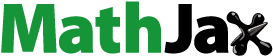
ABSTRACT
We analyse persistent and transient technical efficiency of crop farms in Austria from 2003 to 2017 by estimating the four-component stochastic frontier model using a multi-step procedure and extend it to account for heterogeneity bias by introducing the Mundlak adjustments. Moreover, we examine the determinants of both transient and persistent technical inefficiency. Results show that farms with favourable natural conditions, a higher share of family labour, and a lower share of owned land are more persistently efficient. Farm specialization, size, and farmers’ age are positively associated with transient efficiency, while subsidies have adverse impacts. Significant technological progress coupled with, on average decreasing technical efficiency indicates a diverging sector.
I. Introduction
Stochastic frontier (SF) analysis is commonly applied to evaluate efficiency, decompose productivity, and identify determinants of the efficiency of agricultural holdings. Results from such studies can help evaluate agricultural policies and provide insights for governments on how to improve the performance of farms. The main challenge in examining the efficiency of agricultural holdings is that farms are very heterogeneous regarding production conditions (e.g. altitude, soil quality), size, production orientation (e.g. crop, livestock, and mixed farming), and organization (e.g. share of family labour, ownership of production factors, full-time versus part-time farming). Therefore, it is vital to account for observed and unobserved heterogeneity and separate it from inefficiency. To resolve this, Greene (Citation2005a, Citation2005b) proposed the true fixed and random effects models, which separate firm-effects from a time-varying inefficiency term. These models combine conventional panel data methods with a skewed stochastic component that accounts for inefficiency. However, this approach has the drawback that time-invariant inefficiency is captured as heterogeneity.
To overcome this limitation, Colombi et al. (Citation2014), Kumbhakar, Lien, and Hardaker (Citation2014), and Tsionas and Kumbhakar (Citation2014) simultaneously proposed a four-component stochastic frontier model. This model generalizes the true random effects model and allows for the separation of technical efficiency into persistent (time-invariant) and transient (time-varying) components while controlling for firm-effects and random errors. The four-component model provides insightful information regarding farms’ resource-saving potential and performance in both the short- and long-run (Kumbhakar, Lien, and Hardaker Citation2014; Lien, Kumbhakar, and Alem Citation2018). The persistent inefficiency component potentially captures a lack of competitiveness due to a lack of managerial skills, structural and organizational problems related to the production process, or a systematic waste of inputs (Berisso Citation2019; Lien, Kumbhakar, and Alem Citation2018). In agriculture, unfavourable natural conditions (e.g. altitude and soil quality) may also add to persistent inefficiency (Brümmer Citation2001; Latruffe et al. Citation2004; Madau Citation2007). As Kumbhakar and Heshmati (Citation1995) noted, farms with higher persistent inefficiency levels are unlikely to survive and thus exit the industry; however, these unfavourable production conditions in agriculture are often counterbalanced by compensatory payments, so-called less favoured area payments (Cooper et al. Citation2006). On the other hand, the transient component captures non-systematic issues attributable to short-run rigidities, temporary managerial and behavioural problems, or a suboptimal use of inputs that are solvable in the short-run (Filippini and Greene Citation2016). From a policy perspective, targeting transient inefficiency is often easier and more promising.
Although heterogeneity and time-invariant inefficiency are accounted for, an essential assumption of the four-component model is that heterogeneity is assumed to be random and uncorrelated with the explanatory variables (Colombi, Martini, and Vittadini Citation2017). If this assumption is not fulfilled, this will result in inconsistent estimates of slope parameters and biased efficiency scores. This is especially problematic in agricultural production since firm heterogeneity (e.g. natural production conditions) may be unobserved by the analyst but is observable to farmers, who may, in turn, adjust their input use accordingly. Therefore, a correlation between unobserved heterogeneity and observed input use is likely, if not inevitable. To overcome this heterogeneity bias in the slope parameters, we propose incorporating the Mundlak (Citation1978) adjustments, namely the means over time of all input variables, into the four-component SF model.
From a policy perspective, it is not only interesting to differentiate between transient and persistent inefficiency but also to investigate their determinants. Identifying the main drivers of transient and persistent inefficiency will help governments design policies to foster efficiency. While determinates of persistent inefficiency are often hard to tackle (e.g. natural conditions), determinants of transient inefficiency are often more promising starting points. Kumbhakar, Ghosh, and McGuckin (Citation1991) first proposed incorporating exogenous determinants in the inefficiency term. Battese and Coelli (Citation1995) developed a model for panel data. In the context of the four-component model, Badunenko and Kumbhakar (Citation2017), Colombi, Martini, and Vittadini (Citation2017), and Lai and Kumbhakar (Citation2018a) were the first to introduce determinants of both transient and persistent inefficiency using a simulated maximum likelihood, single-step maximum likelihood, and multi-step approach, respectively. However, their studies focused on non-agricultural sectors. Using farm-level data, Lien, Kumbhakar, and Alem (Citation2018) first analysed the determinants of the transient (but not persistent) technical efficiency of Norwegian farms. Recently, using the multi-step approach, Baležentis and Sun (Citation2020), Minviel and Sipiläinen (Citation2021), and Trnková and Kroupová (Citation2020) analysed the determinants of transient and persistent technical efficiency of Lithuanian dairy, French crop-livestock, and EU dairy farms, respectively. Several other studies have investigated the efficiency of farms using the four-component SF model, although they do not consider determinants in their analyses (e.g. Acosta and De Los Santos-Montero (Citation2019), Bokusheva and Čechura. (Citation2017), and Pisulewski and Marzec (Citation2019)).
The Austrian agricultural sector is an interesting case to study the determinants of transient and persistent inefficiency with a four-component model for several reasons. First, the ongoing structural change in the agricultural sector and recent developments in the Common Agricultural Policy (CAP) of the European Union (EU) have further increased the competitive pressure on farms. In the last three decades, the CAP has been transformed from a policy based on high guaranteed domestic prices, high export subsidies, prohibitive import tariffs, and income transfers to farmers to a much less protected and much more market-oriented policy. With a now much closer link to world market prices, EU farmers face higher price volatility and more competition from abroad and within the EU. In line with the CAP transformations, Austrian agricultural policies in recent years have focused on strengthening competitiveness, promoting sustainable farming and innovation, enhancing rural development, and inducing productive land use (BMLFUW Citation2016). To survive in this sector, increasing productivity and efficiency is crucial. This is especially true for farmers in countries such as Austria, which face natural and scale disadvantages.
Second, farms are relatively heterogeneous in regard to several properties, which may impact efficiency, including natural production conditions (Brümmer Citation2001; Latruffe et al. Citation2004; Madau Citation2007), farm structure and organization (Karagiannis and Sarris Citation2005; Kourtesi, De Witte, and Polymeros Citation2016; Latruffe et al. Citation2005), and farm manager characteristics (Adhikari and Bjorndal Citation2012; Madau Citation2011). Natural production conditions vary, for example, regarding altitude (from 100 to 2,000 metres above sea level), precipitation (from 450 mm to more than 2,750 mm per year), and soil type (Eder, Salhofer, and Scheichel Citation2021). Differences in natural conditions and regional differences in inheritance and succession customs have provoked differences in farm structure and organization. These include land ownership structure, farm size, the degree of specialization, and the share of family labour. For example, in 2016, the share of rented land in total utilized agricultural area (UAA) was only 25% in Tyrol but was 62% in Burgenland and the average farm size varied from 12.6 ha UAA in Styria to 28.1 ha in Lower Austria (Statistik Austria Citation2018).
Finally, farm managers are heterogeneous in regard to their vocational skills and experience (age). In 2016, about 28% of farm managers held an agricultural diploma and 14% had a higher level of vocational education (high school with agricultural background, university degree in agriculture, or master craft certificate), while 58% had mainly practical experience. In regard to age, about 11% of farm managers were younger than 35 years of age, 56% were between 35 and 54 years, 23% were between 55 and 64 years, and 10% were 65 years and older (Statistik Austria Citation2018).
