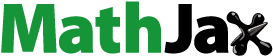
ABSTRACT
The theoretical literature on the FDI-growth relationship suggests that FDI with high technological content might play a peculiar role. To investigate the existence and magnitude of this peculiar effect, we collected data for 28 countries over the period 1989 to 2019 and used GMM techniques for empirical estimation. We employed the U.N. International Standard Classification (ISIC) Revision 3 to classify FDI data and follow the criteria laid down by the Organization for Economic Cooperation and Development (OECD) to distinguish FDI data by technological content. The empirical findings of this article confirm that technology plays an important role in determining the FDI-Growth relationship. Interestingly, the empirical evidence supports a U-shaped relationship between FDI and economic growth when FDI is disaggregated by different technological contents. More specifically, we find strong evidence that in the manufacturing sector, FDI with a higher technological content exhibits a positive association with growth in the host country. We also find evidence pointing towards a positive relationship between FDI and growth in the host country at the other end of the technology spectrum (low-tech). Further investigation confirms the robustness of these findings across different estimation techniques as well as across different sampling strategies.
I. Introduction
The relationship between Foreign Direct Investment (henceforth FDI) and economic growth (henceforth EG) in the host country has been studied widely in the literature. Such keen interest was sparked by the surge in the flow of FDI to a number of host countriesFootnote1 which has taken place in recent decades as multinational firms looked for different ways of expanding their business beyond their national borders ().
Figure 1. FDI inflows, global and by group of economies 1990–2020.
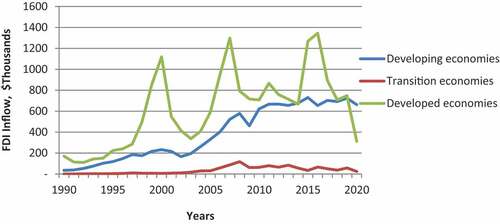
Based on empirical evidence, there is no consensus on whether such a relationship exists or the direction of causality.Footnote2 A broader consensus has been reached on the existence of a relationship between FDI and EG after taking into account threshold effects in a number of covariates, like, but not limited to, human capital, financial development, etc.Footnote3 However, very few studies to date have tried to probe whether the existence of a relationship between FDI and EG depended on the sector of industry the FDI was flowing into or the type of FDI involved.Footnote4
Until recently, lack of granularity in FDI data was a major constraint for anybody who wanted to explore these issues. As these data become more plentiful and more detailed and cover longer time span, this constraint is being relaxed. In particular, in the past data constraints forced scholars and policymakers to treat FDI as a whole, irrespective of the type and sector of the economy. However, more recently, a growing interest has started to appear in probing whether the relationship between FDI and EG changes depending, for example, on the sector concerned (agriculture, manufacturing or services), on the type of FDI involved (horizontal or vertical FDI are dimensions that have been investigated and so too are greenfield investment or mergers and acquisitions) or whether it changes depending on the country FDI originates from. More details on these attempts to distinguish FDI in some way will be given later in the literature review section.
The present study is an attempt at bridging the gap in the existing literature in this direction. More specifically, we focus on the role played by technology transfers to the host country as an important channel linking FDI to EG (two of the most seminal studies in this respect are De Mello Citation1997; Borensztein, Gregorio, and Lee Citation1998). To this end, this article aims at probing whether the effect of FDI on growth varies depending on its technological content. Our objective is to show that the effect of FDI on the EG and development of the recipient nation depends crucially on the technology embodied in it. More specifically, we disaggregate FDI into different sectors and technological contents to differentiate the role of each sector and content on EG. In other words, what we would like to show is that the heterogeneity in the technological content of diverse types of FDI matters.
Based on the evidence we gather, we find that, after differentiating FDI by technological content, a U-shaped relationship emerges relating FDI, technology and EG. At both ends of the technological spectrum, FDI flows with high and low technological content are highly conducive to EG, while the two intermediate types of FDI with medium technological content are not. This result holds across two different estimation techniques – generalized method of moments (GMM) and fixed effects (FE) and two sampling methods – first including countries for which the FDI value is non-zero and then using a full sample of all countries.Footnote5 (More details are given in the main body of the article below). We therefore conclude that this result is not sensitive to the econometric methodology used or to the inclusion or not of zero values for the relevant FDI type. This study contributes to the existing literature in several ways: as mentioned, we uncover important technology spillovers on the economic performance of host countries. But we add to this more nuance and additional findings. First, we find that the positive effect of technology is limited to FDI with high technological content. In contrast, as that technological content drops, the effect of FDI on the EG of the host country becomes indiscernible at best. We conjecture that this finding may be due to other, less benign effects of FDI that may offset wholly or partially the positive effects of technology. However, we also uncover an intriguing feature of FDI at the other end of the technology spectrum, namely low-technology FDI. This FDI type is found to have a positive impact on the economic growth of the recipient country. In this instance, this positive effect is probably not due to technology spillovers. Here, we conjecture that low-tech FDI may better fit with the economic texture of recipient countriesFootnote6
The article is organized as follows: Section II briefly reviews the literature on FDI and EG, which is relevant for our stated goals; Section III lays out the econometric model to be estimated and sketches the econometric challenges being faced in the estimation; Section IV details data, data sources and descriptive statistics; Section V illustrates the results of the estimation exercise, while Section VII mentions possible extensions and future line of work and draws conclusions.
II. A brief look at the existing empirical literature
The literature on the relationship between FDI and EG is extensive, but its review is beyond the scope of this article. Since the focus of this article is to exclusively investigate how different types of FDI impact EG, we shall restrict our review to the literature that specifically covers this part.
Alfaro (Citation2003) can be considered as the first attempt at investigating the above question. By using cross-country data for 47 countries over the period 1981–1999, she differentiated investment into three sectors (primary, manufacturing and services) and studied the effect of FDI inflows in these sectors on EG. She found that the effect of FDI on EG was sector-dependent. Her empirical findings showed that FDI into primary sector had a negative impact on growth, the impact of FDI in manufacturing was positive, while the effect of FDI in the services sector was ambiguous.Alfaro and Charlton (Citation2007) in an extension to this work distinguished different qualities of FDI by adding specific industry characteristics. Using a panel of 29 countries and 15 years (1985–2000), they found a positive relation between FDI and EG in industries with higher skills requirements and in industries more reliant on external capital.
