the matching model aimed at finding a shadow effect for favourites. To avoid possible bias due to a smaller sample size, I used a “nearest-neighbour matching model’’ (propensity score matching) to check whether the results are robust. The findings of this estimation confirm the results of Table 5, as the estimator is still highly significant, as shown in Table A3 in the appendix.
11 For example, in the 2006 season in the highest division in Belgium, RAEC Mons had to play against the future winner’s previous opponent 29 times out of 32. It should be noted that some leagues abandoned this scheduling system, like the first leagues in Belgium in 2007 or in Germany, due to the unfairness.
References
- Altmann, S., A. Falk, and M. Wibral. 2012. “Promotions and Incentives: The Case of Multi-stage Elimination Tournaments.” Journal of Labour Economics 30: 149–174. doi:10.1086/662130.
- Anderson, I. 1999. “Balancing Carry-over Effects in Tournaments.” In Research Notes in Mathematics: Vol. 403. Combinatorial Designs and Their Applications, edited by F. Holroyd, K. Quinn, C. Rowley, and B. Webb, 1–16. London: Chapman & Hall/CRC.
- Bach, N., O. Gürtler, and J. Prinz. 2009. “Incentive Effects in Tournaments with Heterogeneous Competitors - an Analysis of the Olympic Rowing Regatta in Sydney 2000, Management Revue.” The International Review of Management Studies 20: 239–253.
- Brown, J. 2011. “Quitters Never Win: The (Adverse) Incentive Effects of Competing with Superstars.” Journal of Political Economy 119 (5): 982–1013. doi:10.1086/663306.
- Brown, J., and D. Minor. 2014. “Selecting the Best? Spillover and Shadows in Elimination Tournaments.” Management Science 60 (12): 3087–3102. doi:10.1287/mnsc.2014.2014.
- Casas, A., and Y. Fawaz. 2016. “Altitude as Handicap in Rank-order Football Tournaments.” Applied Economics Letters 23 (3): 180–183. doi:10.1080/13504851.2015.1061640.
- Clark, D., and T. Nilssen. 2013. “Learning by Doing in Contests.” Public Choice 156 (1–2): 329–343. doi:10.1007/s11127-011-9905-9.
- Deutscher, C., M. Sahm, S. Schneemann, and H. Sonnabend. 2019. “Strategic Investment Decisions in Multi-stage Contests with Heterogeneous Players.” CESifo Working Papers, No. 7474.
- Dilger, A., and L. Vischer. 2020. “No Home Bias in Ghost Games.” Discussion Paper of the Institute for Organisational Economics 7/2020.
- Fama, E. F. 1970. “Efficient Capital Markets: A Review of Theory and Empirical Work.” The Journal of Finance 25 (2): 383–417. doi:10.2307/2325486.
- Fischer, K., and J. Haucap. 2020. “Does Crowd Support Drive the Home Advantage in Professional Soccer? Evidence from German Ghost Games during the COVID-19 Pandemic.” DICE Discussion Paper, No. 344.
- Forrest, D., J. Goddard, and R. Simmons. 2005. “Odds-setters as Forecasters: The Case of English Football.” International Journal of Forecasting 21 (3): 551–564. doi:10.1016/j.ijforecast.2005.03.003.
- Goossens, D., and F. Spieksma. 2012a. “The Carryover Effect Does Not Influence Football Results.” Journal of Sports Economics 13 (3): 288–305. doi:10.1177/1527002511402932.
- Goossens, D., and F. Spieksma. 2012b. “Soccer Schedules in Europe: An Overview.” Journal of Scheduling 15 (5): 641–651. doi:10.1007/s10951-011-0238-9.
- Hill, B. 2018. “Shadow and Spillover Effects of Competition in NBA Playoffs.” Journal of Sports Economics 19 (8): 1067–1092. doi:10.1177/1527002517704020.