Given this background, the contributions of this study are threefold. First, we apply the four-component model using the multi-step technique proposed by Kumbhakar, Lien, and Hardaker (Citation2014) and extend it to account for heterogeneity bias by introducing the Mundlak adjustments. Second, we investigate the impacts of natural production conditions (altitude and soil quality), farm structure and organization (size, specialization, land ownership, family labour share, and subsidies) and farm manager characteristics (age and vocational education) on transient and persistent technical efficiency. Third, we are the first to investigate the technical efficiency of a panel of crop farms in Austria.
The remainder of this paper is organized as follows: Section 2 presents the four-component stochastic model with determinants of transient and persistent inefficiency; Section 3 describes the utilized data; Section 4 presents the empirical results and discussions; and Section 5 provides concluding remarks.
II. Empirical model
We start from the four-component SF panel data model simultaneously introduced by Colombi et al. (Citation2014), Kumbhakar, Lien, and Hardaker (Citation2014), and Tsionas and Kumbhakar (Citation2014):
where index denotes
production units (farms), and
indicates the periods at which each unit is observed. The dependent variable
is the logarithm of the output of farm
at time
,
is a common intercept,
is the production technology including a time trend
to account for technological change,
is a vector of inputs (in logs), and
is a vector of unknown parameters to be estimated. Changes in the output that are unexplained by input variations are divided into four components: i)
captures time-invariant firm-specific latent (unobserved) heterogeneity; ii)
accounts for persistent (time-invariant) technical inefficiency; iii)
captures transient (time-varying) technical inefficiency; and iv)
is a random noise term.
The four-component model nests many previous panel SF models. For example, by dropping one can obtain Greene’s (Citation2005a, Citation2005b) true fixed or random effects model, depending on whether
is correlated with the error term. In these models, any persistent inefficiency is completely absorbed in the individual-specific constant term. Dropping
leads to models that account for transient and persistent inefficiency, but ignore latent firm heterogeneity (Kumbhakar and Heshmati Citation1995; Kumbhakar and Hjalmarsson Citation1993). Dropping both
and
leads to older time-invariant inefficiency models of Kumbhakar (Citation1987), Pitt and Lee (Citation1981), and Schmidt and Sickles (Citation1984). Dropping both
and
leads to time-variant inefficiency models of Battese and Coelli (Citation1992), Cornwell, Schmidt, and Sickles (Citation1990), and Kumbhakar (Citation1990).
In general, unobserved firm heterogeneity can be modelled as either fixed or random. Fixed effects have the advantage of allowing for correlation between heterogeneity and the explanatory variables and hence provide unbiased estimates of the parameter vector
. Random effects models may result in biased estimates of technology parameters when the unobserved factors are correlated with the explanatory variables. Karagiannis (Citation2014) argues that random effects SF models may be more appropriate for agricultural production given the time-lag between input decisions and output realization and the uncertainty regarding production conditions when the production decisions are made. Hence, one can assume that the correlation between the largely weather-affected stochastic error term and the largely predetermined input variables is zero or very small (Griliches Citation1963). However, even if this were not the case, the fixed effects estimator does not allow the inclusion of any time-invariant variables, e.g. variables accounting for the production environment of the farm unit, in the estimation because of perfect multicollinearity with
.Footnote1
As a solution, we assume firm heterogeneity to be random, but apply Mundlak’s (Citation1978) adjustment terms to reduce potential biases in the technology (slope) parameters and inefficiency estimates as documented in the literature. Therefore, heterogeneity (is modelled as
, where
is a vector of the logged inputs averaged over time for a given firm
,
is the vector of corresponding parameters to be estimated, and
is pure unobserved heterogeniety (Farsi, Filippini, and Kuenzle Citation2005). To explain efficiency differences among farms, we assume that the variances of
and
are functions of defined determinants. Hence, the persistent inefficiency
is nonnegative such that
, where
is a vector of time-invariant determinants of persistent inefficiency and transient inefficiency is nonnegative such that
, where
is a vector of time-varying determinants of transient inefficiency.
In estimating the four-component model, Colombi et al. (Citation2014) implemented a single-step maximum likelihood (ML) procedure, which Colombi, Martini, and Vittadini (Citation2017) extended to include determinants. However, as the complexity of the model increases with more generalized inefficiency settings, the likelihood function becomes more complicated and difficult to achieve convergence (Lai and Kumbhakar Citation2018b). Hence, this approach is cumbersome and challenging to implement in empirical applications (Filippini and Greene Citation2016). Moreover, because of its dimension, the ML estimation will suffer from the numerical integration problem when is large (Lai and Kumbhakar Citation2018b). Given these challenges, Filippini and Greene (Citation2016) proposed a maximum simulated likelihood (MSL) approach, which takes full advantage of the likelihood function while reducing the amount of computation and extreme complexity of the ML approach. Hence, it is relatively easy to implement from the practical point of view (Lai and Kumbhakar Citation2018b). The MSL approach has been adapted and extended by Badunenko and Kumbhakar (Citation2017) and Lai and Kumbhakar (Citation2018b) to include determinants of inefficiency.
Kumbhakar, Lien, and Hardaker (Citation2014) proposed a multi-step procedure to estimate transient and persistent efficiency. This approach of decoupling the estimation of the error component structure and the production frontier is also known as pseudo- or plug-in likelihood estimation (Andor and Parmeter Citation2017; Kumbhakar and Parmeter Citation2019). Lien, Kumbhakar, and Alem (Citation2018) adapted this technique to account for the endogeneity of inputs and output and included determinants of transient efficiency. With this approach, the technology parameters in the production function ( in the first step) are not contaminated by distributional assumptions on the error components which are central to the single-step procedure (Lien, Kumbhakar, and Alem Citation2018; Sun et al. Citation2020). Furthermore, compared to the single-step approach, the multi-step approach is more intuitive, easier to implement, and can have the estimation results verified in each step (Agasisti and Gralka Citation2019; Lien, Kumbhakar, and Alem Citation2018). Although the multi-step procedure is considered inefficient compared to single-step ML, Andor and Parmeter (Citation2017) showed that for a standard cross-section SF model (Aigner, Lovell, and Schmidt Citation1977; Meeusen and Van Den Broeck Citation1977), pseudo-likelihood has almost identical performance to ML estimations.
In the present study, we apply the multi-step method to analyse the production technology, transient and persistent technical efficiency and their determinants for crop farms in Austria. To implement this procedure, we rewrite EquationEquation (1)(1)
(1) to correct for the heterogeneity bias (i.e. the Mundlak adjustments) and introduce determinants of both transient and persistent technical efficiency as:
where ,
and
. EquationEquation (2)
(2)
(2) is a partial linear model for random effects panel data. In this case, the parametric components can be estimated non-parametrically using a local linear kernel regression by first taking the conditional expectation of both sides with respect to
(the combination of
and
) and then subtracting it from EquationEquation (2)
(2)
(2) as suggested by Robinson (Citation1988) and applied in the context of the multi-step SF model by Lien, Kumbhakar, and Alem (Citation2018).
Based on these estimates, a standard random effects estimator is applied to estimate ,
, as well as to predict the values of
and
consistently. Persistent inefficiency
is subsequently estimated using
. By assuming
, the expected value
is parameterized as
, where
is the variance of the persistent inefficiency and
is a vector of unknown parameters to be estimated using the standard SF technique in which
is the dependent variable. We estimate persistent technical efficiency
based on the predictions of
by applying the Jondrow et al. (Citation1982) procedure.
The transient inefficiency is estimated following a similar procedure using
as the dependent variable. We assume
is iid
and
such that
, parameterized as
, where
is the variance of the transient inefficiency and
is a vector of unknown parameters to be estimated. Utilizing Jondrow et al. (Citation1982) and the predictions of
, we estimate transient technical efficiency as
. Overall technical efficiency is calculated as
.
To estimate the empirical model in EquationEquation (2)(2)
(2) , we apply the translog functional form:
where ,
,
, and all other variables are as previously defined.
