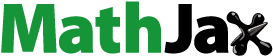
ABSTRACT
This study investigates the asymmetric impacts of oil price and component shocks on inflation in the context of BRICS countries. We firstly decompose the oil price change into supply, demand and risk shocks and subsequently establish an empirical framework to explore asymmetric pass-through using a novel multiple threshold nonlinear autoregressive distributed lag model (MTNARDL). The extended model yields precise asymmetric estimates by splitting oil price change and disaggregated shocks into different partial sums. Our results reveal that significant asymmetries between oil price and inflation are only exhibited in China in the short run, suggests that inflationary effect is more dramatic when oil price decreases. For the supply shocks, strong asymmetries are found in Russia and China in the short term and in South Africa in the long term. Meanwhile demand shocks and risk shocks hold either nil asymmetry or weak, in most cases risk shocks have the weakest effect on inflation and the influence fading fast, demand shocks only exert impact temporarily during extreme fluctuation. Nonetheless, asymmetries are most important and significant at the highest and lowest quintiles. Considering the empirical results, this study provides some policy implications for policymakers and investors.
1. Introduction
Although the use of renewable energy is increasingly valued worldwide, non-renewable energy still dominates the global economy. As a significant contributor to essentially all economic activities, crude oil price fluctuations also play an indispensable role in inflation (Prat and Uctum Citation2011). Although the nexus between oil price and inflation has been investigated for decades, the measured outcomes have yielded mixed and inconsistent results (Kilic and Cankaya Citation2019). Today, it is generally acknowledged that crude oil price variations pass through to inflation at least in part (Edelstein and Kilian Citation2009).
Regarding the impact of oil prices on inflation, the first and most intuitive perception is that oil is a critical industrial raw material, and an increase in the oil price will directly cause the production price index (PPI) to rise, driving up the prices of other production factors and the consumer price index (CPI) in commodity markets. These changes ultimately affect the prices of production materials, wages, and products (Hamilton Citation1983). Traditional economics calls this cost-driven inflation, oil price raise will impose upward pressure on inflation. However, a considerable amount of literature has emphasized that oil price changes have indirect effects on economic growth, consumption expenditure, and unemployment, which may further limit inflation (Bresnahan and Ramey Citation1993; Davis and Haltiwanger Citation2001; Lee and Ni Citation2002). For instance, the high cost of living and uncertainty brought about by oil prices lower the discretionary income of households, contract consumption expenditure, decelerate economic growth and increase unemployment. The Phillips curve tells us that an increase in the unemployment rate reduces inflation. Generally, much literature considers oil price change have both an income and a production cost effect (Hamilton Citation1983; Bohi Citation1991; Hamilton Citation2003; Kilian Citation2008). More specifically, Edelstein and Kilian (Citation2009) and Pal and Mitra (Citation2019) concluded that there are five mechanisms through which oil price changes directly influence inflation, that is, discretionary income effect, uncertainty effect, precautionary savings effect, operating cost effect and reallocation effects, these studies proposed that primarily macroeconomic aggregates would show asymmetric reactions because of different effects. But so far, the transmission of oil prices to inflation has no unified conclusion, the aforementioned effects do not apply to all economies.
Much of the related literature pays attention to changes in oil prices, usually uses level value or the first log-difference, when exploring the linkages between oil price and macroeconomic variables. However, since Kilian (Citation2009), which disentangle oil price into three shocks, the notion of identified different shocks has received completely investigations and understandings. In particularly, based on the causes of oil price fluctuations, Kilian (Citation2009) defined three shocks, namely the supply, aggregate demand and precautionary demand (also called oil specific-demand) shocks by constructing a three variables Structural Vector Autoregressive (SVAR) model, crude oil production and global real economic activity index (which calculated by dry cargo shipping rates) are choosing as proxies for oil supply and demand. However, this classical approach cannot identify changes in precautionary demand are driven by aggregate demand for oil or concerns about future oil supply (Ready Citation2018; Demirer, Ferrer, and Shahzad Citation2020), and the freight rates used in the model reflects aggregate demand with a lag (Anand. and Paul Citation2021). To overcome these weaknesses, Ready (Citation2018) proposed asset price into SVAR and redefined supply, demand and risk shocks. To sum up, while investigating the nexus between oil price and CPI, we simultaneously explore the plausible asymmetric impact of the disaggregated oil price shocks on inflation by using method propose by Ready (Citation2018).
The BRICS, namely Brazil, Russia, India, China, and South Africa are the world’s large emerging economies, and they are experiencing rapid growth. Will international oil price change and component shocks have a spillover effect on their domestic inflation? Is the pass-through between inflation and oil price asymmetric? This study provides some empirical results by applying a novel multiple threshold nonlinear autoregressive distributed lag (MTNARDL) model. The closest study to this is that of Pal and Mitra (Citation2019), however, not only do we analyse the integral oil price, we further estimate the decomposed shocks’ asymmetric impact.
This study contributes to the existing literature in three ways. First, it investigates the asymmetric transmission from both oil price and disaggregated shocks to inflation. Prior studies have often focused on oil price changes but neglected these disaggregated shocks, we extend literature by introducing a novel reasonable decompose method proposed by Ready (Citation2018). Second, multiple threshold models distinguish small to large fluctuations in oil price changes and capture the response of inflation with a more prominent exactness than traditional nonlinear autoregressive distributed lag (NARDL) models, which only discern oil price fluctuations. To the best of our knowledge, this study is the first to use these models examining asymmetry in the context of BRICS. Finally, our outcomes are valuable for policymakers who deal with domestic inflation and investors who are building a portfolio of crude oil with other assets for both short and long-term interests.
The remainder of the article is organized as follows. Section 2 briefly reviews the literature on the oil price change, disentangle shocks and inflation nexus. In Sections 3 and 4, we discuss the methodology and data employed in our study. Empirical findings and discussions are presented in Section 5, conclusions are drawn in Section 6.
