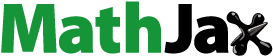
ABSTRACT
This paper presents an empirical investigation on the determinants of workplace accidents across Europe and focuses on the extent to which production-system characteristics (employment sectoral risk, size of firms, temporary contracts), business cycle and socio-economic factors (GDP, level of investments, unemployment, education) and other territorial controls (crime index) might account for cross-country heterogeneity. We use Eurostat data, and our panel is composed of 27 European countries over the period 2010–2018. Implementing different functional forms and estimation methodologies (pooled OLS, panel fixed and random effects models, system-GMM and semiparametric fixed effects model), we find robust evidence that productive-system structural characteristics, business cycle controls and the other territorial variables are effective in explaining European cross-country heterogeneity. Moreover, we find evidence of a nonlinear relationship between GDP and occupational accidents.
I. Introduction
Workplace safety is a relevant issue that has been increasingly attracting the attention of institutions, labour organizations, researchers, and policymakers (European Commission Citation2002, Citation2007; Greer Citation2018; da Silva and Amaral Citation2019; Liu Citation2019; Schulte et al. Citation2019; Tassinari, Chesta, and Cini Citation2020). In Europe, even though the number of occupational injuries shows a decreasing trend, cross-country differences in workplace accident rates show a heterogeneous dynamic mainly related to differences in definitions and measurement (Boone and van Ours Citation2006; Anyfantis, Boustras, and Karageorgiou Citation2018; Ivascu and Cioca Citation2019; Lafuente and Daza Citation2019; Verra et al. Citation2019). In Italy, from 2008 to 2019, the highest percentage of work accidents occurred in those sectors that are typically considered particularly at risk and that employ vulnerable workers (low-skilled, low-paid, low-educated), such as manufacturing industries, construction, trade, and transport activities (Farina et al. Citation2018; Lombardi, Fargnoli, and Parise Citation2019; De Merich et al. Citation2022). Even though, over the past few years, the total accidents in Italy, like in the other European countries, has decreased (Hämäläinen, Saarela, and Takala Citation2009)Footnote1, the work-related risks, however, have not been reduced in a uniform way leaving some categories of workers, companies and sectors overexposed to workplace risks (Cioni and Savioli Citation2016; Giraudo et al. Citation2018; Zambon et al. Citation2018; D’Ambrosio, Leombruni, and Razzolini Citation2022). In Italy, for instance, Central-Northern provinces have a higher proportion of employment in industrial sectors (in comparison to the Southern regions) which pose a higher risk of work accidents/injuries.
The frequency of workplace accidents has been extensively studied in the literature for which, traditionally, it is possible to identify four main groups of factors affecting injuries (see Fabiano, Currò, and Pastorino Citation2004): i) individual factors related to workers characteristics (age, gender) and experience, ii) job-related factors, organization of work and environmental conditions, iii) technology used, and iv) economic factors, such as general economic conditions, unemployment rate, labour and social insurance legislation, business cycles. Cornelissen, Van Hoof, and De Jong (Citation2017) have provided one of the most comprehensive overviews on the determinants of occupational safety, identifying and clustering several possible determinants of occupational injuries in high-risk industries (i.e. construction, petro-chemistry, warehouses, and manufacturing). The resulting seven clusters constitute a suitable framework to identify the possible determinants of workplace accidents since Cornelissen, Van Hoof, and De Jong (Citation2017) considered both theoretical and empirical studies and included determinants which have received so far little attention in previous models (e.g. external factors).
More recently, Theorell (Citation2022) has edited the Handbook of Socioeconomic Determinants of Occupational Health, collecting a robust evidence on key issues in occupational health research, considering both a macro and a micro-level perspective. Specifically, at macro level, working conditions are put in relation to political change, gender, age, precarious employment, national economy, and retirement. At micro level, instead, the analysis focuses on a wide array of factors, ranging from the social patterns in distributions of psychosocial and physical risks at work in general to physical and socioeconomic issues as noise, shift work, under/overemployment, occupational physical activity, job intensity, digitization in modern work, climate change, childhood determinants of occupational health in adult years, and theoretical models currently used in occupational epidemiology (Theorell Citation2022).
As emphasized by Cioni and Savioli (Citation2016), workplace accidents are the result of a process that involves different and multifaceted determinants; our work aims to enrich the literature by investigating the key macroeconomic determinants of workplace accidents, both at European and Italian levels, in an attempt to document the relationships between occupational accidents and economic, territorial and production-system factors affecting workplace risk. Differences in occupational safety and health (OSH) performances are not likely to be the result of firms’ intrinsic characteristics alone, but rather of their interaction with social, political, regulatory, and economic contexts which are therefore investigated.
Based on this strand of literature, the aim of the paper is twofold. First, grounding on a model specification that combines productive system characteristics and socio-economic controls, our empirical analysis investigates, at a macro level of analysis, the main determinants of occupational accident rates, aiming to disentangle European cross-country heterogeneity, within the business cycle theoretical framework. Secondly, we deepen the study of the relationship between the European country heterogenous growth trends and occupational accidents, investigating the existence of a nonlinear relationship between accidents’ rate and per capita GDP increases, for which the accidents’ rate might first grow and then fall. Our empirical analysis relies on a panel dataset composed by 27 European countries, for a time span of 9 years (2010–2018), and we employ alternative econometric approaches (OLS pooled, panel random- and fixed-effects model, system-GMM and semiparametric fixed effects model). The remainder of the manuscript is organized as follows. In section II, we introduce the data, while section III presents our econometric methodology. Estimation results are presented and discussed in section IV. Section V outlines our conclusions and policy implications.