III. Data
The study uses an unbalanced panel of specialized field crop farms from the Austrian fraction of the EU’s Farm Accountancy Data Network (FADN), which covers 2003 to 2017. The farms included in this dataset are a stratified sample representing the Austrian agricultural sector in terms of standard output, production orientation, and altitude. Here, we focus on farms whose revenues from field crops accounted for at least 65% of total farm revenues, on average, over the observed years. Moreover, we exclude farms that are not observed for a minimum of two consecutive years to maintain the panel data features. Effectively, our utilized sample includes 239 farms and 1,781 observations with farms observed for an average duration of eight years. summarizes the descriptive statistics of the variables used in the study.
Table 1. Descriptive statistics (N = 1,781)
The output is measured as total revenues in euros (€). We aggregate production inputs included in the estimated model into four main categories. Labour is measured in standard annual working units (AWU) and includes family and hired labour. Land captures the total utilized agricultural area, including owned and rented farmland, measured in hectares (ha). Capital (in €) refers to the end-of-year value of buildings, machinery, forestry assets, livestock capital, and assets for other activities related to agriculture. Intermediate inputs (in €) capture all variable costs, including seeds, fertilizers, pesticides, feeding, veterinary services, insurance, bought services, energy, and other expenses for agricultural-related activities. All monetary values are deflated using the appropriate price indices from official statistics, with 2010 as the base year. The output and all input variables are normalized around the sample means, defining the point of approximation.
Generally, the determinants of technical efficiency include characteristics of the farm, production conditions, and attributes of the farm manager. As Lien, Kumbhakar, and Alem (Citation2018) noted, transient and persistent inefficiencies capture diverse aspects of performance. Therefore, it seems logical to use different determinants to capture inefficiency differences among farms.Footnote2 To explain transient inefficiency, we include the following determinants (), which are likely to change over the observed period: i) farm size measured in hectares of arable land cultivated; ii) farm specialization in crop production measured by the share of crop revenues in total farm revenues; iii) total subsidies received by the farm in euro; iv) age of farm manager in years; and v) a time variable to capture the development over time.
In explaining persistent inefficiency, it is logical to use determinants that are relatively stable over time (Lai and Kumbhakar Citation2018b; Lien, Kumbhakar, and Alem Citation2018). To maintain the time-invariant feature, we follow Lai and Kumbhakar (Citation2018b) and use the mean over time of the -variables (except for dummies). We include five determinants of persistent inefficiency: i) the altitude of the farm measured at the point of the farm building; ii) the average soil quality index of a farm assigned for tax purposes as a proxy for the potential soil productivity of a farm’s agricultural area (this index is a number between 1 for the least productive land and 100 for the most productive land); iii) two levels of agricultural education of the farm manager are differentiated (we use a dummy equal to one if the farm manager has a higher level of agricultural education, namely a high school diploma with agricultural background, university in agriculture, or master craft certificate in agriculture, and zero otherwise); iv) share of owned farmland to the total utilized agricultural area; and v). share of family labour in total labour used on the farm. Land ownership and family labour are included as determinants of persistent inefficiency because these indicators are relatively constant over time and do not substantially change from year to year. They intend to capture organizational features of the farm, e.g. ownership structure and family versus corporate farm.
In the agricultural economics literature, the impacts of several economic, socio-economic, and environmental factors on farms’ technical efficiency have been investigated. Drawing from these findings, we hypothesize about the expected effects of the chosen determinants on technical efficiency. First, the impact of farm size on farm performance has been a subject of debate for many decades. In the context of developing countries, an inverse relationship between performance and farm size is often supported (Barrett, Bellemare, and Hou Citation2010; Helfand and Taylor Citation2021). On the other hand, when concentrating on technical efficiency as a performance measure, most studies report a positive correlation in developed countries (Latruffe, Davidova, and Balcombe Citation2008; Bojnec and Latruffe Citation2013). While most of the literature deriving empirical results on this issue make little reference to the underlying economic model, Alvarez and Arias (Citation2004) derive a positive relation between farm size and technical efficiency based on a microeconomic model of production. In summary, they show that more efficient producers buy more variable inputs, use them better, and produce more output. Second, the effect of specialization can be either positive or negative. Efficiency gains can be expected with farm specialization as it increases in-depth knowledge of farm managers and concentrates resources on a single activity (Giannakas, Schoney, and Tzouvelekas Citation2001; Karagiannis and Sarris Citation2005). However, Featherstone, Langemeier, and Ismet (Citation1997) find that specialization decreases efficiency if diversification economies persist. Third, the effects of agricultural subsidies on technical efficiency have been highly debated. On the one hand, some studies found positive impacts of subsidies on technical efficiency through income and insurance effects (Kumbhakar and Lien Citation2010; Lien, Kumbhakar, and Alem Citation2018), yet other studies found that subsidies could potentially result in lesser efforts dedicated to farming activities, adversely affecting efficiency (Bojnec and Latruffe Citation2013; Karagiannis and Sarris Citation2005; Zhu and Lansink Citation2010). Fourth, age is expected to positively affect efficiency if age proxies farm managers’ experience as found in several studies (Karagiannis and Sarris Citation2005; Madau Citation2011). However, an adverse effect is plausible if older farms are unable to adapt to new and improved production technologies, such as digitalization (Featherstone, Langemeier, and Ismet Citation1997; Rasmussen Citation2010), or make less effort in the years before they retire.
Regarding persistent inefficiency determinants, we expect crop farms located at lower altitudes to achieve relatively higher efficiency. As Brümmer (Citation2001) noted, farmers face disadvantaged circumstances at higher altitudes, leading to lower output (for a given level of inputs) than farms operating under more favourable conditions. Furthermore, farms with higher soil quality are less likely to face managerial difficulties regarding soil productivity and thus are more efficient than farms with lower soil quality (Adhikari and Bjorndal Citation2012; Nowak, Kijek, and Domańska Citation2015). The effects of the share of owned land on technical efficiency have typically yielded mixed results. Giannakas, Schoney, and Tzouvelekas (Citation2001) and Latruffe, Davidova, and Balcombe (Citation2008) found that farmers who own their farmland adopted better land management practices due to positive effects of ownership rights, therefore increasing their efficiency. On the other hand, in the presence of secure land tenures, Gavian and Ehui (Citation1999) found that farmers with a higher share of rented land tended to be more motivated towards producing efficiently to recover rental costs. The impact of family labour on efficiency is ambiguous. Family labour could be highly driven towards the success of the farm business, primarily as principal-agent problems, such as moral hazard, associated with hired labour are mitigated (Giannakas, Schoney, and Tzouvelekas Citation2001; Karagiannis and Sarris Citation2005). However, Latruffe, Davidova, and Balcombe (Citation2008) found the opposite and argued that farms typically hire skilled labour to perform specific tasks, potentially increasing efficiency. Finally, we expect education, which proxies farmers’ level of knowledge and skills, to positively influence efficiency (Adhikari and Bjorndal Citation2012).
IV. Results and discussions
Table A.1 in Appendix A presents the estimates of the translog production function. At the point of approximation, the estimated production technology satisfies monotonicity and diminishing marginal product conditions, such that all estimated first-order parameters are positive and less than one. The estimated output elasticities are presented in . The elasticity of land is the largest, followed by intermediate inputs, labour, and capital. Unsurprising, results indicate that crop production is strongly dependent on land. Summing up the output elasticities, we estimate an average scale elasticity of 1.04. This implies that sampled farms exhibit increasing returns to scale. Moreover, an average technical progress of approximately 2.2% per annum is estimated.
Table 2. Output elasticities, returns to scale, and technical change
presents the kernel density distributions of persistent, transient, and overall technical efficiency scores. We estimate a mean transient, persistent, and overall technical efficiency of 0.91, 0.96, and 0.87, respectively.Footnote3 We find that persistent efficiency scores are relatively dense, with 88% (77%) of the farms between 0.90 (0.95) and 1. The transient technical efficiency scores are relatively more dispersed, with only 71% (19%) of the farms between 0.90 (0.95) and 1. This suggests that the transient component poses a greater challenge for some crop farms in Austria. Overall technical efficiency follows a similar pattern as transient efficiency in terms of dispersion, suggesting that efficiency gains are still possible for crop farms in Austria.