2. Literature review
Over recent decades, a considerable amount of literature has been published on the pass-through of oil price to inflation. For example, the earliest Hamilton (Citation1983) explored the impact of oil prices on the US macroeconomy, including price levels, and found that oil price shock was one of the reasons for the economic recession in the United States following the Second World War. In a similar line, Hooker (Citation1999) suggested that significant oil price effects on the macroeconomy are indirect and transferred through inflation and interest rates. Cologni and Manera (Citation2008) constructed a structural cointegrated VAR model to examine the influence of inflation on oil price for G7 countries and found an inflationary impact of oil prices except in Japan and the United Kingdom. In the context of the Euro zone, Jacquinot et al. (Citation2009) showed that fluctuations in oil price are indispensably significant for the comprehension of short-term inflation based on the dynamic stochastic general equilibrium (DSGE) model. Similarly, Sek, Teo, and Wong (Citation2015) divided 20 countries into two groups and revealed that oil price directly affected inflation in the low oil-dependency group but indirectly affected the high group. Recently, in the context of Gulf Cooperation Council member countries, Nasir et al. (Citation2019) found positive and significant spillover effects from oil price to inflation.
Nevertheless, there are many scholars who believe that the impact of oil prices on inflation is declining or refuse to admit the major spillover effect from oil price to inflation. For instance, using data from 1962 to 2000, Hooker (Citation2002) indicated that the contribution of oil prices to inflation in the United States has been negligible since 1981. In the same vein, Leblanc and Chinn (Citation2004) estimated the transmission of oil price changes to inflation based on G5 countries using an augmented Phillips curve framework. The results suggested only a modest and weaker effect imposed by oil price. According to De Gregorio et al. (Citation2007), oil price hikes had a reduced impact on price levels compared with previous oil shocks. Working on 19 industrial countries with quarterly datasets spanning from 1970 to 2006, Chen (Citation2009) also found recent declining contagion from oil price shocks to inflation. Gómez-loscos, Gadea, and Montañés (Citation2012) studied G7 countries and observed that the response of inflation to oil price progressively disappeared until the late 1990s. Similarly, Gao, Kim, and Saba (Citation2014) revealed that oil price changes only significantly affect energy-intensive CPIs while influence on most other CPIs are limited, for instance, beverages, food, housing, apparel, and medical care. More recently, Tiwari et al. (Citation2019) selected a wavelet coherency analysis and argued that from January 1871 to June 2018, the oil price-inflation spillover effect has obviously decreased. Similar small or limited effects of oil price on inflation have been reported in many countries, such as Spain (De Gregorio et al. Citation2007), Thailand (Jiranyakul Citation2019) and China (Chen, Zhu, and Li Citation2020).
After Kilian’s (Citation2009) pioneering decomposition method for oil price, there are some studies focusing on relation between identified shocks and inflation. Peersman and Van Robays (Citation2009) early demonstrated that inflationary effects in the Euro area is substantially motivated by supply and aggregate demand shocks. Combine with DSGE and Kilian’s approach, Zhao et al. (Citation2016) found that oil supply shocks only affect China’s inflation in the short-term and demand shocks contribute the most to the inflation. In the same vein, Gong and Lin (Citation2018) carried out that there are significant different impacts on China’s inflation between oil supply and demand shocks. Recently, in the case of G7 countries, Wen, Zhang, and Gong (Citation2021) conducted different response of each sample countries to three type of shocks. Using decompose method proposed by Ready (Citation2018), they examined the dynamic effects of shocks through rolling-window analysis and found risk shocks mainly work during crisis events, such as global financial crisis and European debt crisis.
Although the link between oil price (or component shocks) and inflation has been investigated extensively, the results are far from uniform. One reason is that many scholars hold the symmetric assumption of two variables, but, in reality, deviation is more typical. As discussed in Hamilton (Citation2003, Citation2011), a nonlinear modelling approach would be preferable. Among recent scholarly investigations, a growing body of literature has investigated the asymmetric effect of oil price on inflation. Ibrahim (Citation2015) used NARDL to estimate the asymmetric relationship between oil and food prices, noting that in the long run, there is a significant relation when the oil price increases, but the relation is absent for an oil price decline. To examine the difference between oil importing and exporting countries, Salisu et al. (Citation2017) affirmed the asymmetric effects through a dynamic panel data model. Similarly, by employing state-space models, Lòpez-villavicencio and Pourroy (Citation2019) found that the pass-through from oil price to inflation is significantly larger in inflation-targeting countries when the oil price decreases. In the context of Algeria, Lacheheb and Sirag (Citation2019) found obviously different impacts on inflation between oil price increases and reductions. Based on NARDL, Pal and Mitra (Citation2019) applied a novel MTNARDL model to exactly evaluate the influence of oil price change on US inflation, demonstrating that there is dramatic short-run asymmetry, but it is absent in the long run.
Considering these studies, due to different methodologies, scopes, and failure to clarify the role of asymmetry, mixed results exist in the literature regarding oil price and shocks pass-through into inflation (Raheem, Bello, and Agboola Citation2020). From an empirical perspective, scholars generally use conventional NARDL proposed by Shin, Yu, and Greenwood-nimmo (Citation2014) to evaluate spillover effects from positive and negative changes in oil price. Pal and Mitra (Citation2019) extended a two-way NARDL model by splitting the independent variable into multiple thresholds to capture the nonlinear relation more precisely. In this study, we follow Pal and Mitra (Citation2019) and employ two thresholds and four thresholds NARDL models to estimate the asymmetric impact of oil price and component shocks on inflation for the BRICS.
