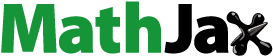
ABSTRACT
This study aims to contribute to the recent literature on the effects of COVID on football teams’ performance, focusing on the impact of ghost games on offensive and defensive technical efficiency. Using season-level data for the top 5 European leagues, a novelty for efficiency studies on football, the analysis compares the ten seasons played before the pandemic outbreak with the only season (2020–21) almost entirely played behind closed doors. A further novel contribution is the methodology – conditional order-m – applied to calculate efficiency scores. Our results show that in the post-COVID season both offensive and defensive efficiency significantly increased for away games, whereas for home games offensive efficiency shows a very slight increase, and defensive efficiency remains basically unchanged. These findings are valid for all the five leagues and provide evidence of a generalized reduction in the home advantage.
I. Introduction
In every competitive industry, firms and organizations constantly try to efficiently use their resources. This implies that, given specific amounts of factor inputs, an organization, or a decision-making unit (DMU) must generate the maximum potential outputs (output-oriented technical efficiency) or, for given specific amounts of outputs, must utilize the minimum necessary inputs (input-oriented technical efficiency), considering constraints such as technical relationships. This is our definition of technical efficiency, fully consistent with the relevant literature (see, e.g. Coelli et al. Citation2005). The football industry also follows this line of conduct as football clubs constantly analyse and appraise their performance on-and-off the pitch in different managerial scenarios, from buying football players to signing commercial deals.
The connection between on-field statistics and success in football has long been the focus of research in sport economics (notable examples include Castellano, Casamichana, and Lago Citation2012; Lepschy, Wäsche, and Woll Citation2020). In this article, we focus on a particular domain of this wide field, the analysis of on-field technical efficiency. As argued in Carmichael and Thomas (Citation2014), this analysis allows the assessment of whether clubs make an effective use of their sporting resources compared to their potential, thus helping the identification of contextual factors critically affecting this effective use, as well as of opportunities for improvement. We rely on data routinely produced and stored on the website www.whoscored.com to estimate football clubs’ on-field technical efficiency and evaluate the impact on this efficiency of the so-called ghost matches policy brought about by the COVID-19 pandemic. We construct and use a panel dataset over a period of twelve seasons from 2009–10 to 2020–21 for the top 5 European leagues (English Premier League, Spanish La Liga, Italian Serie A, German Bundesliga and French Ligue 1), contributing to the operational research literature in sport in three main aspects.
We calculate efficiency scores by adopting the conditional order-m approach (Daraio and Simar Citation2005; De Witte and Kortelainen Citation2013), that provides more robust and reliable estimates of efficiency and of the role of contextual factors presiding to its determination.
By jointly considering the top 5 European leagues throughout a long period, we assess the role that some contextual factors may have on the efficiency of their teams. More in particular, by including the COVID-19 pandemic among these factors, we appraise the modification of the home advantage that has followed its outbreak, focusing separately on offensive and defensive efficiency (Research Question #1).
Relying on our cross-country sample, we assess whether the changes brought about by the COVID-19 pandemic have uniformly affected all the leagues under scrutiny (Research Question #2).
Academic research on football efficiency has been quite extensive. Previous articles have analysed football clubs’ efficiency, in financial and/or sporting terms, in major and minor national championships and knockout competitions: the English Premier League (Dawson, Dobson, and Gerrard Citation2000; Haas Citation2003; Barros and Leach Citation2006); the Spanish Liga (Espitia-Escuer and García-Cebrián Citation2004; González-Gómez and Picazo-Tadeo Citation2010; Barros and Garcia-Del-Barrio Citation2011); the Italian Serie A (Boscá et al. Citation2009; Rossi et al. Citation2019); the German Bundesliga (Tiedemann, Francksen, and Latacz-Lohmann Citation2011); the Portuguese Primeira Liga (Ribeiro and Lima Citation2012); the Greek Super League 1 (Barros and Douvis Citation2009); the Brasilian Serie A (Barros, Assaf, and Sá-Earp Citation2010); the Mexican Liga MX (Dávila and Cebrián Citation2012); the UEFA Champions League (Espitia-Escuer and García-Cebrián Citation2010; Zambon Ferraresi, Lera López, and García Cebrián Citation2017).
In their comprehensive literature review, Kulikova and Goshunova (Citation2013) highlight that a non-parametric frontier methodology, the Data Envelopment Analysis (DEA), is the most common methodological approach to analyse technical efficiency in football. Furthermore, several studies investigate football clubs’ on-field efficiency following a two-stage analysis format where efficiency measurement is carried out through DEA and the evaluation of efficiency determination is conducted through regression analysis on the scores obtained at the previous stage (examples of this procedure also include such important studies as García-Sánchez Citation2007; Boscá et al. Citation2009; Sala-Garrido et al. Citation2009; Villa and Lozano Citation2016). However, this technique suffers from well-known problems of validity and inference. Firstly, the DEA estimator has, under many circumstances, less than root-n convergence to the true production frontier (Simar and Wilson Citation2015). Secondly, being a full frontier estimator and enveloping all the points in the production set, DEA is sensitive to outliers (Kneip, Park, and Simar Citation1998). Finally, DEA does not straightforwardly allow for contextual variables – variables potentially relevant for the production process but beyond the control of the productive units – to influence the support of the production process. Having considered all these issues, we adopted an alternative and more recently developed procedure, the conditional order-m (Daraio and Simar Citation2005), which builds upon the order-m approach proposed in Cazals, Florens, and Simar (Citation2002). Being a partial frontier estimator, the order-m technique is more robust than other non-parametric estimators. Moreover, it routinely has root-n convergence to the true production frontier (Simar and Wilson Citation2015). What is more, for our purposes conditional order-m, and in particular its extension developed in De Witte and Kortelainen (Citation2013), consistently estimates the impact that a discrete event, such as the introduction of ghost matches, may have on the determination of efficiency. To the best of our knowledge, we apply for the first time conditional order-m to the analysis of on-field efficiency of football teams.
Neale (Citation1964) and Szymanski (Citation2003) argue that, since football clubs are grouped into leagues, on-field efficiency should be calculated considering their respective leagues as DMUs. On the other hand, according to Carmichael, Thomas, and Ward (Citation2000), on-field efficiency should be evaluated at the level of each single match. Since Carmichael, Thomas, and Ward (Citation2001), however, the most utilized unit of analysis for performance is the club evaluated at the level of each single season. In our context, we conform to the latter approach for the case of clubs competing in the top 5 European leagues throughout a long period. This choice is consistent with the seminal analysis proposed in Sloane (Citation1971). Furthermore, we are not aware of any match-level dataset available across the Top 5 leagues for the period under scrutiny, possessing the same detailed and consistent information of our dataset. We stress that previous research has never considered the measurement of efficiency in the context of more than two leagues (Boscá et al. Citation2009).
