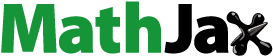
ABSTRACT
It is often suggested by fans, the media, and football commentators that English players are over-valued and receive higher salaries than those of comparable players from different countries. This study examines whether the suggestion can be empirically substantiated. Using a unique database covering all of the European elite leagues, we show that there is an English player value premium of around 40% and a wage premium of 25%. Exploring the reasons for this phenomenon in a regression setting, we find that the excess valuation and salary differential can be partly justified by several factors. First, and most importantly, there is a higher value attributed and wages paid to players in the English Premier League (EPL); second, that English players are more likely to play as strikers than in other positions in EPL clubs; third, that their performance in some positions is somewhat better than the average of players from other nations; fourth, that there are fewer of them in the top European leagues, leading to a shortage in supply. There is, however, evidence that the higher valuation of English attackers and the higher salaries of English attackers and of English midfielders evades explanation by any of these groups of variables.
I. Introduction
Football is a global game, not only in terms of its viewership, with UEFA claiming that Euro 2020 (played in 2021) was one of the most watched moments of all time with a 5.2 billion cumulative live audience,Footnote1 but also from the perspective of players travelling far and wide to sign for clubs in other parts of the world (Magee and Sugden Citation2002). Europe is the most popular destination for emigrating footballers (Poli, Ravenel, and Besson Citation2016), followed by North American (Major League Soccer), with 500 from AfricaFootnote2 and 781 from Brazil alone.Footnote3
According to Baur and Lehmann and Schulze (Citation2008), poorer countries with strong footballing traditions such as Argentina and Brazil tend to be net exporters of players, while richer countries with high-paying leagues are net importers (in particular, China, Western Europe, and the US). Gerhards and Mutz (Citation2017) state that the proportion of foreign nationals playing in the top European leagues increased from 20% in 1995 to 50% in 2015, while our own figures (see below) suggest that this had risen slightly further still to 51% by 2018.
The commercial side of football, such as TV rights, ticket prices, and especially player salaries and transfer values, generates almost as much discussion among fans as the game itself. Wealthy overseas buyers have pumped money into EPL football clubs, beginning with Roman Abramovich’s 2003 purchase of Chelsea Football Club (see Rohde and Breuer (Citation2017) for a review of the developments in football club financing and ownership in the major European leagues). This source of wealth, combined with ballooning TV rights, gate receipts and merchandise sales, have all generated increasing revenues that can be spent on ‘investing in the team’.
Team investment involves purchasing highly valued players who are tradeable assets to be bought and sold (Kesenne Citation2007) from one club to another for increasingly high prices at the top end (Burdekin and Franklin Citation2015). Transfers from one club to another are a vast and growing business (Metelski Citation2021), with the total global player transfer value exceeding ten billion euro in 2019 (Poli, Ravenel, and Besson Citation2020). A recent study by Neri et al. (Citation2021) showed that Italian clubs derive significant net income from their player trades, which also allow the clubs to smooth their reported profits.
What factors affect the prices at which football players will be sold and the salaries they receive? Are their potential talent and past performance the only relevant variables, or are there others? For instance, since players will be focused on their post-tax remuneration, it is possible that differential tax rates might drive country differences in player gross wages.
There has been speculation in the media and among commentators that player nationality also plays a role. Although in their book Kuper and Szymanski (Citation2018) explicitly refer to Dutch, Brazilian and Belgian rather than English players as being overvalued, there are vast numbers of media stories and newspaper articles suggesting that English players in the EPL are priced higher in relation to their talent.Footnote4
If it is indeed an artefact of the data and not just folklore, why might this valuation disparity have arisen? There are at least two commonly cited explanations. First, that EPL clubs do not like transferring their talent to rivals, they would thus require a premium to do so.Footnote5 This argument would suggest that English players transferring from one English club to another would trade for more than comparable stars arriving from an overseas team. Second, the EPL has revenues, at £5.1 billion, that are almost double those of their nearest rival league, the Bundesliga, at £3.1 billion for the season 2019/20 (Deloitte Citation2021), and therefore English players benefit from a greater availability of funds by simply being predominantly based in that country.
Yet, neither of these explanations appear to hold up to close scrutiny. First, the distaste for selling a valued player to a local rival, if it exists, would surely be universal, and should result in higher international movement and a ‘local transfer premium’ from all countries, not just of English players. Regarding the second explanation, we might expect that all players in the EPL would be valued higher as a result of the league’s greater wealth, irrespective of their country of origin, and we empirically test this.
Another potential explanation is that EPL teams are willing to pay more for home players as part of building the former’s brand identity and in line with their ‘home-grown’ narrative. Cox, Hills, and Kennedy (Citation2015), for instance, show that the news media in England employ a nationalistic discourse and a ‘symbolic Englishness’ around Champions League matches featuring English teams. While local supporters might appreciate their team promoting domestic talent, in the current international market for football fans, if Manchester United have more fans in Beijing than Manchester, this would also appear to be a doubtful driver. In addition, Wilson and Ying (Citation2003) found scant support for the suggestion that fans have a preference for players from the team’s country, leading them to conclude that under- or over-representation of particular nationalities arises from the club owner’s or manager’s choices.
A further possibility, yet one which is given scant consideration in the media, is that English players are not actually over-valued. After all, the sums spent on elite academies at the leading clubs mean that talent is spotted and developed from an early school age by high quality coaching staff and facilities. EPL teams spend an average of 6.1 million euro per club, the highest sum in Europe, on their academies.Footnote6 This enables EPL clubs to have more confidence in their assessment of academy players, most of whom are English, since the team’s staff will have had the opportunity to observe them playing for a prolonged period, and thus other EPL clubs would be willing to pay more for them than they would for juniors from overseas academies who are less well known to them and therefore riskier propositions.
The present study addresses this issue, and aims to explain the cross-sectional variation in association football player valuations and wages with a particular focus on whether there is an English player premium and if so, what are its drivers. Uniquely, by utilizing the entire database that lies behind the popular video game Football Manager combined with valuations from Transfermarkt, we are able to use a far wider range of performance measures and other player characteristics than were available to previous studies.Footnote7
We examine the five elite leagues of European football: the English Premier League, League 1 in France, the Bundesliga in Germany, La Liga in Spain and Serie A in Italy for the 2018–19 season. The use of this point in time has the advantage of occurring prior to the COVID-19 pandemic that caused the closure of stadia around the globe, and which resulted in significant losses for football clubs at all levels and interrupted the usual market for the movement of football players.
