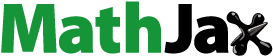
ABSTRACT
This study attempts to empirically investigate the validity of energy-led growth hypothesis for renewable energy sources for developing countries. To this end, this paper estimates the impacts of disaggregated renewable energy sources on economic growth within a multivariate framework including the disaggregated non-renewable energy sources, capital, labour, institutional quality and human capital by using panel data of 32 lower/upper middle income countries over the period 2009 to 2019 and applying spatial dynamic techniques. Our results show the significantly positive impacts of individual renewable sources on economic growth. This study provides the first piece of evidence of spatial spillover effects from renewable energy on economic growth for developing countries. Our analysis reveals the significantly negative impacts of hydroelectricity on economic growth. Our analysis also confirms the importance of labour, institutional quality and human capital in driving economic growth.
I. Introduction
Non-renewable energy has been traditionally considered as a vital driver of economic growth in developing countries (Polat Citation2021). However, the heavy dependence on fossil energy sources has induced the growing concerns over energy supply security, volatility of energy prices and environmental consequences associated with energy production and consumption (Apergis and Payne Citation2012). These concerns have forced developing countries to substitute fossil energy sources with renewable energy sources (Jha and Singh Citation2020). On the one hand, energy transition is considered as a mitigation strategy that developing countries do need to apply in order to respond to the worldwide environmental security challenge expressed through the 2015 Paris agreement on climate change. On the other hand, energy transition is a widely accepted pathway towards sustainable growth for developing countries. As a result, development of renewable energy as a means of mitigating climate change while maintaining economic growth is currently shaping an important policy agenda for developing countries.
Over the past decades, many developing countries have made common effort of increasing investment in renewable energy (Alam et al. Citation2017). According to the Renewable Global Status Report (Citation2016), the investment in renewables in developing countries increased to USD 156 billion in 2015 from USD 20 billion in 2005. The total investment in renewables in developing countries even began to exceed that in developed countries in 2015.Footnote1
However, the development of renewable energy implies high investment costs, partly due to the intermittent nature of some renewable energy sources (Marques and Fuinhas Citation2012). In addition, the observed increase in the share of renewables in current energy mixes does not yet make it possible to compensate for the associated loss in the capacity for producing electricity from fossil fuels (Marques and Fuinhas Citation2012). Both high investment costs of renewable energy and low fossil energy use may induce a serious drag on economic growth that eliminates the positive influence of renewable energy technological progress on economic growth. In this setting, the purpose of this study is to investigate whether and to what extent renewable energy sources contribute to economic growth process in the developing countries. Our research seeks to provide rich policy implications for managing environmental and economic conflicts linked to renewable energy sources and for the design of efficient renewable energy policies in the quest for a sustainable energy system.
The energy-led growth hypothesis (i.e. energy consumption contributes to economic growth) has long been a subject of intense debate in the energy-growth literature.Footnote2 However, the empirical outcomes of the existing studies are still until now inconsistent with each other (Emirmahmutoglu et al. Citation2021).Footnote3 In contrast, the energy-led growth hypothesis for renewable energy sources has only recently been investigated (Apergis and Payne Citation2012; Menegaki Citation2011). The effects of renewable energy sources on economic growth have not been fully examined (Inglesi-Lotz Citation2016). Hence, there is still a long way to go before achieving conclusive results as to the validity of the energy-led growth hypothesis for renewable energy sources. In addition, most of the earlier studies focus on developed countries due to the availability and reliability of data, while with limited emphasis on developing countries, even though the investment in renewable energy in developing economies mushroomed over the near past. Therefore, additional research explaining the impact of renewable energy on economic growth of developing countries is warranted. In this context, this study contributes to the scant literature on the topic in three different aspects.
Firstly, this paper focuses on exploring the impacts of disaggregate renewable energy sources on the economic growth of developing countries. This kind of studies is essential given the important policy implications, that is, the policy makers are suggested to formulate different strategies for each renewable energy source in order to achieve sustainable economic growth (Dogan Citation2016).Footnote4 Moreover, the use of disaggregate renewable energy data allows for capturing the extent to which different countries depend on different energy resources. However, the studies identifying the effects of disaggregate renewable energy sources on economic growth are very scarce (Ohler and Fetters Citation2014).Footnote5 Therefore, a further study on the impacts of disaggregate renewable energy in the developing countries is still necessary.
Second, this paper adds to the scant literature by considering simultaneously the disaggregated renewable and non-renewable energy sources. This consideration allows us to identify the dissimilar effects of energy sources in enhancing the economic growth prospects of developing countries and analyse the substitutability between renewable and non-renewable energy sources (Apergis and Payne Citation2012). The previous studies have largely omitted to include the disaggregated non-renewable energy alongside renewable energy, although this omission may lead to the wrong conclusions and inconsistent results about the renewable energy-growth nexus (Apergis and Payne Citation2012; Marques and Fuinhas Citation2012).Footnote6
Finally, this is the first piece of empirical cross-sectional analyses considering the role of spatial spillover effects for developing countries. The innovation on the model specification in the renewable energy-growth literature is necessary in order to avoid increasing the number of conflicting results and making the policymaking more uncertain (Apergis and Tang Citation2013; Karanfil Citation2009; Ozturk Citation2010). It has been recognized that the economic growth of one country depends on the growth and economic conditions of nearby countries (Basile Citation2008). The spatial spillovers as a major engine of technological progress result from international trade, foreign direct investment, technology transfers and human capital externalities (Ertur and Koch Citation2007; Radmehr, Henneberry, and Shayanmehr Citation2021). Another spatial effect arises from the fact that energy consumption pattern in one region could influence energy consumption in neighbouring regions and therefore, affects economic growth of its neighbours (Fotis, Karkalakos, and Asteriou Citation2017). As a consequence, estimation procedures omitting the spatial effects may cause model misspecification problem, bias the estimated results and hence produce misleading conclusions about policy inferences (Anselin Citation1988; LeSage and Pace Citation2009). Nevertheless, the idea of spatial spillover effects has been fully neglected by the existing literature on the topic focusing on developing countries.Footnote7
The paper is organized as follows. Section II describes the empirical analysis. Section III discusses the main results, and Section IV offers concluding remarks and policy recommendations.
II. Methods
Empirical model and data
Empirical model
To investigate the impacts of renewable energy sources on economic growth, this study applies the following production function:
Where, denotes aggregate output,
represents capital stock,
stands for labour,
and
are respectively renewable and traditional energy sources,
denotes a vector of other control variables that consist of institutional quality and human capital, which potentially influence economic growth. The subscript
denotes country and
denotes year. In this function, capital, labour and energy are treated as separate inputs. Capital, labour, institutional quality and human capital are included in the model in order to avoid omission bias problem (Adekoya et al. Citation2022; Ohler and Fetters Citation2014).Footnote8 The production function defined as EquationEquation. (1)
(1)
(1) is extended to analyse the impacts of disaggregated energy sources on economic growth by replacing
and
with each individual renewable and traditional energy sources.
Using log-linearization, EquationEquation. (1)(1)
(1) could be rewritten as:
Where is the intercept,
,
,
,
and
are the coefficients which measures the output elasticities with respect to capital stock, labour, disaggregated renewable and traditional energy sources, institutional quality and human capital respectively,
refers to individual fixed effect (regional dummies)Footnote9,
correspond to time fixed effect (time trend)Footnote10, and
is error term.
Data
The multivariate framework encompasses real GDP per capita (Y) in constant 2015 US $, real gross fixed capital formation per capita (K) in constant 2015 US $, labour force per capita (L).Footnote11 These data are obtained from the World Bank’s World Development Indicators. Electricity production from biomass & waste (BIOM), or wind (WIND) measured in billions of kilowatt-hours as a proxy for disaggregated renewable energy source (RE). Disaggregated traditional energy source is defined as coal supply (COAL) or oil supply (OIL)Footnote12 measured in million tonnes of oil equivalentFootnote13 or hydroelectric generation (HYDR) measured in billions of kilowatt-hours.Footnote14 The data on BIOM, WIND and HYDR are drawn from the U.S. Energy Information Administration. The data on COAL and OIL are collected from the International Energy Agency.Footnote15 Following Zhang and Kim (Citation2022), the institutional quality (INST) is measured by the arithmetic mean of all six dimensions of the World Bank’s Worldwide Governance Indicators, which include voice and accountability, political stability, government effectiveness, regulatory quality, rule of law and control of corruption.Footnote16 Aggregating all the dimensions of governance into a single index helps to capture the joint impact of institutional quality. To capture variations in human capital across countries and time, we use the human capital index taken from the Penn World Table version 10.0 database.Footnote17 shows the definition of variables and data sources.
Table 1. Definition of variables and data sources.
After identifying the variables used in this study, we collect their data. The selection of the countries and sample period are based on the availability of annual data, spanning the period 2009 to 2019.Footnote18 Our sample is restricted to both lower and upper middle income countries based on the World Bank’s classification for the year 2019.Footnote19 Out of the 106 countries categorized as lower/upper middle income, only 43 countries have data on the variables BIOM and WIND either for the entire period under analysis or for some years of the sample period.Footnote20 During the data collection process, we find that there is no data available on some variables of interest in the case of 10 countries.Footnote21 Thus, this leaves us with a sample of 32 countries.Footnote22 These countries are major global renewable electricity producers, collectively accounting for over half of global renewable electricity production since 2017.Footnote23
After collecting their data, we implement the interpolation method to fill in the missing values of variables BIOM, WIND and COAL so as to obtain a balanced panel dataset.Footnote24 The missing values prevent us from running the spatial panel model because spatial econometrics need balanced panel data in order to take into account the spatial dependence at each point of time (Sanso-Navarro, Vera-Cabello, and Puente-Ajovín Citation2020).Footnote25 In the end, our final balanced panel dataset covers 32 countries over the 11-year period from 2009 to 2019, resulting in 352 observations.