Yet, the contributions that we have highlighted above with respect to empirical method and sample must be considered in the light of the focus of this article. Our analysis investigates the reduction of the home advantage factor during the 2020–21 football season. The outbreak of the COVID-19 pandemic in 2020 allows us to investigate how the home advantage, one of the most studied and best documented phenomena in sports (Courneya and Carron Citation1992; Pollard and Pollard Citation2005), was affected by the absence of spectators. We use this natural experiment scenario to assess how professional football clubs in the top 5 European leagues have reacted to this peculiar circumstance, particularly insofar as their offensive and defensive efficiency was concerned.
The article is organized as follows. In Section II, we present a review of the literature on home advantage, focusing especially on the most recent contributions following the pandemic outbreak. In Section III we describe the methodology adopted for the empirical analysis, whereas Section IV shows and discusses our main results. Section V concludes.
II. Literature review
The phenomenon of home advantage is defined as ‘the consistent finding that the home teams in sport competition win over 50% of the games played under a balanced home and away schedule’ (Courneya and Carron Citation1992, 13). Stadium spectators are mostly supporters of local home teams, whose winning probability increases due to their fans’ support from the stands (Goumas Citation2014; Nevill, Newell, and Gale Citation1996; Pollard Citation2006; Pollard and Gomez Citation2009; Ponzo and Scoppa Citation2018). Despite being a worldwide phenomenon, with variation both over time and across regions, most empirical evidence (Pollard Citation1986; Jamieson Citation2010; Legaz-Arrese, Moliner-Urdiales, and Munguia-Izquierdo Citation2013) shows that home advantage is more prevalent in team sports, such as football in particular, than individual sports.
To explain this phenomenon, researchers have focused their attention on several mechanisms based on the relative importance and interplay of various factors. Three of the mainly discussed factors are: a) home crowd support affecting visiting teams’ performance and referee’s decisions (Schwartz and Barksy Citation1977; Garicano, Palacios-Huerta, and Prendergast Citation2005; Pollard and Pollard Citation2005; Buriamo, Paramio, and Campos Citation2010); b) home teams’ familiarity with local playing conditions (Pollard Citation1986; Barnett and Hilditch Citation1993; Clarke and Norman Citation1995; Moore and Brylinsky Citation1995; Neave and Wolfson Citation2003); and c) visiting teams’ travel fatigue (Courneya and Carron Citation1992; Ponzo and Scoppa Citation2018; Van Damme and Baert Citation2019). Among the other factors discussed (Courneya and Carron Citation1992; Nevill and Holder Citation1999; Neave and Wolfson Citation2003; Pollard Citation2008) there are territoriality, intended as a related aspect of familiarity but also as the humans’ natural inclination to respond to a real or perceived invasion of their territory (Morris Citation1981), and two other elements that are clearly linked to the home crowd presence: psychological effects, deriving from the mental attitude of players and coaches who are well aware of the existence of home advantage, that then becomes a self-perpetuating phenomenon (Pollard Citation1986; Pollard and Pollard Citation2005; Pollard Citation2006); and tactical behaviour, with teams approaching away games with a more cautious and defensive approach (Pollard Citation1986, Citation2006). All these factors directly influence the critical behavioural, psychological and on-field conditions of players, coaches and game officials. Disentangling the influence of these factors requires the experimental manipulation of real-world sport events, but this condition is almost impossible to meet. Alternatively, evidence can primarily be obtained on rare cases with limited sample sizes either indirectly, through the analysis of matches, teams or dimensions with varying attendance and travel burden by drawing conclusions from the characteristics of countries with a varying degree of home advantage, or more directly by considering special circumstances, such as same-stadium derbies, teams moving to a new sport facility or city, or spectators’ ban due to hooligan violence.
The outbreak of COVID-19 pandemic in the early months of 2020 has offered a unique opportunity to carry out a virtual experiment to assess whether matches played in complete absence of spectators – known as ghost matches – significantly alter the home advantage effect. Whilst two out of the three mainly discussed factors explaining home advantage – home teams’ familiarity with local conditions and visiting teams’ travel fatigue – have not been affected by the pandemic outbreak, the health and safety measures imposing behind-closed-door games have removed the third factor – the crowd effects on teams and referees. Indeed, the most recent systematic literature review, conducted by Leitner et al. (Citation2022) and based on 22 peer-reviewed publications, reveals that a great majority of these publications shows that the COVID pandemic, through the occurrence of ghost games, reduced home advantage mainly due to a weaker referee bias and a lack of emotional support from the stands.
Despite this evidence, there are discrepancies in the intensity of this reduction, ranging from strongly reduced to slightly reduced home advantage, with only two studies (Matos et al. Citation2021; Ramchandani and Millar Citation2021) concluding that there was no effect of the ghost games on any of the variables used by the authors to measure home advantage. This leads us to discuss more in detail these inconsistencies in the current literature, that can be attributed to different methodological approaches, type of leagues and sample size (Benz and Lopez Citation2021). From a methodological standpoint, different home advantage metrics such as goals, goal differential (Bryson et al. Citation2021) awarded points (Cueva Citation2020), yellow cards (Bryson et al. 2020), yellow card differential, and other in-game actions such as corner kicks and fouls (Scoppa Citation2021) are used to measure the team outcome variable. Accordingly, while correlation-based approaches include Chi-square and Mann–Whitney tests (Sors et al. Citation2020; Jiménez Sánchez and Lavín Citation2021; Leitner and Richlan Citation2021), models for multiple response variables are also developed through OLS and Poisson linear regression and t-tests (McCarrick et al. Citation2020; Bryson et al. Citation2021). Regarding the object of investigation, both the level of analysis – individual league or across leagues – and whether the leagues are jointly or separately analysed, play a role in affecting the results, as the impact of crowd support might vary according to the context characteristics of each league, to how competitive each league is, and to the quality of the spectators’ support.
Although several explanations and reasons concerning the more or less reduced home advantage in ghost games during the COVID-19 pandemic were provided in the literature, the most widespread are – as already mentioned − 1) a weaker referee bias (Endrich and Gesche Citation2020; Sors et al. 2020; Tilp and Thaller Citation2020; Bryson et al. Citation2021; Fischer and Haucap Citation2021; Hill and Van Yperen Citation2021; Jiménez Sánchez and Lavín Citation2021; Leitner and Richlan Citation2021; Rovetta and Abate Citation2021; Santana, Bettega, and Dellagrana Citation2021; Scoppa Citation2021; Wunderlich et al. Citation2021; Link and Anzer Citation2022), that can be also extended to the Video Assistant Referee (Tilp and Thaller Citation2020; Fischer and Haucap Citation2021; Hill and Van Yperen Citation2021), and 2) the lack of pressure and emotions deriving from the absence of the crowd and having a positive psychological impact on away teams and a negative psychological impact on home teams (McCarrick et al. Citation2020; Sors et al. 2020; Tilp and Thaller Citation2020; Almeida and Werlayne Citation2021; Correia-Oliveira and Andrade-Souza Citation2021; Ferraresi and Gucciardi Citation2021; Fischer and Haucap Citation2021; Leitner and Richlan Citation2021; Rovetta and Abate Citation2021; Scoppa Citation2021; Wunderlich et al. Citation2021; Link and Anzer Citation2022), which can be also linked to the dissolution of the home advantage as a self-perpetuating phenomenon (Hill and Van Yperen Citation2021). Other potential explanations are an enhanced coaches’ interference due to rule changes, more specifically the increase from 3 to 5 in the number of substitutions allowed (Almeida and Werlayne Citation2021; Benz and Lopez Citation2021), and different league’s restart dates and country specific COVID-19 rules (Benz and Lopez Citation2021).