Beugelsdijk, Smeets, and Remco (Citation2008) distinguished the growth effect of FDI into horizontal (market seeking) FDIFootnote7 and vertical (efficiency seeking) FDI. Growth effects were estimated using a sample of 44 host countries over the period 1983 to 2003. The article also splits the sample into developed and developing countries to probe whether differences depend on country-status. The empirical findings confirmed positive and significant growth effects from both horizontal and vertical FDI in developed countries, while no significant relationship was found for the sample of developing countries. The article also found that the effect of horizontal FDI to be about 50% larger than that of vertical FDI.
Chakraborty, Nunnenkamp, and Nunnenkamp (Citation2008) used panel data of 15 industries for India segregating it into three sectors: manufacturing, primary, and services (1987–2000) and found a positive impact of manufacturing FDI on EG but no evidence of a similar relationship was found for primary sector FDI, while the services sector had only transitory effects. Massoud (Citation2008) did a similar exercise using data from Egypt (1974–2005) and found that only manufacturing FDI promotes EG (when interacting with human capital). In another paper (Wang Citation2009) used a sample of 12 Asian economies over the period 1987–1997. He further split the FDI data into manufacturing and non-manufacturing FDI and investigated their impact on EG of host countries.. The empirical results showed that manufacturing FDI had a positive and significant impact on EG of the host country, whereas the effect of FDI inflows in non-manufacturing sectors was ambiguous.
Further studies on FDI attempted to split business activities (both in the manufacturing and services sectors) on the basis of their technological content. Hatzichronoglou (Citation1997), based on R&D intensities in each sector, classified sectors by technological content. Mani (Citation2000) used the same classification as Hatzichronoglou (Citation1997) while studying high-tech exports for a sample of OECD countries. This classification was then finalized in OECD (Citation2003) and referred to in subsequent OECD documents covering technology intensity (see, e.g. OECD Citation2011). We use this classification in our attempt to differentiate FDI by technological content.
Citation2015) use data from the WB–IFC Enterprise Survey to determine backward linkages from FDI for developing countries. Part of the work is to determine the motivation of foreign-owned firms across sectors. The study finds that ‘some sectors (food, wood, auto and auto parts) are more prone than others (textiles and electronics) in developing backward linkages’. Gönel and Aksoy (Citation2016) investigated the relationship between FDI and growth when FDI is differentiated by three sectors of activity. They found that while aggregate FDI has a significant and positive impact on EG, FDI belonging to the three sectors on their own has no effect on EG. They do find, however, that a certain absorptive capacity in the host country is conducive to FDI having a positive effect on EG.
In a recent paper, Shah et al. (Citation2019) looked at the relationship between sectoral FDI and domestic investment in Pakistan. They used an autoregressive distributed lag (ARDL) model for data from Pakistan over the period 1980–2012. They tackle the specific question by splitting FDI into three sectors, primary, manufacturing and services. The results suggest that FDI in the manufacturing and services sectors has a positive impact on domestic investment, while primary sector FDI does not influence domestic investment. In another paper, Jana, Sahu, and Pandey (Citation2019) employed a time-varying parameter model for data from India covering the period 1995–2016 to study the effects of sector-specific FDI inflows on economic growth. The findings of this article revealed that in the case of India, the agriculture sector FDI does not have any positive impact on agriculture production or growth. For manufacturing and services sectors, the study found a positive relationship between FDI and EG.
Ingham, Read, and Elkomy (Citation2019) did a similar study using data from Egypt (1990–2007). They used a fixed effect model for unpublished data from the General Authority of Free Zones and Investment (GAFI). The findings show a positive growth effect in manufacturing and petroleum sectors but not in other sectors, such as finance and retail, tourism, construction and agriculture. Interestingly, the manufacturing sector also exerts positive spillovers on the latter sectors.
Doytch and Uctum (Citation2019) used a sample of 14 Asia Pacific economies, with data spanning 1985–2012 to investigate the impact of services FDI on EG. They first disaggregated FDI by manufacturing and services and then further disaggregated services into financial services, trade services, and business services. Interestingly, this article found services FDI to have a positive impact on growth, while no effect on growth was found for manufacturing FDI.
The present article can be considered as an extension of the above literature. There are two major differences in this article. First, while the existing literature is limited to individual country or to a specific region, this article uses a large global sample of 48 developing and developed countries. Second, the classification of FDI flows by sector in the existing studies is limited to broad sectors, such as agriculture, manufacturing and services. In this article, we use a more detailed classification of FDI based on technological content and include more sectors (see section III below). Interestingly, the results of this article are contrary to Gönel and Aksoy (Citation2016) and we did not find any empirical support for the effect of aggregate FDI on EG of the host country. However, our results suggest an impact on EG for FDI with high and low technological content, respectively.
III. Econometric model, methodology and estimation challenges
The main objective of this article is to investigate whether differentiating FDI by typein particular, distinguishing FDI on the basis of the technological content embedded into it, can help shed further light on the nature of the relationship between FDI and EG beyond what we already know about it.
As a first step, we differentiate FDI by sectors and then by technological content for each sector. The World Investment Directory (henceforth W.I.D.) classifies FDI data according to the U.N. International Standard Classification (ISIC) Revision 3 (UNSD Citation2021). This provides a very detailed and accurate classification of economic activities, which makes the task of grouping sectors by technological content far easier. We follow the OECD criteria for differentiating sectors by technological content (OECD Citation2003), and accordingly use four different groups in the manufacturing (secondary) sector. As for the services sector, we split the sector into two groups: high tech and low tech according to the use of incorporated technologies, R&D intensities and the qualification (skillness) of the workers. To do so, we follow in Anghel (Citation2006) which in turn draws from Citation2003). Next, we employ FDI/GDP ratios for specific sectors and sub-sectors. These details are provided in .