The descriptive statistics for all the variables across the sample after the interpolation process are shown in .Footnote26 The correlation coefficients between disaggregated renewable energy sources and economic growth for all countries are reported in . This table suggests that BIOM/WIND and Y are highly positively and significantly correlated with each other in the majority countries.Footnote27 These results indicate that renewable energy sources play an important role in promoting economic activities across most countries.
Table 2. Descriptive statistics.
Table 3. Correlation between renewable energy and economic growth.
Estimation methods
Spatial dynamic panel model
We employ spatial dynamic panel models in order to simultaneously accommodate dynamic adjustments and spatial dependence and thus produce more accurate and reliable estimation results (Hao and Peng Citation2017).
EquationEquation. (2)(2)
(2) could be extended to include spatial interaction effects by specifying the following two spatial dynamic panel models:
and
Where is the real GDP per capita in country i at year t-1,
is an matrix of explanatory variables,
is an element of the spatial weights matrix,
is the spatial autoregressive coefficient,
represents coefficients of the explanatory variables,
is spatial coefficient of the explanatory variables,
is the individual effect,
corresponds to time fixed effect,
and
are the independent and identically distributed random error term,
represents beta convergence parameter.Footnote28
Before estimating the spatial dynamic econometric models, spatial weight matrix which captures the spatial dependence structure across countries in our sample needs to be defined. In earlier studies of spatial econometrics, the spatial weight matrix was most commonly specified to measure geographic proximity. The merit of geographical matrix is that it is indeed strictly exogenous (Arbia, Battisti, and Di Vaio Citation2010). Recently, economic proximity has been introduced to capture neighbourhood between spatial units. The matrix based on economic attributes could measure the distance beyond the geographical notion that still play an important role in shaping the economic relationships across countries (Anselin Citation2002; Arbia, Battisti, and Di Vaio Citation2010).
Recent literature has argued that international trade often serves as channels for economic transmission across countries and countries are ‘economically closer’ if they conduct a large volume trade with each other (Wang, Wong, and Granato Citation2015). Thus, in line with Nan et al. (Citation2022), our spatial analysis applies a spatial weight matrix that depends on intensity of bilateral trade flows so as to capture the economic interdependency among countries.Footnote29 The trade-intensity-based spatial weight matrix is defined as follows:Footnote30
Where is an element of unstandardized weight matrix
, and
represents total bilateral trade flows (import plus export) between country i and country j. Note that
is the average bilateral trade between country i and country j over the 2009–2019 period.Footnote31 This weight matrix is standardized such that the elements of a row sum up to one (i.e. row standardization).Footnote32
To check the robustness of the estimation results, we use a first-order queen contiguity weight matrix as an alternative form of the spatial weights matrix.Footnote33 The first-order queen contiguity weight matrix is binary and its element takes value 1 if country i and country j share a common geographic border or vertex, and
has a value of 0 otherwise. All elements of this matrix are also standardized before use.
Estimation issue
System Generalized Method of Moments (GMM) estimator is applied to estimate EquationEquation. (3)(3)
(3) and EquationEquation. (4)
(4)
(4) for several reasons.Footnote34 First, the system GMM estimator corrects for not only the endogeneity of the lagged dependent variable and the spatially lagged dependent variable but also the potential endogeneity of other explanatory variables.Footnote35 Thus, given the potential reverse causality between the explained and the explanatory variables, the system GMM estimator may yield consistent estimates of the desired causal effect.Footnote36
Second, the system GMM estimator controls for unobservable heterogeneity specific to countries which may affect both the dependent variable and the explanatory variables and thus ensure the reliability of our causal estimates (Nkoa and Song Citation2020). Third, the system GMM estimator performs better than the difference GMM estimator (Berk, Kasman, and Kılınç Citation2020).Footnote37 To overcome the drawbacks of the difference GMM approach, the system GMM approach estimates a system of two equations simultaneously: an equation in levels with lagged first differences as instruments, and an equation in first differences with lagged levels as instruments (Zheng et al. Citation2013).Footnote38 Fourth, the system GMM estimator is appropriate for short panel data (Roodman Citation2009).Footnote39 Finally, the system GMM estimator has been a popular estimation method for spatial dynamic panel models in the recent studies.Footnote40
Henceforth, accounting for the aforementioned problems, we use the two-step system GMM estimator to estimate the models in the present paper.Footnote41 lnY−1, W*lnY, lnBIOM, lnWIND, lnHYDR, lnCOAL, lnOIL, lnK, lnL, lnINST, W*lnBIOM and W*lnWIND are treated as endogenous in the estimation of EquationEquation. (3)(3)
(3) and EquationEquation. (4)
(4)
(4) . lnHC is considered as the predetermined variable in the estimation of the two equations. The first and above lags of the predetermined variable and the second and above lags of the endogenous variable are used for GMM-type instruments.Footnote42 Regional dummies and time trend are treated as exogenous, and they are instrumented in a IV style.
The consistency of the system GMM estimator depends on whether lagged values of the explanatory variables are valid instruments (Ogunniyi et al. Citation2020). To address this issue, three specification tests, including Hansen over-identification test, Arellano-Bond error autocorrelation test and difference-in-Hansen test, are necessary.Footnote43
III. Empirical results
Spatial autocorrelation test
To explore the existence of spatial autocorrelation in real GDP per capita, we adopt the global Moran’s I index which has been widely used in the literature on spatial studies. The global Moran’s I index describes the extent of the overall spatial relationship across all spatial units. The values of global Moran’s I index range over [−1,1].Footnote44 A positive value of Moran’s I index indicates spatial clustering across the sample countries, with a higher value implying a stronger association (i.e. more positively correlated). By contrast, a negative value denotes spatial dispersion across the sample countries, with a lower value implying a stronger association (i.e. more negatively correlated). A zero value indicates a random distribution of real GDP per capita across countries.
The values of Moran’s I index and the associated P-value for real GDP per capita over the period 2009–2019 by using the trade-intensity-based spatial weight matrix are presented in . As shown, the Moran’s I values for real GDP per capita were positive and statistically significant at the 5% and 10% significance levels for the entire time period, suggesting that real GDP per capita were not randomly distributed but rather exhibited a positive spatial interdependence. It implies that the countries with high real GDP per capita (resp. low) are localized close to other countries with high real GDP per capita (resp. low). Hence, the existence of spatial autocorrelation provides a support for necessity of considering spatial effects in the econometric estimation.
Table 4. Global Moran’s I index of GDP per capita using trade-intensity-based spatial weight matrix.
To further describe the heterogeneity of spatial association cross different geographic units within the areas under investigation, we construct Moran scatter plot (Marbuah and Amuakwa-Mensah Citation2017).Footnote45 Using the cross-sectional data of 2010 and 2018, we draw Moran scatter plot to visualize the atypical spatial associations in the data of economic output (see ). It reveals that in 2010 and 2018 most countries were located in the first and third quadrants, which implies that real GDP per capita displays an obvious positive correlation in spatial distribution. More specifically, the plots show that in 2010 and 2018, 21 countries (66%) and 20 countries (63%) were located in the first and third quadrants.Footnote46 Therefore, the graphical evidence shows the presence of spatial heterogeneity and therefore implies the necessity to consider spatial effects in our empirical analysis.
Panel unit root and cointegration tests
We perform the panel unit root tests and cointegration tests before estimating the empirical models described in the previous section in order to avoid spurious regression problem. To check the stationarity of the variables, HT (Harris and Tzavalis Citation1999) and CIPS (Pesaran Citation2007) unit root tests are used in this study.Footnote47 The CIPS test which produces accurate results in the presence of cross-sectional dependence is preferred because Pesaran’s (Citation2004) cross-section dependence (CD) tests show that each series exhibits cross-sectional dependence (see ). Results for all the unit root tests are reported in . As shown, all the variables become stationary after first differencing. Then the integration of all the variables is of order 1, i.e. I(1).
Table 5. Cross-sectional dependence test.
Table 6. Panel unit root tests.
The unique order of integration of these variables leads us to further test the existence of cointegration between them. In doing so, we perform the panel cointegration tests using the Kao cointegration test (Kao Citation1999).Footnote48 The panel cointegration test results are presented in . We find that the null hypothesis of no cointegration is rejected at either 1%, 5% or 10% significance levels for all five test statistics under the Model A (Biomass & waste) and Model B (Wind). Equally, four out of the five statistics suggest the rejection of the null hypothesis of no cointegrating relationships under the Model C (Biomass & waste and Wind). To sum up, we could conclude the existence of a long-run cointegration relationship among the variables used in this paper. The evidence of unit root tests and cointegration tests support that the empirical analysis discussed in the next subsection is free from spurious regression problem.
Table 7. Kao panel cointegration tests.
Spatial dynamic regression results
presents the estimated results of the spatial dynamic system GMM panel models. The diagnostic statistics reported in the show that our spatial dynamic system GMM estimates meet all specification tests, indicating that our estimates are effective and consistent. As expected, the first-order autocorrelation (AR(1)) is present and the second-order autocorrelation (AR(2)) is absent. Hansen tests fail to reject the null hypothesis of jointly valid instruments for all estimated models, indicating that all the instrumental variables used in this study are effective. At the same time, difference-in-Hansen tests fail to reject the null hypothesis of exogeneity of the set of examined instruments, confirming that the instruments are reasonable and effective. In addition, in accordance with Roodman’s (Citation2009) rule of thumb, the number of instruments is not larger than that of countries in all of the estimated models.