However, the metrics used in the above-mentioned studies concerned the previously listed key variables, but never included the measurement of teams’ technical efficiency. This a potentially relevant gap in the literature, as an assessment of whether the COVID-19 pandemic affected the effective use of the clubs’ sporting resources can shed novel light on the strength and nature of the nexus between the pandemic and the modification of the home advantage. In this paper we fill this gap by considering an extensive production set, based on data from the top 5 European leagues (England, France, Germany, Italy and Spain) for the last twelve seasons. We provide measures of football clubs’ efficiencies before and after the onset of ghost matches and, modelling this event as a contextual variable, we give a quantitative assessment of its impact on the technical efficiency of football clubs.
III. Conditional order-m and regression
A longstanding issue in the field of nonparametric efficiency analysis relates to the treatment of contextual variables, that is, variables that are potentially relevant for the production process but also beyond the control of the productive units under observation. A first traditional solution to this problem is based on the partition of the sample according to different categories of contextual variables and is only applicable when the contextual variable is binary or categorical and is likely to involve a considerable reduction of the sample over which efficiency scores are computed, and the direction of the influence of the contextual variable on efficiency is not known a priori (Charnes, Cooper, and Rhodes Citation1981). Another solution involves the comparison of the observations under scrutiny with other observations that have the same or a more detrimental value of the contextual variable (Banker and Morey Citation1986). In this case, the influence of the contextual variable on efficiency must be known a priori, and there may be computational problems of the contextual variable being not continuous. Hence, the above solutions imply that only either continuous or discrete contextual variables can be considered and make it very difficult to deal with several contextual variables simultaneously. These strictures have prompted many researchers to resort to a two-stage approach, involving first the calculation of the efficiency scores without any allowance for the contextual factors, and then the appraisal of the role of these factors through regression analysis. There are various issues with this approach, the main one arguably being the requirement for the so-called separability condition to be fulfilled, as pointed out by Simar and Wilson (Citation2007). This condition effectively requires that contextual variables do not affect the shape of the attainable input–output set and can hardly be thought to be fulfilled a priori in many situations, including the present one. Why production sets should be in principle uniform across countries, or uniformly touched by post-pandemics institutional changes? Hence, in this article we do not rely on the assumption of separability among inputs, outputs, and contextual variables. Accordingly, for our analysis we adopt the conditional order-m approach, initiated by Cazals, Florens, and Simar (Citation2002), Daraio and Simar (Citation2005) and further developed by De Witte and Kortelainen (Citation2013).Footnote1
The conditional order-m approach is based on the fundamental idea of using contextual variables to identify the most similar observations, and estimating the efficiency around windows of these similar observations. Comparing the efficiency scores obtained unconditionally and conditionally on this similarity yields information about the impact of contextual variables that does not require the separability assumption, does not require any assumption on the direction of the influence of contextual variables on efficiency and allows for many contextual variables – both continuous and discrete – to be brought into play at the same time.
More formally, for a given input vector , used to produce output vector
, (unconditional) order-m output-oriented technical efficiency will be estimated by:
where is the empirical counterpart of the survival function
defined over a subset of m observations randomly drawn with replacement from a set of n observations. The partial frontier obtained in this way is less sensitive to outliers than the global frontier comprising all n observations. Observations with an efficiency score above unity are inefficient (their outputs must be expanded in a proportion of
to reach the order-m frontier). Observations with an efficiency score equal to unity are efficient (they are located on the order-m frontier). Finally, observations with an efficiency score below unity are classified as ‘super-efficient’. In empirical applications, the size of the m subset is chosen in a way that stabilizes the proportion of super-efficient observations in the sample. As we have already noted, the order-m approach is more robust to outliers than most non-parametric estimators, being a partial frontier estimator. Moreover, this estimator routinely has root-n convergence to the true production frontier (Simar and Wilson Citation2015).
Next, for a given input vector , used to produce output vector
, in an environment characterized by contextual variables
, estimation of conditional order-m output-oriented efficiency will be given by:
Now, , the empirical counterpart of the survival function
is defined over a subset of m observations drawn from a set of n observations, with the probability of drawing a given subset depending on a value of z and the kernel function estimated around this value. Note the difference with the unconditional case, where the probability of being drawn is equal for all observations. As a result, in the conditional model one compares like with like and does not need to impose any assumption of separability on the data generating process. In the present application, the definition of the kernel function allows for continuous, ordered discrete (categorical), and unordered discrete (binary) variables, along the lines of De Witte and Kortelainen (Citation2013). The kernel bandwidths for these variables are estimated through least-squares cross validation (Li and Racine Citation2008).
Once estimates of both unconditional and conditional order-m efficiency are available, one can compute for each observation the ratio:
Regressing (3) over the Z variables yields estimates of the influence of these variables on efficiency that are not liable to the criticisms levelled to the traditional two-stage approach (see on this De Witte and Kortelainen Citation2013, 2406). In order to do so, we rely on nonparametric regression analysis. This flexible specification does not impose any functional form on the relationship between efficiency scores and the contextual variables, allowing at the same time the calculation of standard errors for the regression coefficients (Li and Racine Citation2007).
IV. Empirical analysis and results
IV.1. Production set and contextual variables
Our analysis covers twelve seasons (2009–10 to 2020–21) of the top 5 European domestic leagues according to the Union of European Football Associations (UEFA) ranking: English Premier League, Spanish La Liga, Italian Serie A, German Bundesliga and French Ligue 1. We rely on season-level team data drawn from the website www.whoscored.com, and the 2019–20 season was excluded from the analysis as it is not possible to differentiate between pre-COVID and post-COVID production sets. We also argue that, during the 2019–20 season, teams and officials might have needed time to adjust to the new environment, possibly providing a somehow unclear picture of the change in their behaviour. Finally, unlike the other four leagues under investigation, French Ligue 1 did not resume and complete the aforementioned season after the suspension due to the pandemic outbreak. Therefore, our dataset comprises a total of 1078 observations: 980 pre-COVID and 98 post-COVID. The post-COVID sample, although much smaller than the pre-COVID one, is large enough to provide reliable evidence, especially if no significant behavioural differences emerge across the five leagues under scrutiny. We come back to this point when commenting our evidence in .