II. Data
Our panel dataset is composed by 27 European countries observed for 9 years (from 2010 to 2018). The data are provided by Eurostat, both for the dependent variable and the independent variables, which are described in detail in the following sections. briefly illustrates the main characteristics of the quantitative analysis, in which the occupational accident rate is modelled as the dependent variable of an array of regression models involving several regressors (see ). Specifically, the panel analysis focuses on 27 OECD countries and a time span of 9 years (from 2010 to 2018). In the reminder of this Section, we briefly illustrate the dependent variable and the selection of independent variables used in the panel analysis.
Table 1. An overview of the quantitative analysis.
Table 2. Independent variables description.
Dependent variable
The dependent variable in the regression model is measured as the incidence rate of workplace accidents resulting in the number of serious accidents per 100,000 persons in employment. In particular, the numerator is the number of accidents that occurred during the year; the denominator is the reference population (i.e. the number of persons in employment) expressed in 100,000 persons. An accident at work is defined as a discrete occurrence in the course of work which leads to physical or mental harmFootnote2 If the accident does not lead to the death of the victim, it is called a ‘non-fatal’ (or ‘serious’) accident, and it is intended involving more than three calendar days of absence from work.
shows the average percentage rate of change in OA from 2010 to 2018 for the 27 countries of our panel.
Aware of the phenomenon of underreporting, the purpose of this indicator is not to give a picture of OSH performance in the countries illustrated, but to provide a descriptive statistical representation of which countries experience a reduction or an increase in the change of OA rates. Based on the evidence, Lithuania, Romania, Latvia, France, Slovakia and Croatia are the countries with the largest average positive percentage change (increase) between the previous and following years among the 27 countries considered. The other countries with an average increase in OAR from 2010 to 2018 are Luxembourg, Hungary, Portugal and Ireland. The countries, on the other hand, with the largest negative percentage change (reduction) rates are Norway, Italy, Czech Republic, Denmark, Netherlands and United Kingdom. Greece, Slovenia, Austria, Finland, Bulgaria, Spain, Estonia, Germany, Poland and Sweden, although to a lesser extent, still exhibit a negative average percentage change from the previous to the following year.
Independent variables
We include a set of explanatory variables, which are divided in three macro-categories: production-system, socio-economic, and other territorial variables. The independent variables were chosen according to those most commonly used in the economic literature on workplace safety, accident and injury research, and they are described below. summarizes them.
Production-system variables
A substantial part of the literature focuses on the relationship between workplace accidents (non-fatal) and types of worker contracts Lopez et al. (Citation2008). Dupré (Citation2001) found that, in European countries, the risk of accidents for temporary workers who had been employed for less than two years was particularly high in the construction, health and social sectors. Fabiano et al. (Citation2008) using Italian data for the period 2000–2004, show that workers employed by temporary-help agencies suffer a higher injury frequency index (compared to employees that are directly hired) due to lack of experience, insufficient specific knowledge, and inadequate training. Virtanen et al. (Citation2005) found that temporary workers may have a higher risk of psychological morbidity and work-related injuries as compared to permanent workers. However, Benavides et al. (Citation2006) found that, even though temporary workers seem to have a higher risk for work-related injuries than permanent workers, after controlling for the length of employment the probability of accidents is quite similar in both groups. The firm size is also considered a relevant variable able to exert a significant impact on the level of occupational risk (Fabiano, Currò, and Pastorino Citation2004) finding an inverse correlation between the frequency index of workplace accident and the firms’ size.
In literature, a great deal of agreement exists on the type of economic sector which plays a determining role in occupational accident risk levels: this is confirmed by the fact that the differences, between sectors, found in this respect persist even when controlling for heterogeneity between clusters of countries (Lenaerts et al. Citation2022). The sectoral perspective is relevant particularly for studying the risks associated to the physical environment (Leigh et al. Citation1990; Leigh Citation1989; Maiti and Bhattacherjee Citation1999; Maiti, Bhattacherjee, and Bangdiwala Citation2001; Maiti, Chatterjee, and Bangdiwala Citation2004; Haslam et al. Citation2005; Khanzode, Maiti, and Ray Citation2012, Citation2011), which depend on the production process, the materials used, the equipment endowment, as well as the activities carried out during the work activity (Eurofound Citation2012; Wadsworth and Walters Citation2014; Lenaerts et al. Citation2022); for those employed in manual work, physical health risks remain the strongest predictor of injuries (Kubicek, Bhanugopan, and O’Neill Citation2019; Toch et al. Citation2014).