Table 8. Dynamic SYS-GMM results using economic distance weight matrix.
Turning to the coefficient estimates, the estimated coefficient on the lagged dependent variable (lnY−1) is positive and statistically significant at the 1% level in each model, implying that GDP per capita has a path-dependence effect. This evidence corroborates the necessity of using the dynamic panel model.Footnote49 Moreover, the estimated coefficients of lnY−1 are inferior to 1 across all the specifications, indicating that there is conditional beta convergence in GDP per capita across countries when spatial effect is accounted for. According to the estimation results shown in , the conditional rates of convergence () range from 0.088 to 0.181.Footnote50
The estimated coefficients of the spatially lagged dependent variable (W* lnY) are significantly positive at either 1%, 5% or 10% significance levels across all the models (except model (4)). This finding reveals the positive spatial dependence of GDP per capita, that is, the GDP in one country is positively affected by its neighbouring countries’ GDP. This result is consistent with Chica-Olmo, Sari-Hassoun, and Moya-Fernández (Citation2020) evidence derived from 26 European countries over the period 1991–2015. Our finding further provides evidence for regional interconnectivity implying that the growth performance of developing countries depends on the growth rate of neighbouring regions.
The estimated parameters of electricity production from biomass & waste (lnBIOM) are positively and highly significant across all the models.Footnote51 The estimated coefficients on wind power (lnWIND) are uniformly positive and consistent across all the models, albeit it is not statistically significant in two specifications. These results support the presence of the energy-led growth hypothesis for renewable energy sources.Footnote52 Our findings are in line with Chen, Pinar, and Stengos (Citation2020), Ito (Citation2017) and Le, Chang, and Park (Citation2020) who conclude that renewable energy is a driver of economic growth in developing countries. Our results further illustrate that individual renewable sources have positive impact on economic growth to different extents, and therefore it is necessary to disentangle the aggregate measures into disaggregate measures.Footnote53 This is consistent with the evidence reported in Armeanu, Vintila, and Gherghina (Citation2017) and Ohler and Fetters (Citation2014).
The estimated coefficient of the spatial lag for lnBIOM is significantly negative in model (2), and the coefficient of the spatial lag for lnWIND is significantly negative in both model (4) and model (6). These results suggest that the development of biomass & waste or wind power in neighbouring countries reduces the economic growth in home country. These results, to our knowledge for the first time, provide evidence of spatial spillover effects from renewable energy on economic growth for developing countries. These findings further justify that the heterogeneous spatial impacts of some particular renewable energy sources on economic growth may be masked by the total measure.
A possible explanation for negative spatial effects lies with the interactions between faster development of biomass & waste or wind power in nearby countries and the investment competition effect between the country and its neighbourhood. That is to say, faster development of biomass & waste or wind power in nearby countries benefitting from better renewable policy supports may trigger a re-location of foreign firms from a specific country to these countries.
For biomass, another possible explanation for this result is that a country in the development phase of the biomass sector may lead neighbouring countries to export raw or non-processed products, and large-scale production in the neighbouring countries could have a negative impact on their own potential growth.Footnote54 Concerning the wind sector, another possible explanation for the negative spatial effects may be due to the displacement of environmentally harmful technologies. Concretely, the maturity of wind technology in surrounding countries may induce local country to increase its wind imports from the surrounding countries. A higher share of wind imports render wind power more competitive to alternative existing carbon-intensive fossil technologies, and thereby contributing to displace the latter in importing countries (Garsous and Worack Citation2022). The displacement of environmentally harmful technologies may subsequently result in economic losses (Xia and Song Citation2017).
Turning to the coefficients of traditional energy sources, the estimates, on the whole, show a negative and significant impact of hydroelectricity (lnHYDR) on economic growth, whereas a positive and insignificant impact of coal (lnCOAL) and oil (lnOIL)
on economic growth. Our result about the negative coefficient of hydroelectricity is inconsistent with the findings of Solarin and Ozturk (Citation2015) and Ummalla and Samal (Citation2018), showing that hydropower is a driving force to enhance the economic growth.Footnote55 Our result is not surprising because hydroelectricity usage empirically proves to be harmful to the environment and by extension to the economic growth (Dash, Dash, and Sethi Citation2022). Our new evidence that is different from the finding of previous studies may be due to the use of different estimation techniques, different countries and sample period. The observed positive effect of oil (lnOIL) on economic growth is consistent with the evidence reported in Long et al. (Citation2015) and Marques and Fuinhas (Citation2012), suggesting that oil is a stimulus to economic growth.
The insignificant (with mixed signs) coefficient for coal (lnCOAL) is largely in line with the existing studies whose findings are at best inconclusive. Some studies lent their view in support of the coal as a driver of economic growthFootnote56 while others hold the opposite view.Footnote57 In fact, the inconclusive findings may be explained by two opposing forces and the net effect of coal on economic growth depends on the relative strength of each opposing force. On the one hand, coal is a relatively cheap energy source and thus remains the engine of economic growth (Marques and Fuinhas Citation2012). On the other hand, coal is the most carbon-intensive energy source which could damage the environment and therefore the economic expansion (Marques and Fuinhas Citation2012; Udi, Bekun, and Adedoyin Citation2020).Footnote58 These results attest to the importance of considering the dissimilar and even contrary effects of disaggregated traditional energy sources on economic growth.
Conforming to the findings of many studies, a positive and significant effect of labour on economic growth is observed.Footnote59 It implies that labour is a major driver behind the economic growth of developing countries. However, this is not the case for capital. As shown in , the coefficients of lnK are positive in models (1) - (5). These findings support the earlier studiesFootnote60 which reported that capital enhances economic growth. In contrast, the coefficient of lnK is negative in model (6). This result is consistent with the recent findings of Topcu, Altinoz, and Aslan (Citation2020), suggesting that capital has a negative effect on economic growth.Footnote61 Thus, the impact of capital on economic growth deserves more attention.
Next we turn to the coefficients of control variables. We observe that the estimated coefficients of institutional quality (lnINST) are positively and significantly at either 1%, 5% or 10% significance levels across all the models (except model (3)). This outcome is in accordance with a large body of literatureFootnote62 and supports the well documented insight that institutional quality is a critical driver of economic growth. We also find that human capital (lnHC) exerts a statistically significant impact on economic growth in models (3), (4) and (6). In addition, the positive effects of human capital are stable in all the models. Our results confirm the previous evidenceFootnote63, suggesting that human capital fosters economic growth.
Finally, to provide further validation of the baseline empirical estimates, we carry out an additional sensitivity analyses via alternative weight matrix specifications. Estimates of these robustness checks, reported in Appendix (see ), show that the main results are quite similar to the baseline and do not depend on the choice of weight matrix.
IV. Conclusion and policy recommendations
Given the increasing investment in renewable energy in developing countries, this paper attempts to test the energy-led growth hypothesis for renewable energy sources for these countries. To this end, the present paper uses a balanced panel data of 32 lower/upper middle income countries over the period 2009 to 2019 and applies spatial dynamic techniques to investigate the impacts of disaggregate renewable energy sources on economic growth within a multivariate framework including the disaggregated non-renewable energy, capital, labour, institutional quality and human capital.
This paper provides statistically significant evidence for the positive impacts of electricity production from biomass & waste and wind power on economic growth of developing countries. Overall, these results validate the energy-led growth hypothesis for renewable energy sources. This study shows the first piece of evidence of spatial spillover effects from disaggregated renewable energy on economic growth for developing countries. Our results provide support on the existence of spatial dependence of economic growth across countries. Our analysis reveals the significantly negative impacts of hydroelectricity on economic growth. Our analysis also confirms the importance of labour, institutional quality and human capital in driving economic growth.
Our evidence of spatial spillover effects from renewable energy on economic growth points to the need to consider a spatial dependence in terms of renewable energy transition which has important policy implications for economic outcome. The spatial dependence of transition towards renewable energy is characterized by uneven distribution of renewable energy supply and demandFootnote64, energy technological disparities in spaceFootnote65, as well as competitive dynamics in technology developmentFootnote66 (Noseleit Citation2018). These characteristics provide a rationale for government to pay special attention to the potential difficulties caused by country borders as spatial barriers that can hamper energy transition (Noseleit Citation2018). The spillover mechanism of energy transition further plays a crucial role in sustainable growth processes.
Given the extent to which the economic growth of developing countries depends on various renewable energy resources, policymakers should overcome current barriers to renewable energy deployment and pursue active policies to promote renewable energy for sustainable growth. First of all, government should play a fundamental role in how to finance the energy transition on both the supply side (R&D and infrastructures) and the demand side (education and communication to change individual and social preferences).Footnote67 Second, in developing countries, the renewable energy sector refers to infant industries that do require particular forms of government assistance based upon specific instruments of industrial or sector-oriented policyFootnote68 Third, energy policies directed at the diffusion of renewable energies should strengthen the entire value chain from the manufacturer to the end user.Footnote69 Finally, in the current globalized context where externalities, free-riding and public budget constraints play an important role, traditional policy tools such as taxes or subsides should be complemented with other solutions based on new financial engineering and leverage jointly with the restoration of missing markets (pollution permits).