It is worth clarifying that not all the games in the 2020–21 season were played behind closed doors. The evolution of the number of COVID infections led national and local governments to allow the presence of a very limited number of spectators in certain games. However, across the five leagues analysed, only 10% of the overall games (181 out of 1826) were not played behind closed doors, and the average capacity utilization for those games was only 10.96% (transfermarkt.com, 2022). In Italy (where only 1,000 spectators were allowed), France and Germany, most of these games were played in the early stages of the season, as stadium attendance was again not allowed from the end of October to the end of the season (only in Germany, three games in the final fixture were played with a very limited attendance: 100, 250 and 2,000 spectators respectively), whereas in England and Spain spectators were allowed towards the end of the season. It is reasonable to argue that our results on season-level efficiency are not significantly affected by such a limited number of games and by such a low capacity utilization, implying the almost entire lack of home advantage also in those games. shows a breakdown of the games played with partial attendance for each of the five leagues.
Table 1. Games played with partial attendance in season 2020-21.
Our production sets are differentiated by offence and defence, home and away games, and are broadly based on Boscá et al. (Citation2009). All the variables included in them are listed in , whereas presents their main descriptive statistics. These variables include outputs (such as points, goals scored, inverse of goal conceded) and inputs (aspects of the game that are instrumental in producing the outputs). The outputs and inputs used for the calculation of offensive and defensive efficiency are detailed respectively in .Footnote2 Our contextual variables include, of course, a post-COVID binary variable, and other factors that we describe below. Since our main aim is to assess the impact of the absence of crowd due to the health and safety measures implemented after COVID outbreak on teams’ offensive and defensive efficiency, we present these statistics differentiating between the pre-COVID and post-COVID situations. It clearly emerges that, on the one hand, teams’ home performance has worsened in relation to all the three output variables considered: points gained (average decreased from 1.64 to 1.45), goals scored (average decreased from 1.55 to 1.48) and goals conceded (average increased from 1.16 to 1.32). On the other hand, teams’ away performance has significantly improved, with more points gained (from 1.11 to 1.29) and goals scored (from 1.16 to 1.32) and fewer goals conceded (from 1.55 to 1.48). These variations do not appear to be the outcome of corresponding changes in inputs. Possession does not change significantly, and we notice a generalized decrease in home and away shots, through balls and crosses, both made and conceded (this reduction is particularly significant for through balls and less strong for crosses). Tackles also decrease, whereas there is an increase in dribbles. These two developments also apply, although with varying strength, both to home and away games. Overall, this is confirmation that the hypothesis of variations in offensive and defensive technical efficiency due to the COVID-19 pandemic seems to be worth inquiring. Ghost games did not consistently impact on the input levels but may have done so on the teams’ ability to use these inputs effectively.
Table 2. Offensive inputs and outputs and contextual variables.
Table 3. Main descriptive statistics, No. of observations = 1078.
Table 4. Efficiency scores: offence.
Table 5. Efficiency scores: defence.
Paramount among our contextual variables is a post-COVID binary variable that requires no further comment here. Other contextual variables potentially impacting on teams’ efficiency are a) the number of managers employed during a season, that is used as a proxy for an unlucky season (teams that incur an unlucky season change manager once or more than once: we do not ascribe any causal influence to the managers’ change; yet inclusion of this variable improves the model by controlling for the influence of possibly random events leading to managers’ change), and 2) the number of penalties attempted for the offensive efficiency, the number of penalties converted by the opponents for the defensive efficiency.Footnote3 The penalties variables include zero values. Therefore, there are serious computational problems in including them among the inputs, while it is, in our opinion, appropriate and novel to use them as determinants of efficiency.
Like possession, the number of managers employed does not appreciably change before and after the COVID-19 outbreak. On the other hand, the number of penalties converted by the opponents at home games, and the number of penalties attempted at away games, significantly increase. One can relate these findings to similar results in the literature for other variables related to the referees’ behaviour (Endrich and Gesche Citation2020; Sors et al., 2020; Tilp and Thaller Citation2020; Bryson et al. Citation2021; Fischer and Haucap Citation2021; Hill and Van Yperen Citation2021; Jiménez Sánchez and Lavín Citation2021; Leitner and Richlan Citation2021; Rovetta and Abate Citation2021; Santana, Bettega, and Dellagrana Citation2021; Scoppa Citation2021; Wunderlich et al. Citation2021; Link and Anzer Citation2022). We will illustrate below their connection to the changes that we have noticed in the offensive and defensive outputs.
The contextual variables also include binary variables for all leagues (the English Premier League being the default category). The inclusion of the league dummies is by itself a novelty for the literature and will be commented again below. Moreover, to assess the robustness of our findings related to the post-COVID dummy, we also use a placebo binary variable for 2018–19 and a set of binary variables accounting for the potential confounding role of the introduction of the Video Assistant Referee (VAR). The placebo variable is a safeguard against our results being driven by unknown factors evolving over time independently of the COVID-19 outbreak (when including this variable, estimation was carried out from 2010–11 to 2018–19). The introduction of the VAR was modelled through a series of binary variables appropriately differentiated across countries, as VAR was introduced in 2017–18 in Italy and Germany, in 2018–19 in Spain and France and only in 2019–20 in England.Footnote4
4.2. Findings
The main descriptive statistics of the efficiency scores for both unconditional and conditional order-m are presented in , respectively for offensive and defensive efficiency.Footnote5 These results are obtained for the baseline production set, where balls kicked into the opposing team’s area are proxied by through balls. In the Appendix we show the results from a robustness check of our specification done by replacing through balls with crosses. Results are qualitatively unchanged, although efficiency is slightly lower (therefore we chose the set with through balls as our baseline case).
The following remarks are in order about the scores obtained in empirical analysis. As explained in Section 3, we provide output-oriented scores that vary in principle between one and infinity. A score equal to one denotes an efficient observation whose output cannot be increased, being already at its potential level, while a score greater than one denotes inefficient observations. The greater the score, the more output must be expanded to reach its potential level and consequently the more inefficient is the observation concerned. We also have a few super-efficient observations with a score lower than one.
A further remark is that mean and median efficiencies are high, especially if allowance is made for contextual variables. Given the large size of our sample (1078 observations), this result cannot be due to the ‘curse of dimensionality’ associated to a high ratio between the number of variables (inputs and outputs) and the number of observations (see, e.g. Charles, Aparicio, and Zhu Citation2019) and vouchsafes for a good specification of our production set.
As expected, our technical efficiency measures increase as contextual variables are included in the analysis. This is particularly true when penalties are included along with league dummies, number of managers and the post-COVID dummy. When carrying out these calculations, league dummies and the post-COVID dummy were, of course, treated as binary variables, while the number of managers was treated as a categorical variable.
Regressing the appropriate version of (3), with and without penalties, over the contextual variables yields estimates of the influence of these variables on offensive and defensive efficiency. The results of the regressions are shown in .Footnote6
Table 6. Regressions from conditional order-m: away offensive efficiency.