Intersectoral differences in terms of activities and tasks performed by workers are also directly related to the occupational structure of each sector. In particular, sectors dominated by blue-collar employments, such as construction, agriculture, industry and transport, typically have less secure physical environments; in contrast, sectors dominated by white-collar occupations, such as financial services, education and public administration, have better levels of physical environment (Lenaerts et al. Citation2022). Therefore, while sectors dominated by occupations with a higher level of education and generally higher labour income are subject to OSH risks more related to psychological factors (such as stress, and anxiety), the blue-collars are exposed to higher accidents’ risk (Dorman Citation2000).
Socio-economic variables
Since the pioneering study by Kossoris (Citation1938) on the relationship between occupational accidents and the business cycles, several studies (Ussif Citation2004; Davies, Jones, and Nuňez Citation2009; Fortin, Lanoie, and Laporte Citation1996) have found a pro-cyclical relation between the business cycle and the occupational injuries rates, showing that the number of accidents tends to increase during economic upswings and vice versa (Robinson Citation1988; Nichols Citation1991; Fabiano et al. Citation1995); periods of economic slow-downs may accompany a reduction in the number of injuries. Furthermore, macroeconomic indicators, such as gross domestic product (Asfaw, Pana-Cryan, and Rosa Citation2011) or unemployment rate (Brooker, Frank, and Tarasuk Citation1997) have been primarily used to study their respective incidence on occupational accidents. Other economic characteristics such as unemployment rate and labour and social-insurance legislation (Blank, Diderichsen, and Andersson Citation1996) are also considered relevant variables. Some authors have suggested that workers tend to underreport minor injuries during a recession (Boone and van Ours Citation2006), which reduce the official number of minor injuries that they report in times of crisis.
Territorial variables
The number of thefts is taken as a proxy for the level of crime within a given country. The underlying hypothesis is that a higher level of crime is related to lower OSH compliance by firms. Regulation and compliance might have an impact on OSH levels and this is demonstrated in several empirical studies. Viscusi (Citation1986), for instance, estimates a statistically significant impact of OSH regulation on lost working days due to accidents. Weil (Citation1996), on the other hand, investigates the effect of regulation on firm compliance, as measured by the number of violations of expected standards. In particular, the work investigates the effect of inspections – one of the main control instruments in OSH regulation – which impose fines and penalties for violations. One of the main outcomes of this study shows that compliance decisions would be made on the basis of potential, rather than actual, penalties. Concerning the role of the sequence and frequency of inspections on the compliance of firms with OSH regulations, Ko, Mendeloff, and Gray (Citation2010) find that from the first to the second inspection there is at most a 31% drop in the number of violations (i.e. higher level of compliance), with a smaller drop in the case of serious violations; furthermore, the effect of repeat inspections on serious violations is greater in planned inspections than in other types of inspections.
provides an overview of the selected variables and their summary statistics. At first glance, the data are characterized by a high variability.
Table 3. Descriptive statistics.
The research assumption is that work safety is a complex phenomenon influenced by both micro (individual-related, job-related) and macroeconomic factors (GDP, unemployment), which should be analysed comprehensively. Our main contributions are: i) to extend the literature in this vein by understanding the main macroeconomic determinants of the incidence of occupational accidents; and ii) to test the existence of a non-linear relationship between the occupational accident rate and the GDP level, while controlling for production-system characteristics and unobserved heterogeneity. On this background, we test for two main hypotheses:
-Hp1: Territorial heterogeneity of workplace accidents, at both European and Italian level, can be explained by recurring to a macro level of analysis, within the business cycle theoretical framework;
-Hp2: The relationship between workplace accidents and the GDP level is complex (de La Fuente et al. Citation2014; Asfaw, Pana-Cryan, and Rosa Citation2011) and we may expect that it takes a non-linear (and non-monotonic) relationship. Indeed, occupational accidents rate may increase with economic wealth due, for instance, to the higher probability of reporting more injuries (Boone and van Ours Citation2006; Davies, Jones, and Nuňez Citation2009), or to the share of new or inexperienced workers in the total labour force increases during economic expansions (Boone and van Ours Citation2006; Robinson Citation1988); however, the relationship between workplace accidents and GDP may invert after reaching a certain wealth threshold, while controlling for production-system, institutional and macroeconomic characteristics. Generally speaking, countries with higher per capita income may exhibit higher levels of health and safety workplace measures and regulations, not only because firms will tend to pay more attention to the workers’ safety conditions (i.e. healthier and safer workers can work more efficiently and with higher labour productivity) but also because workers might have a higher perception of the potential risks associated with the probability of occurrence of workplace accidents. One view (Bonamore, Carmignani, and Colombo Citation2015) is that in time of economic downturn and rising unemployment, tighter firms’ budget constraints might imply cuts in workplace safety-related equipment and measures. Economic expansions are, instead, typically characterized by declining unemployment, improved labour market conditions with higher expenditures on health and safety workplace measures. The quality of the workplace systems might, therefore, improve because of the positive effects that economic upturns have on the investment capacity of the firms. If the non-linear relationship is confirmed, then the GDP coefficient will be positive and the coefficient associated to the square of the GDP variable will be negative. If our hypothesis is correct, then, at aggregate level, we should observe an inverted U-shaped relationship: at lower levels of income, an increase of per capita GDP might initially be associated with an increase of occupational accident rates; but when the level of income is sufficiently high, then further increases of per capita GDP will decrease occupational accidents rate. To the best of our knowledge, the previous literature does not provide any evidence on the existence of this potential non-linearity in estimating the determinants of workplace accidents at macro level; thus, the present study is oriented to fill this gap.