Table 1. FDI decomposition by sector and by technology.
Table 2. FDI variables with definitions.
For empirical estimation, we, first, run a benchmark panel regression (EquationEquation 1(1)
(1) ) with the (annual) growth rate of GDP per capita as the dependent variable and centred on the FDI/GDP ratio as the main regressor as follows:
where is the (real) annual growth rate of GDP per capita for country i at time t and
is the FDI/GDP ratio for country i at time t. We also include the lagged value of the dependent variable (
) as first regressor and a set of standard control variables included in the vector
. These comprise domestic credits (d_credit), inflation, population growth (pop_growth), trade, government expenditures (gov_exp), gross fixed capital formation (gfcf) and our measure of human capital (hc), which is a human capital index taken from Penn World Tables 9.1Footnote8 (see Section V on data and sources for more detail). All the controls’ values are measures for country i at time t. It follows that
is a vector of coefficients corresponding to the vector of controls
Footnote9and
is a standard error term. The need to account for any remaining country specific characteristics not already controlled for would suggest the use of the fixed effect model (in short: FE) in this regression. The Hausman test confirms that the fixed effects model is superior to the random effects model in our case.Footnote10 However, we can further reduce the risk of obtaining biased results by employing the system Generalized Method of Moments technique (system GMM). GMM estimation is an improvement on FE estimation in at least three ways.
First, the model, as specified in EquationEquation (1)(1)
(1) is dynamic insofar as it includes the lagged value of the dependent variable as one of the regressors. GMM estimation enables us to account for the dynamic nature of the model.
Second, the length of the period under study (from 1989 to 2019) increases the likelihood of structural breaks somewhere along the line. Once again, GMM estimation is equipped to handle structural breaks in the data, while FE estimation is not.
Finally, and perhaps most decisively, GMM estimation is a lot better than FE estimation at addressing the endogeneity bias, frequently lurking in this type of panel data, where it is hard to know ex ante the direction of causality (if any) between the designated dependent variable (EG in this case) and independent regressors of interest (here: FDI).
For the sake of completeness of exposition and intellectual openness, it must be said that GMM estimation carries the risk of some bias of its own. For example, a blind use of this technique can lead to instrument proliferation and consequent so-called overfitting bias. A large time dimension T can also bias the results. Finally, the use of weak instruments (not correlated with the dependent variable but not orthogonal to the other regressors) can bias the results in the direction of the OLS estimator.
We test the weakness of the instruments by running a standard Sargan test. We address the overfitting bias caused by instrument proliferation in two ways. We suitably reduce the number of lags and apply the collapse option available in Stata. We report on the number of instruments for all GMM regressions in each table.
In this paper, we use the GMM model in regression (1) and all the other annual panel regressions used in this article. However, in order to allay any fears related to GMM estimation, we also report our results from FE estimation in appendix B, .
The second equation differentiates FDI by type. In EquationEquation (2)(2)
(2) , we replace the total FDI/GDP ratio (in EquationEquation 1
(1)
(1) ) with the sum of sectoral FDI/GDP ratios from the Primary, Secondary and Services sectors. Everything else is the same as in EquationEquation (1)
(1)
(1) .
Next, in order to explore differences in FDI types with differing technological content associated with the growth rate of GDP per capita, in EquationEquation (3)(3)
(3) we replace the FDI/GDP ratio with the sum of the six sub-variables resulting from the full FDI decomposition according to technological content. These technology-related distinctions are referred as high-tech (HTFDI), medium high-tech (MHTFDI), medium low-tech (MLTFDI) and low-tech (LTFDI). Accordingly, we replace the variable FDI with the sum of the sub-variables HTFDISec, MHTFDISec, MLTFDISec, LTFDISec, HTFDIServ and LTFDIServ and their respective coefficients, then add the same set of control variables as in EquationEquation (1)
(1)
(1) . As a result, EquationEquation (3)
(3)
(3) looks as follows:
EquationEquation (3)(3)
(3) is the main equation we use in this article to empirically verify our research question.
As part of a robustness test, we run an additional equation (EquationEquation 4(4)
(4) ) which focuses on the secondary sector only by including its four different types of FDI as in EquationEquation (3)
(3)
(3) but leaving out the two FDI types of the services sector. Hence, EquationEquation (4)
(4)
(4) includes the sum of FDI/GDP ratios from the secondary sector only, differentiated by technological content:
Since the empirical literature on FDI-EG relationship is inconclusive, in this article, we expect the sign to be positive or negative. Lagged GDP is also expected to have a positive sign. For sector-specific FDI, the literature (as stated in Section II above) has gathered mixed evidence. However, a majority of studies suggest a positive relationship between manufacturing sector FDI and EG, while the same is not supported for services and primary sectors. In this article, we have disaggregated industries into secondary, primary and services sectors and then further disaggregated the data for the secondary and services sectors by their technological content as discussed above. To the best of our knowledge, there is no research is available on this disaggregation.Footnote11 In our view, technology will further strengthen the relationship.
As for control variables, theoretically, domestic credit (to the private sector) is expected to have a positive impact on growth. However, empirical evidence in the literature is mixed. Khalid and Marasco (Citation2019) discussed this issue and found a negative relationship between EG and domestic credit.Footnote12 Hence, we leave the impact of domestic credit on growth on empirical verification. Inflation is expected to have a negative impact on growth. Neoclassical growth theory suggests that population increase can impact growth negatively. Crowding-out literature suggests that increases in government expenditure should have a negative impact on growth, especially if the country is facing a persistent budget deficit. Both trade and gross investment are expected to have a positive impact on growth. Finally, human capital should help improve productivity and impact growth in a positive manner.
IV. Data, sources and descriptive statistics
This section discusses the data used in our study. First, we illustrate and discuss FDI data and sources, including a discussion of the criteria and the sources that were used for the FDI decomposition we adopted. Later, we list the data and sources concerning all the other variables used in the study.