Table 7. Regressions from conditional order-m: home defensive efficiency.
Our research question #1 is whether ghost games had an impact on teams’ offensive and defensive technical efficiency in home and away games. Our evidence shows that in the post-COVID season efficiency increased for away games – offensively, but also defensively. More specifically, the post-COVID dummy is always strongly significant and positive in the regressions focusing on the away offensive efficiency () and is significant and positive in the regressions focusing on the away defensive efficiency, especially if penalties are considered (). On the other hand, the home offensive efficiency shows a very slight increase, as the post-COVID dummy is weakly significant and positive only with penalties (), whereas technical efficiency remains basically the same as before for home defence, as the post-COVID dummy shows some significance only if penalties are not considered – hence, the only relevant changes that have occurred in the post-COVID season are those related to the awarding of penalties (). The placebo test is always successful, as the related dummy is never significant (Columns 3 and 4 in all the tables). Also, inclusion of the VAR dummy never impacts on our findings about the post-COVID season (Columns 5 and 6), which confirms the robustness of our results.
Table 8. Regressions from conditional order-m: away defensive efficiency.
Table 9. Regressions from conditional order-m: home offensive efficiency.
Among other factors affecting efficiency, the Ligue 1 coefficient is always significant and negative, whereas the Bundesliga coefficient is significant and negative only when penalties are taken into account. A possible explanation of the consistently lower efficiency of French teams relies on their relatively lower quality, as evidenced by their average roster value over the period under investigation (94.3 m euros) in comparison with the other four leagues (254.2 m Premier League, 176.4 m La Liga, 153.6 m Serie A and 146 m Bundesliga). Deloitte’s Annual Review of Football Finance (Citation2014, Citation2015, Citation2016, Citation2017, Citation2018) also shows how the average team payroll in Ligue 1 (1.11b euros) is the lowest among the top 5 European leagues (2.95b Premier League, 1.63b La Liga, 1.44b Bundesliga and 1.41b Serie A). The other contextual variables have always the desired sign: penalties_attempted has a positive impact on offensive efficiency, penalties_conceded a negative impact on defensive efficiency, whereas n_managers – our proxy for an unlucky season – always shows the expected negative sign for both types of efficiency.
Our Research Question # 2 is whether the impact of ghost games is different across the five leagues analysed. For this reason, we tested for a differential league effect of the post-COVID dummy, where the null hypothesis is that ghost matches affect teams’ technical efficiency uniformly. To this purpose, we created interactive terms between the league and the post-COVID dummies and tested for their significance. The related findings, reported at the bottom lines of , indicate that the post-COVID dummy affects all leagues equally. Home defensive efficiency is the only case where there is some weak evidence of a differential post-COVID country effect (the full estimates, available upon request, make clear that there is an extra positive impact for Spain), but even this marginal exception vanishes when considering an alternative production set (with crosses replacing through balls; this evidence is available upon request).
These findings are consistent with the main results of the recent literature on the impact of ghost games on teams’ performance (Endrich and Gesche Citation2020; Reade and Singleton Citation2020; Reade, Schreyer, and Singleton Citation2020; Tilp and Thaller Citation2020; Fischer and Haucap Citation2021) and show a reduction in the home advantage also when the analysis is focused on teams’ technical efficiency. As evidenced in our literature review, home advantage can be explained by the interaction of several factors, with the most discussed in literature being home crowd support affecting visiting teams’ performance and referee’s decisions, home teams’ familiarity with local playing conditions and visiting teams’ travel fatigue. Since the latter two factors are not affected by the ghost games, it is reasonable to argue that the erosion of the home advantage is determined by the reduced pressure on away teams deriving from the lack of home crowd support and leading to a more effective use of their sporting resources. This is also likely to weaken the psychological effect of the home advantage as a self-perpetuating phenomenon (Pollard Citation1986; Pollard and Pollard Citation2005; Pollard Citation2006). On the other hand, as our descriptive analysis show no significant post-COVID changes in ball possession and a generalized decrease in shots, through balls and crosses, visiting teams do not appear to have adopted a less cautious and more offensive approach to the game.
V. Conclusions
This study aims to contribute to the recent literature on the effects of COVID on football teams’ performance, focusing on the impact of playing behind closed doors – due to the health and safety measures following the COVID-19 pandemic outbreak – on offensive and defensive technical efficiency. Using a long season-level dataset for the top 5 European leagues, a novelty for efficiency studies on football, the analysis compares the ten seasons (2009–10 to 2018–19) played before the pandemic outbreak with the only season (2020–21) almost entirely played behind closed doors. The methodology applied to calculate the efficiency scores is the conditional order-m, whose application represents a further novel contribution to the literature on football teams’ efficiency.
Our results show that in the post-COVID season both offensive and defensive efficiency significantly increased for away games, whereas for home games offensive efficiency shows a very slight increase, and defensive efficiency remains basically unchanged. These results are valid for all the five leagues under investigation, as there is no consistent evidence of differential post-COVID country effect. These findings are aligned with most results from the recent literature on the impact of ghost games on teams’ performance and show an erosion of the home advantage also when the analysis is focused on teams’ technical efficiency. This more effective use of the teams’ sporting resources is likely due to the reduced pressure on visiting teams deriving from the lack of home crowd support.
Disclosure statement
No potential conflict of interest was reported by the author(s).
Notes
1 In principle, we could test for the existence of the separability condition through the test suggested in Daraio, Simar, and Wilson (Citation2018). This test, however, runs into very serious computational problems, especially when several contextual variables must be considered at the same time (Wilson Citation2020, 60)). Besides, it is not likely that the separability assumption could hold in our case, as was already noticed above.
2 In these baseline production sets, balls kicked into the opposing team’s area are proxied by through balls. We proxy the same variable by crosses in a production set used for a robustness check.
3 The difference in the penalty variables used for offensive and defensive efficiency is due to the lack of data for the number of penalties attempted by the opponents in our dataset. However, our data show high correlation between penalties attempted and penalties scored, therefore our results are not heavily affected.
4 We stress that this exercise cannot yield a proper counterfactual assessment of the introduction of the VAR, which we are currently carrying out in a companion paper.
5 These scores were computed through a script written in R. We gratefully acknowledge Kristof De Witte for his generosity in sharing his scripts with us.
6 The regressions presented in have also been carried out on the alternative production set with crosses in place of through balls. The results, available upon request, are virtually unchanged. The only difference worth mentioning is that no differential post-COVID effect exists any longer for the Liga’s home defensive efficiency.
References
- Almeida, C. H., and S. L. Werlayne. 2021. “Professional Football in Times of COVID-19: Did the Home Advantage Effect Disappear in European Domestic Leagues?” Biology of Sport 38 (4): 693–701. doi:10.5114/biolsport.2021.104920.