Empirical results concerning the determinants of transient and persistent inefficiency are presented in . A negative sign of the coefficients implies a decrease in the variance of the inefficiency function and, therefore, a positive impact on technical efficiency and vice versa. Comparing our results with those from previous research advises some caution, given that only a few studies utilize the four-component model separating transient efficiency from persistent efficiency and firm heterogeneity. Those who apply the four-component model with determinants are not all specifically on crop farms but investigate dairy farms (Trnková and Kroupová Citation2020; Baležentis and Sun Citation2020) and mixed farms (Minviel and Sipiläinen Citation2021) instead. Only Lien, Kumbhakar, and Alem (Citation2018) and Berisso (Citation2019) look at crop farms. The core of previous studies that investigate the determinants of technical efficiency either utilize cross-sectional models as first proposed by Huang and Liu (Citation1994), Kumbhakar, Ghosh, and McGuckin (Citation1991), and Reifschneider and Stevenson (Citation1991) or ‘pseudo’ panel data models introduced by Battese and Coelli (Citation1995). However, this ‘classical’ inefficiency effects model, though designed for use with panel data, is not a panel-data treatment in the classical sense: The inefficiency terms are assumed to be independent over time and observations of a single firm in various time periods are treated as observations of independent firms just as in cross-sectional models. Hence, these models do not account for time-invariant firm heterogeneity or distinguish between transient and persistent inefficiency. The same applies to commonly used two-step approaches which use nonparametric DEA analysis in the first step to derive efficiency scores for single years and regress them on determinants in the second step.Footnote4
Table 3. Determinants of persistent and transient inefficiency in the pre-truncated function
Discussing the transient efficiency results, we find a positive effect of farm size on efficiency. This is in line with our finding of increasing returns to scale and results from Berisso (Citation2019) who estimates a positive effect of scale on the transient efficiency of smallholder cereal farms in Ethiopia. Trnková and Kroupová (Citation2020) relate size to persistent efficiency and cannot identify a positive effect. Based on classical inefficiency effects models, which do not distinguish between transient and persistent efficiency, Zhu and Lansink (Citation2010) found strong positive farm size effects for crop farms in Germany and Sweden but negative effects for those in the Netherlands. Based on DEA and a second stage regression, Bojnec and Latruffe (Citation2013) revealed that size has a positive effect on technical efficiency but a negative effect on allocative efficiency, resulting in a positive effect on overall economic efficiency among Slovenian farms.
In regard to specialization, we find a positive effect on efficiency. Specialization is typically associated with a relatively higher level of skill. Moreover, the added pressure of reliance on income from a single farm activity could provide additional motivation and rationalize the higher technical efficiency observed for specialized farms (Karagiannis and Sarris Citation2005). A positive impact of farms’ specialization on transient technical efficiency is in line with a comparable study by Baležentis and Sun (Citation2020) for Lithuanian dairy farms. Trnková and Kroupová (Citation2020) relate specialization to persistent efficiency and find a positive effect. Looking at studies not specifically measuring transient efficiency, Zhu and Lansink (Citation2010) find a positive impact for crop farms in Sweden and the Netherlands but not Germany. Moreover, positive effects of specialization on technical efficiency are also reported by Karagiannis and Sarris (Citation2005) and Latruffe et al. (Citation2005).
The effect of subsidies on efficiency is one of the most extensively discussed issues in agricultural policy analysis. Minviel and Latruffe (Citation2017) performed a meta-analysis on this issue based on 68 studies and 195 distinct results. They find that slightly more than half of the models find a significant negative effect of subsides, one-quarter yield a significant positive effect, while the rest report non-significant effects. Moreover, they discuss how the observed effect is sensitive to the way subsidies are modelled (e.g. total subsidy value, subsidy as a share of farm revenue, specific types of subsidies, etc.). In addition, Latruffe et al. (Citation2016) find that CAP subsidies have a heterogeneous effect across countries for nine western EU countries over an eighteen-year period. Our results indicate a negative effect of subsidies on transient efficiency, which is in line with results from comparable studies by Minviel and Sipiläinen (Citation2021) (who also estimate a negative impact of subsidies on persistent efficiency), Baležentis and Sun (Citation2020), and Trnková and Kroupová (Citation2020). In regard to Austria, it has to be mentioned that a high share of agricultural subsidies is through agri-environmental programmes. In the case of crop farms, on average, about 37% of total subsidies were agri-environmental payments in 2017 (BMLFUW Citation2018). These payments are often linked to restrictions on the production system (i.e. restricted use of chemical fertilizer) or require additional inputs (i.e. planting cover crops).
Furthermore, we find an inverse U-shaped effect of age on transient technical efficiency, which can be explained by learning-by-doing, especially in the first years of management. However, we see that this effect decreases with age and calculate that the turning point, on average, is at age 51, which is close to the sample’s average age, 50. An inverse U-shaped effect of age on transient efficiency is also detected by Berisso (Citation2019); Baležentis and Sun (Citation2020) do not include a squared age variable and estimate a positive effect.
Lastly, we find that transient technical efficiency declines over time. This may indicate that the sector is diverging. While some farms are active in adopting new technologies and pushing the frontier, as indicated by significant technological progress, others probably remain passive and are unable to catch up (Hadley Citation2006). Our findings are in line with results by Baležentis and Sun (Citation2020) but contrast those of Berisso (Citation2019) and Minviel and Sipiläinen (Citation2021), who find transient efficiency to increase over time.
Regarding the effects of environmental factors on persistent efficiency, we find a negative impact of altitude and a positive effect of soil quality. The only other study investigating the effects of environmental factors on persistent efficiency is Berisso (Citation2019). The study finds a significant impact of the location of farms in different agro-ecological zones, defined by altitude and other factors. In the context of Austria, the negative effect for altitude and the positive effect of soil quality is unsurprising and in line with many other studies that do not explicitly analyse persistent efficiency but instead examine technical efficiency. In particular, Kourtesi, De Witte, and Polymeros (Citation2016), Madau (Citation2007), and Brümmer (Citation2001) find a negative impact of altitude and Adhikari and Bjorndal (Citation2012), Latruffe et al. (Citation2004), and Nowak, Kijek, and Domańska (Citation2015) find a positive effect of soil quality.
We observe a positive relationship between land tenancy and persistent efficiency. Specifically, we find that the greater the share of owned land, the lower the persistent efficiency. To the best of our knowledge, no other study investigates the link between the share of owned land and persistent efficiency. However, Trnková and Kroupová (Citation2020) report positive effects of the share of rented land on transient technical efficiency. According to this study, a higher share of rented land may motivate farmers to work harder due to the obligation to pay rent. Results for studies that do not distinguish between transient and persistent efficiency are mixed. For example, Kourtesi, De Witte, and Polymeros (Citation2016) report a positive impact of the share of rented land on the technical efficiency of cereal farms in Greece, while Giannakas, Schoney, and Tzouvelekas (Citation2001) found the opposite for that wheat farms in Saskatchewan. They argue that agency problems resulting from information asymmetries and misaligned motives between the contracting parties could explain this finding. Similarly, Latruffe, Davidova, and Balcombe (Citation2008) and Hadley (Citation2006) found that the share of rented land negatively impacted crop farms’ technical efficiency in the Czech Republic, England, and Wales. According to Giannakas, Schoney, and Tzouvelekas (Citation2001), the effects of land tenancy on efficiency in the agricultural sector seem to depend mainly on dynamics within land markets and the nature of tenancy agreements. In Austria, Leonhardt, Braito, and Penker (Citation2021) found that land rentals are secured and long-term. Therefore, in the absence of agency problems and well-functioning land markets, farms with more rented land are more likely to use all inputs more efficiently to recover costs, including land rents (Gavian and Ehui Citation1999; Kourtesi, De Witte, and Polymeros Citation2016).