Our study involves several innovations building on existing research in this area. First, we are able to employ a larger number of data points than available in many existing studies since we cover all elite players in the top five European leagues rather than a single country. The top leagues in these nations taken for a single footballing year comprise a relatively homogeneous sample, and there is considerable transfer activity between them. We obtain valuations for all players (over 2,000) in all five leagues. Second, we emphasize a player’s nationality and the leagues that they play in, which turn out to be crucial factors in influencing player valuations and wages. Third, we have more detailed, specialist information on player performance than was available to previous researchers as well as other characteristics. To the best of our knowledge, we are the first to use the Football Manager database in the context of player valuations.Footnote8 Fourth, our parallel examination of wage and valuation data from different sources but yielding ostensibly similar findings adds strength to the results. In summary, we find that the English player value and salary premia can partly be explained by these factors, and most noticeably that the English premium is partly an EPL premium, although it is also related to player skill.
The remainder of this paper develops as follows. Section II discusses the existing literature on the determinants of football player valuations and wage rates, and Section III outlines UEFA’s Financial Fair Play rule and its possible influences on the dynamics of the player market. Section IV discusses our sources of data and the methods that we use to analyse them, including detailed variable motivations and descriptions. Finally, Section V presents the results and Section VI provides concluding remarks and draws the paper to a close.
II. Existing research on football player values and salaries
Given the public fascination with player values and the importance of transfer prices in determining a club’s financial position, there has been surprisingly little research on the factors that affect valuations. A crucial reason behind this is probably because valuation data was previously unavailable as it cannot be directly observed, and instead papers have focused on transfer fees. As we document below in section IV, this is undesirable for several reasons and limits the scale and scope of research that can be undertaken.
Among existing studies, Frick (Citation2007, ) presents a valuable summary of the studies that modelled the determinants of transfer fees up to the point that study was written. The majority of early studies were focused on the Bundesliga (e.g. Feess, Frick, and Muehlheusser Citation2004) or the EPL (e.g. Dobson and Gerrard Citation1999), primarily due to a lack of data availability on player salaries from other leagues until that time. Although most of the latest papers have continued to use data from only one country, some multi-league studies have emerged (e.g. Behravan and Razavi Citation2021; Felipe et al. Citation2020).
Table 1. Player and valuation summary statistics.
Table 2. Numbers and percentages of players of each nationality in the top five European leagues.
Table 3. Average player performance statistics by nationality.
As might be anticipated, studies seeking to explain cross-sectional variations in player values are predominantly focused on performance. For instance, using data from La Liga for half of the 2014–15 season, He, Cachucho, and Knobbe (Citation2015) focus on the link between forward players’ performance, using data generated by Opta but sourced from WhoScored, and their market value, obtained from TransferMarkt. They find that players committing a lower number of fouls, shots and goals, assists, and accurate dribbling, are the key success measures.
Singh and Lamba (Citation2019) also use crowd-sourced measures of performance, but from the FIFA18 video game, finding that it adds significantly to player valuation predictive accuracy. Behravan and Razavi (Citation2021) similarly use FIFA game data (the 2020 updated version) as a means to estimate the market values of a very large sample of almost 20,000 players using a combination of particle swarm optimization and support vector machines.
In a recent paper, Depken and Globan (Citation2021) present evidence of a significant ‘English premium’, although this relates to players of any nationality moving to English clubs rather than English players per se, which is the focus of our study. They argue that this premium arose directly from the TV broadcasting deals that the Premier League signed from 2012 that provided more revenue than was available to other leagues.
A further study, by Ante (Citation2019), employs data on 389 transfers of outfield players into the five leading European leagues, confirming the relevance of previously established variables in determining transfer fees, including the player’s age and height, as well as the number of minutes they were on the pitch in the prior season. Interestingly, Ante also finds that players who commit more fouls elicit higher transfer values. Key to that study, he demonstrates the negotiating power of external advisors in nudging up the prices of the more expensive players.
Using a somewhat different approach, Müller, Simons, and Weinmann (Citation2017) construct a multi-level dynamic panel regression with random effects to predict player market values. The empirically most relevant explanatory variables are the (log of the) previous market value, the number of minutes on the pitch, age-squared, position-specific performance calculations, and media profile measures. They find that the accuracy of a model-based approach to determining actual transfer prices is close to that of the crowd-sourced estimates.
There has also been a solid parallel stream of research on player salaries, and one would presume that a comparable set of variables would be relevant for valuations as well and we can therefore draw upon the salaries literature in determining relevant explanatory variables. For instance, performance and skill are important determinants of player salaries (Fabrizio Montanari, Silvestri, and Bof Citation2008) and playing for the national team as well as superior performance were positive predictors of wage differentials for Italian football players according to Lucifora and Simmons (Citation2003). However, wages are likely to be influenced more by past performance rather than future potential compared with player valuations (Gerhards and Mutz Citation2017, 226).
A useful summary of studies on the determinants of player salaries is also provided in Frick (Citation2007, ). Relevant explanatory variables for determining player wages (with signs on estimated parameters in parentheses) in Frick’s summary were: age (+), age-squared (-), international caps (±), non-European player (-), South American (+), games played in season (+), out-field player (+), goals scored (+), tackles (+), assists (+), contract length (+). Torgler and Schmidt (Citation2007) observe a non-linear relationship between player pay and their individual performance using an eight-year panel from the Bundesliga.
III. Financial fair play and the home-grown rule
As we argue in this section, European-level requirements relating to the nationality composition of teams could cause distortions in the market for football players. Specifically, Financial Fair Play (FFP) is a set of rules introduced by the Union of European Football Associations (UEFA) in 2011. Despite the substantial increases in incomes at elite football clubs since the turn of the millennium that were discussed above, clubs at all levels were finding themselves in an increasingly perilous financial position, with costs rising even faster than revenues. This led to growing deficits that UEFA believed to be unsustainable – see, for example, UEFA (Citation2018) for the organization’s recent evaluation of football finances.