- Banker, R. D., and R. C. Morey. 1986. “Efficiency Analysis for Exogenously Fixed Inputs and Outputs.” Operations Research 34 (4): 513–521. doi:10.1287/opre.34.4.513.
- Barnett, V., and S. Hilditch. 1993. “The Effect of an Artificial Pitch Surface on Home Team Performance in Football (Soccer).” Journal of the Royal Statistical Society: Series a (Statistics in Society) 156 (1): 39–50. doi:10.2307/2982859.
- Barros, C. P., A. Assaf, and F. Sá-Earp. 2010. “Brazilian Football League Technical Efficiency: A Simar and Wilson Approach.” Journal of Sports Economics 11 (6): 641–651. doi:10.1177/1527002509357530.
- Barros, C. P., and J. Douvis. 2009. “Comparative Analysis of Football Efficiency Among Two Small European Countries: Portugal and Greece.” International Journal of Sport Management and Marketing 6 (2): 183–199. doi:10.1504/IJSMM.2009.028801.
- Barros, C. P., and P. Garcia-Del-Barrio. 2011. “Productivity Drivers and Market Dynamics in the Spanish First Division Football League.” Journal of Productivity Analysis 35 (1): 5–13. doi:10.1007/s11123-010-0196-9.
- Barros, C. P., and S. Leach. 2006. “Performance Evaluation of the English Premier Football League with Data Envelopment Analysis.” Applied Economics 38 (12): 1449–1458. doi:10.1080/00036840500396574.
- Benz, L. S., and M. J. Lopez. 2021. “Estimating the Change in Soccer’s Home Advantage During the Covid-19 Pandemic Using Bivariate Poisson Regression.” AStA Advances in Statistical Analysis 1–28 https://doi.org/10.1007/s10182-021-00413-9.
- Boscá, J. E., V. Liern, A. Martínez, and R. Sala. 2009. “Increasing Offensive or Defensive Efficiency? an Analysis of Italian and Spanish Football.” Omega 37 (1): 63–78. doi:10.1016/j.omega.2006.08.002.
- Bryson, A., P. Dolton, J. J. Reade, D. Schreyer, and C. Singleton. 2021. “Causal Effects of an Absent Crowd on Performances and Refereeing Decisions During COVID-19.” Economics Letters 198: 109664. doi:10.1016/j.econlet.2020.109664.
- Buriamo, B., J. L. Paramio, and C. Campos. 2010. “The Impact of Televised Football on Stadium Attendance in English and Spanish League Football.” Soccer & Society 11(4): 461-474 ().
- Carmichael, F., and D. Thomas. 2014. “Team Performance: Production and Efficiency in Football.” In Handbook on the Economics of Professional Football, edited by J. Goddard and P. Sloane, 143–165. Cheltenham: Edward Elgar.
- Carmichael, F., D. Thomas, and R. Ward. 2000. “Team Performance: The Case of English Premiership Football.” Managerial and Decision Economics 21: 31–45. doi:10.1002/1099-1468(200001/02)21:1<31:AID-MDE963>3.0.CO;2-Q.
- Carmichael, F., D. Thomas, and R. Ward. 2001. “Production and Efficiency in Association Football.” Journal of Sports Economics 2 (3): 228–243. doi:10.1177/152700250100200303.
- Castellano, J., D. Casamichana, and C. Lago. 2012. “The Use of Match Statistics That Discriminate Between Successful and Unsuccessful Soccer Teams.” Journal of Human Kinetics 31: 137–147. doi:10.2478/v10078-012-0015-7.
- Cazals, C., J. P. Florens, and L. Simar. 2002. “Nonparametric Frontier Estimation: A Robust Approach.” Journal of Econometrics 106 (1): 1–25. doi:10.1016/S0304-4076(01)00080-X.
- Charles, V., J. Aparicio, and J. Zhu. 2019. “The Curse of Dimensionality of Decision-Making Units: A Simple Approach to Increase the Discriminatory Power of Data Envelopment Analysis.” European Journal of Operational Research 279 (3): 929–940. doi:10.1016/j.ejor.2019.06.025.
- Charnes, A., W. W. Cooper, and E. Rhodes. 1981. “Evaluating Program and Managerial Efficiency: An Application of Data Envelopment Analysis to Program Follow Through.” Management Science 27: 668–697. doi:10.1287/mnsc.27.6.668.
- Clarke, S. R., and J. M. Norman. 1995. “Home Ground Advantage of Individual Clubs in English Soccer.” Journal of the Royal Statistical Society: Series D (The Statistician) 44 (4): 509–521.
- Coelli, T. J., D. D. P. Rao, C. J. O’-Donnell, and G. E. Battese. 2005. An Introduction to Efficiency and Productivity Analysis. 2nd ed. New York, NY: Springer.
- Correia-Oliveira, C. R., and V. A. Andrade-Souza. 2021. “Home Advantage in Soccer After the Break Due to COVID-19 Pandemic: Does Crowd Support Matter?” International Journal of Sport and Exercise Psychology 1–12. doi:10.1080/1612197X.2021.1934716.
- Courneya, K. S., and A. V. Carron. 1992. “The Home Advantage in Sport Competitions: A Literature Review.” Journal of Sport & Exercise Psychology 14: 28–39. doi:10.1123/jsep.14.1.13.
- Cueva, C. 2020. Animal Spirits in the Beautiful Game. Testing Social Pressure in Professional Football During the COVID-19 Lockdown. OSF Preprints.
- Daraio, C., and L. Simar. 2005. “Introducing Environmental Variables in Nonparametric Frontier Models: A Probabilistic Approach.” Journal of Productivity Analysis 24 (1): 93–121. doi:10.1007/s11123-005-3042-8.
- Daraio, C., L. Simar, and P. W. Wilson. 2018. “Central Limit Theorems for Conditional Efficiency Measures and Tests of the ‘Separability’ Condition in Non‐parametric, Two‐stage Models of Production.” The Econometrics Journal 21 (2): 170–191. doi:10.1111/ectj.12103.
- Dávila, C. G. T., and L. I. G. Cebrián. 2012. “Eficiencia Y Resultados Deportivos: Aplicación a la Liga Mexicana de Fútbol.” Revista Movimiento Humano 3: 61–76.
- Dawson, P., S. Dobson, and B. Gerrard. 2000. “Stochastic Frontiers and the Temporal Structure of Managerial Efficiency in English Soccer.” Journal of Sports Economics 1 (4): 341–362. doi:10.1177/152700250000100402.
- De Witte, K., and M. Kortelainen. 2013. “What Explains the Performance of Students in a Heterogeneous Environment? Conditional Efficiency Estimation with Continuous and Discrete Environmental Variables.” Applied Economics 45 (17): 2401–2412. doi:10.1080/00036846.2012.665602.
- Deloitte. 2014. Deloitte Annual Review of Football Finance. Deloitte.
- Deloitte. 2015. Deloitte Annual Review of Football Finance. Deloitte.
- Deloitte. 2016. Deloitte Annual Review of Football Finance. Deloitte.