3. Methodology
3.1. Identification of oil price shocks
Before exploring the asymmetric impact of oil price changes on inflation, we firstly disentangle oil prices to different components using Ready’s (Citation2018) approach which categorized oil price by asset prices. According to Ready (Citation2018) identification strategy, demand shocks are defined as global oil producing firms’ portion of returns which is orthogonal to the innovations to the volatility index (VIX) of CBOE (Chicago Board Options Exchange). The innovations to the VIX are defined as proxy for risk shocks, then residuals of oil price variation which are orthogonal to both risk and demand shocks are represented as supply shocks. Consider the following matrix form:
Let st, dt, vt denoted the supply shocks, demand shocks and risk shocks, respectively, Δpt represents the changes in oil prices, is return of oil firms, and
is unexpected changes to VIX, based on an autoregressive moving average model, namely ARMA(1,1) specification, so that
Ready (Citation2018) imposes the following condition to ensure orthogonality among three shocks:
In equation 3, represents the covariance matrix of
,
,
and
are the variance of the three types of shocks. As we can see, Ready’s (Citation2018) method is another defined under SVAR framework and in line with Kilian (Citation2009).
3.2. Asymmetric analysis methods
Following Pal and Mitra (Citation2019), we test the asymmetric pass-through from oil price and component shocks to inflation using an MTNARDL approach proposed by Pal and Mitra (Citation2015, Citation2016) both for the short and long run. Their model was inspired by a seminal nonlinear ARDL suggested by Shin, Yu, and Greenwood-nimmo (Citation2014) wherein the independent variable(s) can be split into positive and negative partial sum components to estimate the asymmetric effect. While Shin, Yu, and Greenwood-nimmo (Citation2014) built upon their work on Pesaran, Shin, and Smith (Citation2001), which proposed a traditional ARDL model, we introduce the methodology step by step.
3.2.1 ARDL model
To begin, we present the following ARDL equation based on Pesaran, Shin, and Smith (Citation2001)
where CPI stands for consumer price index, Oil indicates crude oil price, in this article the Oil series also will be replace with three types of shocks. is the first difference operator, and
captures the error term. n1 and n2 are the lag orders. For the coefficients,
and
represent the short run while
and
represent the long run. The bounds test method proposed by Pesaran, Shin, and Smith (Citation2001) can be used to assess the potential cointegrating relation through the F-statistic where the null hypothesis is no cointegration for H0:
. Although the general lineal ARDL model does not estimate asymmetry, it has the following advantages. First, the technique can be used regardless of whether the underlying variables are order zero or one and by avoiding an order of integration as order two. Second, the technique can easily distinguish long- and short-term dynamics. Third, even if the regressors are endogenous, the model could be employed (Pesaran, Shin, and Smith Citation2001; Pal and Mitra Citation2019).
3.2.2. NARDL model
To capture the asymmetric effect, Shin, Yu, and Greenwood-nimmo (Citation2014) proposed nonlinear ARDL wherein regressors are decomposed into two partial sums, namely, positive and negative. In our study, Oil is expressed as follows:
where and
are partial sums that capture the increase and decrease of the oil price change, expressed as:
where , we reconstruct a NARDL model based on equation (7):
where all variables are characterized as in equation (4). In addition to the advantages of the ARDL model, long-run and short-run asymmetry can be tested by the standard Wald test. For the long run, the null hypothesis is . A significant difference in these two values would affirm a asymmetric relationship in the long run. In the same way,
indicates that there is no asymmetry in the short run. Moreover, the existence of cointegration could be assessed using the bounds test where the null hypothesis (
) means no cointegration.
3.2.3. Multiple threshold NARDL
The single threshold ARDL of Shin, Yu, and Greenwood-nimmo (Citation2014) just disintegrates the independent variable into positive and negative partial sums while MTNARDL proposed by Pal and Mitra (Citation2015, Citation2016) splits the regressor into different quantiles to explore the asymmetric transmission of the regressor with minor to major fluctuation. Gradually, we introduce two thresholds at the 30th and 70th quantiles to decompose oil price change into three partial sums:
In specification (8), ,
and
are three partial sums series calculated as below wherein
and
are used to present the 30th and 70th quantiles
where is a dummy variable that equals 1 when the conditions stated within
are satisfied, else zero. The two thresholds NARDL model can be specified as given below:
where. Rejection of the null hypothesis of H0:
could confirm cointegration among the three variables. In addition, the Wald test is used to assess short-run asymmetry with the null of no asymmetry for H0:
. Finally, for long-run asymmetry, H0 is
. Further, we split oil price change into five partial sums that set four thresholds at the 20th, 40th, 60th, and 80th quantiles. Then, the NARDL model can be denoted as:
For all the above models, following Bhutto and Chang (Citation2019) and Pal and Mitra (Citation2019), we take the same steps as follows. First, we estimate equation (4), (7) (10), and (11) using the OLS technique. Second, we estimate the cointegration relationship using the Wald test and, thus, obtain the F-statistic that will be compared with the asymptotic upper bound critical value. Finally, the standard Wald test is again applied to consider whether the effect of oil price change is symmetric or asymmetric on CPI both in the long and the short run. Like the ARDL model, the NARDL and MTNARDL have several advantages: (1) They are applicable regardless of whether the underlying variables are integrated of order one I(1) or zero I(0), thereby expanding the scope of the model’s application. (2) These models can estimate transmission between the independent and dependent variables both in the short and long run simultaneously. (3) The MTNARDL model considers the influence of the dependent variable on extreme positive and negative values of the independent variable and identifies the response to moderate changes in the independent variable (Bhutto and Chang Citation2019). However, one limitation of the MTNARDL model should be noted, namely, the sample size used for estimation continues to decrease as the threshold increases. For instance, a single threshold divides the estimated sample into two halves, four thresholds make the estimated number of samples become one-fifth of the original, and fewer samples reduce the accuracy of estimates. There are more than 200 observations used for every country, and we decompose the series into five partial sums at most. Thus, we are left with more than 45 unique observations, which is considered adequate for the analysis. Another limitation is that they cannot be used when any variables are beyond order one, so we conduct the unit root test before our analysis.