Most of the discussion – both in the scholarly literature and in the media – has focused upon the aspects of FFP that are concerned with the way clubs are managed, embodying the requirement that, broadly, revenues and costs must balance over the medium-term. But there is also a slightly lesser-known UEFA rule relating to the number of ‘home-grown players’ (HGP) that a team must have on its roster. This rule requires that each squad must contain no more than 17 players who do not fulfil the HGP criterion. The rest of the squad, up to a total of 25 players, must be ‘home-grown’.Footnote9 In a further technicality, players aged under-21 can be included in addition to the limit of 25 players per squad. A ‘home-grown player means a player who, irrespective of nationality or age, has been registered with any club affiliated to The Football Association or the Football Association of Wales for a period, continuous or not, of three entire seasons, or 36 months, before his 21st birthday (or the end of the season during which he turns 21)’.Footnote10
The rule was introduced in 2006 and has been in force since then. Although it was presumably not UEFA’s intention, a side-effect of the HGP rule has been to increase demand for English players and overseas players who have been ‘developed’ in English Academies. As we demonstrate below, England is by far a net importer of players. While it does not map directly onto nationality, the HGP rule effectively limits the ability of EPL clubs to bring in foreign nationals with proven track records, who are willing to reside in England in large numbers. This means that, to satisfy the rule, EPL clubs need to have at least eight locally developed players. Other European countries are arguably less affected by the HGP rule since fewer players of other nationalities go there as their squads are considerably less international. The number of HGPs has risen since the rule was introduced (Dalziel et al. Citation2013), and although this trend could have occurred in the absence of the requirement, it seems unlikely in an increasingly globalized game.
Although it would not be possible to test its effects directly, our study provides an opportunity to examine one of the primary implications of the HGP rule – namely its effect on the values and wages of players where there are imbalances between supply and demand leading to a shortage of HGPs in some markets.
IV. Methods and data
Empirical modelling approach
We follow the majority of existing research in this area by employing OLS regressions as the main modelling approach. Some prior studies (e.g. Carmichael, Forrest, and Simmons Citation1999; Feess, Frick, and Muehlheusser Citation2004) have employed the Heckman two-step process to allow for possible selection bias since only a subset of players are actually transferred during the researchers’ sample periods. However, this is not necessary in our case since we focus on valuations (and salaries) rather than transfers and as such, we are able to include all players whether they moved club or not and hence there is no potential for selection effects.Footnote11
Data
We construct a database by obtaining information from Football Manager, stored in Microsoft Access format, by pulling information for players and clubs and we queried the latter so that each player could be assigned to their appropriate league. We removed all non-player staff and players not from the five main leagues from the query. We then web-scraped the value of every player from the five main leagues on Transfermarkt and the wage data from Capology. Transfermarkt is a now well-known website that provides a source of information on football news, blogs, transfer activity and (importantly for our study) transfer values estimated using an approach we describe below;Footnote12 Capology is a website that provides football player wages and other financial information.Footnote13 We used the fuzzy lookup package in Excel to match every player in the main file to their value from Transfermarkt and wage from Capology, removing any players with no value or wage attached. A fuzzy match is required due to frequent minor differences in the spellings of names across the two platforms or in whether middle initials and the like are included in the name field.
For direct comparability, all player values and salaries are measured in British pounds, irrespective of their nationality or league. We include not only regular first team members, but all players on the roster, leading to a total of 2,730 observations. Both the Transfermarkt and Football Manager data are taken for the end of the 2017–18 season in May 2018, and the exchange rates that prevailed at that time were used to convert the values into pounds.
Determining player values
There are several possible methods for determining appropriate valuations for football players:
Examining the actual traded prices when players are sold by one club to another. Unfortunately, while the most intuitively appealing approach since it is based on genuine market prices rather than hypothetical estimates, this approach is a non-starter due to several issues:
The proportion of players who move from one club to another in a given season is relatively small, implying that to enable the number of data points to be sufficiently large would require covering multiple years or the inclusion of lower leagues, thereby reducing the homogeneity of the sample. Observed football player transfers suffer from a selectivity bias, although it is possible to mitigate this (see Ruijg and van Ophem Citation2015).
If players leave at the end of the contract with their current club, they will move to their next one at zero transfer fee – this is known as the ‘Bosman ruling’, although the player may receive a substantial signing-on bonus from their new club (Frick Citation2007, 431).
Player fees often involve contingent charges that make assessing the true transfer price tricky. Sometimes, a deal involves cash plus player X as a ‘makeweight’ in return for player Y.
Transfer prices often vary as a function of the financial position of the selling and purchasing clubs rather than being purely based on the quality of the player (see Poli, Ravenel, and Besson Citation2020).
Estimating values using a statistical model. Here, each player is viewed as a ‘bundle of characteristics’, including physical characteristics (e.g. age, height, weight), performance outcomes (e.g. successful passes, goals scored, minutes played) and profile or marketability measures (e.g. Google searches of the player’s name, number of YouTube video watches, media appearances). This approach has been used to good effect by Müller, Simons, and Weinmann (Citation2017), but in our case it would not be appropriate to use such a set of variables to generate player valuations and then to use the same variables for determining the factors that explain why one player is worth more than another. In essence, this would imply using a single set of measures to construct a dependent variable and to explain it.
Using ‘crowd-sourced’ estimates based on an aggregation of fans’ opinions. Among these three approaches, the third appears on the surface to be the most tenuous but has received considerable empirical support as providing appropriate valuations. Herm, Callsen-Bracker, and Kreis (Citation2014) calculate the correlation between such estimated values and actual transfer prices to be over 0.9. Leading Müller, Simons, and Weinmann (Citation2017) to state that, ‘Transfermarkt’s accuracy in estimating market value is remarkable’ (p.612). An advantage of this approach is that the valuations are, to a large extent, independent of the player’s current contractual position and the relative negotiating strength of the two parties in the context of a transfer, allowing us to focus on the intrinsic characteristics of the footballer. A further benefit is that such valuations are available for a wide range of players in different leagues irrespective of whether they have recently moved between clubs or not, or whether they are relatively young, inexperienced players or have many years of observable performance.