We find that the share of family labour affects persistent technical efficiency positively. In Austria, farms are typically inherited, making family labour the residual claimant, providing additional long-term incentives to run the farm efficiently. Again, no other study has investigated the effect of the share of family labour on persistent efficiency. However, Trnková and Kroupová (Citation2020) investigated the impact of hired labour on persistent efficiency and found a positive effect. Looking at technical efficiency in general rather than persistent efficiency, Giannakas, Schoney, and Tzouvelekas (Citation2001) found that family farms attained higher efficiency and argued that family labour tends to be more motivated to mitigate potential agency problems (i.e. moral hazard and suboptimal performance) typically associated with hired labour. Similarly, Kourtesi, De Witte, and Polymeros (Citation2016) and Latruffe, Davidova, and Balcombe (Citation2008), using DEA techniques, found that among crop farms in Greece and the Czech Republic, respectively, farms with higher shares of hired labour in total labour achieved higher technical efficiency levels than family-farms. The authors argued that the nature of contractual agreements and comparatively higher qualifications associated with hired farm labour could account for the increase in efficiency.
Finally, the results show a negative association between persistent inefficiency and the educational level, although this relationship is insignificant.
V. Conclusions
Given natural disadvantages and the structure of the sector, (crop) farms in Austria have to strive to be able to compete in an increasingly competitive business environment. Though the CAP still provides income support through decoupled direct payments, domestic product prices are now closely linked to the world market. Hence, the need to be more efficient becomes a precondition to survive in an increasingly competitive environment. Government can support farms in their attempts to become more competitive through different policies. To be able to do this, it is crucial to identify the sources of inefficiency.
To obtain a comprehensive view of the technical efficiency of crop farms in Austria, we apply the four-component SF model proposed by Kumbhakar, Lien, and Hardaker (Citation2014), which decomposes technical efficiency into a transient and persistent component while controlling for firm heterogeneity and random shocks. We extend this model to correct any potential heterogeneity bias by implementing the Mundlak (Citation1978) adjustments and including exogenous determinants of transient and persistent inefficiency.
We apply our model to an unbalanced panel of 239 crop farms from 2003 to 2017. Based on our results, we derive several conclusions and policy recommendations. First, from a methodological perspective, farm heterogeneity is correlated with the explanatory variables, i.e. input levels. Therefore, including the Mundlak (Citation1978) adjustment terms to the random effects is necessary to ensure that the estimated slope parameters of the production function and efficiency terms are consistent and unbiased.
Second, we estimate a mean overall technical efficiency of 0.87, implying a potential for improvement. Overall, technical efficiency is a product of a transient technical efficiency of 0.91 and a persistent efficiency of 0.96. The majority of farms (83%) attained a persistent efficiency level that was higher than the transient component. These findings indicate that although improvements in persistent efficiency are still possible, transient inefficiency is more of a challenge for crop farms. Hence, the government should concentrate on helping farmers to overcome transient inefficiencies.
Third, concerning the determinants of persistent technical efficiency, we observe that farms with favourable natural conditions (i.e. lower altitudes and higher soil quality), a higher share of family labour, and a lower share of owned land (i.e. a higher rental share), are more persistently efficient. To find a negative impact of unfavourable natural conditions is no surprise and undisputable. In Austria, as in most other EU countries, unfavourable production conditions are often compensated by so-called less favoured area payments. Our results rationalize such payments if the goal is to keep agricultural production in such areas.
The finding that farms with a higher share of family labour are more efficient is important since family farms are the politically favoured form of farm organization in Austria, as outlined, for example, by every elected government during the last several decades as shown in their programs (e.g. Republik Österreich (Citation2020) and Republik Österreich (Citation2017)) and statements by agricultural interest groups (Langer-Wenninger Citation2021). Our results show that this goal does not infringe on farm performance. Moreover, a common way to support family farms, in particular, is through social insurance and the tax system.
As in many other EU countries, agricultural land sales markets are relatively thin (Ciaian, Kancs, and Espinosa Citation2016). In Austria, only 0.25% of the total UAA are sold each year (Fankhauser et al. Citation2016). Given the decreasing quantity of available UAA, growing farm size, and thin land sales markets, agricultural land renting has gained importance over the past few decades. The share of farms that farm only their owned land and do not rent is, on average, 38% for Austria, but only around 25% in the main crop farming regions of Lower Austria and Burgenland (Statistik Austria Citation2018). Hence, it is an important observation that high rental shares do not impair technical efficiency. This result may be closely related to the fact that, according to Leonhardt, Braito, and Penker (Citation2021), rental contracts in Austria are very secure and mostly long-term.
Fourth, in regard to the determinants of transient technical efficiency, we find that more specialized, larger farms managed by farmers at a certain age (i.e. maximum efficiency is at age 51) and are less dependent on subsidies are more efficient in the short-run. Crop farms in Austria are relatively small compared to farms in many other EU countries. For example, in 2014, the average arable crop farm (as defined by the EU classification) in neighbouring Germany had 141 ha UAA and € 229,000 standard output (a monetary measure of farm size as defined by the EU) while the average comparable farm in Austria had 31 ha and about € 41,000 standard output (BMEL Citation2015; BMLFUW Citation2016). Given our results, the government should encourage small- to medium-scale farms to increase their size. Most important in this regard, particularly for crop farms, is probably access to and the affordability of land. Moreover, given our results regarding the effect of the share of owned land on efficiency, land rentals could be a mechanism farms could use to increase their sizes. However, according to Eurostat (Citation2018), Austria had the third-highest rental prices of the 20 Member States for which data was available in 2016. This makes it expensive for farms to expand. While in a few EU countries (e.g. Belgium and France), a maximum rental price is defined by law, this is not the case in Austria where sales and renting land are subject to the general freedom of contract (Salhofer and Leonhardt ; Vranken, Tabeau, and Roebeling Citation2021).
The impact of subsidies on farm performance is controversially discussed in the literature. For example, in a study estimating the technical efficiency of dairy farms in nine different EU countries, Latruffe et al. (Citation2016) found negative effects for three countries, positive effects for three countries, and no effect for three countries. However, they also found that the decoupling of subsidies from production decisions in the 2003 CAP reform weakened the impact of subsidies on technical efficiency. Our results, mainly covering the period of decoupled subsidies from 2005 onwards, confirm a negative impact of subsidies on farm performance. Moreover, there is ample evidence that decoupled CAP payments capitalize into land (rental) prices to some extent (Ciaian et al. Citation2021; Salhofer and Feichtinger Citation2020; Varacca et al. Citation2021). Hence, this makes it more expensive for farms to increase in size. Furthermore, in the last two CAP reforms (2005 and 2015), each farmer was allocated a certain number of tradable entitlements with a specific value, which can be activated if that farmer owns or rents at least the same number of eligible hectares. Thus, governments can decrease the degree of capitalization of subsides into land values by making entitlements more abundant with respect to eligible hectares (Ciaian, Kancs, and Swinnen Citation2008; Courleux et al. Citation2008; Kilian and Salhofer Citation2008).
Finally, we observe significant technical progress, coupled with a decrease in average technical efficiency. This points to the potential of some farms to increase productivity and competitiveness while others fall behind. Technological progress is closely linked to investments. Between 2007 and 2013, approximately one quarter (30,000) of farms received almost € 600 million in total (€ 20,000 per farm) of non-refundable investment aid (BMLFUW Citation2018). From a policy perspective, the crucial question is whether this considerable budget should be spent on farmers who are falling behind or on the front-runners. The latter would probably spur productivity and efficiency of the sector but would further increase structural change.
Acknowledgments
We presented earlier versions of this paper at the XVI European Workshop on Efficiency and Productivity Analyses, the 59th Annual Conference of the GEWISOLA (German Association of Agricultural Economics) and the XVI EAAE Congress and are thankful to the discussants and participants for their valuable comments. We are also grateful to the editor and anonymous reviewers; their suggestions and comments helped us improve the paper significantly. We thank Gudbrand Lien for providing help with stata codes and Thea Nielsen Ritter for her careful editing. The project was supported by the Austrian Science Fund (FWF) under project number I 3505. The research was conducted as part of the Research Group FORLand supported by the Deutsche Forschungsgemeinschaft (DFG, German Research Foundation) - 317374551 (https://www.forland.hu-berlin.de). We are grateful to the Austrian Federal Ministry for Agriculture, Regions and Tourism for providing data.