Meanwhile, the policymakers should attach equal importance to energy security alongside renewable energy deployment.Footnote70 The increasing pressure to decarbonize energy systems has triggered a strong interest in favour of the integration of renewable energy sources in electricity mix (Hache and Palle Citation2019; Radulescu and Sulger Citation2022). However, the integration of renewable energy sources in electricity mix poses energy security challenges for several reasons (Radulescu and Sulger Citation2022). First, large-scale integration of renewable energy sources may introduce additional uncertainty to an existing power system due to the intermittent nature of these sources (Das et al. Citation2018; Hache and Palle Citation2019).Footnote71 In this context, a flexible power system is critical to ensure large-scale penetration of intermittent renewable energy sources (Das et al. Citation2018).Footnote72 It is widely perceived that flexible backup unitFootnote73 is an essential part of the solution to cope with the intermittency. Nevertheless, the subsidized renewable energy sources distort investment incentives for conventional backup capacity and thus the adverse investment effects of renewable energy sources may pose a threat to adequate electricity supply in the long-run (Liebensteiner and Wrienz Citation2020). Second, pursuing renewable energy transition may curtail energy sovereignty.Footnote74 The pressure for fighting against climate change pushes the countries, in which renewable resource endowments are limited and energy system infrastructures are less developed, to integrate their power systems with neighbouring countries (Thaler and Hofmann Citation2022). The cross-border power systems allow improving reliability and availability of electricity. Despite this, the integration into cross-border power systems require common rules and lead to reduced autonomy in energy policymaking (Thaler and Hofmann Citation2022). Third, renewable energy transition policies may induce new energy security challenges. The transition towards renewable energy may result in geopolitical consequences, which are less conflictual than those of fossil fuels, by transforming patterns of cooperation and conflict between countries (Hache Citation2018; Scholten et al. Citation2020). The expansion of renewable energy technologies could lead to new interdependences, especially, dependences to critical minerals and metals (Hache Citation2018; Scholten et al. Citation2020). The dependences to critical minerals and metals may exacerbate competition for access to these materials among countries that compete for industry in renewable generation technologies (Scholten et al. Citation2020).
The current limitations regarding available data prevent the extension of analysis to additional years and to more countries, and prevent the investigation of other renewable energy sources across developing countries, and therefore it is left for further research.
Acknowledgments
The authors gratefully acknowledge the financial support from the MOMENTOM (MOlecules and Materials for the ENergy of TOMorrow) project, a Strategic Research Initiative of the Université Paris-Saclay. We are also grateful for comments from colleagues in GDRI International Development Economics conference at Université Clermont Auvergne, 15-16 November 2018 in Clermont-Ferrand. In particular, we sincerely thank the anonymous reviewers for the constructive comments and suggestions, which helped us to substantially improve our manuscript.
Disclosure statement
No potential conflict of interest was reported by the authors.
Additional information
Funding
Notes
1 The report also revealed that the top ten renewable energy investors consisted of six developing countries and four developed countries in 2015. The six developing countries are Brazil, China, India, Mexico, the Russian Federation and South Africa. The four developed countries include Chile, Germany, Japan and United States.
2 The energy-growth literature focuses on testing four hypotheses: the growth hypothesis (unidirectional causality from energy to economic growth); the conservation hypothesis (unidirectional causality from economic growth to energy); the feedback hypothesis (bidirectional causality between energy and economic growth); the neutrality hypothesis (no causality between energy and economic growth). See Apergis and Payne (Citation2012) and Tugcu, Ozturk, and Aslan (Citation2012) for a discussion in detail.
3 The differences in sample periods, econometric methodologies and countries’ characteristics are considered as the main reasons of the rather conflicting results (Ozturk Citation2010; Tugcu, Ozturk, and Aslan Citation2012).
4 In contrary, the results from the analysis of aggregate renewable energy cannot be generalized to disaggregate renewable energy and thus the policy suggestions based on this kind of studies are hard to implement (Fang and Chen Citation2017).
5 To our knowledge, only the following two studies have provided disaggregated analyses for developed countries. Ohler and Fetters (Citation2014) have provided empirical evidence by examining the causal relationship between individual renewable sources (including biomass, geothermal, hydroelectric, solar, waste, and wind) and economic growth using data from 20 OECD countries for the period 1990–2008. Armeanu, Vintila, and Gherghina (Citation2017) have analysed the influence of disaggregate renewable energy (including biomass, hydropower, geothermal, wind and solar) on the economic growth of European Union (EU)-28 countries for the period of 2003–2014. These two studies highlight the importance of this new avenue of research.
6 Among the studies reviewed, only one study, Long et al. (Citation2015), differentiated between disaggregated renewable and non-renewable energy sources.
7 To our knowledge, only the following three studies have made important contributions by capturing the idea of spatial effects. Chica-Olmo, Sari-Hassoun, and Moya-Fernández (Citation2020) investigated the spatial dependence between GDP and aggregated renewable energy consumption by using a spatial Durbin model with two-way fixed effects and focusing on 26 European countries over the period 1991–2015. Radmehr, Henneberry, and Shayanmehr (Citation2021) explored the relationships among economic growth, aggregated renewable energy consumption and carbon emissions by applying spatial simultaneous equations models with a generalized spatial two-stage least squares method and analysing panel data from 21 European countries over the period 1995–2014. Cui, Weng, and Song (Citation2022) investigated the spatial effects of both aggregated renewable energy consumption and financial inclusion on economic growth by employing a spatial Durbin model with fixed effects and using panel data from 40 countries for the period 2010–2020.
8 Literature concerning economic growth has indicated that physical capital and labour are the conventional factors of economic production (see Arbex and Perobelli Citation2010). Numerous studies have documented the important role of institutional quality and human capital in economic growth (see Fang and Chen Citation2017; Zallé Citation2019). Therefore, we incorporate capital, labour, institutional quality and human capital into the production process.
9 The individual fixed effect is included in the model to control for time-invariant regional characteristics, which may affect both economic growth and renewable energy supply, such as resource endowment and climate conditions. The inclusion of individual fixed effect enables us to yield causal inference. We thank an anonymous reviewer for pointing out this issue. Regional dummies distinguish regions to which countries belong. All the countries in the sample are divided into Asian, African, European and American regions.
10 The time fixed effect is incorporated into the model to account for time-dependent macro factors that may influence both economic performance and renewable energy supply capacity, such as, financial crises, external energy demand shocks, and changes over time in global energy prices. We use a linear time trend to control for time-specific factors.
11 We divide the capital and labour variables by total population (extracted from World Development Indicators) to calculate the fixed capital per capita and labour force per capita.
12 We could not include natural gas production in our analyses because of the large missing information in both the U.S. Energy Information Administration and the International Energy Agency datasets which severely restricts the number of countries available for analysis.
13 We use Citation2022 conversion factors to convert coal supply and oil supply measured in terajoule into million tonnes of oil equivalent.
14 We consider hydropower as a traditional energy source for two reasons. First, the development of hydropower unavoidably results in environmental risks, such as, forest ecosystem degradation and water quality deterioration. Second, hydropower has been a technically well-established energy source and has served as the foundation energy source for many countries. Thus, hydropower hardly reflects the advanced development of energy resources. We thank an anonymous reviewer for pointing out these issues.
15 The U.S. Energy Information Administration database also contain data on coal production and petroleum and other liquids production. However, these data are largely unavailable for many countries (especially for developing countries) under our studied period. Thus the International Energy Agency database is preferred.
16 We use percentile rank in order to avoid the possibility of negative or zero scores in the logarithm. The percentile rank ranging from 0 corresponding to lowest rank to 100 being the highest.
17 Human capital index has been widely used in previous studies (see, e.g. Yao et al. (Citation2019)).
18 We choose 2009 as the starting period because the data on the variables BIOM and WIND are largely unavailable for the earlier period. We select 2019 as the ending year since the data on the variables COAL and OIL are available only until this year for most countries.
19 Both lower and upper middle income countries are included in the sample in order to increase the sample size and better fulfil the requirements of the estimation method used in the next subsection. We do not consider low income countries in our analysis because the data for the variable WIND is missing for almost all the low income countries spanning 11 years (2009–2019).
20 We allow countries with a maximum of six missing observations for each variable (BIOM and WIND), in whose case we interpolate the data. So the countries with gaps in excess of 6 years are excluded from the sample, like Bosnia and Herzegovina, and Lebanon.
21 Concretely, the countries Azerbaijan, Ecuador, Fiji, Guyana, Moldova and Vanuatu are excluded from the sample due to the lack of data on coal supply over the entire studied period. In addition, Bulgaria, Cuba, Philippines and South Africa are excluded from the analysis for the missing human capital data.
22 Cambodia is also not included in our sample, because the exact same values for the variable WIND are reported for all the 11 years under analysis. In detail, this study covers Argentina, Bangladesh, Belarus, Bolivia, Brazil, China, Colombia, Costa Rica, Dominican Republic, Egypt, Guatemala, Honduras, India, Indonesia, Iran, Jamaica, Jordan, Kazakhstan, Kenya, Mexico, Morocco, Nigeria, North Macedonia, Pakistan, Peru, Russian Federation, Serbia, Sri Lanka, Thailand, Turkey, Ukraine and Vietnam. Among these countries, 11 countries are located in Asia, 4 countries are located in Africa, 6 countries are located in Europe, and 11 countries are located in America. Thus, our sample covers countries with different geographical proximity.