III. Econometric methodology
Since Hartwig’s et al. (Citation1997) cross-country analysis, an extensive stream of literature has deepened the relationship between workplace accidents (or workers compensations) and the business cycle. The main insight in the background of these studies is that macro-economic trends can affect the drivers and frictions of occupational accidents. To substantiate this hypothesis several empirical works (Hartwig et al. Citation1997; Brooker, Frank, and Tarasuk Citation1997; Ussif Citation2004; Boone and van Ours Citation2006; Davies, Jones, and Nuňez Citation2009; Asfaw, Pana-Cryan, and Rosa Citation2011) find evidence of a correlation between occupational accidents rates and major macro-economic indicators (GDP, fixed investments, unemployment rate).
In order to analyse the heterogeneity of the occupational accident phenomenon at European level (Hp1), this paper aims to extend this literature by including, besides to the main business cycle indicators, also the productive system and institutional characteristics of the EU-27 countries considered in our panel. In this context, we begin by testing the presence of a link between the annual occupational accidents and the main countries’ productive characteristics, controlling for business cycle indicators and institutional characteristics. The OLS estimation equation takes the following log-log form:
where the subscripts i and t represent, respectively, the country and the time period. All variables are expressed in natural logarithms. OA is the number of occupational accidents rate measured as the number of serious accidents per 100.000 persons in employment, X is a vector of countries’ productive system characteristics (employment risk, large firms, temporary contracts), Z is a vector of business cycle controls (per capita GDP, secondary education, fixed investments over GDP, unemployment), and U is a vector of other territorial variables (crime index). Finally, T and FE are, respectively, years and geographical fixed effects, ε is the time-varying error term which stands for a well-behaved error term distributed IID (0, σ2).Footnote3
This first approach provides the estimates of the pooled OLS, and the panel fixed (FE) and random (RE) effects models. In order to choose between the fixed and random estimate, both the Breusch-Pagan Lagrange Multiplier Test (1980) and the Hausman Test (Hausman Citation1978) reveal that the fixed effects model is the most appropriate one. The risk of hysteresis in the perpetration of accidents at work has raised the attention on the phenomenon that todays’ occupational accidents rate can be related to previous ones. Countries past occupational accidents may indeed affect current workplace injuries. For instance, a countries’ occupational accident rate in the previous year can explain the level of adherence to occupational safety regulations in the previous year, which in turn can explain a low standard in occupational safety policy enforcement that can determine in the following year a higher level of occupational accidents.
For these reasons, the relevance of a dynamic path associated to occupational accidents rates suggests that OLS coefficients could be inconsistent due to the correlation of OAt-1 and the error term, even implementing a first difference approach. Therefore, we complement the standard panel approach with a dynamic panel data analysis (Arellano and Bond Citation1991; Arellano and Bover Citation1995; Blundell and Bond Citation1998). With the implementation of an auto-regressive approach, we are able to enhance the accuracy of the OLS estimates and to include the entire history and transition over time of our model.
In our case, given that the panel units are larger with respect to the time periods, we opt for the system-GMM estimator (Arellano and Bover Citation1995) that is more efficient (Baltagi Citation2005) than the difference-GMM estimator (Arellano and Bond Citation1991). Our choice is also confirmed by comparing the magnitude of the coefficients of the lagged dependent variable from the difference- and system-GMM, with those obtained from the pooled OLS and the panel fixed effects (P-FE).
We test Hp1 by also dealing with the dynamic and simultaneity problems arising by the hysteresis of occupational accidents. In particular, we estimate the baseline specification model in EquationEquation (1)(1)
(1) through a system-GMM (Arellano and Bond Citation1991; Arellano and Bover Citation1995) by also including on the right-hand side the lagged dependent variable. The system-GMM estimation takes the following equation form:
All variables are expressed in natural logarithms. The subscripts i and t represent the country and the time period, respectively. On the right-hand side of EquationEquation (2)(2)
(2) , the explanatory variables are the same of previous EquationEquation (1)
(1)
(1) plus the lagged dependent variable (OAt-1) which was included into the model in order to identify the persistency in the dynamics of occupational accidents; ηi is a country fixed effect and ξt a time fixed effect; εit stands for a well-behaved error term distributed IID (0, σ2).
The system-GMM estimator (Arellano and Bond Citation1991; Arellano and Bover Citation1995) controls for both time-invariant country-specific effects and endogeneity criticalities that arise from the lagged value of occupational accidents rate. The validity of the instruments used are tested by the Sargan test of over-identifying restrictions to examine the consistency of the instruments and by the Arellano and Bond (Citation1991) test for the serial correlation of the disturbances up to the second order.