4. Data
This paper utilizes two different datasets in monthly frequency from January 2000 to March 2021. The first is to disentangle oil price into three component shocks and the second is for asymmetric analysis between oil price shocks and BRICK’s CPIs. In line with Ready (Citation2018), we firstly use the percentage changes of the second nearest maturity New York Mercantile Exchange (NYMEX) crude-light sweet oil futures contract as proxy for oil prices and logarithm of VIX options data for risk shocks, but for oil-producing firms returns, Ready (Citation2018) selects the World Integrated Oil and Gas Producer Index from DataStream, Zheng, Zhou, and Wen (Citation2021) conducts that these data unable to include major producers such as Saudi Aramco, so we use percentage changes of NYSE Arca Oil Index as the proxy for produce companies following Anand. and Paul (Citation2021). VIX and NYSE Arca Oil Index are from Yahoo Finance,Footnote1 oil price data are accepted from Energy Information Administration (EIA).
Once the shocks are constructed, we focus on ARDL, NARDL and MTNARDL models to examine whether the changes in crude oil price and their shocks have asymmetric effects on CPI in BRICS. We use the Europe Brent Spot Price FOB (dollars per barrel) to represent the crude oil price (denoted as Oil), and the data were retrieved from the EIA. All CPI data are from the OECD database taking 2015 as the base year (2015 = 100). Following Pal and Mitra (Citation2019), all variables have not been seasonally adjusted. shows the descriptive statistics of the underlying variables. It can be found that supply shocks highlight the positive mean value while demand and risk shocks are negative, which means the overall supply shock is positive while other two negative. Moreover, the value of kurtosis of supply shocks are greater than others. According to the standard deviations, we can know that oil price and risk shocks are volatile due to the high value.
Table 1. Descriptive statistics
5. Empirical analysis and discussion
According to the limitation of ARDL, NARDL and MTNARDL stated in methodology, none of the variables of order two I (2) should be integrated. Hence, before our analysis, we first used the ADF test proposed by Dickey and Fuller (Citation1979), the PP test proposed by Phillips and Perron (Citation1988), and the KPSS test proposed by Kwiatkowski et al. (Citation1992) to assess the order of integration of all variables both at level and first difference values. shows these results. However, these three tests still cannot identify any structural breaks, we further conduct unit root test provided by Perron (Citation1997) and the outcomes are set out in . All results illustrate that three types of shocks are stationary at level value and oil price stationary at the first difference, whereas for CPI, the five countries’ outcomes are mixed, but all the series are either I (0) or I (1), meeting the requirements of our models.
Table 2. Unit root tests without structural break
Table 3. Unit root with breaks
Next, to estimate whether underlying variables embed nonlinearity characteristics, we employed BDS test proposed by Broock et al. (Citation1996) and shows the findings of z-statistics. In the case of BDS test the null hypothesis is that the series is identically distributed and independent, it can be seen from the that all calculated statistics reject the null hypothesis except for demand shock. So, we can conclude that apart from the demand shock, all other time series undertaking are nonlinear and chaotic in nature.
Table 4. BDS test of independence
In addition, the Toda-Yamamoto causality test (Toda and Yamamoto Citation1995) is implemented to look into the causality linkage between variables. To put it simply, we abbreviate three shocks as S (supply shock), D (demand shock) and R (risk shock). In the following section of this paper, we will keep these abbreviations in the same format. As shown in , majority of pairs have no causality, but in the case of Russia and South Africa, there exist moderate relationships between oil price (shocks) and CPI. While for China, there is no causality among all pairs.
Table 5. Toda-Yamamoto causality results
After confirming all variables’ stationary, nonlinearity characteristics and Toda-Yamamoto causalities, we first use the ARDL model to assess the cointegration relation among the BRICS. presents the outcomes of linear ARDL. The top half of every panel shows the coefficients in the short run while the bottom shows the long run. The last row of panels provides the F-statistics employed by the Wald test, and they are compared with the asymptotic upper bound critical value estimated by Pesaran, Shin, and Smith (Citation2001). In addition, −1 in brackets means one period lagging. As the null of no cointegrating relation among the underling variables is significantly rejected in five countries (refer to the F-statistics), the cointegrated relationships between oil price and CPI, also component shocks and CPI, are affirmed through the ARDL model for all the countries. However, the ARDL model cannot assess the asymmetric effect. Next, we conduct the NARDL model to split oil price change into two partial sums to distinguish the different response of CPI on oil price and shocks increases (positive variation) and decreases (negative variation). shows the results.
Table 6. ARDL results
Table 7. NARDL results
In , the row labelled F statistic verifies the cointegration between oil price (three shocks) change and CPI in cases of BRICS. This is in line with the ARDL results. Wald_short and Wald_long, respectively, represent the F-statistics used by the Wald test to assess asymmetry in the short run (with H0: ) and long run (with H0:
). As show in Panel A, for all BRICS the asymmetric effect between oil price and CPI is absent. Compared to other results of the same type, a NARDL model employed by Lacheheb and Sirag (Citation2019) in the context of Algeria only found an asymmetric pass-through in the long run. However, Pal and Mitra (Citation2019), using the NARDL for the United States, noted that the asymmetric spillover effect only exists in the short run while Ibrahim (Citation2015), in the case of Malaysian food inflation, confirmed asymmetric pass-through both in the short and long run. There is no uniform perception due to choosing different countries and sample time spans.
From the significance of Wald_short, we find short-run supply shocks’ asymmetric impacts in Russia and China, demand shocks’ asymmetric impact in China and risk shocks’ asymmetric impact in India. Whereas in long term, wo just find a significant asymmetric impact of supply shock in South Africa. Specifically, from the perspective of supply shocks, it provides negative effects on Russia but positive effects on China and South Africa refer to significant coefficients. Consider demand shocks which means growth and prosperity of world economy, it is associated with a moderate inflation in most countries. As for risk shocks, which are used in Ready (Citation2018) to present unanticipated changes in market discount rates, the coefficients about regressor R only statistically significant in India, and the results don’t seem to be reliable, so we conduct more precise MTNARDL models to examine the asymmetry.