In the present study, we therefore adopt this third approach to estimating player prices. Transfermarkt, the source of our player valuations, was established in 2001 and now provides a comprehensive website displaying football player transfer news, player valuations and other information for all the major leagues in Europe. Considerable detail on how the valuations are determined is presented in Herm, Callsen-Bracker, and Kreis (Citation2014) and Müller, Simons, and Weinmann (Citation2017), but in summary Transfermarkt uses ‘crowdsourcing’ or ‘citizen science’, where a self-registered panel of people provide their own valuations and upload them onto the website. Those providing valuations can use any method they wish, although Transfermarkt provides a list of relevant factors (and further details on the methods it uses) that crowd valuers can use on its website.Footnote14 The organization then weights the individual valuations to create an overall number for each player, assigning greater influence to those it seems to be more knowledgeable. It is worth noting that while Transfermarkt is comprehensive and has received some support in the literature, recent research has also argued that despite being significantly correlated with actual transfer prices, the crowd-sourced values are downwardly biased estimates (Coates and Parshakov Citation2022).
presents the average player values (in millions of British pounds) and salaries (in thousands of pounds per week), for all nationalities and separated by English versus non-English players and by field position. Along with other summary measures. From these univariate measures in Panel A, it is evident that English players are both higher valued and better paid than those of other European nations and than the average of all other countries. The average age of English players is within the range of the averages for other countries, although the former are slightly heavier (i.e. they have a larger BMI).
Panels B and C of present player valuations and wages respectively, each separated by nationality and by field position. It is clear that averaging across our entire database, the further forward a player is positioned, the higher their valuations and salaries. The final two columns in Panels B and C provide -test statistics for the null hypothesis of no difference between the values and wages respectively of English and non-English players and their associated
-values. Statistically, there is no significant difference between the average values of English and non-English goalkeepers or mid-fielders, but English defenders are worth on average of almost £5 million (70%) more than their non-English counterparts, while English attackers are worth just over £4 million (64%) more. The ultimate row shows that, across all positions taken together, English players are valued at 138% of their non-English counterparts, a difference that is significant at the 1% level. This provides prima facie evidence that indeed the English player premium exists and is considerable. The wage information in Panel C reveals that English players are paid significantly more in defending and mid-field positions and averaged across all positions.
Explanatory variables
We are able to examine the factors affecting player valuation by utilizing a unique set of data collected by the video game, Football Manager. To construct the database behind the video game, the company makes use of ratings of players each time they are in a match by over 1,000 local researchers, who each provide scores for the players under various headings based on their individual contribution to the real-life football games that they have watched.Footnote15 Football Manager then aggregates all of the scores from individual researchers, and provides overall figures for players on each of 14 technical, 14 mental and 8 physical attributes, measured 1–20 scale. The ratings on Football Manager are already used by EPL clubs in their own scouting activities via the application Prozone recruiter.Footnote16 Football Manager includes not only comprehensive data on player performance, but also on a wealth of other footballer characteristics including information on age, height, weight, player ambition, international appearances and goals, and behavioural traits. We now list and define all of the explanatory variables we employ in the model, motivated by support from the existing literature.
Nationality
In addition to the pressures created by FFP for clubs to ensure that they have a certain proportion of players from their own country, there is evidence that, even after allowing for experience and performance, nationality is a significant predictor of transfer price. For instance, the results in Feess, Frick, and Muehlheusser (Citation2007) show that citizens of other countries command significantly higher transfer fees in the German league than those from the home nation. Ante (Citation2019) suggests that the premium valuations applied to some nationalities might be league-specific and dependent upon the linguistic and cultural ‘fit’ of the player in that league – for instance, those from Latin American countries may integrate more effortlessly into la Liga than other European leagues due to their shared languages.
League
As we have noted, the EPL has almost twice the revenue of its nearest European rival league and both Ante (Citation2019) and Felipe et al. (Citation2020) find that playing in the EPL is a significant predictor of higher player value.
Position
Players’ preferred positions can be separated naturally into four categories: strikers/forwards, midfielders, defenders and goalkeepers. While quality should be valued in all positions, the literature suggests that, all else equal, strikers tend to garner higher valuations and salaries because they have a higher profile in the team and get more attention from fans, giving them more publicity (see Frick Citation2007).
Age
Age has been shown in several previous studies to have a non-linear (n-shaped) relationship with player value, which studies typically tend to model using age and age-squared as explanatory variables to capture the trade-off between experience versus potential (see, for example, Dobson, Gerrard, and Howe Citation2000 or Lehmann & Schultze, Lehmann and Schulze Citation2008). When footballers amass experience and a track record from their teenage years through their 20s while still having the prospect of many good years ahead of them (barring a career-ending injury), their value tends to grow. But as players advance past 30, their transfer price tends to decline along with their expected number of future playing years, although their fame and marketability might continue to ride high.
Other player physical characteristics
Players who can handle the ball equally well with both feet tend to be more highly valued than those who are either only left-footed or only right-footed (Bryson, Frick, and Simmons Citation2013).
A player’s height and weight can also influence their on-field ability (see Bryson, Frick, and Simmons Citation2013), dependent upon the position in which play. For instance, being tall is useful for goalkeepers and for out-field players heading the ball, while strikers often have a low body mass index (BMI), making them fast and defenders tend to be bigger-built. We employ BMI in the regression models, measured as weight in kilogrammes divided by height in metres squared.
International appearances
Performance in international matches can also influence a player’s value. For instance, Kiefer (Citation2012) reports that Thomas Müller’s value more than doubled after his highly successful world cup appearance in 2010. While there are no international matches taking place during the season covered by our data, it immediately followed the 2018 world cup that took place in Russia and was won by France. We include the number of international appearances as a potential explanatory variable in the regression models.
Performance indicators
Intuitively, transfer prices and estimated valuations have been found to be strongly correlated with recent performance judged using measures that are relevant for the player’s position on the field (e.g. Herm, Callsen-Bracker, and Kreis Citation2014). We include a range of position-specific performance variables in the core regressions. These variables and their relevance are largely self-explanatory:
For goalkeepers: aerial ability; handling; kicking; one-on-ones; reflexes; rushing out.
For attackers: ambition, controversy, acceleration, agility, anticipation, balance, composure, concentration, consistency, crossing, decisions, finishing, first touch, free kicks, important matches, jumping, left foot, marking, pace, passing, right foot, stamina, strength, technique, work rate.