In the subsequent stage of the analysis, we provide an insight on the link between occupational accidents and economic growth by testing the existence of a non-linear and non-monotonic relationship (Hp2). We believe that advancements in technology (i.e., Industry 4.0) can provide solutions able to enhance workers’ health, safety, and wellbeing, enabling the workforce to shift from low- to high-productivity sectors. Thus, countries that have exploited new technologies displace, with respect to the others, higher levels of GDP potentially justifying a cross-country inverted-U-shaped relationship between OA and GDP. Our hypothesis, therefore, is that an increasing GDP can improve social welfare and the overall security of a society; however, because of the different development stages of one country, the economic and technological structures will be different, and their impacts on occupational accidents may also be different, depending on whether occupational safety and health requirements are regarded as necessary, normal or luxury goods. In this perspective, we start by estimating through pooled OLS, fixed and random effects model the following equation:
where, all variables are again in natural logarithm and i and t represent the country and the time period, respectively. The other elements of the equations have been set out above and are self-explanatory.
We then estimate EquationEquation (3)(3)
(3) by recurring to the following system-GMM estimation:
Finally, in order to ascertain the robustness of our GDP quadratic specification, we also implement the alternative two-stage semiparametric fixed effects additive model (Baltagi and Li Citation2002):
Where unlike previous models, GDP enters the model nonlinearly. All variables are again expressed in natural logarithms. The specified additive model is built in order to satisfy the stochastic equicontinuity condition and is pointwise asymptotically normal. Thus, it achieves the standard one-dimensional rate of convergence and has the same asymptotic accuracy as if the nuisance terms were known with certainty. Furthermore, for the parametric component, the estimates are asymptotically normal. Note, we estimate g (∙) using b-spline (k = 4). In the parametric part of the estimation, we include the previous set of controls Xpsc and the time.
IV. Estimation results
As widely explained in the previous section, our preferred econometric methodology is the GMM-system estimator that allows us to control for dynamics in occupational accidents rates and joint endogeneity of the explanatory variables and for country-specific effects. For comparative purposes, we also present results obtained by mean of the pooled OLS estimator, which ignores the presence of country-specific effects and treats all variables as exogenous, and the random effects (RE)/fixed effects (FE) estimators, which controls for the presence of unobserved country-specific heterogeneity. The results of the pooled OLS estimates are presented in ; the panel RE/FE and system-GMM results are displayed in .
Table 4. Pooled OLS estimations (dependent variable: OA rate).
Table 5. Panel Fixed/Random effects model and system-GMM (dependent variable: OA rate).
In , Model 1 shows the results of the baseline model where occupational accidents rate is regressed only against the vector of the chosen covariates relative to the productive-system variables, i.e. employment risk, large firms, and temporary contracts. In models 2 and 3, the business cycle-related explanatory variables (i.e. per capita GDP, fixed investments/GDP, unemployment) and some other socio-economic controls (i.e. secondary education, crime) are added. Overall evidence, at this very first stage of analysis, confirms the consistent effect exerted by all the different sets of covariates on occupational accident rates, across all the estimates. The obtained positive and highly statistically significant relationships support the argument that higher levels of employment risk, temporary contracts, per capita GDP, unemployment, and crime may accompany an increase in the rate of occupational accidents. Likewise, the obtained negative and highly statistically significant relationships allow us to hold that higher levels of large firms, secondary education, and fixed investments/GDP may drive a reduction in the rate of occupational accidents.
As it is shown in , the obtained panel fixed/random effects models and the system-GMM results confirm, with a high degree of reliability, that the employed approach to the assessment of the occupational accidents rate is adequate. The negative relationship with the firms’ large size is consistent with the hypothesis by main literature (Fabiano, Currò, and Pastorino Citation2004) according to which the number of accidents declines with the increase of company’s size. It is often argued (Salminen Citation1993, Citation1998) that large companies have fewer accidents since they have more knowledge and better financial resources for workplace health and safety investments. With regard to the level of education, the negative and statistically significant relationship is in line with the relevant literature (Cioni and Savioli Citation2016).
As we can observe, in the system-GMM model, the coefficient of the lagged dependent variable is positive and highly statistically significant suggesting that occupational accidents rate displays persistence over time, i.e. the dynamics and current complex workplace safety conditions can subsequently develop more accidents. The negative and highly statistically relationship with fixed investments/GDP confirms that occupational accidents are influenced by economic cycles; thus, higher levels of fixed investments/economic upturns might reduce the number of occupational accidents. The inverse relationship with unemployment rate is also consistent with relevant literature (i.e. Ferguson, McNally, and Booth Citation1985; Rasmussen Citation1987; Vredenburgh Citation2002; Boone and van Ours Citation2006; de La Fuente et al. Citation2014): the idea behind this finding is that, if unemployment is high, workers are reluctant to report accidents because they fear that employers will hold this against them. From a policy perspective, this result implies that, in times of high unemployment, safety auditors should encourage people to report accidents. Finally, the estimates highlight a positive relationship between occupational accidents rate and crime, thus suggesting, in line with our hypothesis, that countries with a higher level of crime are exposed to lower OSH compliance by firms, resulting in an increase of occupational accidents.