Disclosure statement
No potential conflict of interest was reported by the author(s).
Additional information
Funding
Notes
1 Although our production function in EquationEquation (1)(1)
(1) does not include a vector of time-invariant environmental variables as discussed in Good et al. (Citation1993), it can easily accommodate this extension. In the spirit of Battese and Coelli (Citation1995), we include environmental variables as determinants of persistent technical efficiency. Following Coelli, Perelman, and Romano (Citation1999), it is also possible to include environmental variables in the production function as efficiency determinants.
2 Heshmati, Kumbhakar, and Kim (Citation2018) and Lai and Kumbhakar (Citation2018b) used a different set of determinants to explain both transient and persistent technical efficiency differences, while Colombi, Martini, and Vittadini (Citation2017) and Lai and Kumbhakar (Citation2018a) used the same determinants for both.
3 Based on the multi-step four-component model without determinants similar to Kumbhakar, Lien, and Hardaker (Citation2014), we estimate a mean transient, persistent, and overall technical effciency of 0.91, 0.93, and 0.85, respectively, with similar kernel distributions. Results are available upon request.
4 More recent studies, which are able to distinguish between transient and persistent efficiency (Kumbhakar and Heshmati Citation1995) or between firm heterogeneity and transient inefficiency (Green’s (Citation2005a; Citation2005b) true and random effects models), rarely include inefficiency determinants.
References
- Acosta, A., and L. A. De Los Santos-Montero. 2019. “What Is Driving Livestock Total Factor Productivity Change? A Persistent and Transient Efficiency Analysis.” Global Food Security 21: 1–12. doi:https://doi.org/10.1016/j.gfs.2019.06.001.
- Adhikari, C. B., and T. Bjorndal. 2012. “Analyses of Technical Efficiency Using SDF and DEA Models: Evidence from Nepalese Agriculture.” Applied Economics 44 (25): 3297–3308. doi:https://doi.org/10.1080/00036846.2011.572856.
- Agasisti, T., and S. Gralka. 2019. “The Transient and Persistent Efficiency of Italian and German Universities: A Stochastic Frontier Analysis.” Applied Economics 51 (46): 5012–5030. doi:https://doi.org/10.1080/00036846.2019.1606409.
- Aigner, D., C. A. K. Lovell, and P. Schmidt. 1977. “Formulation and Estimation of Stochastic Frontier Production Function Models.” Journal of Econometrics 6 (1): 21–37. doi:https://doi.org/10.1016/0304-4076(77)90052-5.
- Alvarez, A., and C. Arias. 2004. “Technical Efficiency and Farm Size: A Conditional Analysis.” Agricultural Economics 30 (3): 241–250. doi:https://doi.org/10.1111/j.1574-0862.2004.tb00192.x.
- Andor, M., and C. Parmeter. 2017. “Pseudolikelihood Estimation of the Stochastic Frontier Model.” Applied Economics 49 (55): 5651–5661. doi:https://doi.org/10.1080/00036846.2017.1324611.
- Badunenko, O., and S. C. Kumbhakar. 2017. “Economies of Scale, Technical Change and Persistent and Time-varying Cost Efficiency in Indian Banking: Do Ownership, Regulation and Heterogeneity Matter?” European Journal of Operational Research 260 (2): 789–803. doi:https://doi.org/10.1016/j.ejor.2017.01.025.
- Baležentis, T., and K. Sun. 2020. “Measurement of Technical Inefficiency and Total Factor Productivity Growth: A Semiparametric Stochastic Input Distance Frontier Approach and the Case of Lithuanian Dairy Farms.” European Journal of Operational Research 285 (3): 1174–1188. doi:https://doi.org/10.1016/j.ejor.2020.02.032.
- Barrett, C. B., M. F. Bellemare, and J. Y. Hou. 2010. “Reconsidering Conventional Explanations of the Inverse Productivity–size Relationship.” World Development 38 (1): 88–97. doi:https://doi.org/10.1016/j.worlddev.2009.06.002.
- Battese, G. E., and T. J. Coelli. 1992. “Frontier Production Functions, Technical Efficiency and Panel Data: With Application to Paddy Farmers in India.” Journal of Productivity Analysis 3 (1–2): 153–169. doi:https://doi.org/10.1007/BF00158774.
- Battese, G. E., and T. J. Coelli. 1995. “A Model for Technical Inefficiency Effects in A Stochastic Frontier Production Function.” Empirical Economics 20 : 325–332. doi:https://doi.org/10.1007/BF01205442.
- Berisso, O. 2019. “Analysis of Factors Affecting Persistent and Transient Inefficiency of Ethiopia’s Smallholder Cereal Farming.” In Efficiency, Equity and Well-Being in Selected African Countries, edited by P. Nilsson, and A. Heshmati, 199–228, Cham: Springer.
- BMEL. 2015. Agrarpolitischer Bericht. Bundesminister für Ernährung und Landwirtschaft. Berlin: BMEL.
- BMLFUW. 2016. Grüner Bericht 2016. Bericht über die Situation der Österreichischen Land- und Forstwirtschaft. Vienna: BMLFUW.
- BMLFUW. 2018. Grüner Bericht 2018. Bericht über die Situation der Österreichischen Land- und Forstwirtschaft. Vienna: BMLFUW.
- Bojnec, Š., and L. Latruffe. 2013. “Farm Size, Agricultural Subsidies and Farm Performance in Slovenia.” Land Use Policy 32: 207–217. doi:https://doi.org/10.1016/j.landusepol.2012.09.016.
- Bokusheva, R., and L. Čechura. 2017. Evaluating Dynamics, Sources and Drivers of Productivity Growth at the Farm Level. OECD Food, Agriculture and Fisheries Papers (Issue No.106) . Paris: OECD Publishing.
- Brümmer, B. 2001. “Estimating Confidence Intervals for Technical Efficiency: The Case of Private Farms in Slovenia.” European Review of Agriculture Economics 28 (3): 285–306. doi:https://doi.org/10.1093/erae/28.3.285.
- Ciaian, P., D. Kancs, and J. F. M. Swinnen (2008). Static and Dynamic Distributional Effects of Decoupled Payments: Single Farm Payments in the European Union. In LICOS Discussion Paper No. 207.
- Ciaian, P., D. Kancs, and M. Espinosa. 2016. The Impact of the 2013 CAP Reform on the Decoupled Payments’ Capitalization into Land Values. Joint Research Centre, European Commission, EUR 27940 EN . Luxembourg: Publications Office of the European Union.
- Ciaian, P., E. Baldoni, K. d’Artis, and D. Drabik. 2021. “The Capitalization of Agricultural Subsidies into Land Prices.” Annual Review of Resource Economics 13 (1): 1–22. doi:https://doi.org/10.1146/annurev-resource-102020-100625.
- Coelli, T. J., S. Perelman, and E. Romano. 1999. “Accounting for Environmental Influences in Stochastic Frontier Models: With Application to International Airlines.” Journal of Productivity Analysis 11 (3): 251–273. doi:https://doi.org/10.1023/A:1007794121363.
- Colombi, R., G. Martini, and G. Vittadini. 2017. “Determinants of Transient and Persistent Hospital Efficiency: The Case of Italy.” Health Economics (United Kingdom) 26: 5–22. doi:https://doi.org/10.1002/hec.3557.
- Colombi, R., S. C. Kumbhakar, G. Martini, and G. Vittadini. 2014. “Closed-skew Normality in Stochastic Frontiers with Individual Effects and Long/short-run Efficiency.” Journal of Productivity Analysis 42 (2): 123–136. doi:https://doi.org/10.1007/s11123-014-0386-y.
- Cooper, T., D. Baldock, M. Rayment, T. Kuhmonen, I. Terluin, V. Swales, X. Poux, D. Zakeossian, and M. Farmer. 2006. An Evaluation of the Less Favoured Area Measure in the 25 Member States of the European Union. Brussels: Institute for European Environmental Policy.