As stated earlier, the main constraint we faced in the empirical analysis was the availability of FDI data classified by the sector of activity. Unfortunately, there is no single source where all data are available. To overcome this issue, we use different sources to collect data for the variables used in this study.Footnote13 Typically, data collection in this respect is the responsibility of individual countries’ central banks or statistical offices. Additionally, several international agencies (United Nations, OECD, World Bank, to name but a few) have at different times attempted to collate data concerning countries in different geographical areas. In this study, we collected data for 48 countries, spanning the period between 1989 and 2019. The number of countries after dropping to zero values for the relevant FDI variables goes down to 28. We also used the full sample to segregate data into the high-income countries’ (31 countries) and European region (26 countries).
It is important to note that the sample of 48 countries (both developed and developing) used in this study includes five main regions: 6 countries are from East Asia and the Pacific, 26 countries are from Europe and Central Asia, 13 are from North and Latin America, 1 (Tunisia) is from the Middle East and Africa and 2 (India and Pakistan) are from South Asia. The most notable absences from this sample, due to lack of detailed FDI data, are China and Russia. The full list of countries is available in Appendix A, .
We used three different sources to collect data. Data on human capital come from the Penn World Tables (version 9.1). This source gives a human capital index, which is based on the average years of schooling from Barro and Lee (BL, 2013) and an assumed rate of return to education, based on Mincer equation estimates around the world (Psacharopoulos, 1994).Footnote14 Data for the dependent variable, growth rate of GDP per capita, as well as data on all other regressors – domestic credit, government expenditure (both measured as ratios to GDP), population growth (annual percent), trade (defined as the sum of exports and imports of goods and services, measured as a share of GDP), gross fixed capital formation (percent of GDP) and inflation *GDP deflator, percent) are obtained from the World Bank World Development IndicatorsFootnote15 Individual countries’ central banks and statistical bodies are used to collect data on FDI-related variables (FDI and its classification by sectors and technology content).
We first report the summary and correlation statistics across all variables concerned (see Appendix ):
One apparent feature in the data is that the number of observations drops drastically when it comes to FDI variables, which confirms that FDI data constitute the bottleneck for this study. However, it is of note that we managed to keep the number of observations above the 1000 mark for all the FDI variables when classified by technological content. The very small magnitude of the ratios in the FDI decomposition based on technology reflects the fact that the FDI inflow (the numerator) is generally a tiny proportion of GDP (the denominator).
For correlation statistics, the correlation coefficients provide a first hint of the findings that will come with the regressions. For example, the FDI is negatively correlated with EG (value: −0.036), whereas HT FDI Sec is positively correlated with EG (value: 0.0893).
V. Results and robustness checks
The results of empirical estimation are presented and discussed below. We discuss here the results of the GMM regressions after dropping zero values for the FDI/GDP ratios.Footnote16 These results are presented in .
Table 3. Dynamic system GMM non-zero countries.
We start with EquationEquation 1.(1)
(1) In contrast to many earlier studies with respect to FDI regressors, we find that the association between FDI Total and EG, though positive, is not significant, at least in GMM regression. This unexpected result might be due to some heterogeneity in the effect of different FDI types across sectors on EG. To verify this, we run EquationEquation (2)
(2)
(2) through (Equation4
(4)
(4) ).
Before we do that, we briefly comment on the control variables. Domestic credit is statistically significant and has a negative sign as expected. Consistent with theoretical expectations, inflation has a negative sign but is mostly statistically insignificant except for EquationEquation 4.(4)
(4) Similarly, a faster growth in population has a negative and significant impact on EG. However, the result concerning Trade is surprising. Theoretically and empirically, we anticipated trade to have a positive relationship with EG. Trade serves as an indicator of openness and is expected to enhance growth. The results in confirm this positive relationship, but only for models 1 and 2 where the coefficient of trade is positive but only significant in model 1. In models 3 and 4, the trade coefficient is negative but statistically insignificant. Statistical insignificance may be interpreted as no relationship between trade and growth. As expected, government expenditure has a negative and statistically significant sign. Domestic investment (gfcf) has a positive impact on growth and is mostly significant (except model 1). Finally, human capital (hc) has a positive relationship with growth but is statistically insignificant. In general, our control variables are significant and have the expected sign.Footnote17
Table 4. Total FDI and growth, comparative view.
EquationEquation (2)(2)
(2) , which features FDI decomposition broadly by sector, indicates that there is a highly significant and positive association between FDI in the manufacturing sector and EG. This result is in line with a good part of the earlier literature on the subject. FDI in services is positive but not significant, while in primary sector, FDI is negative and slightly significant (at 10%) confirming the presence of heterogeneity across sectors.
As already discussed earlier, the main goal of this study is not to arrive at a final settlement in the debate on the growth-fostering role of FDI but rather to investigate the role of technology (if any) in the FDI-EG relationship. To this end, we decompose FDI by technological content as explained in Section III and run regressions (3) and (4). As already mentioned, we do this mindful of the argument that technology may be one of the main channels through which FDI may benefit recipient countries. Regression (3) features the full decomposition. Now, with respect to the secondary sector, the FDI/GDP ratios at both ends of the technology spectrum are significant and positive, indicating a positive association between FDI of these types and EG.
The coefficient attached to HT FDI Sec is 1.680, that is, an increase in HT FDI Sec by 100% points (by 1) increases the growth rate of GDP per capita by 168% points. Obviously, for a correct interpretation, we need to rescale to account for the small magnitude of the relevant variables. Hence, for example, a 10% increase in the mean value of HT FDI Sec for our sample, from 0.05% to 0.055%, will be associated with an increase of 0.084% percentage points in the growth rate of GDP per capita (obtained by multiplying the original coefficient by the actual increase in percentage points, 0.005). Similar interpretations can be given to other significant coefficients in the regression. Regression (3) points to the very important role that HT FDI Sec may have in the FDI-EG relationship. As we move down the technology spectrum, the coefficients lose significance, only to return significantly (at the 1% level) at the other end of the spectrum with a coefficient value of 1.155. This result points to LT FDI Sec as another important determinant of EG. The same regression also shows the importance of technology in the Services sector where the coefficient of HT FDI Serv, with a value of 0.0602, is highly significant and positive.