23 Authors’ own calculation based on data from U.S. Energy Information Administration. In detail, our sample of 32 countries collectively accounts for 44.5%, 46%, 45.4%, 46.8%, 46.9%, 48%, 48.5%, 49.5%, 50.1%, 51.1%, and 52.2% of global renewable electricity production in 2009, 2010, 2011, 2012, 2013, 2014, 2015, 2016, 2017, 2018 and 2019, respectively.
24 The missing information rates of the variables BIOM, WIND and COAL are 6.25%, 6.82% and 1.99%, respectively.
25 Therefore, we capitalize on the available data and interpolate the missing values by assuming a linear relation and using an inverse distance weight with the available value that is the nearest having the largest weight (Castañeda Rodríguez Citation2018). The missing values are estimated through interpolation with the help of the Stata command mipolate.
26 We further compute coefficient of variation that is defined as the ratio of standard deviation to mean and used to measure the relative dispersion of the variables. In detail, the coefficient of variation for WIND, COAL, BIOM, HYDR, OIL, K, INST, HC and L are 4.589, 4.118, 2.991, 2.844, 2.005, 0.656, 0.322, 0.26 and 0.195. Thus, WIND is the most volatile explanatory variable, followed in order by COAL, BIOM, HYDR, OIL, K, INST, HC and L.
27 For example, the highest correlation value between BIOM and Y is found in China (0.9919), followed by Turkey (0.9589) and Mexico (0.9526). The highest correlation value between WIND and Y is found in Turkey (0.9936), followed by China (0.9912) and India (0.9840).
28 If is statistically significant and inferior to 1, the beta convergence of real GDP per capita is confirmed.
29 Larger value of intensity of bilateral trade flows between two countries implies higher dependence between the countries.
30 The choice of a spatial weight matrix is relatively subjective as it is not guided by economic theory (Hao and Peng Citation2017; Marbuah and Amuakwa-Mensah Citation2017).
31 To avoid the possible endogeneity problem, we follow Ertur and Koch (Citation2011) by calculating the average bilateral trade over the 2009–2019 period. Data on trade flows for each country are taken from the UN Comtrade Database.
32 Row standardization allows us to interpret the spatial spillover effects as an average of all neighbours (You and Lv Citation2018).
33 The queen continuity criterion is one of the most popular criteria for creating geographic weight matrix in the existing literature (see e.g. Cho, Chen, and Poudyal Citation2010; Leiva, Vasquez-Lavín, and Oliva Citation2020, among many others).
34 The system GMM estimator is suggested by Arellano and Bover (Citation1995) and Blundell and Bond (Citation1998).
35 Spatial panel literature widely uses maximum likelihood estimator. However, this estimator would produce inconsistent and biased estimates if the potential endogeneity of other explanatory variables is present (Zheng et al. Citation2013).
36 In this study, endogeneity arising from the reverse causality between renewable energy sources and economic growth may be a serious problem in our model. We are grateful to an anonymous referee for the advice to tackle the problem of reverse causality in our empirical models. One can imagine that, for instance, the worldwide economic crisis may decrease dramatically the investment in renewable energy sources (Li et al. Citation2021). The presence of endogeneity in our model could also potentially be explained by the reverse causality from traditional energy sources to economic growth. This reverse causality hypothesis touches the energy-growth literature, which provides evidence on the causality running from economic growth to energy use (see Acheampong et al. Citation2021). Another important concern of endogeneity in our model related to the potential feedback effects from economic growth to institutional quality. That is because the existing literature suggests that economic growth causes improvement in institutional quality (Law, Lim, and Ismail Citation2013).
37 The difference GMM estimator is proposed by Arellano and Bond (Citation1991). In the difference GMM approach, the strategy is to remove the individual fixed effect by proceeding with the first difference of level equation and then use lagged variables as the instruments of endogenous variables in the difference equation (Trotta, Hansen, and Sommer Citation2022; Zheng et al. Citation2013). The difference GMM estimator suffers from the weak instrument problem which can result in large finite-sample bias and poor precision (Ogunniyi et al. Citation2020; Trotta, Hansen, and Sommer Citation2022). Weak instrument problem means that lagged levels of the dependent variable are weak instruments for first differences (Ogunniyi et al. Citation2020; Trotta, Hansen, and Sommer Citation2022).
38 This allows the introduction of more instruments and thus can dramatically improve estimation efficiency (Roodman Citation2009; Trotta, Hansen, and Sommer Citation2022).
39 The short panel data means large cross-sectional dimension with short time dimension, as in our case (N = 32; N = 11).
40 See e.g. Espoir and Sunge (Citation2021) and Zheng et al. (Citation2013), among many others.
41 The system GMM method can be divided into one-step and the two-step estimation methods in accordance with different choices of the weight matrix. The one-step estimator assumes that the error terms are homoscedastic across groups and over time. The two-step estimator uses the estimated residuals from the one-step estimator to construct more efficient heteroskedasticity consistent GMM weighting matrix (Davidson and MacKinnon Citation2004). In addition, the two-step estimation has been proven to be more efficient than the one-step estimation.
42 The two techniques suggested by Roodman’s (Citation2009) are further used together to address the problem of ‘too many instruments’. This problem means that a high instrument count can over fit endogenous variables and weaken the power of the Hansen test to detect invalidity of the system GMM instruments (Roodman Citation2009). On the one hand, we use only certain lags instead of all available lags for instruments (limiting lags) and combine instruments by adding them into smaller sets (collapsing instruments). On the other hand, conforming to Roodman’s (Citation2009) rule of thumb, we keep the number of instruments never larger than the number of individual units in the panel. The regressions are run using Stata command xtabond2.
43 The Hansen over-identification test examines the overall validity of the instruments by employing the null hypothesis that all instruments as a group are exogenous. The acceptance of this hypothesis means that the instruments are not correlated with the error term (i.e. the instruments are valid). The Arellano-Bond error autocorrelation test is performed under the null hypothesis of no first- or second-order serial correlation in the first-differenced error term. The assumption of no first-order serial correlation should be rejected, whereas the hypothesis of no second-order serial correlation should be accepted. The difference-in-Hansen test investigates the validity of instrument subsets by employing the null hypothesis of exogeneity of the set of examined instruments. Failure to reject the null hypothesis implies that the instruments are exogenous.
44 The global Moran’s I index can be expressed as: Where
is the number of spatial units (i.e. countries).
and
represent real GDP per capita of country
and
, respectively.
is the mean value of real GDP per capita, i.e.
.
is an element of the spatial weight matrix.
45 The Moran scatter diagram plots the spatial lag of variable (Wz) against the original value (z). The values are distributed into four quadrants which correspond to the four types of local spatial association between a region and its neighbours. The first quadrant (upper-right), which depicts a region with a high value is surrounded by neighbours with high values, representing a positive spatial autocorrelation (High-High, HH). The second quadrant (upper-left), which represents a low-value region is surrounded by high-value regions, indicating a negative spatial autocorrelation (Low-High, LH). The third quadrant (lower-left), represents a low-value region is surrounded by low-value regions, meaning a positive spatial autocorrelation (Low-Low, LL). The fourth quadrant (lower-right), represents a high-value region is surrounded by low-value regions, denoting a negative spatial autocorrelation (High-Low, HL).
46 As an illustration in 2010, Belarus and Argentina were located in the first quadrant (i.e. High-High clustering), meaning that countries with high real GDP per capita surrounded by other countries with high real GDP per capita. Kenya and Sri Lanka were found in the third quadrant (i.e. Low-Low clustering), implying that countries with low real GDP per capita surrounded by other countries with low real GDP per capita.
47 The HT is the first generation test that ignores cross-sectional dependence. The CIPS is the second generation test that allows for cross-sectional dependence. The null hypothesis for these two tests is that a unit root exists.
48 The mean of the series across panels is subtracted before performing the panel cointegration test in order to mitigate the impact of cross-sectional dependence (Levin, Lin, and Chu Citation2002; You and Lv Citation2018).
49 The estimated coefficients of lnY−1 lie between the fixed effects estimate (which is downward biased) and the pooled OLS estimate (which is upward biased), indicating that the system GMM estimates are not subjected to significant finite sample bias (Zheng et al. Citation2013).
50 The convergence rate is obtained using
and
is the coefficient of lnY−1 (Hao and Peng Citation2017).
51 The countries which have biomass surpluses tend to use it rather locally in order to replace fossil fuels. In this regard, the positive and significant estimated parameters (lnBIOM) confirm that the local use of biomass can positively affect the economic growth of these countries with large local markets and suitable processing infrastructures.
52 We further use the novel panel Granger non-causality test, developed by Juodis, Karavias, and Sarafidis (Citation2021), to provide supportive evidence of one-way causality running from renewable energy sources to economic growth. This test accounts for cross-sectional dependence in the data series, efficiently estimates the panel Granger non-causality in heterogeneous or homogeneous panels and is robust for panel data with a moderate time dimension. So this test is suitable for our dataset. Under the null hypothesis, there is no causality in any cross-section units of the panel. Under the alternative hypothesis, there is causality in at least one cross-section unit. This test is applied on the first difference of the series under examination, because this test requires that all the variables must be stationary. The estimated results of the test with two lags are presented in . As shown, it suggests to reject the null hypothesis at either 1%, 5% or 10% significance levels. Therefore, the Granger causal relationships from individual renewable sources to economic growth is evidenced for the whole region under examination.
53 The coefficients of lnBIOM range from 0.0133 to 0.0246, and the coefficients of lnWIND range from 0.0012 to 0.0034 (see ).