For midfielders: adaptability, controversy, potential ability, acceleration, agility, anticipation, balance, composure, concentration, consistency, crossing, finishing, first touch, left foot, natural fitness, pace, penalties, right foot, stamina, strength, tackling, vision, work rate.
For defenders: determination, professionalism, temperament, potential ability, acceleration, agility, bravery, decisions, finishing, first touch, flair, free kicks, injury proneness, jumping, leadership, left foot, long shots, marking, movement, pace, positioning, right foot, stamina, strength, tackling, teamwork.
V. Results
In this section, the results are presented, initially focused on summarizing the roles of individual variables before moving onto the regression framework.
Player numbers: supply and demand
The EPL, League 1, La Liga and Serie A all comprise 20 teams, while the Bundesliga has 18, and each squad averages 28 players. Therefore, the demand for players is roughly homogeneous across the five leagues, and consequently, from this perspective the implications of the FFP rule regarding home-grown players should have comparable impacts in each country. However, there could be differential impacts of the HGP rule from the supply side (the number of players available for each nation’s home teams).
presents the number and percentage of players of each nationality (English, French, German, Italian, Spanish and Others) playing in each of the European leagues. The table shows two fascinating features. First, of the five European nationalities, England has by far the fewest players in these top divisions, comprising only 216 out of 1,547 (13%) of the total. Second, the English extremely rarely play overseas, with only six out of 216 (3%) playing abroad. In the 2018–19 season, just one played in Italy and not a single one in Spain. This is in contrast to the French, who, of the five nations, are the most mobile with 102 of 407 (25%) playing in another country. Panel B of shows that the EPL has by far the highest percentage of non-local players with only a third from the home nation. It also has over 50% hailing from outside the five European countries, which is the highest percentage. The French and Spanish leagues both have around 55% home nation players with very few drawn from the other European countries.
League effects
It is widely known that the English Premier League (EPL) has the largest revenues of any soccer league in the World on many measures. For example in the 2019/20 season, the EPL generated 52% of its revenues from broadcasts – some £2.6 Billion, as well as £1.7 Billion from Sponsorship and Commercial income. Match day receipts made up just 13% of the income at £683 million. All these income streams are almost double those that their nearest rival, the Bundesliga, managed to achieve for the same reporting period (Deloitte Citation2021). As a result, it is perhaps not surprising that those who play in the EPL are more highly valued and better paid than those in other leagues. It is also the case, nonetheless, that the most common nationality among players in the EPL is English, as shows. Reading across the first row, just over a third of EPL players are English, with 5% from each of France and Spain, 2% from Germany, 1% from Italy and around half from the rest of the world.
Position effects
While football is a team sport, with all players sharing the credit for wins and taking joint responsibility for losses, the plaudits are usually not evenly distributed over the field. In particular, strikers tend to get more credit for wins, they have more visibility and sell more merchandise. These features result in higher average valuations for attackers than other out-field players, and the lowest values of all for goalkeepers. This effect would be unrelated to the English player premium if nationality was unrelated to player position, but is that the case?
In untabulated results, we observe that English players are more likely than those from the other four European nations to play as attackers (34% compared with just 26% for Germany and 29% for France). Although the English also comprise the highest proportion of goalkeepers, which constitute the lowest average priced position on the pitch, the differences are less stark, with 14% English players in goal compared with 13% for Germany and 11% for France.
Performance effects
As discussed above, the Football Manager database includes a substantial number of available performance measures. Some studies such as He, Cachucho, and Knobbe (Citation2015) employ a shrinkage estimator (LASSO) in order to incorporate information from a large number of highly correlated performance indicators. However, in order to retain the interpretability of each measure, we instead judiciously select a sub-set of particular measures that are the most relevant for each player position.
Although the regressions below employ the set of individual performance measures listed above, for ease of presentation, employs an overall performance measure of current ability, defined by Football Manager as being ‘based on a scale from 1–200, where 1 means the worst in a competitive league anywhere in the world ever, and 200 means the best in a competitive league anywhere in the world ever. The best players in the world should have about 180–195. Only Diego Maradona and Pele would have 200’.
We then take the averages for all players and also for English and non-English players. We do so separately for each field position, since the relevant metrics are different in each case, with the final two columns in the table reporting -test statistics for the difference between English and non-English and associated
-values respectively. English players display superior aggregate performance in all out-field positions (attacker, midfielder and defender) than the comparable average of all non-English players, but in none of the three cases are these differences statistically significant. There is, however, evidence that English goalkeepers perform highly significantly worse than their non-English counterparts, with the former having performance measures on average around 10% lower (110.06 versus 121.87). Aggregating across all positions in the final row of , we find that English players have a marginally higher average performance measures, (125.50 versus 125.17), but this difference is now highly statistically significant due to the larger sample size when all positions are combined compared with when we examine them separately.
Regression results
The various summary statistics presented above suggest that, compared with their international counterparts, English players are more highly valued and better paid, are more likely to be strikers or goalkeepers, are far more likely to play in the EPL, and have slightly superior performance (except for their goalkeepers, who are significantly worse). Thus, it appears that either the greater English tendency to play as attackers or the riches of the EPL might explain the English player premium that we documented in , but it is clear that a multi-variable approach is warranted.
provides the first set of core regression results where the dependent variable is the natural logarithm of player value in pounds, and standard errors are presented in parentheses. Note that we now switch from analysing actual player values to using their logarithms due to the variable’s considerable skewness in levels. We also include a range of performance measures in the regressions but in order to preserve space we do not report the coefficient estimates, analogous to fixed effect estimates commonly not being reported.
Table 4. Regression results to explain player log-values with country, league, player position dummy variables and performance variables.
We might expect that performance would have more explanatory power than any other group of variables and it is possible that English players are more highly valued and better paid than those of other nationalities due to their superior skill. These conjectures are examined in . We run separate regressions for each player position since the performance statistics are different in each case and they therefore cannot be easily combined or compared. For each of the four positions, we report the results from two sets regressions – both with and without the league dummy variables – since these may have a tendency to swamp other effects. It would be possible to apply a regularization technique such as ridge regression or LASSO; however, since our focus is on comparing the explanatory power between groups of variables rather than on prediction, our concentration is on model interpretation and hence we retain the use of OLS estimation. Variance inflation factors (VIFs) are all within acceptable limits and do not highlight an issue with multicollinearity. Footnote17
A primary variable of interest is the English dummy variable. In untablulated results where it is included as the sole explanatory variable, the estimate is positive and significant at the 1% level, with the reference entity being the rest of the world. In both the full specification (fourth column of ) and the one without the league dummies, the English player dummy remains significant at the 5% and 1% levels respectively for attackers. It is not, however, statistically significant for any of the other field positions (goalkeepers, midfielders or defenders). None of the other nationality dummies are positive and significant in except for marginally significant results for French goalkeepers; the estimates for the Italian nationality variable are strongly significant and negative for all positions except goalkeepers.