In regression (4) which focuses on the manufacturing sector only, the results are almost identical to those of regression (3). Hence, an increase in HT FDI Sec by 100% points would now have a slightly weaker impact on EG by raising the growth rate of GDP per capita by 145% points. In other words, a 10% increase in HT FDI Sec would increase EG by 0.0072%. As in EquationEquation 3(3)
(3) , neither MHT FDI Sec nor MLT FDI Sec would have a significant impact on EG, but LT FDI Sec would (at the 1% significance level).Footnote18 As a robustness check, we also ran all four equations using FE estimation. These results are reported in Appendix B, .Footnote19
Digging deeper: zero values, stratification by income and by geography
One important remark is in order with respect to the FDI/GDP ratios. When decomposing FDI by type, the data on FDI/GDP ratios include several zero values, particularly with respect to FDI with high technological content. One simple way of dealing with this issue is to drop the zero values from the sample for the variable of concern. In spite of its apparent simplicity and seeming crudeness, this strategy may not be clearly inferior to a number of alternative strategies that entail the use of alternative estimators [Kareem and Kareem 2019]. This is particularly true when the variable featuring the zero values is not the dependent variable but one (or more) of the independent regressors as in our case He et al. (Citation2014). For this reason, in this study we chose to give baseline status to the results after dropping the zero values in the FDI/GDP ratios but also to retain and report results for the full dataset inclusive of the zero values. In this sub-section, instead of offering a detailed description of all the results for the full sample, we chose to only underline the main differences in results between the full sample and the baseline case with non-zero countries. Accordingly, results of GMM and FE estimates (for the full sample) are provided in Appendix and B8, respectively.
In EquationEquation (1)(1)
(1) , FDI is now negative and significant in GMM regression (but not in FE regression). This is most likely due to the fact that, in contrast with the baseline case, the manufacturing sector FDI now does not have a significant impact on EG. It is remarkable, however, that after diversifying FDI by technology, there is no longer any major difference between the baseline results and the full-sample regressions: in all cases both High-Tech FDI and Low-Tech FDI have a significant and positive impact on EG, whereas both middle categories, Middle-High-Tech and Middle-Low-Tech FDI fail to exhibit any relationship with EG. As already pointed out in the introduction, this U-shaped relationship between different FDI types (by technology) and EG is remarkably robust throughout different estimation techniques (GMM or FE) as well as different sampling strategies (only non-zero countries versus full sample). Finally, to control for heterogeneity in our sample, we stratified countries by geography and by income. With the former stratification, the sample was shrunk to contain European countries only. Europe was the only geographical area that guaranteed a sufficient number of countries to keep the analysis meaningful. As for the stratification by income, we restricted the sample to high-income countries only. Once again, we could not give consideration to other income groups separately, as this would have shrunken the sample size too far. For the same reason in all these further stratifications, we did not drop countries with zero values. Both Europe and the high-income group returned results that are by and large similar to those already obtained when all countries were considered. Since there is an overlap between the European sample and the high-income sample, only GMM and FE estimation results for the high-income countries sample are reported in Appendix and B9, respectively. Results concerning the European sample are available upon request.
In particular, we again find no evidence of any effect of total FDI on EG, but we do find that manufacturing FDI has a positive impact on EG, while the effect of primary FDI on EG is negative. The effect of FDI types on EG after classifying FDI by technology are also confirmed along the same lines as in the sample with all countries, though in these instances of Europe and the high-income group, the positive association of High-Tech FDI and Low-Tech FDI with EG is less significant than before. The fall in the significance level, which is now between 5% and 10%, is perhaps due to a weaker impact, technology spillovers might have on well-developed economies such as those in Europe and those classified as high income. Finally, the signs and significance levels for all control variables are the same as in the full sample including the whole world.
VI. Discussion
In view of the above remarks, our results are interesting and contain more than one element of surprise. First, we do not find any statistically significant relationship between aggregate FDI and growth in the host country. This is somewhat surprising already since this finding is not in line with many past studies on the same topic. There are, however, quite a number of studies that, like ours, fail to spot a relationship between aggregate FDI and growth, hence our result is not entirely unexpected.Footnote20 We attribute the lack of a relationship to the heterogeneity of effects on EG across different FDI types that manifests itself in the classification by sector.
The evidence gathered after classifying FDI by technological content reinforces this argument. This evidence points to the existence of a U-shaped relationship between different FDI types and growth, which is robust across all different estimation techniques as well as all sampling strategies. To display this robustness most clearly, we decided to display the main results once again in comparative format across estimation techniques and sampling methods. This is done in and .
Table 5. FDI by sectors and growth, comparative view.
Table 6. FDI by technology and growth, comparative view.
concerns total FDI, while is about FDI by sector of activity, and finally regards FDI by technological content. No further detailed comment is provided here as these results were amply illustrated earlier on in the text.
This evidence shows that FDI affects growth by playing its role as a vehicle for technology transfers to the host country. However, once the technological content drops, as for the intermediate categories of MHT FDI Sec and MLT FDI Sec, we cannot find a statistically significant positive relationship with growth. Clearly, with respect to these FDI types, the technology spillover involved is weaker and perhaps not sufficient to create that positive association between FDI and economic growth. Even more striking is that the positive relationship between FDI and growth resurfaces when a flow of LT FDI Sec is involved.