54 Among the selected countries, the Russian Federation is one of the worldwide leaders in the production and export of industrial roundwood, with a large forest stock. The attractiveness of the neighbouring country confines the Russian wood industry to a primary specialization. It exports most of its industrial roundwood to China whose growing demand exceeds local production. This international position and the Russian fossil fuels-oriented energy policy do not encourage investment in local processing infrastructure allowing the deployment of the local use of biomass for energy purposes and a move upmarket in the associated value chain. The resulting increase in demand and employment of skilled labour related to this industry would drive human capital away from innovation activities within the biomass sector, reducing growth over the long run (Grossman and Helpman Citation1991; Young Citation1991).
55 The studies on the nexus between hydroelectricity and economic growth are still scarce in the energy-growth literature (Dash, Dash, and Sethi Citation2022; Ummalla and Samal Citation2018).
56 See Chen et al. (Citation2022) and Long et al. (Citation2015).
57 See Marques and Fuinhas (Citation2012) and Udi, Bekun, and Adedoyin (Citation2020).
58 The economic benefit of coal use may be outweighed by the economic costs of mitigating carbon emissions (Apergis and Payne Citation2010).
59 See Koçak and Şarkgüneşi (Citation2017) and Le, Boubaker, and Nguyen (Citation2021).
60 See Apergis and Payne (Citation2012) and Koçak and Şarkgüneşi (Citation2017).
61 The negative impact of capital on economic growth may be due to the inadequacy of capital accumulation that is the most important limit to sustainable economic growth (Nweke, Odo, and Anoke Citation2017; Topcu, Altinoz, and Aslan Citation2020). Because the impact of capital accumulation on economic growth depends on the intensity of its determinants (such as savings and foreign direct investments), and the changes in these determinants could affect positively or negatively capital accumulation which in turn affect the economy as a whole (see Nweke, Odo, and Anoke (Citation2017) for a detailed discussion).
62 See e.g. Maruta, Banerjee, and Cavoli (Citation2020) and Nawaz, Iqbal, and Khan (Citation2014), among many others.
63 See Fang and Chen (Citation2017) and Siddiqui and Rehman (Citation2017).
64 The imperfectly correlated renewable energy supply and demand across countries promotes the development of cross-border energy markets so as to share ‘back-up’ production capacities and meet excess demand (Abrell and Rausch Citation2016).
65 The uneven development of new energy technology in space is responsible for the adoption and diffusion speed of renewable energy innovation across countries. The countries that are spatially proximate to the location of innovation may experience an ease technology diffusion, whereas, the countries that are far away from the innovation may experience substantial delay in adopting new technological solutions.
66 Different domestic and foreign technologies compete in space may result in more complex spatial patterns of energy transition towards renewable sources.
67 Initial investment costs are much more significant in emerging renewable energies, such as, wind (biomass) power, than in the conventional alternative. These financing costs might offset the ‘natural’ comparative advantage that developing countries may have.
68 Such as, tax credits or exemptions, employment credits, privileged access to scarce factors of production, and provision of credit at below market rates..
69 Because the diffusion of renewable energies not only depends on the improvement of scientific and technical knowledge, but also depends on the potential adopters, the endowment of natural resources, the development level and the policies that are practiced.
70 Achieving energy security remains a difficult task for developing countries (Gyeltshen Citation2022). Although the issue of energy security is pervasive in the literature, there is no consensus on the definition of energy security (Guivarch and Monjon Citation2017). The definitions of energy security have evolved over time, from the initial concept of securing oil supply, later extended to secure the whole energy supply system, recently, the concept has encompassed global warming and sustainability aspects (Gyeltshen Citation2022).
71 The intermittency problem has been a major constraint for transitioning to an energy system based primarily on renewable energy sources, given that power supply must match power demand at any time (Helm and Mier Citation2021).
72 A power system is flexible if it can manage production or consumption in support the power system stability (Loisel et al. Citation2022). The sources of power system flexibility include thermal power plants, energy storage, demand-side management and grid interconnections (Das et al. Citation2018; Loisel et al. Citation2022).
73 For example, gas or coal fired power plants.
74 Energy sovereignty is defined as a nation’s ability and authority to control, regulate and manage its own energy (Hansen and Moe Citation2022; Jewell, Cherp, and Riahi Citation2014).
References
- Abrell, J., and S. Rausch. 2016. “Cross-Country Electricity Trade, Renewable Energy and European Transmission Infrastructure Policy.” Journal of Environmental Economics and Management 79: 87–113. doi:10.1016/j.jeem.2016.04.001.
- Acheampong, A. O., E. Boateng, M. Amponsah, and J. Dzator. 2021. “Revisiting the Economic Growth–Energy Consumption Nexus: Does Globalization Matter?” Energy Economics 102: 105472. doi:10.1016/j.eneco.2021.105472.
- Adekoya, O. B., O. S. Yaya, J. A. Oliyide, and S. M. A. Posu. 2022. “Growth and Growth Disparities in Africa: Are Differences in Renewable Energy Use, Technological Advancement, and Institutional Reforms Responsible?” Structural Change and Economic Dynamics 61: 265–277. doi:10.1016/j.strueco.2022.02.020.
- Alam, M. S., S. R. Paramati, M. Shahbaz, and M. Bhattacharya. 2017. “Natural Gas, Trade and Sustainable Growth: Empirical Evidence from the Top Gas Consumers of the Developing World.” Applied Economics 49 (7): 635–649. doi:10.1080/00036846.2016.1203064.
- Anselin, L. 1988. Spatial Econometrics: Methods and Models. Dordrecht: Kluwer Academic Publishers.
- Anselin, L. 2002. “Under the Hood Issues in the Specification and Interpretation of Spatial Regression Models.” Agricultural Economics 27 (3): 247–267. doi:10.1111/j.1574-0862.2002.tb00120.x.
- Apergis, N., and J. E. Payne. 2010. “Coal Consumption and Economic Growth: Evidence from a Panel of OECD Countries.” Energy Policy 38 (3): 1353–1359. doi:10.1016/j.enpol.2009.11.016.
- Apergis, N., and J. E. Payne. 2012. “Renewable and Non-Renewable Energy Consumption-Growth Nexus: Evidence from a Panel Error Correction Model.” Energy Economics 34 (3): 733–738. doi:10.1016/j.eneco.2011.04.007.
- Apergis, N., and C. F. Tang. 2013. “Is the Energy-Led Growth Hypothesis Valid? New Evidence from a Sample of 85 Countries.” Energy Economics 38: 24–31. doi:10.1016/j.eneco.2013.02.007.
- Arbex, M., and F. S. Perobelli. 2010. “Solow Meets Leontief: Economic Growth and Energy Consumption.” Energy Economics 32 (1): 43–53. doi:10.1016/j.eneco.2009.05.004.
- Arbia, G., M. Battisti, and G. Di Vaio. 2010. “Institutions and Geography: Empirical Test of Spatial Growth Models for European Regions.” Economic modelling 27 (1): 12–21. doi:10.1016/j.econmod.2009.07.004.
- Arellano, M., and S. Bond. 1991. “Some Tests of Specification for Panel Data: Monte Carlo Evidence and an Application to Employment Equations.” The Review of Economic Studies 58 (2): 277–297. doi:10.2307/2297968.
- Arellano, M., and O. Bover. 1995. “Another Look at the Instrumental Variable Estimation of Error-Components Models.” Journal of Econometrics 68 (1): 29–51. doi:10.1016/0304-4076(94)01642-D.
- Armeanu, D. S., G. Vintila, and Ş. Gherghina. 2017. “Does Renewable Energy Drive Sustainable Economic Growth? Multivariate Panel Data Evidence for EU-28 Countries.” Energies 10 (3): 381. doi:10.3390/en10030381.
- Basile, R. 2008. “Regional Economic Growth in Europe: A Semiparametric Spatial Dependence Approach.” Papers in Regional Science 87 (4): 527–544. doi:10.1111/j.1435-5957.2008.00175.x.
- Berk, I., A. Kasman, and D. Kılınç. 2020. “Towards a Common Renewable Future: The System-GMM Approach to Assess the Convergence in Renewable Energy Consumption of EU Countries.” Energy Economics 87: 103922. doi:10.1016/j.eneco.2018.02.013.
- Blundell, R., and S. Bond. 1998. “Initial Conditions and Moment Restrictions in Dynamic Panel Data Models.” Journal of Econometrics 87 (1): 115–143. doi:10.1016/S0304-4076(98)00009-8.
- Castañeda Rodríguez, V. M. 2018. “Tax Determinants Revisited. An Unbalanced Data Panel Analysis.” Journal of Applied Economics 21 (1): 1–24. doi:10.1080/15140326.2018.1526867.
- Chen, H., K. Liu, T. Shi, and L. Wang. 2022. “Coal Consumption and Economic Growth: A Chinese City-Level Study.” Energy Economics 109: 105940. doi:10.1016/j.eneco.2022.105940.
- Chen, C., M. Pinar, and T. Stengos. 2020. “Renewable Energy Consumption and Economic Growth Nexus: Evidence from a Threshold Model.” Energy Policy 139: 111295. doi:10.1016/j.enpol.2020.111295.
- Chica-Olmo, J., S. Sari-Hassoun, and P. Moya-Fernández. 2020. “Spatial Relationship Between Economic Growth and Renewable Energy Consumption in 26 European Countries.” Energy Economics 92: 104962. doi:10.1016/j.eneco.2020.104962.