As indicated earlier, the single threshold (namely, standard NARDL) model does not precisely detect the spillover effect of all changes in oil prices from small to large. Therefore, we introduce two thresholds, namely, the 30th and 70th quantiles (presented by and
), to decompose oil price change into three partial sums. Estimated coefficients of the two thresholds NARDL are showcased in based on equation (10). Firstly we pay attention to panel A, following the significant coefficient of Δ(Oil(
)) and Oil(
) (−1) in the context of India and China, it illustrates that oil price decreases put a positive impact on CPI both in short and long run, but short effects are more remarkable due to larger values. The storyline for this phenomenon may proceed as Raheem, Bello, and Agboola (Citation2020): the cost of production will reduce because of lower oil prices. Thus, disposable income and consumption will increase and lastly, economics activities might bear an inflationary effect. Whereas in Russia, the fall of oil price pulls down the CPI in short run, these findings are consistent with Bjørnland (Citation2009) and Filis and Chatziantoniou (Citation2014). For South Africa, the CPI trend is always upward in the short run regardless of whether the oil price is increasing or decreasing. However, the different coefficients of Δ(Oil(
) (−1)) and Δ(Oil(
) (−1)) support that the change in CPI is higher when the oil price increases. To ensure these results are valid and to ferret out any conceivable uneven connection between oil price and CPI, we additionally decompose the oil price series into five partial sums with the 20th, 40th, 60th and 80th quantiles. More thresholds could examine whether the pass-through of oil price fluctuated for various degrees of fluctuation. presents the results following equation (11). Likewise, the above conclusions are verified and valid.
Table 8. MTNARDL (two thresholds) results
Table 9. MTNARDL (four thresholds) results
Turn to three types of shocks both in , majority of coefficients in Brazil are not pronounced, even oil price, nevertheless, there is nil asymmetry both in short and long term. For Russia, supply shocks bring most significant negative impacts on CPI, it is worth noting that the highest threshold demand shocks increase in short run, refer to Δ(D()) in and Δ(D(
)) in , dramatically accelerate domestic inflation. For India, risk shocks reduces inflation in the short run, but raises it back with a lag period corresponding to coefficients of Δ(R(
)) and Δ(R(
) (−1)) in . Moreover, there are long term effects under supply shocks in for Russia, India and South Africa, this may be due to the vulnerability of economic growth and high dependence on crude oil. Consider results about China, all four coefficients of Δ(S(
)) and Δ(D(
)) in are pronounced which disclose that both supply or demand shocks decrease would lead short-term inflationary effect in China, besides, demand shock have a moderate persistent effect based on long run coefficient in two tables. Lastly, put the spotlight on South Africa, the most important characteristic is the significance of long run coefficient of supply shock, additionally, uttermost raise in demand, refer to Δ(D(
) (−1)) in and Δ(D(
) (−1)) in , temporarily precipitate domestic deflation in short term.
To sum up, we collect the four results for ARDL, NARDL, and MTNARDL with two thresholds and four thresholds in and . With reference to , all F-statistics used for testing cointegrated relationships are tabulated in . Five countries are confirmed to hold a cointegration relationship between the underlying variables. More crucial, in , we capture all Wald_short and Wald_long values from to to compare the strength of asymmetry both in the short and long run. In Panel A the nulls of no asymmetry are only rejected for China in the short run, implying strong asymmetric transmission between inflation and oil price change. Whereas for other BRICS the asymmetric effect between oil price and CPI is absent. These views are supported by substantial literature that suggests the influence of oil prices on inflation is declining or refuses to admit the major spillover from oil price to inflation, such as Hooker (Citation2002), De Gregorio et al. (Citation2007), Leblanc and Chinn (Citation2004), Gómez-loscos, Gadea, and Montañés (Citation2012), and Tiwari et al. (Citation2019). In the short run study of China, coefficients of partial sums at the lowest and highest thresholds present different signs and absolute value, which confirms that an oil price decrease leads to a rise in inflation faster, while an increase in oil price will cause slight deflation. A possible explanation is that as suggested by Raheem, Bello, and Agboola (Citation2020), lower oil prices reduce product costs and increase disposable income and consumption. The discretionary income effect will lead to an inflationary impact on the economy, but the operating cost effect will do the same. When oil prices are low, energy-related consumption is stimulated because of a reduction in operating costs. On the contrary, when oil prices are sharply increasing, the uncertainty and concern about precautionary saving are all required to convey an articulated effect on sluggish economic activities and joblessness, and, along these lines, cause deflation.
Table 10. Test statistic to ascertain the strength of the cointegrated relationship
Table 11. Test statistic to ascertain the asymmetry strength in the short and long run
Surprisingly, the strength of asymmetry in Panel C and D () are almost nil and weak, while several strong asymmetries are exhibited in Panel B. This suggests that only supply shock holds asymmetric pass-through effects. More specifically, in short run supply shock establishes a pronounced asymmetry for China and Russia, the world’s largest oil importing country and second largest oil exporter. Significant coefficients at the lowest and highest thresholds reveal that substantial supply shock decreases inhibit inflation at a higher level in Russia (refer to Δ(S()) and Δ(S(
))), but fuel inflation in China (refer to Δ(S(
)) and Δ(S(
) (−1))). Ready’s supplyFootnote2 shocks are defined as difficulty to produce crude oil, as a major net oil exporter, Russia will make more profits with a higher price due to reduction in production, hence lead to a currency appreciation and hedge devaluation risk on currency (Lin and Su Citation2020), moreover, oil exporter can attract more international investors when supply shocks occur and enhance their economic status (Yang, Cai, and Hamori Citation2017). Contrastingly, other net oil importers have to passively suffer negative influence on supply shocks and facing upward inflation (Filis, Degiannakis, and Floros Citation2011). As for South Africa in the long run, inflation increases and then decreases as supply shocks increase, a possible reason is that central bank will adopt measures to hedge against risks in case of supply shocks are fluctuating sharply. It is worth mentioning that nil asymmetry in demand and risk shocks does not mean they have on effect on inflation, there is a large volume of research verify these shocks produce impact on output and inflation, such as (Gómez-loscos, Gadea, and Montañés Citation2012), (Anzuini, Pagano, and Pisani Citation2015), (Herwartz and Plödt Citation2016). In this article, we focus our research on the asymmetric effects and do not find asymmetric impacts of these two shocks on inflation.