To explain the U-shaped relationship that emerges in our study between different FDI types and economic growth, we conjecture the following role for technology: different FDI types seem to be carriers of different effects. The positive effects probably include technology spillovers that can be interpreted broadly as inclusive of new managerial skills and novel production processes. These effects will work most strongly when FDI is characterized by high technological content.Footnote21 The spillover effects may become weaker as the technological content decreases, allowing for other, perhaps more negative influences to prevail. For medium-high and medium-low tech FDI, foreign investment carried out by large multinational enterprises may crowd out existing smaller local firms in at least two ways. First, the larger multinational firms, by exploiting their larger size, enjoy scale economies that can raise entry barriers, which in turn change the competitive structure of the market in which they enter. The new, higher barriers to entry may push pre-existing local firms out of business and may discourage new ones from entering the market.Footnote22 Second, the multinational firms may also be able to obtain privileged access to credit, particularly if local credit markets are less developed and more shallow. As a result, local firms may find it more difficult to finance their business.Footnote23 These two factors, higher barriers to entry requiring more funding and rationed access to credit, which reduces funding, jointly have the potential to crowd local firms out of the market in which they operate after the entry of larger multinational enterprises. If a sufficient number of local firms succumb, the net effect of foreign direct investment on the growth rate of the recipient economy may become unclear or even negative.Footnote24 However, when its technological content is lowest, we again find strong evidence of a positive impact of FDI on the EG of the host country. Often low-tech FDI is of the efficiency-seeking type, and it is likely to be labour-intensive: For this reason its goal may be to take advantage of the availability of cheaper labour in the recipient country. FDI in the textile sector is one such example. To give this conjecture some footing in economic theory, we invoke a standard Heckscher-Ohlin argument here: the arrival of labour-intensive low-tech FDI would help disproportionately expand the production possibilities of labour-abundant host countries thereby explaining its positive impact on the EG of these countries.Footnote25 In order to further verify and strengthen our results, we also run a regression of low-tech FDI alone on growth (keeping all the other FDI types out) and found a positive and significant impact of low-tech FDI on growth in the GMM model for the non-zero baseline case.Footnote26
The above discussion clarifies that FDI is not all equal and differentiating it by technological content is crucial. Both high tech and low tech FDI hold the highest promise of fostering economic growth in the host economy. Policy makers of countries interested in attracting FDI should strive to focus on the high technology and low technology sectors and give less encouragement to investment flowing into medium-high-tech and medium-low-tech sectors.
VII. Conclusions and suggestions for future work
This study exploits the increasing level of detail in FDI data that is slowly but steadily becoming available to bring to the fore the role played by technology in the FDI-EG relationship. Despite all the efforts and a very large body of literature, a certain amount of controversy still lingers on about the existence and direction of causality in this relationship. However, the contribution of this study is rather clear: FDI types are not all equal in affecting the economy of the host country. Indeed, a particularly effective way to reveal the heterogeneity of different FDI types in this respect is to classify them by technological content and track the different impact each type has on the EG of the host country. In this respect, our results do not leave room for much doubt: technology plays a crucial role in the growth-fostering function of FDI. This study shows that this result is robust across different estimation techniques as well as across different sampling strategies. It is shown that the conclusions reached on the matter did not change whether GMM estimation or FE estimation was used. Likewise, conclusions were the same whether countries with zero values were dropped (baseline case) or not. We also found that low-tech FDI fosters EG too though probably through different channels.
The results have important policy implications. However, though pursuing GDP growth is important, there is now a broad consensus that this is not the only measure of the well being of countries. In this regard, the United Nations has produced a list of sustainable development goals (SDG). Specifically, when devising policies geared towards attracting FDI, policymakers around the world should ensure that the effects of inward FDI on the recipient countries are in line with the United Nation’s Sustainable Development Goals 8 and 9. SDG 8 calls for inclusive and sustainable economic growth, while SDG 9 is to build resilient infrastructure, promote inclusive and sustainable industrialization and foster innovation United Nations (Citation2015). Policies designed to achieve inclusive and sustainable growth would bring important societal impacts.
This study can be extended in a number of ways. As more data become available, the sample size can be broadened. This step allows us to focus on specific geographical areas or income groups besides Europe and the high-income countries as already done here.
Another possibility would be to compare and contrast the short run and the long-run effects of different FDI types on EG. Finally, it would be interesting to probe if there is a nexus between the various FDI technological categories and the country of origin of these FDI types.
Disclosure statement
No potential conflict of interest was reported by the author(s).
Notes
1 Although flows of capital experienced a drop since 2016 for developed countries, transitional and developing economies continued to enjoy an upward trend in FDI flows until 2019.
2 If there is any causality between the two, it can run both ways and there are multiple channels through which this causality may manifest itself (see, e.g. Almfraji and Almsafir, Citation2014, for a literature review. For causality from EG to FDI, see, e.g. Lamsiraroj and Doucouliagos (Citation2015).
3 Seminal papers on threshold effects in the FDI – EG relationship are Borenzstein et al. (Citation1998) for human capital, Alfaro et al. (2008) for financial development and Kose et al. (Citation2009) for both financial and institutional development in the broader context of the relationship between financial integration and EG. Khalid and Marasco (Citation2019) investigate if the impact of FDI on growth is dependent on the channel of integration used to attract FDI.
4 Alfaro (Citation2003) is the pioneer study in this respect. Other relevant references are given in Section 2 on the literature review.
5 While decomposing FDI by type, we noted that the data on FDI/GDP ratios include several zero values, particularly with respect to the FDI with high technological content. Hence, in the main analysis we used a sub-sample of countries by removing those with zero values for the variable of concern. We re-estimate the model by using a full sample for consistency check.
6 A more complete discussion of these arguments is given in Section 5.2.
7 Another major distinction in FDI is horizontal versus vertical. Horizontal FDI is where multi-plant firms duplicate roughly the same activities in multiple Countries. It is believed that the bulk of FDI is horizontal rather than vertical.
8 Penn World Table 9.1
9 Indeed, in all other equations throughout this article too, the vector of control variables is always preceded by a vector of coefficients.
10 The value of the chi-square test statistic (with 7 degrees of freedom) was 67 (with p-value = 0.000) for the annual regression of equation 1. Thus the null hypothesis that the random model is appropriate is rejected. The Hausman test was also carried out for the 5-year average regressions of equation 8 (see below). Test results (not reported but available upon request) confirmed the fixed effect model as the appropriate choice in that case too.