- Cho, S. H., Z. Chen, and N. C. Poudyal. 2010. “Spatial Structure of Agricultural Production in China.” Applied Economics 42 (16): 2031–2040. doi:10.1080/00036840701765320.
- Cui, L., S. Weng, and M. Song. 2022. “Financial Inclusion, Renewable Energy Consumption, and Inclusive Growth: Cross-Country Evidence.” Energy Efficiency 15 (6): 1–19. doi:10.1007/s12053-022-10051-y.
- Dash, D. P., A. K. Dash, and N. Sethi. 2022. “Designing Hydro-Energy Led Economic Growth for Pollution Abatement: Evidence from BRICS.” Environmental Science and Pollution Research 29 (21): 31252–31269. doi:10.1007/s11356-021-17890-9.
- Das, P., J. Mathur, R. Bhakar, and A. Kanudia. 2018. “Implications of Short-Term Renewable Energy Resource Intermittency in Long-Term Power System Planning.” Energy Strategy Reviews 22: 1–15. doi:10.1016/j.esr.2018.06.005.
- Davidson, R., and J. G. MacKinnon. 2004. Econometric Theory and Methods. New York: Oxford University Press.
- Dogan, E. 2016. “Analyzing the Linkage Between Renewable and Non-Renewable Energy Consumption and Economic Growth by Considering Structural Break in Time-Series Data.” Renewable Energy 99: 1126–1136. doi:10.1016/j.renene.2016.07.078.
- Emirmahmutoglu, F., Z. Denaux, T. Omay, and A. K. Tiwari. 2021. “Regime Dependent Causality Relationship Between Energy Consumption and GDP Growth: Evidence from OECD Countries.” Applied Economics 53 (19): 2230–2241. doi:10.1080/00036846.2020.1857330.
- Ertur, C., and W. Koch. 2007. “Growth, Technological Interdependence and Spatial Externalities: Theory and Evidence.” Journal of Applied Econometrics 22 (6): 1033–1062. doi:10.1002/jae.963.
- Ertur, C., and W. Koch. 2011. “A Contribution to the Theory and Empirics of Schumpeterian Growth with Worldwide Interactions.” Journal of Economic Growth 16 (3): 215–255. doi:10.1007/s10887-011-9067-0.
- Espoir, D. K., and R. Sunge. 2021. “Co2 Emissions and Economic Development in Africa: Evidence from a Dynamic Spatial Panel Model.” Journal of environmental management 300: 113617. doi:10.1016/j.jenvman.2021.113617.
- Fang, Z., and Y. Chen. 2017. “Human Capital and Energy in Economic Growth – Evidence from Chinese Provincial Data.” Energy Economics 68: 340–358. doi:10.1016/j.eneco.2017.10.007.
- Fotis, P., S. Karkalakos, and D. Asteriou. 2017. “The Relationship Between Energy Demand and Real GDP Growth Rate: The Role of Price Asymmetries and Spatial Externalities Within 34 Countries Across the Globe.” Energy Economics 66: 69–84. doi:10.1016/j.eneco.2017.05.027.
- Garsous, G., and S. Worack. 2022. “Technological Expertise as a Driver of Environmental Technology Diffusion Through Trade: Evidence from the Wind Turbine Manufacturing Industry.” Energy Policy 162: 112799. doi:10.1016/j.enpol.2022.112799.
- Grossman, G. M., and E. Helpman. 1991. “Trade, Knowledge Spillovers, and Growth.” European economic review 35 (2–3): 517–526. doi:10.1016/0014-2921(91)90153-A.
- Guivarch, C., and S. Monjon. 2017. “Identifying the Main Uncertainty Drivers of Energy Security in a Low-Carbon World: The Case of Europe.” Energy Economics 64: 530–541. doi:10.1016/j.eneco.2016.04.007.
- Gyeltshen, S. 2022. “Analysis of Bhutan’s Energy Policies in Relation to Energy Security and Climate Change: Policy Perspective.” Energy Policy 170: 113223. doi:10.1016/j.enpol.2022.113223.
- Hache, E. 2018. “Do Renewable Energies Improve Energy Security in the Long Run?” International Economics 156: 127–135. doi:10.1016/j.inteco.2018.01.005.
- Hache, E., and A. Palle. 2019. “Renewable Energy Source Integration into Power Networks, Research Trends and Policy Implications: A Bibliometric and Research Actors Survey Analysis.” Energy Policy 124: 23–35. doi:10.1016/j.enpol.2018.09.036.
- Hansen, S. T., and E. Moe. 2022. “Renewable Energy Expansion or the Preservation of National Energy Sovereignty? Norwegian Renewable Energy Policy Meets Resource Nationalism.” Political Geography 99: 102760. doi:10.1016/j.polgeo.2022.102760.
- Hao, Y., and H. Peng. 2017. “On the Convergence in China’s Provincial per Capita Energy Consumption: New Evidence from a Spatial Econometric Analysis.” Energy Economics 68: 31–43. doi:10.1016/j.eneco.2017.09.008.
- Harris, R. D. F., and E. Tzavalis. 1999. “Inference for Unit Roots in Dynamic Panels Where the Time Dimension is Fixed.” Journal of Econometrics 91 (2): 201–226. doi:10.1016/S0304-4076(98)00076-1.
- Helm, C., and M. Mier. 2021. “Steering the Energy Transition in a World of Intermittent Electricity Supply: Optimal Subsidies and Taxes for Renewables and Storage.” Journal of Environmental Economics and Management 109: 102497. doi:10.1016/j.jeem.2021.102497.
- IEA, 2022. World Energy Statistics. https://www.iea.org/data-and-statistics/data-product/world-energy-statistics
- Inglesi-Lotz, R. 2016. “The Impact of Renewable Energy Consumption to Economic Growth: A Panel Data Application.” Energy Economics 53: 58–63. doi:10.1016/j.eneco.2015.01.003.
- Ito, K. 2017. “CO2 Emissions, Renewable and Non-Renewable Energy Consumption, and Economic Growth: Evidence from Panel Data for Developing Countries.” International Economics 151: 1–6. doi:10.1016/j.inteco.2017.02.001.
- Jewell, J., A. Cherp, and K. Riahi. 2014. “Energy Security Under de-Carbonization Scenarios: An Assessment Framework and Evaluation Under Different Technology and Policy Choices.” Energy Policy 65: 743–760. doi:10.1016/j.enpol.2013.10.051.
- Jha, A. P., and S. K. Singh. 2020. “Does Diversity Matter? A Fresh Inquiry into the Energy, Economy and Environment Nexus.” Applied Economics 52 (12): 1349–1362. doi:10.1080/00036846.2019.1666205.
- Juodis, A., Y. Karavias, and V. Sarafidis. 2021. “A Homogeneous Approach to Testing for Granger Non-Causality in Heterogeneous Panels.” Empirical economics 60 (1): 93–112. doi:10.1007/s00181-020-01970-9.
- Kao, C. 1999. “Spurious Regression and Residual-Based Tests for Cointegration in Panel Data.” Journal of Econometrics 90 (1): 1–44. doi:10.1016/S0304-4076(98)00023-2.
- Karanfil, F. 2009. “How Many Times Again Will We Examine the Energy-Income Nexus Using a Limited Range of Traditional Econometric Tools?” Energy Policy 37 (4): 1191–1194. doi:10.1016/j.enpol.2008.11.029.
- Koçak, E., and A. Şarkgüneşi. 2017. “The Renewable Energy and Economic Growth Nexus in Black Sea and Balkan Countries.” Energy Policy 100: 51–57. doi:10.1016/j.enpol.2016.10.007.
- Law, S. H., T. C. Lim, and N. W. Ismail. 2013. “Institutions and Economic Development: A Granger Causality Analysis of Panel Data Evidence.” Economic Systems 37 (4): 610–624. doi:10.1016/j.ecosys.2013.05.005.
- Le, T. H., S. Boubaker, and C. P. Nguyen. 2021. “The Energy-Growth Nexus Revisited: An Analysis of Different Types of Energy.” Journal of environmental management 297: 113351. doi:10.1016/j.jenvman.2021.113351.
- Le, T. H., Y. Chang, and D. Park. 2020. “Renewable and Nonrenewable Energy Consumption, Economic Growth, and Emissions: International Evidence.” The Energy Journal 41 (2). doi:10.5547/01956574.41.2.thle.
- Leiva, M., F. Vasquez-Lavín, and R. D. P. Oliva. 2020. “Do Immigrants Increase Crime? Spatial Analysis in a Middle-Income Country.” World Development 126: 104728. doi:10.1016/j.worlddev.2019.104728.
- LeSage, J. P., and R. K. Pace. 2009. Introduction to Spatial Econometrics. Boca Raton: CRC Press.
- Levin, A., C. -F. Lin, and C. -S. Chu. 2002. “Unit Root Tests in Panel Data: Asymptotic and Finite-Sample Properties.” Journal of Econometrics 108 (1): 1–24. doi:10.1016/S0304-4076(01)00098-7.
- Liebensteiner, M., and M. Wrienz. 2020. “Do Intermittent Renewables Threaten the Electricity Supply Security?” Energy Economics 87: 104499. doi:10.1016/j.eneco.2019.104499.
- Li, M., N. M. Hamawandy, F. Wahid, H. Rjoub, and Z. Bao. 2021. “Renewable Energy Resources Investment and Green Finance: Evidence from China.” Resources Policy 74: 102402. doi:10.1016/j.resourpol.2021.102402.