Robustness checks
The findings of our estimations, however, could potentially undermine two main issues. On the one side, the effect over the dependent variable of the differences in the propensity to report occupational accidents across our panel of 27 European countries. In particular, the phenomenon of underreporting could vary by national context and, even though constant over the short-time span of our analysis, could affect the panel random effects model estimates. On the other side, the different sectoral composition of the economic and productive systems of the 27 countries included in our panel could lead to a conflation of industry-specific determinants of workplace accidents.Footnote4
As pointed out in the literature, underreporting of workplace accidents is a multidimensional issue that is strictly correlated to national administrative requirements, institutional frameworks, economics cycle, and cultural heritage (Boone and van Ours Citation2006; Hall Citation2016; Picchio and van Ours Citation2017). Even though we believe these factors to be structural and rigid over time – i.e. especially in the short time horizon of our analysis -, we provide a robustness check with this respect for the random-fixed effects estimation presented in the previous section, by extending our analysis to fatal accidents. Indeed, including fatal accidents in the measurement of our dependent variable reduces to the minimum the risk of measurement error due to the underreporting phenomenon.
In , we implement our approach using alternatively as dependent variable the fatal occupational accident rate (model 1 and 2) and the total occupational accident rate (non-fatal plus fatal, model 3 and 4). From our estimations results, it is possible to see that when investigating exclusively on fatal work accident rates our model specification remains overall statistically appropriate and robust both in the panel fixed and random effects model estimations. Moreover, in models 3 and 4, to reduce the extent of the possible influence of underreporting with respect to the baseline estimations, we also consider as dependent variable the overall work accidents rate (non-fatal plus fatal accident rates). The overall estimation results, even in this case, provide a sounding confirmation of the findings presented in : signs and statistical significance of the estimated parameters hold.
Table 6. Robustness check - Panel Fixed/Random effects model (dependent variable: OA rate – fatal and total workplace accidents).
Moreover, in , in order to address more carefully the differences in the composition of the productive and economic systems among our 27 European countries, to reinforce the validity of our baseline estimation, we furtherly implement our analysis by focusing on the two sectors that exhibit the highest OA rates (Takala Citation2019): construction and manufacturing. As shown in , considering both panel fixed and random effects models, the findings provide a further confirmation (in terms of statistical significance, signs and magnitude) of the results presented in the baseline estimations in .
Table 7. Robustness check - Panel Fixed/Random effects model (dependent variable: construction OA rate).
Occupational accidents and growth
Ultimately, with regard to the shape of the relationship between occupational accident rate and per capita GDP level, we test Hp2, employing the same set of estimation strategies. The existence of a non-linear and non-monotonic relationship will imply that for each unit increase of per capita GDP, workplace accidents will increase at a decreasing rate, generating greater occupational safety gains. Our final estimation results are presented in .
Table 8. Testing the existence of a non-linear and non-monotonic relationship between GDP and occupational accidents rate (Dependent variable: OA rate).
We find evidence of an inverted U-shaped relationship between the rate of OA and the real per capita GDP, in a time of transition towards a new technological paradigm in Europe. This is an important consideration if economic growth policies are to be used as a strategy to improve workplace safety and health measures. The implementation of macroeconomic expansionary policies would have a positive impact on workplace environments, increasing investments in technological innovation, new machineries and safer equipment, higher training of workers, enhancing the overall occupational safety levels and the quality of the workplace systems. The results are robust to endogeneity linked to the transitional dynamics and persistency of the OA rate over time. The transition towards the new Industry 4.0 paradigm, currently in progress in the time period of our analysis, generates a skill biased technological change (SBTC) that, if exploited, could provide solutions able to enhance workers’ health, safety and wellbeing, reducing OA rates.
To provide a further robustness check to the reliability of the quadratic specification, we estimate EquationEquation 5(5)
(5) using a semiparametric fixed effects estimator (Baltagi and Li Citation2002). Therefore, we estimate the occupational accidents rate allowing the GDP to enter the model nonlinearly. In , we plot the nonparametric b-spline estimate of g(GDP); we can observe that the nonparametric estimate (red line) depicts a clear inverted U-shaped curve. This result provides an ‘unconstrained’ sounding reassessment of the non-linear relationship between GDP and occupational accidents rate obtained with the implemented parametric approaches.
Figure 2. Semiparametric Fixed effects model (Baltagi and Li Citation2002).
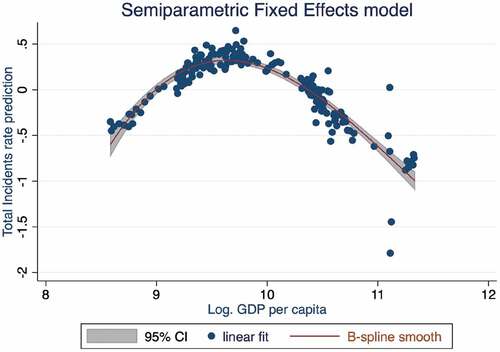
V. Conclusions
Our results can give rise to some relevant policy implications. Overall, our cross-country analysis reveals that the heterogeneity of OA rates is significantly correlated to productive systems characteristics, to the business cycle and to some other territorial variables. Based on our findings, the improvement of both macro- and micro-economic conditions should be the priority for new policies on work safety. In particular, policymakers and employers should be aware of the correlation between the business cycle and the occurrence of workplace accidents, which calls the need for additional safety measures during economic expansions. Although definitive conclusions cannot be drawn from the results of this study, some interesting insights do emerge. Firstly, in relation to the production system controls we can highlight the following findings: the higher is the share of large firms, the lower is the OA rates (confirming previous empirical literature); the existence of a positive, even though feeble, correlation between temporary contracts and OA (in line with Cioni and Savioli Citation2016); the higher is the employment risk attached to the productive sector, the higher is the OA rate. Secondly, in relation to the business cycle controls: the OA rate increases with real per capita GDP (confirming previous empirical literature); the higher is the level of education, the lower is the OA rate (Fotta and Bockosh Citation2000; Jeong Citation1999; Kletz Citation1993; de La Fuente et al. Citation2014; Cioni and Savioli Citation2016); the higher is the propensity to invest in fixed assets, the lower is the OA rate (Blank, Laflamme, and Diderichsen Citation1996; Sari et al. Citation2004; Asfaw, Pana-Cryan, and Rosa Citation2011). Finally, the estimations reveal that countries with a higher level of crime generate lower OSH compliance by firms, thus determining an increase in the number of occupational accidents.