11 Marasco (Citation2021) takes a preliminary look at the data using this disaggregation and as such constitutes a partial exception.
12 Law and Singh (Citation2014) and Arcand et al. (Citation2015) found similar empirical evidence.
13 This is because while FDI data worldwide have now been collected more or less systematically for a very broad cross section of countries for at least 30 years, and they are readily accessible from sources such as the UNCTAD, data collection on FDI differentiated by sector of industry has been much more scattered and hard to come by.
14 As explained in the document “Human Capital in PWT 9.0”.
15 Please see Section 4 for description and discussion of the model used and the control variables included in this study..
16 In general, it is true that small N can introduce a sample bias in GMM estimation. This will depend on whether N is big enough to accommodate all relevant instruments. In our case N = 28 and we have 14, 16, 20 and 18 instruments respectively in our regressions. To further address this concern, we also tested the robustness of our results by shortening T to less than N (from 31 to 25 by starting the sample from 1995 instead of 1989) and found no change in our results. We also run fixed effects regressions for all four specifications and the results were in line with GMM.
17 We also performed some additional tests to ensure that model estimates are valid. First, we conducted VIF tests for multicollinearity for all four models. These results are reported in Appendix table B3. These results do not suggest any multicollinearity. Second, we performed panel unit root tests to check any possible stationarity. Again, the results, in general, reject non-stationarity. These results are reported in Appendix table B4.
18 See footnote 21.
19 As a further robustness check, we also grouped data into 5-years intervals and then re-estimated the model using both GMM and fixed effects regressions for equations (1) through (4) on the corresponding averages. For the sake of brevity, we do not report these results in the article. However, these results are available from corresponding author upon request. It is important to note, though, that grouping the data into 5-years average significantly reduced the number of observations and the power of the tests. Nonetheless, the results confirm the results obtained from the annual regressions. In particular, after we disaggregate data by technological content (equation 3a), we have confirmation that, in the manufacturing sector, the positive association between FDI at both ends of the technology spectrum (high and low) and EG remains significant, at the 1% and 5% levels respectively, while the two intermediate categories of FDI (medium-high-tech and medium-low-tech) remain statistically insignificant.
20 Almfraji and Almsafir (Citation2014). Also see Forte and Moura (Citation2013).
21 Borenzstein et al. (Citation1998) and De Mello (Citation1997) are some of the early seminal articles on technology spillovers of FDI. For more recent contributions, see literature review by Shushu Feng (Citation2020).
22 For example, Kokko and Thang (Citation2014) find evidence of crowding-out while documenting the impact of FDI on the survival of domestic private firms in Vietnam.
23 Harrison and McMillan (Citation2003) in a study centered on Ivory Coast, a country with market imperfections, find that local firms are more credit-constrained than foreign firms and that borrowing by foreign firms exacerbates the credit constraints of domestic firms
24 Marasco (Citation2021) contains the same discussion of these effects .
25 Nunnenkamp and Spatz (Citation2003) is an example of an earlier study advancing this kind of argument.
26 We run individual regressions for the other FDI types too which confirmed the results of . These results are reported in Appendix table B10.
References
- Alfaro, L. 2003. Foreign Direct Investment and Growth: Does the Sector Matter?. Harvard Business School Mimeo, Harvard. http://www.grips.ac.jp/teacher/oono/hp/docu01/paper14.pdf
- Alfaro, L., and A. Charlton, 2007. “Growth and the Quality of Foreign Direct Investment: Is All FDI Equal?.” CEP Discussion Papers dp0830, Centre for Economic Performance, LSE.
- Almfraji, M. A., and M. K. Almsafir. 2014. “Foreign Direct Investment and Economic Growth Literature Review from 1994 to 2012.” Procedia – Social and Behavioral Sciences 129: 206–213. doi:10.1016/j.sbspro.2014.03.668.
- Anghel, B. 2006. “Foreign Direct Investment in Transition Countries: A Dynamic Analysis at Firm Level.” Universidad Autonoma de Barcelona, April available at https://citeseerx.ist.psu.edu/viewdoc/download?doi=10.1.1.408.6849&rep=rep1&type=pdf.
- Arcand, J. L., E. Berkes, and U. Panizza. 2015. “Too Much Finance?” Journal of Economic Growth 20: 105–148. doi:10.1007/s10887-015-9115-2.
- Arellano, M., and S. Bond. 1991. “Some Tests of Specification for Panel Data: Monte Carlo Evidence and an Application to Employment Equations.” The Review of Economic Studies 58 (2): 277. doi:10.2307/2297968.
- Ayhan Kose, M., E. S. Prasad, and A. D. Taylor. 2011. “Thresholds in the Process of International Financial Integration.” Journal of International Money and Finance, Elsevier 30 (1): 147–179.
- Beugelsdijk, S., R. Smeets, and C. J. Z. Remco. 2008. “The Impact of Horizontal and Vertical FDI on Host’s Country Economic Growth.” International Business Review 17 (4): 452–472. doi:10.1016/j.ibusrev.2008.02.004.
- Borensztein, E., J. Gregorio, and J. Lee. 1998. “How Does Foreign Direct Investment Affect Economic Growth?” Journal of International Economics 45 (1): 115–135. doi:10.1016/S0022-1996(97)00033-0.
- Chakraborty, C., Nunnenkamp, and P. Nunnenkamp. 2008. “Economic Reforms, FDI and Economic Growth in India: A Sector Level Analysis.” World Development 36 (7): 1192–1212. doi:10.1016/j.worlddev.2007.06.014.
- De Mello, L. R., Jr. 1997. “Foreign Direct Investment in Developing Countries and Growth: A Selective Survey.” The Journal of Development Studies 34 (1): 1–34. doi:10.1080/00220389708422501.
- Doytch, N., and M. Uctum. 2019. “Spillovers from Foreign Direct Investment in Services: Evidence at the Sub-Sectoral Level for the Asia-Pacific.” Journal of Asian Economics 60 (1): 33–44. doi:10.1016/j.asieco.2018.10.003.