- Hlavac, M. 2018. “Stargazer: Well-Formatted Regression and Summary Statistics Tables.” https://cran.r-project.org/web/packages/stargazer/index.html, R package version 5.2.2
- Ho, D. E., K. Imai, G. King, and E. A. Stuart. 2007. “Matching as Nonparametric Preprocessing for Reducing Model Dependence in Parametric Causal Inference.” Political Analysis 15 (3): 199–236. doi:10.1093/pan/mpl013.
- Ho, D. E., K. Imai, G. King, and E. A. Stuart. 2011. “MatchIt: Nonparametric Preprocessing for Parametric Causal Inference.” Journal of Statistical Software 42 (8): 1–28. doi:10.18637/jss.v042.i08.
- Konrad, K. 2009. Strategy and Dynamics in Contests. New York: Oxford University Press.
- Lackner, M., R. Stracke, U. Sunde, and R. Winter-Ebmer. 2015. “Are Competitors Forward Looking in Strategic Interactions? Evidence from the Field.” IZA Discussion Paper, No. 9564.
- Lago, C., L. Casais, and J. Sampaio. 2010. “The Effects of Situational Variables on Distance Covered at Various Speeds in Elite Soccer.” European Journal of Sport Science 10 (2): 103–109. doi:10.1080/17461390903273994.
- Mohr, M., P. Krustrop, and J. Bangsbo. 2003. “Match Performance of High-standard Soccer Players with Special Reference to Development of Fatigue.” Journal of Sports Sciences 21: 519–528. doi:10.1080/0264041031000071182.
- Pettersson-Lidbom, P., and M. Priks. 2010. “Behavior under Social Pressure: Empty Italian Stadiums and Referee Bias.” Economics Letters 108 (2): 212–214. doi:10.1016/j.econlet.2010.04.023.
- Pope, D. G., and M. E. Schweitzer. 2011. “Is Tiger Woods Loss Averse? Persistent Bias in the Face of Experience, Competition, and High Stakes.” American Economic Review 101 (1): 129–157. doi:10.1257/aer.101.1.129.
- Rampini, E., A. J. Coutts, C. Castagna, R. Sassi, and F. M. Impellizzeri. 2007. “Variations in Top Level Soccer Match Performance.” International Journal of Sports Medicine 28: 1018–1024.
- Rohde, M., and C. Breuer. 2017. “Financial Incentives and Strategic Behavior in European Professional Football: A Match Day Analysis of Starting Squads in the German Bundesliga and UEFA Competitions.” International Journal of Sport Finance 12: 160–182.
- Rosen, S. 1986. “Prices and Incentives in Elimination Tournaments.” American Economic Review 76: 921–939.
- Russel, K. 1980. “Balancing Carry-over Effects in Round Robin Tournaments.” Biometrika 67 (1): 127–131. doi:10.1093/biomet/67.1.127.
- Ryvkin, D. 2011. “Fatigue in Dynamic Tournaments.” Journal of Economics & Management Strategy 20 (4): 1011–1041. doi:10.1111/j.1530-9134.2011.00314.x.
- Spann, M., and B. Skiera. 2009. “Sports Forecasting: A Comparison of the Forecast Accuracy of Prediction Markets, Betting Odds and Tipsters.” Journal of Forecasting 28 (1): 55–72. doi:10.1002/for.1091.
- Sunde, U. 2009. “Heterogeneity and Performance in Tournaments: A Test for Incentive Effects Using Professional Tennis Data.” Applied Economics 41 (25): 3199–3208. doi:10.1080/00036840802243789.
Appendix
Table A1. Descriptive statistics of selected variables in three treatments
Table A2. Spillover effects from future champions on home and away teams
Table A3. Shadow effects on favourites using another matching method
Table A4. Spillover effects on underdogs
Table A5. Shadow and spillover effects on favourites and underdogs in the period
Table A6. Regression results of spillover effects on away wins
Table A7. Regression results of spillover effects on home wins with season-league effects