6. Conclusion
This article lays the groundwork for a discussion on the underpinnings of contagion from oil price and shocks to inflation. With regard to the BRICS, we firstly decompose the oil price change into supply, demand and risk shocks using approach provided by Ready (Citation2018), subsequently, we establish an empirical framework to explore asymmetric pass-through by using an MTNARDL model proposed by Pal and Mitra (Citation2015, Citation2016). Compared to conventional NARDL, which decomposes the regressor to a positive and negative partial sum, we split oil price change and three types of shocks into three and five partial sums because, in doing so, we yield exact evaluations and more detailed and minute outcomes. More comprehensively, we showcase the results of ARDL and NARDL to reveal the nexus among underlying variables.
The empirical results can be expressed as the following: first, significant asymmetry between oil price and inflation are only exhibited in China in the short run. More specifically, in the short run, CPI in China increases at a faster rate when oil prices fall, but when oil prices rise, CPI declines at a slower pace. This suggests that inflationary effect is more dramatic when oil price decrease, significantly reduce the operate cost and stimulate consumption. Second, for the supply shocks, strong asymmetries are exhibited in Russia and China in the short run and in South Africa in the long term. Oil exporter such as Russia will make more profits with a higher price due to reduction in production in both short and long run, hence lead to currency appreciation and deflation, supply shock reduction will stimulate China’s inflation temporarily whereas for South Africa, in the long time CPI is always rising regardless of whether supply shocks rise or fall but the impact is controlled in response to extreme fluctuations. Finally, demand shock and risk shock holds ether nil asymmetry or weak, in most cases risk shocks have the weakest effect on inflation and disappear in the short term, demand shocks only exert impact temporarily during extreme changes.
We find three policy implications considering the outcomes. First, BRICS’ authorities should pay more attention to asymmetric pass-through from oil price to inflation, in particular, lower oil prices have a greater impact on inflation than higher in China. Meanwhile, the need for government intervention is unquestionable. After distinguishing a positive or negative impact, some policy tools, such as subsidies and taxes, should be employed to achieve price stability. For instance, because the pass-through of oil price on China’s inflation might mainly act on consumption, governments ought to detail approaches that stimulate consumption when oil prices increase. For South Africa, in the long run, the government should work to reduce the cost of products. The second implication is that policymakers ought to identify different oil price shocks, oil importing country, such as China, and oil exporter like Russia, are more sensitive to supply fluctuation, while demand shock and risk shock exert slight and temporarily impacts, more measures should be adopted to deal with supply shock. Finally, the third implication is for investors with an interest in establishing portfolios of crude oil with other assets. By identifying the nexus between oil prices and inflation, as well as the nexus between inflation and other asset prices, investors could allocate assets more rationally both for short and long-run interests.
It is erroneous to assume that oil price and component shocks have identical and significant spillover effects on countries since each country has diverse levels of development, energy dependence, income, even different industrial structures, exchange regimes and energy legislations. Accordingly, oil price might influence inflation in a disparate way. Further research might explore the underlying variables to explain the link between oil price and inflation, and more possible influencing factors should be added to the empirical model. In future, we will further expand the existing study based on the grouping of oil importers and exporters and the introduce exchange rate regime to analysis. Additionally, the use of MTARDL can be extended.
Disclosure statement
No potential conflict of interest was reported by the author(s).
Notes
References
- Anand., B., and S. Paul. 2021. “Oil Shocks and Stock Market: Revisiting the Dynamics.” Energy Economics 96:105-111.
- Anzuini, A., P. Pagano, and M. Pisani. 2015. “Macroeconomic Effects of Precautionary Demand for Oil.” Journal of Applied Econometrics 30 (6): 968–986. doi:https://doi.org/10.1002/jae.2419.
- Bhutto, N. A., and B. H. Chang. 2019. “The Effect of the Global Financial Crisis on the Asymmetric Relationship between Exchange Rate and Stock Prices.” High Frequency 2 (3–4): 175–183. doi:https://doi.org/10.1002/hf2.10033.
- Bjørnland, H. C. 2009. “Oil Price Shocks and Stock Market Booms in an Oil Exporting Country.” Scottish Journal of Political Economy 56 (2): 232–254. doi:https://doi.org/10.1111/j.1467-9485.2009.00482.x.
- Bohi, D. R. 1991. “On the Macroeconomic Effects of Energy Price Shocks.” Resources and Energy 13 (2): 145–162. doi:https://doi.org/10.1016/0165-0572(91)90012-R.
- Bresnahan, T. F., and V. A. Ramey. 1993. “Segment Shifts and Capacity Utilization in the US Automobile Industry.” The American Economic Review 83: 213–218.
- Broock, W. A., J. A. Scheinkman, W. D. Dechert, and B. Lebaron. 1996. “A Test for Independence Based on the Correlation Dimension.” Econometric Reviews 15 (3): 197–235. doi:https://doi.org/10.1080/07474939608800353.
- Chen, J., X. Zhu, and H. Li. 2020. “The Pass-through Effects of Oil Price Shocks on China’s Inflation: A Time-varying Analysis.” Energy Economics 86: 104695. doi:https://doi.org/10.1016/j.eneco.2020.104695.
- Chen, -S.-S. 2009. “Oil Price Pass-through into Inflation.” Energy Economics 31 (1): 126–133. doi:https://doi.org/10.1016/j.eneco.2008.08.006.
- Cologni, A., and M. Manera. 2008. “Oil Prices, Inflation and Interest Rates in a Structural Cointegrated VAR Model for the G-7 Countries.” Energy Economics 30 (3): 856–888. doi:https://doi.org/10.1016/j.eneco.2006.11.001.
- Davis, S. J., and J. Haltiwanger. 2001. “Sectoral Job Creation and Destruction Responses to Oil Price Changes.” Journal of Monetary Economics 48 (3): 465–512. doi:https://doi.org/10.1016/S0304-3932(01)00086-1.