- Cornwell, C., P. Schmidt, and R. C. Sickles. 1990. “Production Frontiers with Cross-sectional and Time-series Variation in Efficiency Levels.” Journal of Econometrics 46 (1–2): 185–200. doi:https://doi.org/10.1016/0304-4076(90)90054-W.
- Courleux, F., H. Guyomard, F. Levert, and L. Piet (2008). How the EU Single Farm Payment Should Be Modelled: Lump-sum Transfers, Area Payments or … What Else? In Working Paper SMART – LERECO N°08-01.
- Eder, A., K. Salhofer, and E. Scheichel. 2021. “Land Tenure, Soil Conservation, and Farm Performance: An Eco-efficiency Analysis of Austrian Crop Farms.” Ecological Economics 180: 106861. doi:https://doi.org/10.1016/j.ecolecon.2020.106861.
- Eurostat. (2018). Agricultural Land Prices and Rents. In Newsrelease 48/2018. http://ec.europa.eu/eurostat/documents/2995521/8756523/5-21032018-AP-N.pdf/b1d0ffd3-f75b-40cc-b53f-f22f68d541df
- Fankhauser, J., R. Günther, H. Mayrhofer, A. Reinl, E. Reisinger, T. Resl, and J. Schauer. 2016. “Entwicklungen am Bodenmarkt: Aktuelle Situation und Empfehlungen. Endbericht der AG Bodenmarkt.” Bericht Im Auftrag Der Landesagrarreferenten-Konferenz.
- Farsi, M., M. Filippini, and M. Kuenzle. 2005. “Unobserved Heterogeneity in Stochastic Cost Frontier Models: An Application to Swiss Nursing Homes.” Applied Economics 37 (18): 2127–2141. doi:https://doi.org/10.1080/00036840500293201.
- Featherstone, A. M., M. R. Langemeier, and M. Ismet. 1997. “A Nonparametric Analysis of Efficiency for A Sample of Kansas Beef Cow Farms.” Journal of Agricultural and Applied Economics 29 (1): 175–184. doi:https://doi.org/10.1017/S1074070800007653.
- Filippini, M., and W. Greene. 2016. “Persistent and Transient Productive Inefficiency: A Maximum Simulated Likelihood Approach.” Journal of Productivity Analysis 45 (2): 187–196. doi:https://doi.org/10.1007/s11123-015-0446-y.
- Gavian, S., and S. Ehui. 1999. “Measuring the Production Efficiency of Alternative Land Tenure Contracts in a Mixed Crop-livestock System in Ethiopia.” Agricultural Economics 20 (1): 37–49.
- Giannakas, K., R. Schoney, and V. Tzouvelekas. 2001. “Technical Efficiency, Technological Change and Output Growth of Wheat Farms in Saskatchewan.” Canadian Journal of Agricultural Economics 49 (2): 135–152. doi:https://doi.org/10.1111/j.1744-7976.2001.tb00295.x.
- Good, D., M. Nadiri, L. H. Roller, and R. C. Sickles. 1993. “Efficiency and Productivity Growth Comparisons of European and U.S. Air Carriers: A First Look at the Data.” Journal of Productivity Analysis 4: 115–125. doi:https://doi.org/10.1007/BF01073469.
- Greene, W. 2005a. “Fixed and Random Effects in Stochastic Frontier Models.” Journal of Productivity Analysis 23 (1): 7–32. doi:https://doi.org/10.1007/s11123-004-8545-1.
- Greene, W. 2005b. “Reconsidering Heterogeneity in Panel Data Estimators of the Stochastic Frontier Model.” Journal of Econometrics 126 (2): 269–303. doi:https://doi.org/10.1016/j.jeconom.2004.05.003.
- Griliches, Z. 1963. “Estimation of the Aggregate Production Function from Cross-sectional Data.” Journal of Farm Economics 45: 419–428. doi:https://doi.org/10.2307/1235997.
- Hadley, D. 2006. “Patterns in Technical Efficiency and Technical Change at the Farm-level in England and Wales, 1982–2002.” Journal of Agricultural Economics 57 (1): 81–100. doi:https://doi.org/10.1111/j.1477-9552.2006.00033.x.
- Helfand, S. M., and M. P. H. Taylor. 2021. “The Inverse Relationship between Farm Size and Productivity: Refocusing the Debate.” Food Policy 99:101977 .
- Heshmati, A., S. C. Kumbhakar, and J. Kim. 2018. “Persistent and Transient Efficiency of International Airlines.” European Journal of Transport and Infrastructure Research 18 (2): 213–238.
- Huang, C. J., and J. -T. Liu. 1994. “Estimation of a Non-Neutral Stochastic Frontier Production Function.” Journal of Productivity Analysis 5 (2): 171–180. doi:https://doi.org/10.1007/BF01073853.
- Jondrow, J., C. A. K. Lovell, I. S. Materov, and P. Schmidt. 1982. “On the Estimation of Technical Inefficiency in the Stochastic Frontier Production Function Model.” Journal of Econometrics 19: 233–238. doi:https://doi.org/10.1016/0304-4076(82)90004-5.
- Karagiannis, G., and A. Sarris. 2005. “Measuring and Explaining Scale Efficiency with the Parametric Approach: The Case of Greek Tobacco Growers.” Agricultural Economics 33 (s3): 441–451. doi:https://doi.org/10.1111/j.1574-0864.2005.00084.x.
- Karagiannis, G. 2014. “Modeling Issues in Applied Efficiency Analysis: Agriculture.” Economics and Business Letters 3 (1): 12–18. doi:https://doi.org/10.17811/ebl.3.1.2014.12-18.
- Kilian, S., and K. Salhofer. 2008. “Single Payments of the CAP: Where Do the Rents Go?” Agricultural Economics Review 9 (2): 96–106.
- Kourtesi, S., K. De Witte, and A. Polymeros. 2016. “Technical Efficiency in the Agricultural sector-Evidence from a Conditional Quantile - Based Approach.” Agricultural Economics Review 17 (2): 100–123.
- Kumbhakar, S. C., and A. Heshmati. 1995. “Efficiency Measurement in Swedish Dairy Farms: An Application of Rotating Panel Data, 1976-88.” American Journal of Agricultural Economics 77 (3): 660–674. doi:https://doi.org/10.2307/1243233.
- Kumbhakar, S. C., and C. F. Parmeter. 2019. ”Implementing Generalized Panel Data Stochastic Frontier Estimators.” Tsionas, M. ed. Panel Data Econometrics: Theory, edit byElsevier 225–249.
- Kumbhakar, S. C., G. Lien, and J. B. Hardaker. 2014. “Technical Efficiency in Competing Panel Data Models: A Study of Norwegian Grain Farming.” Journal of Productivity Analysis 41 (2): 321–337. doi:https://doi.org/10.1007/s11123-012-0303-1.
- Kumbhakar, S. C., and G. Lien. 2010. “Impact of Subsidies on Farm Productivity and Efficiency.” In The Economic Impact of Public Support to Agriculture, an International Perspective, edited by V. E. Ball, R. Fanfani, and G. Luciano, 109–124, New York: Springer.
- Kumbhakar, S. C., and L. Hjalmarsson. 1993. “Technical Efficiency and Technical Progress in Swedish Dairy Farms.” In The Measurement of Productive Efficiency and Productivity Change—techniques and Applications, edited by H. O. Fried, C. A. K. Lovell, and S. S. Schmidt, 256–270, New York: Oxford University Press.
- Kumbhakar, S. C., S. Ghosh, and J. T. McGuckin. 1991. “A Generalized Production Frontier Approach for Estimating Determinants of Inefficiency in U.S. Dairy Farms.” Journal of Business and Economic Statistics 9 (3): 279–286.
- Kumbhakar, S. C. 1987. “The Specification of Technical and Allocative Inefficiency in Stochastic Production and Profit Frontiers.” Journal of Econometrics 34 (3): 335–348. doi:https://doi.org/10.1016/0304-4076(87)90016-9.