Although in unreported results we find that the league effects are very significant when player performance is not incorporated in the model (with valuations being much higher for the English Premier League than others), in , there are barely any significant coefficients on the league dummies – either positive or negative – except for a positive estimate on the Premier League for the midfield position that is significant at the 10% level; in other words, the performance effects subsume those from the league dummies when both are included together in the model. We interpret these findings as implying that on the whole, the richer leagues have been successful in attracting more skilled players, a point that we follow up on in our discussion of below.
Table 5. Regression results to explain player log-wages with country, league, player position dummy variables and performance variables.
Table 6. Shapley-Owen decomposition for groups of variables.
Regarding the final block of variables in the model, only parameters on the squared age terms are consistently significant in a statistical sense, and they are negative. This – when taken together with the positive but less significant effects from the linear age term – implies that player valuations rise in the early stages of their careers as they gain experience, exposure and a track record. But then their valuations gradually drop off as the number of years left in their careers diminish, hence the negative sign on the squared term that will increasingly dominate as the value of the age variable becomes larger.
Although the signs are mostly negative, the estimates on BMI are not statistically significant in , and neither are they on international appearances. Again, if these variables are used in a regression that excludes performance, they are statistically significant with negative and positive signs respectively. Taken together, these results suggest that a players weight will affect their performance, and international appearances will also be correlated with performance (presuming that national teams will select their most accomplished players based on recent matches) so that when performance is included in the model, BMI and international appearances have no further explanatory role.
repeats the analysis of but for log-wages instead of log-values, and the findings from the two dependent variables are very similar. There is an English player wage premium that is now highly significant for both attackers and midfielders (and for defenders when the league dummies are excluded from the model in the final column) but there are no other strong or consistent nationality effects. The Premier League dummy variable is also more frequently significant in than , indicating that its players are paid more than those of other leagues even after jointly allowing for their nationalities and skill levels.
Interestingly, the number of international appearances that a player accumulated has a highly significant and positive effect on player wages for those in any of the four field positions, which was not the case for valuations. A possible interpretation of this finding is that participating successfully on the international stage can give a player perceptions of ‘star quality’ that will sell more merchandise for clubs, and which the player is able to monetize through their wage negotiations even if their intrinsic skill does not warrant it.
There is also a slight switch in the degree of significance of the coefficient on the age and age squared terms, where the former becomes larger and more significant and the latter becomes smaller, albeit the signs remain the same as those of the comparable terms in . The likely explanation is that a players wages continue to rise for a period even as they reach and exceed their valuation peak since their reputation and fan base remain strong, and therefore wages peak at a later age than valuations.
Finally, the model fit summary statistics in the ultimate panels of both suggest that for all player positions, the fit of the models is good – perhaps, surprisingly so given that these are cross-sectional regressions, with typical adjusted R-squared values of 0.6–0.8. As the results from discussed below will show, most of this explanatory power comes from the performance statistics and the R-squared value typically only rises by 0.01–0.02 when the league effect dummy variables are also added. The regression F-statistics are very highly significant for all the model specifications presented in the table.
In order to facilitate comparisons of the relative explanatory power of the groups of variables, presents the Shapley-Owen decompositions of the values for various groups of variables. Each column in the table represents a different set of variable groups, with the dependent variable always the log of the player values. The first column including percentages shows that the league that the player’s team is in (EPL, Bundesliga, etc.) is the most important group of variables, explaining 38% of the variation in log-values. Interestingly, the number of international appearances alone also explains over a third of the variation, with nationality accounting for a little over a fifth of the variation and player characteristics (age, BMI, etc.) explaining a mere 4%.
The second column including percentages adds the group of performance variables, and once this is done, it draws in almost three quarters of the explanatory power, reducing that left for international appearances to just 3%. This suggests that when performance is not explicitly included in the model, international appearances constitute a weaker proxy for it. The effect of the league is also considerably reduced to 7% when performance is included, demonstrating the importance of allowing for individual player skill when modelling valuations. The final column in drops the performance variables and instead includes the player’s position, which diminishes the explanatory roles of all of the other variables in roughly equal proportions compared to the first column of percentages.
VI. Conclusions
Popular opinion and the media have long suggested that English association football players are more expensive when transferring to other teams and are paid more than those of other nationalities, but is this viewpoint justified by empirical evidence? This study has sought to determine the possible existence, and the potential causes, of the English player value and wage premia as part of a wider study on the factors that affect football player valuations and salaries.
We find that, on average, English players are worth more than others by around 40% and they are paid c.25% more. We find support for several plausible explanations for this effect. First, the number of English players in the elite European leagues is smaller than the numbers of any other nationality. This provides a simple, partial explanation for the English player premium, that it is simply the supply side of the law of supply and demand: the prices of English players is bid up because there are fewer of them. While it would not be possible to test the impact of UEFA’s home-grown player rule directly even with a longitudinal dataset due to the number of other vast structural shifts that have taken place in football’s commercial side over the past decade, the limited numbers of English players does appear to have combined with the HGP rule to create a distortion in the market.
Second, English players are considerably more likely than those of other leagues to play in an attacking position, and the statistics in both this study and others are strongly indicative of forwards being more highly valued and paid than those who play in other field positions. Attacking players are more likely to appear on a match’s score sheet, and hence they receive more publicity and sell more merchandise than those in other positions.
Third, we find some indication that the performance of English players is rated more highly than those of other nations in out-field positions, although not significantly so; on the other hand, English goalkeepers are perceived to perform particularly poorly.