- Feng, S. 2020. “Technology Transfer Spillover from FDI-A Comprehensive Literature Review, Proceedings of the 4th International Symposium on Business Corporation and Development in South-East and South Asia Under B&R Initiative (ISBCD 2019), Series: Advances in Economics.” Business and Management Research, Atlantis Press. Can be retrieved from https://www.atlantis-press.com/proceedings/isbcd-19/125941798
- Forte, R., and R. Moura. 2013. “The Effects of Foreign Direct Investment on the Host Country Economic Growth —theory and Empirical Evidence.” The Singapore Economic Review 58 (3): 1–28. doi:10.1142/S0217590813500173.
- Gönel, F., and T. Aksoy. 2016. “Revisiting FDI-Led Growth Hypothesis: The Role of Sector Characteristics.” Resource Policy 25 (8): 1144–1166. doi:10.1080/09638199.2016.1195431.
- Harrison, A., and M. McMillan. 2003. “Does Direct Foreign Investment Affect Domestic Credit Constraints?” Journal of International Economics 61: 73–100. doi:10.1016/S0022-1996(02)00078-8.
- Hatzichronoglou, T. 1997. “Revision of the High-Technology Sector and Product Classification.” OECD Science, Technology and Industry Working Papers, 1997/2, OECD Publishing.
- H, W. W., P. Crits-Christoph, R. Gallop, W. Tang, D. Chen, and X. Tu. 2014. “On the Implication of Structural Zeros as Independent Variables in Regression Analysis: Applicatoin to Alcohol Research.” Journal of Data Science 12: 439–460.
- Iamsiraroj, S., and H. Doucouliagos. 2015. “Does Growth Attract FDI?” Economics: The Open-Access, Open-Assessment E-Journal 9 (2015–19): 1–35. doi:10.5018/economics-ejournal.ja.2015-19.
- Ingham, H., R. Read, and S. Elkomy. 2019. “Aggregate and Heterogenous Sectoral Effects of Foreign Direct Investment in Egypt.” Review of Development Economics 24 (4): 1–18. doi:10.1111/rode.12698.
- Jana, S. S., T. N. Sahu, and K. D. Pandey. 2019. “Foreign Direct Investment and Economic Growth in India: A Sector-Specific Analysis.” Asia Pacific Journal of Management Research and Innovation 15 (1–2): 53–67. doi:10.1177/2319510X19849731.
- Khalid, A. M. A., and A. Marasco. 2019. “Do Channels of Financial Integration Matter for Fdi’s Impact on Growth? Empirical Evidence Using a Panel.” Applied Economics 51 (37): 4025–4045. doi:10.1080/00036846.2019.1588945.
- Kokko, A., and T. T. Thang. 2014. “Foreign Direct Investment and the Survival of Domestic Private Firms in Viet Nam.” Asian Development Review 31 (1): 53–91. doi:10.1162/ADEV_a_00025.
- Law, S. H., and N. Singh. 2014. “Does Too Much Finance Harm Economic Growth.” Journal of Banking & Finance 41 (C): 36–44. doi:10.1016/j.jbankfin.2013.12.020.
- Mani, S. 2000. “Exports of High Technology Products from Developing Countries: Is It Real or a Technological Artifact?.” U.N.U.-INTECH Discussion Paper Series, #2000-1
- Marasco, A. 2021. “Foreign Direct Investment, Technology and Economic Growth: What the Data Say.” Ekonomi-tek 10 (2): 89–110. https://dergipark.org.tr/en/pub/ekonomitek/issue/65446/959481
- Massoud, N. 2008. FDI and Growth in Emerging Markets: Does Sectoral Distribution Matter? – the Case of Egypt, (Cass WP-EMG 05-2008). London: Cass Business School.
- Nunnenkamp, P., and J. Spatz 2003. “Foreign Direct Investment and Economic Growth in Developing Countries: How Relevant are Host-Country and Industry Characteristics?.” Kiel Working Paper 1176, Kiel University.
- OECD. 2003. OECD Science, Technology and Industry Scoreboard 2003. Paris: OECD Publishing.
- OECD. 2011. “OECD Science, Technology and Industry Scoreboard 2011, OECD Publishing, Paris. Penn World Table 9.0.” http://ghdx.healthdata.org/record/penn-world-table-version-91
- Sanchez-Martin, M. E., J. De Pinies, and A. Kassia 2015. “Measuring the Determinants of Backward Linkages from FDI in Developing Economies: Is It a Matter of Size?.” Policy Research Working Paper 7185, World Bank Group, January.
- Shah, S. H., H. Hasnat, S. Cottrell, and M. H. Ahmad. 2019. “Sectoral FDI Inflows and Domestic Investment in Pakistan.” Journal of Policy Modeling 42: 96–111. doi:10.1016/j.jpolmod.2019.05.007.
- UNCTAD. 2021. “World Investment Report 2015.” Geneva: UNCTAD. accessed November 20 2018. https://unctad.org/en/PublicationsLibrary/wir2021_en.pdf
- United Nations. 2015. “Sustainable Development Goals.” accessed 18 February 2023 https://sdgs.un.org/goals
- UNSD. 2021. International Standard Industrial Classification of All Economic Activities (ISIC) Revision 3. New York, USA: United Nations Statistics Department. https://unstats.un.org/unsd/classifications/Family/Detail/2.
- Wang, M. 2009. “Manufacturing FDI and Economic Growth: Evidence from Asian Economies.” Applied Economics 41: 991–1002. doi:10.1080/00036840601019059.
Appendix A
Table A1. List of countries.
Appendix B
Table B1. Summary statistics.
Table B2. Correlation table (full correlation data – inclusive of control variables).
Table B3. Variance inflation factor.
Table B4. Panel unit root test results.
Table B5. Fixed effects in non-zero countries.
Table B6. Dynamic system GMM full sample.
Table B7. Dynamic system GMM high-income countries.
Table B8. Fixed effects (full sample).
Table B9. Fixed effects high-income countries.
Table B10. Secondary sector FDI classified by technology-dynamic system GMM (non-zero countries).