- Deloitte. 2017. Deloitte Annual Review of Football Finance. Deloitte.
- Deloitte. 2018. Deloitte Annual Review of Football Finance. Deloitte.
- Endrich, L. M., and T. Gesche. 2020. “Home-Bias in Referee Decisions: Evidence from “Ghost Matches” During the COVID19-Pandemic.” Economics Letters 197: 109621. doi:10.1016/j.econlet.2020.109621.
- Espitia-Escuer, M., and L. I. García-Cebrián. 2004. “Measuring the Efficiency of Spanish First-Division Soccer Teams.” Journal of Sports Economics 5 (4): 329–346. doi:10.1177/1527002503258047.
- Espitia-Escuer, M., and L. I. García-Cebrián. 2010. “Measurement of the Efficiency of Football Teams in the Champions League.” Managerial and Decision Economics 31 (6): 373–386. doi:10.1002/mde.1491.
- Ferraresi, M., and G. Gucciardi. 2021. “Who Chokes on a Penalty Kick? Social Environment and Individual Performance During COVID-19 Times.” Economics Letters 203: 109868. doi:10.1016/j.econlet.2021.109868.
- Fischer, K., and J. Haucap. 2021. “Does the Crowd Support Drive the Home Advantage in Professional Soccer? Evidence from German Ghost Games During the COVID-19 Pandemic.” Journal of Sports Economics 22(8): 982-1008 . .
- García-Sánchez, I. M. 2007. “Efficiency and Effectiveness of Spanish Football Teams: A Three-Stage-DEA Approach.” Central European Journal of Operations Research 15 (1): 21–45. doi:10.1007/s10100-006-0017-4.
- Garicano, L., I. Palacios-Huerta, and C. Prendergast. 2005. “Favoritism Under Social Pressure.” The Review of Economics and Statistics 87 (2): 208–216. doi:10.1162/0034653053970267.
- González-Gómez, F., and A. J. Picazo-Tadeo. 2010. “Can We Be Satisfied with Our Football Team? Evidence from Spanish Professional Football.” Journal of Sports Economics 11 (4): 418–442. doi:10.1177/1527002509341020.
- Goumas, C. 2014. “How Does Crowd Support Contribute to Home Advantage in Soccer?” Journal of Sports Behavior 37 (3): 236.
- Haas, D. J. 2003. “Productive Efficiency of English Football Teams – a Data Envelopment Analysis Approach.” Managerial and Decision Economics 24 (5): 403–410. doi:10.1002/mde.1105.
- Hill, Y., and N. W. Van Yperen. 2021. “Losing the Home Field Advantage When Playing Behind Closed Doors During COVID-19: Change or Chance?” Frontiers in Psychology 12. doi:10.3389/fpsyg.2021.658452.
- Jamieson, J. P. 2010. “The Home Field Advantage in Athletics: A Meta-Analysis.” Journal of Applied Social Psychology 40 (7): 1819–1848. doi:10.1111/j.1559-1816.2010.00641.x.
- Jiménez Sánchez, Á., and J. M. Lavín. 2021. “Home Advantage in European Soccer Without Crowd.” Soccer Society 22 (1–2): 152–165. doi:10.1080/14660970.2020.1830067.
- Kneip, A., B. U. Park, and L. Simar. 1998. “A Note on the Convergence of Nonparametric DEA Estimators for Production Efficiency Scores.” Econometric Theory 14 (6): 783–793. doi:10.1017/S0266466698146042.
- Kulikova, L. I., and A. V. Goshunova. 2013. “Measuring Efficiency of Professional Football Club in Contemporary Research.” World Applied Sciences Journal 25 (2): 247–257.
- Legaz-Arrese, A., D. Moliner-Urdiales, and D. Munguia-Izquierdo. 2013. “Home Advantage and Sports Performance: Evidence, Causes and Psychological Implications.” Universitas Psychologica 12 (3): 933–943. doi:10.11144/Javeriana.upsy12-3.hasp.
- Leitner, M. C., F. Daumann, F. Follert, and F. Richlan. 2022. “The Cauldron Has Cooled Down: A Systematic Literature Review on Home Advantage in Football During the COVID-19 Pandemic from a Socio-Economic and Psychological Perspective.” Management Review Quarterly 1–29 https://doi.org/10.1007/s11301-021-00254-5.
- Leitner, M. C., and F. Richlan. 2021. “Analysis System for Emotional Behavior in Football (ASEB-F): Matches of FC Red Bull Salzburg Without Supporters During the COVID-19 Pandemic.” Humanities and Social Sciences Communities 8(1): 1-11 (1).
- Lepschy, H., H. Wäsche, and A. Woll. 2020. “Success Factors in Football: An Analysis of the German Bundesliga.” International Journal of Performance Analysis in Sport 20 (2): 150–164. doi:10.1080/24748668.2020.1726157.
- Li, Q., and J. Racine. 2007. Nonparametric Econometrics: Theory and Practice. Princeton: Princeton University Press.
- Li, Q., and J. S. Racine. 2008. “Nonparametric Estimation of Conditional CDF and Quantile Functions with Mixed Categorical and Continuous Data.” Journal of Business and Economic Statistics 26 (4): 423–434. doi:10.1198/073500107000000250.
- Link, D., and G. Anzer. 2022. “How the COVID-19 Pandemic Has Changed the Game of Soccer.” International Journal of Sports Medicine 43 (1): 83–93. doi:10.1055/a-1518-7778.
- Matos, R., D. Monteiro, R. Antunes, D. Mendes, J. Botas, J. Clemente, and N. Amaro. 2021. “Home-Advantage During COVID-19: An Analysis in Portuguese Football League.” International Journal of Environmental Research and Public Health 18 (7): 3761. doi:10.3390/ijerph18073761.
- McCarrick, D., M. Bilalic, N. Neave, and S. Wolfson. 2020. “Home Advantage During the COVID-19 Pandemic in European Football.” Psychology of Sport and Exercise 56: 102013. doi:10.1016/j.psychsport.2021.102013.
- Moore, J. C., and J. Brylinsky. 1995. “Facility Familiarity and the Home Advantage.” Journal of Sport Behavior 18 (4): 302–311.
- Morris, D. 1981. The Soccer Tribe. London: Cape.
- Neale, W. C. 1964. “The Particular Economics of Professional Sports: A Contribution to the Theory of the Firm in Sporting Competition and in Market Competition.” The Quarterly Journal of Sports Economics 78 (1): 1–14. doi:10.2307/1880543.
- Neave, N., and S. Wolfson. 2003. “Testosterone, Territoriality, and the ‘Home Advantage’.” Physiology & Behavior 78 (2): 269–275. doi:10.1016/S0031-9384(02)00969-1.
- Nevill, A. M., and R. L. Holder. 1999. “Home Advantage in Sport: An Overview of Studies on the Advantage of Playing at Home.” Sports Medicine 28 (4): 221–236. doi:10.2165/00007256-199928040-00001.