Fourth, and importantly, we observe a strong league effect, whereby the average values and salaries of players in the English Premier League is significantly higher than those of any of the other four leagues, even after controlling for a range of other factors, and English players benefit from being the ‘home nation’. When we construct separate models for each field position, the value premium still remains for attackers. We therefore conclude that English footballers playing in a forward position do appear to be overvalued, since the premium evades explanation by league- and performance-related variables, although this is not the case for those located in other parts of the field. The same also applies to wages, where those of English attackers and midfielders are significantly higher than those of other nations after controlling for league and performance.
These findings cannot be attributed to the HGP rule, which would presumably encourage Premier League teams to fill their quotas with home-grown goalkeepers or defenders, who would be cheaper, and they cannot be attributed to league or performance effects. It might be that Premier League clubs are willing to overpay for a small number of high-profile English players, prominently located high up the pitch, to please those fans or sponsors who might be expecting to see such players beyond the extent that their skills warrant.
A final picture emerging from our analysis that is worthy of note and in some ways the flip-side of the English premium is the persistent and statistically significant Italian discount, which appears to defy explanation. Even accounting for their under-representation in the EPL,Footnote18 their performance (as a nation winning the Euro 2020 tournament), field position and other characteristics do not justify their low average valuations. Investigating this phenomenon would be a fascinating topic for future studies.
Acknowledgments
We are very grateful to Sports Interactive for allowing us access to their dataset. We also thank three anonymous referees of this journal as well as Mobolaji Alabi, Tom Markham, James Reade, Andrew Urquhart, and seminar participants at the former School of Accounting and Finance, University of Bristol, and at the Birmingham Business School, University of Birmingham, for very useful comments on a previous version of this paper.
Disclosure statement
No potential conflict of interest was reported by the authors.
Notes
3 See https://football-observatory.com/IMG/sites/mr/mr25/en/or Poli, Ravenel, and Besson (Citation2017).
4 For instance, an article by McCarthy reports that within the most expensive back four players (i.e. defenders) of all time, three would be English (Shaw, Stones and Walker), yet, he argued, none of them would have been the best in the league in that position at the time. This alleged over-valuation has existed for at least a decade, and in 2014 football coach Steve Bruce famously called the phenonemen‘madness’ – see https://www.theguardian.com/football/2014/sep/13/football-news-in-brief.
5 See, for example, https://sportslens.com/3-reasons-why-english-players-cost-more/8303/.
7 While Gasparetto and Barajas (Citation2022) use some data from Football Manager, to our knowledge this is the first study that uses the wide range of player performance statistics.
8 As far as we are aware, the only existing study that employs Football Manager data, Tosato and Wu (Citation2018) uses it to select players for the AS Roma team alone.
9 The rule is nicely summarized in a news article on the Premier League website, which we quote from: https://www.premierleague.com/news/1869523.
10 See the Premier League website reference in the previous footnote.
11 We should also note that while our core variable of interest, namely player nationality, is unquestionably exogenous, the allocation of players to leagues (and pitch positions) is not random and this ‘equilibrium sorting’ will likely cause some correlations between the explanatory variables, which we do not address in the study.
12 See https://www.transfermarkt.co.uk.
13 The website states that in order to construct wage data they ‘rely on a network of insiders directly involved in contract negotiations as well as publications around the world. When these are still not sufficient, Capology’s algorithms provide a best estimate’. – see https://www.capology.com.
14 https://www.transfermarkt.co.uk/market-value-definition/thread/forum/357/thread_id/3433.
15 See https://www.inputmag.com/gaming/volunteer-labor-is-the-lifeblood-of-football-manager or also https://www.footballmanager.com/the-byline/researcher-interviews-scotland#desktop for details.
17 These are not shown due to space constraints.
18 In the 2020/21 there was only one of the Italian EURO 2020 winning team who was playing in the ELP, Jorginho at Chelsea.
References
- Ante, L. 2019. “Determinants of Transfer Fees: Evidence from the Five Major European Football Leagues.” Working Paper Faculty of Business, University of Hamburg.
- Behravan, I., and S. M. Razavi. 2021. “A Novel Machine Learning Method for Estimating Football players’ Value in the Transfer Market.” Soft Computing 25: 2499–2511. doi:10.1007/s00500-020-05319-3.
- Bryson, A., B. Frick, and R. Simmons. 2013. “The Returns to Scarce Talent: Footedness and Player Remuneration in European Soccer.” Journal of Sports Economics 14 (6): 266–286. doi:10.1177/1527002511435118.
- Burdekin, R., and M. Franklin. 2015. “Transfer Spending in the English Premier League: The Haves and the Have Nots.” National Institute Economic Review 232: R4–17. doi:10.1177/002795011523200102.
- Carmichael, F., D. Forrest, and R. Simmons. 1999. “The Labor Market in Association Football: Who Gets Transferred and for How Much.” Bulletin of Economic Research 51 (2): 125–150. doi:10.1111/1467-8586.00075.
- Coates, D., and P. Parshakov. 2022. “The Wisdom of Crowds and Transfer Market Values.” European Journal of Operational Research 301: 523–534. doi:10.1016/j.ejor.2021.10.046.
- Cox, R., L. Hills, and E. Kennedy. 2015. “Myths of Nation in the Champions League Soccer &.” Society 16 (5–6): 674–692. doi:10.1080/14660970.2014.963309.
- Dalziel, M., P. Downward, R. Parrish, G. Pearson, and A. Semens. 2013. “Study on the Assessment of Uefa’s ‘Home Grown Player Rule’ Negotiated Procedure EAC/07/2012.” Working Paper, The University of Liverpool and Edge Hill University.
- Deloitte. 2021. Annual Review of Football Finance, Sports Business Group. July 2021, https://www2.deloitte.com/uk/en/pages/sports-business-group/articles/annual-review-of-football-finance.html.
- Depken, C. A., III, and T. Globan. 2021. “Football Transfer Fee Premiums and Europe’s Big Five.” Southern Economic Journal 87 (3): 889–908. doi:10.1002/soej.12471.
- Dobson, S., and B. Gerrard. 1999. “The Determination of Player Transfer Fees in English Professional Soccer.” Journal of Sport Management 13 (4): 259–279. doi:10.1123/jsm.13.4.259.
- Dobson, S., B. Gerrard, and S. Howe. 2000. “The Determination of Transfer Fees in English Nonleague Football.” Applied Economics 32 (9): 1145–1152. doi:10.1080/000368400404281.