- De Gregorio, J., O. Landerretche, C. Neilson, C. Broda, and R. Rigobon. 2007. “Another Pass-through Bites the Dust? Oil Prices and Inflation [With Comments].” Economia 7: 155–208.
- Demirer, R., R. Ferrer, and S. J. H. Shahzad. 2020. “Oil Price Shocks, Global Financial Markets and Their Connectedness.” Energy Economics 88: 104771. doi:https://doi.org/10.1016/j.eneco.2020.104771.
- Dickey, D. A., and W. A. Fuller. 1979. “Distribution of the Estimators for Autoregressive Time Series with a Unit Root.” Journal of the American Statistical Association 74: 427–431.
- Edelstein, P., and L. Kilian. 2009. “How Sensitive are Consumer Expenditures to Retail Energy Prices?” Journal of Monetary Economics 56 (6): 766–779. doi:https://doi.org/10.1016/j.jmoneco.2009.06.001.
- Filis, G., and I. Chatziantoniou. 2014. “Financial and Monetary Policy Responses to Oil Price Shocks: Evidence from Oil-importing and Oil-exporting Countries.” Review of Quantitative Finance and Accounting 42 (4): 709–729. doi:https://doi.org/10.1007/s11156-013-0359-7.
- Filis, G., S. Degiannakis, and C. Floros. 2011. “Dynamic Correlation between Stock Market and Oil Prices: The Case of Oil-importing and Oil-exporting Countries.” International Review of Financial Analysis 20 (3): 152–164. doi:https://doi.org/10.1016/j.irfa.2011.02.014.
- Gao, L., H. Kim, and R. Saba. 2014. “How Do Oil Price Shocks Affect Consumer Prices?” Energy Economics 45: 313–323. doi:https://doi.org/10.1016/j.eneco.2014.08.001.
- Gómez-loscos, A., M. D. Gadea, and A. Montañés. 2012. “Economic Growth, Inflation and Oil Shocks: Are the 1970s Coming Back?” Applied Economics 44 (35): 4575–4589. doi:https://doi.org/10.1080/00036846.2011.591741.
- Gong, X., and B. Lin. 2018. “Time-varying Effects of Oil Supply and Demand Shocks on China’s Macro-economy.” Energy 149: 424–437. doi:https://doi.org/10.1016/j.energy.2018.02.035.
- Hamilton, J. D. 1983. “Oil and the Macroeconomy since World War II.” Journal of Political Economy 91 (2): 228–248. doi:https://doi.org/10.1086/261140.
- Hamilton, J. D. 2003. “What Is an Oil Shock?” Journal of Econometrics 113 (2): 363–398. doi:https://doi.org/10.1016/S0304-4076(02)00207-5.
- Hamilton, J. D. 2011. “Nonlinearities and the Macroeconomic Effects of Oil Prices.” Macroeconomic Dynamics 15 (S3): 364–378. doi:https://doi.org/10.1017/S1365100511000307.
- Herwartz, H., and M. Plödt. 2016. “The Macroeconomic Effects of Oil Price Shocks: Evidence from a Statistical Identification Approach.” Journal of International Money and Finance 61: 30–44. doi:https://doi.org/10.1016/j.jimonfin.2015.11.001.
- Hooker, M. A. 1999. “Oil and the Macroeconomy Revisited.” Available at SSRN 186014.
- Hooker, M. A. 2002. “Are Oil Shocks Inflationary? Asymmetric and Nonlinear Specifications versus Changes in Regime.” Journal of Money, Credit and Banking 34 (2): 540–561. doi:https://doi.org/10.1353/mcb.2002.0041.
- Ibrahim, M. H. 2015. “Oil and Food Prices in Malaysia: A Nonlinear ARDL Analysis.” Agricultural and Food Economics 3 (1). doi:https://doi.org/10.1186/s40100-014-0020-3.
- Jacquinot, P., M. Kuismanen, R. Mestre, and M. Spitzer. 2009. “An Assessment of the Inflationary Impact of Oil Shocks in the Euro Area.” The Energy Journal 30 (1). doi:https://doi.org/10.5547/0195-6574-EJ-Vol30-No1-3.
- Jiranyakul, K. 2019. “Oil Price Shocks and Domestic Inflation in Thailand.” Available at SSRN 2578836.
- Kilian, L. 2008. “Exogenous Oil Supply Shocks: How Big are They and How Much Do They Matter for the US Economy?” The Review of Economics and Statistics 90 (2): 216–240. doi:https://doi.org/10.1162/rest.90.2.216.
- Kilian, L. 2009. “Not All Oil Price Shocks are Alike: Disentangling Demand and Supply Shocks in the Crude Oil Market.” American Economic Review 99 (3): 1053–1069. doi:https://doi.org/10.1257/aer.99.3.1053.
- Kilic, E., and S. Cankaya. 2019. “Oil Prices and Economic Activity in BRICS and G7 Countries.” Central European Journal of Operations Research 28 (4): 1315–1342. doi:https://doi.org/10.1007/s10100-019-00647-8.
- Kwiatkowski, D., P. C. Phillips, P. Schmidt, and Y. Shin. 1992. “Testing the Null Hypothesis of Stationarity against the Alternative of a Unit Root: How Sure are We that Economic Time Series Have a Unit Root?” Journal of Econometrics 54 (1–3): 159–178. doi:https://doi.org/10.1016/0304-4076(92)90104-Y.
- Lacheheb, M., and A. Sirag. 2019. “Oil Price and Inflation in Algeria: A Nonlinear ARDL Approach.” The Quarterly Review of Economics and Finance 73: 217–222. doi:https://doi.org/10.1016/j.qref.2018.12.003.
- Leblanc, M., and M. D. Chinn. 2004. “Do High Oil Prices Presage Inflation? the Evidence from G-5 Countries.” UC Santa Cruz Economics Working Paper 561(4): 04-04.
- Lee, K., and S. Ni. 2002. “On the Dynamic Effects of Oil Price Shocks: A Study Using Industry Level Data.” Journal of Monetary Economics 49 (4): 823–852. doi:https://doi.org/10.1016/S0304-3932(02)00114-9.