- Kumbhakar, S. C. 1990. “Production Frontiers, Panel Data, and Time-varying Technical Inefficiency.” Journal of Econometrics 46 (1–2): 201–211. doi:https://doi.org/10.1016/0304-4076(90)90055-X.
- Lai, H., and S. C. Kumbhakar. 2018a. “Endogeneity in Panel Data Stochastic Frontier Model with Determinants of Persistent and Transient Inefficiency.” Economics Letters 162 (2): 5–9. doi:https://doi.org/10.1016/j.econlet.2017.10.003.
- Lai, H., and S. C. Kumbhakar. 2018b. “Panel Data Stochastic Frontier Model with Determinants of Persistent and Transient Inefficiency.” European Journal of Operational Research 271 (2): 746–755. doi:https://doi.org/10.1016/j.ejor.2018.04.043.
- Langer-Wenninger, M. 2021. Für bäuerliche Familienbetriebe eine positive Zukunftsperspektive schaffen.Landwirtschaftskammer Oberösterreich .https://www.google.com/search?client=firefox-b-d&q=F%C3%BCr+b%C3%A4uerliche+Familienbetriebe+eine+positive+Zukunftsperspektive+schaffen .
- Latruffe, L., B. E. Bravo-Ureta, A. Carpentier, Y. Desjeux, and V. H. Moreira. 2016. “Subsidies and Technical Efficiency in Agriculture: Evidence from European Dairy Farms.” American Journal of Agricultural Economics 99 (3): 783–799. doi:https://doi.org/10.1093/ajae/aaw077.
- Latruffe, L., K. Balcombe, S. Davidova, and K. Zawalinska. 2004. “Determinants of Technical Efficiency of Crop and Livestock Farms in Poland.” Applied Economics 36 (12): 1255–1263. doi:https://doi.org/10.1080/0003684042000176793.
- Latruffe, L., K. Balcombe, S. Davidova, and K. Zawalinska. 2005. “Technical and Scale Efficiency of Crop and Livestock Farms in Poland: Does Specialization Matter?” Agricultural Economics 32 (3): 281–296. doi:https://doi.org/10.1111/j.1574-0862.2005.00322.x.
- Latruffe, L., S. Davidova, and K. Balcombe. 2008. “Application of a Double Bootstrap to Investigation of Determinants of Technical Efficiency of Farms in Central Europe.” Journal of Productivity Analysis 29 (2): 183–191. doi:https://doi.org/10.1007/s11123-007-0074-2.
- Leonhardt, H., M. Braito, and M. Penker. 2021. “Why Do Farmers Care about Rented Land? Investigating the Context of Farmland Tenure.” Journal of Soil and Water Conservation 76 (1): 89–102. doi:https://doi.org/10.2489/jswc.2021.00191.
- Lien, G., S. C. Kumbhakar, and H. Alem. 2018. “Endogeneity, Heterogeneity, and Determinants of Inefficiency in Norwegian Crop-producing Farms.” International Journal of Production Economics 201: 53–61. doi:https://doi.org/10.1016/j.ijpe.2018.04.023.
- Madau, F. A. 2007. “Technical Efficiency in Organic and Conventional Farming: Evidence Form Italian Cereal Farms.” Agricultural Economics Review 8 (1): 5–22.
- Madau, F. A. 2011. “Parametric Estimation of Technical and Scale Efficiencies in Italian Citrus Farming.” Agricultural Economics Review 12 (1): 91–111.
- Meeusen, W., and J. Van Den Broeck. 1977. “Efficiency Estimation from Cobb-Douglas Production Functions with Composed Error.” International Economic Review 18 (2): 435–444. doi:https://doi.org/10.2307/2525757.
- Minviel, J. J., and L. Latruffe. 2017. “Effect of Public Subsidies on Farm Technical Efficiency: A Meta-analysis of Empirical Results.” Applied Economics 49 (2): 213–226. doi:https://doi.org/10.1080/00036846.2016.1194963.
- Minviel, J. J., and T. Sipiläinen. 2021. “A Dynamic Stochastic Frontier Approach with Persistent and Transient Inefficiency and Unobserved Heterogeneity.” Agricultural Economics 52 (4): 575–589.
- Mundlak, Y. 1978. “On the Pooling of Time Series and Cross Section Data.” Econometrica 46 (1): 69–85. doi:https://doi.org/10.2307/1913646.
- Nowak, A., T. Kijek, and K. Domańska. 2015. “Technical Efficiency and Its Determinants in the European Union Agriculture.” Agricultural Economics (Czech Republic) 61 (6): 275–283.
- Pisulewski, A., and J. Marzec. 2019. “Heterogeneity, Transient and Persistent Technical Efficiency of Polish Crop Farms.” Spanish Journal of Agricultural Research 17 (1): 1–14. doi:https://doi.org/10.5424/sjar/2019171-13926.
- Pitt, M. M., and L.-F. Lee. 1981. “The Measurement and Sources of Technical Inefficiency in the Indonesian Weaving Industry.” Journal of Development Economics 9 (1): 43–64. doi:https://doi.org/10.1016/0304-3878(81)90004-3.
- Rasmussen, S. 2010. “Scale Efficiency in Danish Agriculture: An Input Distance-function Approach.” European Review of Agricultural Economics 37 (3): 335–367. doi:https://doi.org/10.1093/erae/jbq023.
- Reifschneider,D., and R. Stevenson. 1991. “Systematic Departures from the Frontier: A Framework for the Analysis of Firm Inefficiency.” International Economic Review 32 (3): 715–723. doi:https://doi.org/10.2307/2527115.
- Republik Österreich. (2017). Zusammen für Österreich. Regierungsprogramm 2017 - 2020. Vienna: Bundesnzleramt Österreich.
- Republik Österreich. 2020. Aus Verantwortung für Österreich. Regierungsprogramm 2020 – 2024 Vienna: Bundeskanzleramt.
- Robinson, P. M. 1988. “Root-N-Consistent Semiparametric Regression.” Econometrica 56 (4): 931–954. doi:https://doi.org/10.2307/1912705.
- Salhofer, K., and P. Feichtinger. 2020. “Regional Differences in the Capitalisation of First and Second Pillar Payments of the CAP into Land Rental Prices.” European Review of Agricultural Economics 48 (1): 8–41.
- Schmidt, P., and R. C. Sickles. 1984. “Production Frontiers and Panel Data.” Journal of Business and Economic Statistics 2 (4): 367–374.
- Statistik Austria. 2018. Agrarstrukturerhebung 2016. Vienna: Statistik Austria.
- Sun, H., B. K. Edziah, X. Song, A. K. Kporsu, and F. Taghizadeh-Hesary. 2020. “Estimating Persistent and Transient Energy Efficiency in Belt and Road Countries: A Stochastic Frontier Analysis.” Energies 13 (15): 15. doi:https://doi.org/10.3390/en13153837.
- Trnková, G., and Z. Ž. Kroupová. 2020. “Determinants of Persistent and Transient Technical Efficiency of Milk Production in EU.” E and M: Economics and Management 23 (4): 39–54.
- Tsionas, E. G., and S. C. Kumbhakar. 2014. “Firm Heterogeneity, Persistent and Transient Technical Inefficiency: A Generalized True Random-effects Model.” Journal of Applied Econometrics 29 (1): 110–132. doi:https://doi.org/10.1002/jae.2300.
- Varacca, A., G. Guastella, S. Pareglio, and P. Sckokai. 2021. “A Meta-analysis of the Capitalisation of CAP Direct Payments into Land Prices.” European Review of Agricultural Economics: 1–24.
- Vranken, L., E. Tabeau, and P. Roebeling, and Associates, with contributions from country experts and. (2021). Deliverable 3: Final Report on Data and Information on Agricultural Land Market Regulations across EU MS “ Agricultural land market regulations in the EU Member States.
- Zhu, X., and A. O. Lansink. 2010. “Impact of CAP Subsidies on Technical Efficiency of Crop Farms in Germany, the Netherlands and Sweden.” Journal of Agricultural Economics 61 (3): 545–564. doi:https://doi.org/10.1111/j.1477-9552.2010.00254.x.
Appendix
Table A1: Estimates of the translog production function