Taken all together, our findings imply that OSH targets, besides pursuing the aims established in principle 10 of the European Pillar of Social Rights (Healthy, safe and well-adapted work environment and data protection) are crucial drivers for improving the sustainability and competitiveness of the EU economy.
Our findings call for a main policy recommendation. According to our estimates, fixed capital investments, hazardous and labour-intensive workplaces, and firms’ size, represent the main determinants of OA rates. This implies that the risk of underinvestment in OSH of small and medium enterprises (SMEs), especially during economic and financial downturns – as experienced throughout the 2007–2008, the 2012 sovereign debt, and the COVID-19 pandemic crises – becomes higher and socially concerning. This raises the issue whether or not relying solely on regulatory remedies and sermons is still sufficient. In our view, especially in recession periods, it becomes urgent to implement carrots policies (i.e. direct and indirect public aid schemes) to support OSH investments. In particular, in this vein, Italy is adopting this type of policy option throughout a specific initiative implemented by the Italian National Institute for Insurance against Accidents at Work (INAIL), providing direct financial grants to SMEs for both tangible and intangible OSH investments.
Disclosure statement
No potential conflict of interest was reported by the author(s).
Additional information
Funding
Notes
1 Hämäläinen, Saarela, and Takala (Citation2009) states that although globally the number of accidents has risen slightly, the trend in Europe is declining. One of the reasons for this decrease could be the introduction of stricter occupational health and safety legislation. Moreover, Takala (Citation2019) confirms the downward trend and lists a number of reasons: improvements in technology, processes and methods, better leadership, management and safety and health efforts, a reduction in the number of workers in hazardous industries and changing economic structures. Again, mechanization, automation and prefabrication processes are oriented to create jobs that are less prone to injury.
2 It includes all accidents in the course of work, whether they happen inside or outside the premises of the employer, on the premises of another employer, in public places or during transport (including road traffic accidents or accidents in any other mean of transportation) and at home (such as during teleworking); it also includes cases of acute poisoning and wilful acts of other persons. Instead, it excludes: accidents on the way to or from work (commuting accidents); deliberate self-inflicted injuries; occurrences caused solely by a medical condition (such as heart attack or stroke) that occurred during work, i.e. which were not (at least partially) caused by the occupational activity of the victim; accidents to members of the public, for example family members of a worker who are not working; and occupational diseases. https://ec.europa.eu/eurostat/cache/metadata/en/hsw_acc_work_esms.htm..
3 A further approach for dealing with the cross country differences would imply the inclusion of a full set of time/country (or other covariate/country) interaction terms. However, this option is not feasible in our setting due to multicollinearity, as it is confirmed by the VIF test (well above the threshold of 10).
4 We would like to thank an anonymous referee for pointing this out.
References
- Anyfantis, I., G. Boustras, and A. Karageorgiou. 2018. “Maintaining Occupational Safety and Health Levels During the Financial Crisis – a Conceptual Model.” Safety Science 106: 246–254. doi:10.1016/j.ssci.2016.02.014.
- Arellano, M., and S. Bond. 1991. “Some Tests of Specification for Panel Data: Monte Carlo Evidence and an Application to Employment Equations.” The Review of Economic Studies 58 (2): 277–297. doi:10.2307/2297968.
- Arellano, M., and O. Bover. 1995. “Another Look at the Instrumental-Variable Estimation of Error- Components Models.” Journal of Econometrics 68 (1): 29–52. doi:10.1016/0304-4076(94)01642-D.
- Asfaw, A., R. Pana-Cryan, and R. Rosa. 2011. “The Business Cycle and the Incidence of Workplace Injuries: Evidence from the U.S.A.” Journal of Safety Research 42: 1–8. doi:10.1016/j.jsr.2010.10.008.
- Baltagi, B. H. 2005. Econometric Analysis of Panel Data. 3rd ed. New York: John Wiley & Sons Inc.
- Baltagi, B. H., and Q. Li. 2002. “On Instrumental Variable Estimation of Semiparametric Dynamic Panel Data Models.” Economics Letters 76: 1–9. doi:10.1016/S0165-1765(02)00025-3.
- Benavides, F. G., J. Benach, C. Muntaner, G. L. Delclos, N. Catot, and M. Amable. 2006. “Associations Between Temporary Employment and Occupational Injury: What are the Mechanisms?” Occupational Environmental Medicine 63 (6): 416–421. doi:10.1136/oem.2005.022301.