- Lin, B., and T. Su. 2020. “Does Oil Price Have Similar Effects on the Exchange Rates of BRICS?” International Review of Financial Analysis 69: 101461. doi:https://doi.org/10.1016/j.irfa.2020.101461.
- Lòpez-villavicencio, A., and M. Pourroy. 2019. “Inflation Target and (A)symmetries in the Oil Price Pass-through to Inflation.” Energy Economics 80: 860–875. doi:https://doi.org/10.1016/j.eneco.2019.01.025.
- Nasir, M. A., A. A. Al-Emadi, M. Shahbaz, and S. Hammoudeh. 2019. “Importance of Oil Shocks and the GCC Macroeconomy: A Structural VAR Analysis.” Resources Policy 61: 166–179. doi:https://doi.org/10.1016/j.resourpol.2019.01.019.
- Pal, D., and S. K. Mitra. 2015. “Asymmetric Impact of Crude Price on Oil Product Pricing in the United States: An Application of Multiple Threshold Nonlinear Autoregressive Distributed Lag Model.” Economic Modelling 51: 436–443. doi:https://doi.org/10.1016/j.econmod.2015.08.026.
- Pal, D., and S. K. Mitra. 2016. “Asymmetric Oil Product Pricing in India: Evidence from a Multiple Threshold Nonlinear ARDL Model.” Economic Modelling 59: 314–328. doi:https://doi.org/10.1016/j.econmod.2016.08.003.
- Pal, D., and S. K. Mitra. 2019. “Asymmetric Oil Price Transmission to the Purchasing Power of the U.S. Dollar: A Multiple Threshold NARDL Modelling Approach.” Resources Policy 64: 101508. doi:https://doi.org/10.1016/j.resourpol.2019.101508.
- Peersman, G., and I. Van Robays. 2009. “Oil and the Euro Area Economy.” Economic Policy 24 (60): 603–651. doi:https://doi.org/10.1111/j.1468-0327.2009.00233.x.
- Perron, P. 1997. “Further Evidence on Breaking Trend Functions in Macroeconomic Variables.” Journal of Econometrics 80 (2): 355–385. doi:https://doi.org/10.1016/S0304-4076(97)00049-3.
- Pesaran, M. H., Y. Shin, and R. J. Smith. 2001. “Bounds Testing Approaches to the Analysis of Level Relationships.” Journal of Applied Econometrics 16 (3): 289–326. doi:https://doi.org/10.1002/jae.616.
- Phillips, P. E. T. E. R. C. B., and P. Perron. 1988. “Testing for a Unit Root in Time Series Regression.” Biometrika 75 (2): 335–346. doi:https://doi.org/10.1093/biomet/75.2.335.
- Prat, G., and R. Uctum. 2011. “Modelling Oil Price Expectations: Evidence from Survey Data.” The Quarterly Review of Economics and Finance 51 (3): 236–247. doi:https://doi.org/10.1016/j.qref.2011.03.003.
- Raheem, I. D., A. K. Bello, and Y. H. Agboola. 2020. “A New Insight into Oil Price-inflation Nexus.” Resources Policy 68: 101804. doi:https://doi.org/10.1016/j.resourpol.2020.101804.
- Ready, R. C. 2018. “Oil Prices and the Stock Market*.” Review of Finance 22 (1): 155–176. doi:https://doi.org/10.1093/rof/rfw071.
- Salisu, A. A., K. O. Isah, O. J. Oyewole, and L. O. Akanni. 2017. “Modelling Oil Price-inflation Nexus: The Role of Asymmetries.” Energy 125: 97–106. doi:https://doi.org/10.1016/j.energy.2017.02.128.
- Sek, S. K., X. Q. Teo, and Y. N. Wong. 2015. “A Comparative Study on the Effects of Oil Price Changes on Inflation.” Procedia Economics and Finance 26: 630–636. doi:https://doi.org/10.1016/S2212-5671(15)00800-X.
- Shin, Y., B. Yu, and M. Greenwood-nimmo. 2014. “Modelling Asymmetric Cointegration and Dynamic Multipliers in a Nonlinear ARDL Framework.” Festschrift in Honor of Peter Schmidt, no. Springer.281-314.
- Tiwari, A. K., J. Cunado, A. Hatemi-J, and R. Gupta. 2019. “Oil Price-inflation Pass-through in the United States over 1871 to 2018: A Wavelet Coherency Analysis.” Structural Change and Economic Dynamics 50: 51–55. doi:https://doi.org/10.1016/j.strueco.2019.05.002.
- Toda, H. Y., and T. Yamamoto. 1995. “Statistical Inference in Vector Autoregressions with Possibly Integrated Processes.” Journal of Econometrics 66 (1–2): 225–250. doi:https://doi.org/10.1016/0304-4076(94)01616-8.
- Wen, F., K. Zhang, and X. Gong. 2021. “The Effects of Oil Price Shocks on Inflation in the G7 Countries.” The North American Journal of Economics and Finance 57: 101391. doi:https://doi.org/10.1016/j.najef.2021.101391.
- Yang, L., X. J. Cai, and S. Hamori. 2017. “Does the Crude Oil Price Influence the Exchange Rates of Oil-importing and Oil-exporting Countries Differently ? A Wavelet Coherence Analysis.” International Review of Economics & Finance 49: 536–547. doi:https://doi.org/10.1016/j.iref.2017.03.015.
- Zhao, L., X. Zhang, S. Wang, and S. Xu. 2016. “The Effects of Oil Price Shocks on Output and Inflation in China.” Energy Economics 53: 101–110. doi:https://doi.org/10.1016/j.eneco.2014.11.017.
- Zheng, Y., M. Zhou, and F. Wen. 2021. “Asymmetric Effects of Oil Shocks on Carbon Allowance Price: Evidence from China.” Energy Economics 97: 105183. doi:https://doi.org/10.1016/j.eneco.2021.105183.