- Nevill, A. M., S. M. Newell, and S. Gale. 1996. “Factors Associated with Home Advantage in English and Scottish Soccer Matches.” Journal of Sports Sciences 14 (2): 181–186. doi:10.1080/02640419608727700.
- Pollard, R. 1986. “Home Advantage in Soccer: A Retrospective Analysis.” Journal of Sport Sciences 4: 237–248. doi:10.1080/02640418608732122.
- Pollard, R. 2006. “Home Advantage in Soccer: Variations in Its Magnitude and a Literature Review of the Inter-Related Factors Associated with Its Existence.” Journal of Sport Behavior 29 (2): 341–352.
- Pollard, R. 2008. “Home Advantage in Football: A Current Review of an Unsolved Puzzle.” The Open Sports Sciences Journal 1 (1): 12–14. doi:10.2174/1875399X00801010012.
- Pollard, R., and M. A. Gomez. 2009. “Home Advantage in Football in South-West Europe: Long-Term Trends, Regional Variation, and Team Difference.” European Journal of Sport Science 9 (6): 341–352. doi:10.1080/17461390903009133.
- Pollard, R., and G. Pollard. 2005. “Long-Term Trends in Home Advantage in Professional Team Sports in North America and England.” Journal of Sports Sciences 23: 337–350. doi:10.1080/02640410400021559.
- Pollard, R., and G. Pollard. 2005. “Venteja de Ser el Equipo Local En Fútbol: Una Reseña de Su Existencia Y Causas.” Rev Int Fútbol Ciencia 3 (1): 31–44.
- Ponzo, M., and V. Scoppa. 2018. “Does the Home Advantage Depend on Crowd Support? Evidence from Same-Stadium Derbies.” Journal of Sports Economics 19 (4): 562–582. doi:10.1177/1527002516665794.
- Ramchandani, G., and R. Millar. 2021. “Investigating the “Twelfth Man” Effect in Five European Domestic Football Leagues: A COVID-19 Induced Natural Experiment.” Journal of Global Sport Management 1–15. doi:10.1080/24704067.2021.1951614.
- Reade, J., D. Schreyer, and C. Singleton. 2020. ”Eliminating Supportive Crowds Reduces Referee Bias.” SSRN Electronic Journal. Available at SSRN 3743972. doi:10.2139/ssrn.3743972.
- Reade, J. J., and C. Singleton. 2020. “European Football After COVID-19.” A New World Post COVID-19 349.
- Ribeiro, A. S., and F. Lima. 2012. “Portuguese Football League Efficiency and Players’ Wages.” Applied Economics Letters 19 (6): 599–602. doi:10.1080/13504851.2011.591719.
- Rossi, G., D. Goossens, G. L. Di Tanna, and F. Addesa. 2019. “Football Team Performance Efficiency and Effectiveness in a Corruptive Context: The Calciopoli Case.” European Sport Management Quarterly 19 (5): 583–604. doi:10.1080/16184742.2018.1553056.
- Rovetta, A., and A. Abate. 2021. “The Impact of Cheering on Sports Performance: Comparison of Serie a Statistics Before and During COVID-19.” Cureus 13 (8). doi:10.7759/cureus.17382.
- Sala-Garrido, R., V. L. Carrión, A. M. Esteve, and J. E. Boscá. 2009. “Analysis and Evolution of Efficiency in the Spanish Soccer League.” Journal of Quantitative Analysis in Sports 5 (1). doi:10.2202/1559-0410.1143.
- Santana, H. A. P., O. B. Bettega, and R. A. Dellagrana. 2021. “An Analysis of Bundesliga Matches Before and After Social Distancing by COVID-19.” Science and Medicine in Football 5 (1): 152–165. doi:10.1080/24733938.2021.1903540.
- Schwartz, B., and S. F. Barksy. 1977. “The Home Advantage.” Social Forces 55 (3): 641–661. doi:10.2307/2577461.
- Scoppa, V. 2021. “Social Pressure in the Stadiums: Do Agents Change Behaviour Without Crowd Support?” Journal of Economic Psychology 82: 102344. doi:10.1016/j.joep.2020.102344.
- Simar, L., and P. W. Wilson. 2007. “Estimation and Inference in Two-Stage, Semi-Parametric Models of Production Processes.” Journal of Econometrics 136 (1): 31–64. doi:10.1016/j.jeconom.2005.07.009.
- Simar, L., and P. W. Wilson. 2015. “Statistical Approaches for Non-Parametric Frontier Models: A Guided Tour.” International Statistical Review 83 (1): 77–110. doi:10.1111/insr.12056.
- Sloane, P. J. 1971. “The Economics of Professional Football: The Football Club as a Utility Maximiser.” Scottish Journal of Political Economy 18 (2): 121–146. doi:10.1111/j.1467-9485.1971.tb00979.x.
- Sors, F., M. Grassi, T. Agostini, and M. Murgia. 2021. “The Sound of Silence in Association Football: Home Advantage and Referee Bias Decrease in Matches Played Without Spectators.” European Journal of Sport Science 21 (12): 1597–1605.
- Szymanski, S. 2003. “The Economic Design of Sporting Contests.” Journal of Economic Literature 41 (4): 1137–1187. doi:10.1257/jel.41.4.1137.
- Tiedemann, T., T. Francksen, and U. Latacz-Lohmann. 2011. “Assessing the Performance of German Bundesliga Football Players: A Non-Parametric Metafrontier Approach.” Central European Journal of Operations Research 19 (4): 571–587. doi:10.1007/s10100-010-0146-7.
- Tilp, M., and S. Thaller. 2020. “COVID-19 Has Turned Home-Advantage into Home-Disadvantage in the German Soccer Bundesliga.” Frontiers in Sports and Active Living 2: 165. doi:10.3389/fspor.2020.593499.
- Van Damme, N., and S. Baert. 2019. “Home Advantage in European International Soccer: Which Dimension of Distance Matters?” Economics 13. doi:10.5018/economics-ejournal.ja.2019-50.
- Villa, G., and S. Lozano. 2016. “Assessing the Scoring Efficiency of a Football Match.” European Journal of Operational Research 255 (2): 559–569. doi:10.1016/j.ejor.2016.05.024.
- Wilson, P. W. 2020. “Package ‘FEAR’.” August 31. https://pww.people.clemson.edu/Software/FEAR/fear.html
- Wunderlich, F., M. Weigelt, R. Rein, and D. Memmert. 2021. “How Does Spectator Presence Affect Football? Home Advantages in European Top-Class Football Matches Played Without Spectators During the COVID-19 Pandemic.” PLoS One 16 (3): e0248590. doi:10.1371/journal.pone.0248590.
- Zambon Ferraresi, F., F. Lera López, and L. I. García Cebrián. 2017. “Sports Results Measurement and Efficiency in UEFA Champions League.” No. ART-2017-103980.