- Fabrizio Montanari, F., G. Silvestri, and F. Bof. 2008. “Performance and Individual Characteristics as Predictors of Pay Levels: The Case of the Italian ‘Serie A’.” European Sport Management Quarterly 8 (1): 27–44. doi:10.1080/16184740701814381.
- Feess, E., B. Frick, and G. Muehlheusser. 2004. “Legal Restrictions on Buyout Fees: Theory and Evidence from German Soccer.” IZA Discussion Papers 1180, Institute for the Study of Labor (IZA).
- Feess, E., B. Frick, and G. Muehlheusser. 2007. “Contract Duration and Player Performance: Empirical Evidence from German Soccer.” Working Paper Faculty of Management and Economics, Witten Herdecke University, Witten.
- Felipe, J. L., A. Fernandez-Luna, P. Burillo, L. E. de la Riva, J. Sanchez-Sanchez, and J. Garcia-Unanue. 2020. “Money Talks: Team Variables and Player Positions That Most Influence the Market Value of Professional Male Footballers in Europe.” Sustainability 12 (9): 3709. doi:10.3390/su12093709.
- Frick, B. 2007. “The Football players’ Labor Market: Empirical Evidence from the Major European Leagues.” Scottish Journal of Political Economy 54 (3): 422–446. doi:10.1111/j.1467-9485.2007.00423.x.
- Gasparetto, T., and A. Barajas. 2022. “Wage Dispersion and Team Performance: The Moderation Role of Club Size.” Journal of Sports Economics 23 (5): 548–566. doi:10.1177/15270025211067793.
- Gerhards, J., and M. Mutz. 2017. “Who Wins the Championship? Market Value and Team Composition as Predictors of Success in the Top European Football Leagues.” European Sciences 19 (3): 223–242. doi:10.1080/14616696.2016.1268704.
- He, M., R. Cachucho, and A. Knobbe. 2015. “Football Player’s Performance and Market Value.” Working Paper, LIACS, Leiden University, The Netherlands.
- Herm, S., H. -M. Callsen-Bracker, and H. Kreis. 2014. “When the Crowd Evaluates Soccer players’ Market Values: Accuracy and Evaluation Attributes of an Online Community.” Sport Management Review 17: 484–492. doi:10.1016/j.smr.2013.12.006.
- Kesenne, S. 2007. “The Peculiar International Economics of Professional Football in Europe.” Scottish Journal of Political Economy 54: 388–399. doi:10.1111/j.1467-9485.2007.00421.x.
- Kiefer, S. 2012. “The Impact of the Euro 2012 on Popularity and Market Value of Football Players.” Working Paper Westfälische Wilhelms-Universität Münster, 11/2012.
- Kuper, S., and S. Szymanski. 2018. Soccernomics. Revised Edition ed. London: Harper Collins.
- Lehmann, E. E., and G. G. Schulze. 2008. “What Does It Take to Be a Star? The Role of Performance and the Media for German Soccer Players.” Applied Economics Quarterly 54 (1): 59–70. doi:10.3790/aeq.54.1.59.
- Lucifora, C., and R. Simmons. 2003. “Superstar Effects in Sport – Evidence from Italian Soccer.” Journal of Sports Economics 4 (1): 35–55. doi:10.1177/1527002502239657.
- Magee, J., and J. Sugden. 2002. ““The World at Their Feet”.” Journal of Sport and Social Issues 26 (4): 421–437. doi:10.1177/0193732502238257.
- Metelski, A. 2021. “Factors Affecting the Value of Football Players in the Transfer Market.” Journal of Physical Education and Sport 21 (2): 1150–1155.
- Müller, O., A. Simons, and M. Weinmann. 2017. “Beyond Crowd Judgments: Data-Driven Estimation of Market Value in Association Football.” European Journal of Operational Research 263: 611–624. doi:10.1016/j.ejor.2017.05.005.
- Neri, L., A. Russo, M. Di Domizio, and G. Rossi. 2021. “Football Players and Asset Manipulation: The Management of Football Transfers in Italian Serie a.” European Sport Management Quarterly, Forthcoming. doi:10.1080/16184742.2021.1939397.
- Poli, R., L. Ravenel, and R. Besson. 2016. “Foreign Players in Football Teams.” CIES Football Observatory Monthly Report (12 February): https://football-observatory.com/IMG/pdf/mr12_eng.pdf
- Poli, R., L. Ravenel, and R. Besson. 2017. “World Expatriate Footballers.” CIES Football Observatory Monthly Report (25 May): https://football-observatory.com/IMG/sites/mr/mr25/en/
- Poli, R., L. Ravenel, and R. Besson. 2020. “Scientific Evaluation of the Transfer Value of Football Players.” CIES Football Observatory Monthly Report 53.
- Rohde, M., and C. Breuer. 2017. “The Market for Football Club Investors: A Review of Theory and Empirical Evidence from Professional European Football.” European Sport Management Quarterly 17 (3): 265–289. doi:10.1080/16184742.2017.1279203.
- Ruijg, J., and H. van Ophem. 2015. “Determinants of Football Transfers.” Applied Economics Letters 22 (1): 12–19. doi:10.1080/13504851.2014.892192.
- Singh, P., and P. S. Lamba. 2019. “Influence of Crowdsourcing, Popularity and Previous Year Statistics in Market Value Estimation of Football Players.” Journal of Discrete Mathematical Sciences and Crypotgraphy 22 (2): 113–126. doi:10.1080/09720529.2019.1576333.
- Torgler, B., and S. L. Schmidt. 2007. “What Shapes Player Performance in Soccer? Empirical Findings from a Panel Analysis.” Applied Economics 39 (18): 2355–2369. doi:10.1080/00036840600660739.
- Tosato, M., and J. Wu. 2018. “An Application of PART to the Football Manager Data for Players Clusters Analyses to Inform Club Team Formation.” Big Data and Information Analytics 3 (1): 43–54.
- UEFA. 2018. Club Licensing Benchmarking Report https://www.footballbenchmark.com/library/2020_uefa_club_licensing_benchmarking_report
- Wilson, D. P., and Y. -H. Ying. 2003. “Nationality Preferences for Labour in the International Football Industry.” Applied Economics 35 (14): 1551–1559. doi:10.1080/000368403200010048.