- Loisel, R., L. Lemiale, S. Mima, and A. Bidaud. 2022. “Strategies for Short-Term Intermittency in Long-Term Prospective Scenarios in the French Power System.” Energy Policy 169: 113182. doi:10.1016/j.enpol.2022.113182.
- Long, X., E. Y. Naminse, J. Du, and J. Zhuang. 2015. “Nonrenewable Energy, Renewable Energy, Carbon Dioxide Emissions and Economic Growth in China from 1952 to 2012.” Renewable and Sustainable Energy Reviews 52: 680–688. doi:10.1016/j.rser.2015.07.176.
- Marbuah, G., and F. Amuakwa-Mensah. 2017. “Spatial Analysis of Emissions in Sweden.” Energy Economics 68: 383–394. doi:10.1016/j.eneco.2017.10.003.
- Marques, A. C., and J. A. Fuinhas. 2012. “Is Renewable Energy Effective in Promoting Growth?” Energy Policy 46: 434–442. doi:10.1016/j.enpol.2012.04.006.
- Maruta, A. A., R. Banerjee, and T. Cavoli. 2020. “Foreign Aid, Institutional Quality and Economic Growth: Evidence from the Developing World.” Economic modelling 89: 444–463. doi:10.1016/j.econmod.2019.11.008.
- Menegaki, A. N. 2011. “Growth and Renewable Energy in Europe: A Random Effect Model with Evidence for Neutrality Hypothesis.” Energy Economics 33 (2): 257–263. doi:10.1016/j.eneco.2010.10.004.
- Nan, S., Y. Huo, W. You, and Y. Guo. 2022. “Globalization Spatial Spillover Effects and Carbon Emissions: What is the Role of Economic Complexity?” Energy Economics 112: 106184. doi:10.1016/j.eneco.2022.106184.
- Nawaz, S., N. Iqbal, and M. A. Khan. 2014. “The Impact of Institutional Quality on Economic Growth: Panel Evidence.” Pakistan development review 53 (1): 15–31. doi:10.30541/v53i1pp.15-31.
- Nkoa, B. E. O., and J. S. Song. 2020. “Does Institutional Quality Affect Financial Inclusion in Africa? A Panel Data Analysis.” Economic Systems 44 (4): 100836. doi:10.1016/j.ecosys.2020.100836.
- Noseleit, F. 2018. “Renewable Energy Innovations and Sustainability Transition: How Relevant are Spatial Spillovers?” Journal of Regional Science 58 (1): 259–275. doi:10.1111/jors.12340.
- Nweke, G. O., S. I. Odo, and C. I. Anoke. 2017. “Effect of Capital Formation on Economic Growth in Nigeria.” Asian Journal of Economics, Business and Accounting 5 (1): 1–16. doi:10.9734/AJEBA/2017/36075.
- Ogunniyi, A. I., G. Mavrotas, K. O. Olagunju, O. Fadare, and R. Adedoyin. 2020. “Governance Quality, Remittances and Their Implications for Food and Nutrition Security in Sub-Saharan Africa.” World Development 127: 104752. doi:10.1016/j.worlddev.2019.104752.
- Ohler, A., and I. Fetters. 2014. “The Causal Relationship Between Renewable Electricity Generation and GDP Growth: A Study of Energy Sources.” Energy Economics 43: 125–139. doi:10.1016/j.eneco.2014.02.009.
- Ozturk, I. 2010. “A Literature Survey on Energy–Growth Nexus.” Energy Policy 38 (1): 340–349. doi:10.1016/j.enpol.2009.09.024.
- Pesaran, M. H., 2004. General Diagnostic Tests for Cross Section Dependence in Panels. University of Cambridge, Faculty of Economics, Cambridge Working Papers in Economics No. 0435.
- Pesaran, H. M. 2007. “A Simple Panel Unit Root Test in the Presence of Cross-Section Dependence.” Journal of Applied Econometrics 22 (2): 265–312. doi:10.1002/jae.951.
- Polat, B. 2021. “The Impact of Renewable and Nonrenewable Energy Consumption on Economic Growth: A Dynamic Panel Data Approach.” Asia-Pacific Journal of Accounting & Economics 28 (5): 592–603. doi:10.1080/16081625.2018.1540936.
- Radmehr, R., S. R. Henneberry, and S. Shayanmehr. 2021. “Renewable Energy Consumption, CO2 Emissions, and Economic Growth Nexus: A Simultaneity Spatial Modeling Analysis of EU Countries.” Structural Change and Economic Dynamics 57: 13–27. doi:10.1016/j.strueco.2021.01.006.
- Radulescu, D., and P. Sulger. 2022. “Interdependencies Between Countries in the Provision of Energy.” Energy Economics 107: 105799. doi:10.1016/j.eneco.2021.105799.
- REN21, 2016. Renewables Global Status Report Paris: REN21 Secretariate.
- Roodman, D. 2009. “How to Do Xtabond2: An Introduction to Difference and System GMM in Stata.” The Stata Journal 9 (1): 86–136. doi:10.1177/1536867X0900900106.
- Sanso-Navarro, M., M. Vera-Cabello, and M. Puente-Ajovín. 2020. “Regional Convergence and Spatial Dependence: A Worldwide Perspective.” The Annals of Regional Science 65 (1): 147–177. doi:10.1007/s00168-020-00978-4.
- Scholten, D., M. Bazilian, I. Overland, and K. Westphal. 2020. “The Geopolitics of Renewables: New Board, New Game.” Energy Policy 138: 111059. doi:10.1016/j.enpol.2019.111059.
- Siddiqui, A., and A. U. Rehman. 2017. “The Human Capital and Economic Growth Nexus: In East and South Asia.” Applied Economics 49 (28): 2697–2710. doi:10.1080/00036846.2016.1245841.
- Solarin, S. A., and I. Ozturk. 2015. “On the Causal Dynamics Between Hydroelectricity Consumption and Economic Growth in Latin America Countries.” Renewable and Sustainable Energy Reviews 52: 1857–1868. doi:10.1016/j.rser.2015.08.003.
- Thaler, P., and B. Hofmann. 2022. “The Impossible Energy Trinity: Energy Security, Sustainability, and Sovereignty in Cross-Border Electricity Systems.” Political Geography 94: 102579. doi:10.1016/j.polgeo.2021.102579.
- Topcu, E., B. Altinoz, and A. Aslan. 2020. “Global Evidence from the Link Between Economic Growth, Natural Resources, Energy Consumption, and Gross Capital Formation.” Resources Policy 66: 101622. doi:10.1016/j.resourpol.2020.101622.
- Trotta, G., A. R. Hansen, and S. Sommer. 2022. “The Price Elasticity of Residential District Heating Demand: New Evidence from a Dynamic Panel Approach.” Energy Economics 112: 106163. doi:10.1016/j.eneco.2022.106163.
- Tugcu, C. T., I. Ozturk, and A. Aslan. 2012. “Renewable and Non-Renewable Energy Consumption and Economic Growth Relationship Revisited: Evidence from G7 Countries.” Energy Economics 34 (6): 1942–1950. doi:10.1016/j.eneco.2012.08.021.
- Udi, J., F. V. Bekun, and F. F. Adedoyin. 2020. “Modeling the Nexus Between Coal Consumption, FDI Inflow and Economic Expansion: Does Industrialization Matter in South Africa?” Environmental Science and Pollution Research 27 (10): 10553–10564. doi:10.1007/s11356-020-07691-x.
- Ummalla, M., and A. Samal. 2018. “The Impact of Hydropower Energy Consumption on Economic Growth and CO2 Emissions in China.” Environmental Science and Pollution Research 25 (35): 35725–35737. doi:10.1007/s11356-018-3525-6.
- Wang, M., M. C. S. Wong, and J. Granato. 2015. “International Comovement of Economic Fluctuations: A Spatial Analysis.” World Development 67: 186–201. doi:10.1016/j.worlddev.2014.10.016.
- Xia, F., and F. Song. 2017. “Evaluating the Economic Impact of Wind Power Development on Local Economies in China.” Energy Policy 110: 263–270. doi:10.1016/j.enpol.2017.08.030.
- Yao, Y., K. Ivanovski, J. Inekwe, and R. Smyth. 2019. “Human Capital and Energy Consumption: Evidence from OECD Countries.” Energy Economics 84: 104534. doi:10.1016/j.eneco.2019.104534.
- You, W., and Z. Lv. 2018. “Spillover Effects of Economic Globalization on CO2 Emissions: A Spatial Panel Approach.” Energy Economics 73: 248–257. doi:10.1016/j.eneco.2018.05.016.
- Young, A. 1991. “Learning by Doing and the Dynamic Effects of International Trade.” The Quarterly Journal of Economics 106 (2): 369–405. doi:10.2307/2937942.
- Zallé, O. 2019. “Natural Resources and Economic Growth in Africa: The Role of Institutional Quality and Human Capital.” Resources Policy 62: 616–624. doi:10.1016/j.resourpol.2018.11.009.
- Zhang, H., and H. Kim. 2022. “Institutional Quality and FDI Location: A Threshold Model.” Economic modelling 114: 105942. doi:10.1016/j.econmod.2022.105942.
- Zheng, X., F. Li, S. Song, and Y. Yu. 2013. “Central Government’s Infrastructure Investment Across Chinese Regions: A Dynamic Spatial Panel Data Approach.” China Economic Review 27: 264–276. doi:10.1016/j.chieco.2012.12.006.
Appendix
Table A1. Juodis, Karavias and Sarafidis (2021) panel causality test.
Table A2. Dynamic SYS-GMM results using queen contiguity matrix – robustness checks.