- Blank, V., F. Diderichsen, and R. Andersson. 1996. “Technological Development and Occupational Accidents as a Conditional Relationship: A Study Over Eighty Years in the Swedish Mining Industry.” Journal of Safety Research 27 (3): 137–146. doi:10.1016/0022-4375(96)00014-X.
- Blank, V., L. Laflamme, and F. Diderichsen. 1996. “The Impact of Major Transformations of a Production Process on Age-Related Accident Risks: A Study of an Iron-Ore Mine.” Accident Analysis and Prevention 28: 627–636. doi:10.1016/0001-4575(96)00035-8.
- Blundell, R., and S. R. Bond. 1998. “Initial Conditions and Moment Restrictions in Dynamic Panel Data Models.” Journal of Econometrics 87: 115–143. doi:10.1016/S0304-4076(98)00009-8.
- Bonamore, G., F. Carmignani, and E. Colombo. 2015. “Addressing the Unemployment–Mortality Conundrum: Non-Linearity is the Answer.” Social Science & Medicine 126: 67–72. doi:10.1016/j.socscimed.2014.12.017.
- Boone, J., and J. C. van Ours. 2006. “Are Recessions Good for Workplace Safety?” Journal of Health Economics 25 (6): 1069–1093. doi:10.1016/j.jhealeco.2006.03.002.
- Brooker, A. S., J. Frank, and V. S. Tarasuk. 1997. “Back Pain Claim Rates and the Business Cycle.” Social Science and Medicine 45 (3): 429–439. doi:10.1016/S0277-9536(96)00359-0.
- Cioni, M., and M. Savioli. 2016. “Safety at the Workplace: Accidents and Illnesses.” Work, Employment and Society 30 (5): 858–875. doi:10.1177/0950017015590759.
- Cornelissen, P. A., J. J. Van Hoof, and M. D. De Jong. 2017. “Determinants of Safety Outcomes and Performance: A Systematic Literature Review of Research in Four High-Risk Industries.” Journal of Safety Research 62: 127–141. doi:10.1016/j.jsr.2017.06.009.
- D’Ambrosio, A., R. Leombruni, and T. Razzolini. 2022. “Trading of Wage for Workplace Safety? Gaps Between Immigrants and Natives in Italy.” Economia Politica 39 (3): 903–960. doi:10.1007/s40888-020-00209-1.
- da Silva, S. L. C., and F. G. Amaral. 2019. “Critical Factors of Success and Barriers to the Implementation of Occupational Health and Safety Management Systems: A Systematic Review of Literature.” Safety Science 117: 123–132. doi:10.1016/j.ssci.2019.03.026.
- Davies, R., P. Jones, and I. Nuňez. 2009. “The Impact of the Business Cycle on Occupational Injuries in the UK.” Social Science and Medicine 69: 178–182. doi:10.1016/j.socscimed.2009.04.033.
- de La Fuente, V. S., M. A. Camino López, I. F. Fontaneda González, O. J. Alcántara, and D. O. Ritzel. 2014. “The Impact of Economic Crisis on Occupational Injuries.” Journal of Safety Research 48: 77–85. doi:10.1016/j.jsr.2013.12.007.
- De Merich, D., M. G. Gnoni, A. Guglielmi, G. J. Micheli, G. Sala, F. Tornese, and G. Vitrano. 2022. “Designing National Systems to Support the Analysis and Prevention of Occupational Fatal Injuries: Evidence from Italy.” Safety Science 147: 105615. doi:10.1016/j.ssci.2021.105615.
- Dorman, P. 2000. “If Safety Pays, Why Don’t Employers Invest in It?” In Systematic Occupational Health and Safety Management: Perspectives on an International Development, edited by K. Frick, P. Jensen, M. Quinlan, and T. Wilthagen, 351–365. Amsterdam: Pergamon.
- Dupré, D. 2001. Accidents at Work in the EU – 1998-1999. Statistics in Focus: Population and Social Conditions, Eurostat Theme 3 – 16/2001. Luxembourg: Publications Office of the European Union.
- Eurofound. 2012. Fifth European Working Conditions Survey. Luxembourg: Publications Office of the European Union.
- European Commission. 2002. Adapting to Change in Work and Society: A New Community Strategy on Health and Safety at Work 2002–2006, DG Employment. Luxembourg: Publications Office of the European Union.
- European Commission. 2007. Improving Quality at Work: Community Strategy 2007–2012 on Health and Safety at Work. Improving the Quality and Productivity at Work, DG Employment. Luxembourg: Publications Office of the European Union.
- Fabiano, B., F. Currò, and R. Pastorino. 2004. “A Study of the Relationship Between Occupational Injuries and Firm Size and Type in the Italian Industry.” Safety Science 42 (7): 587–600. doi:10.1016/j.ssci.2003.09.003.
- Fabiano, B., F. Currò, A. P. Reverberi, and R. Pastorino. 2008. “A Statistical Study on Temporary Work and Occupation Accidents: Specific Risk Factors and Risk Management Strategies.” Safety Science 46 (3): 535–544. doi:10.1016/j.ssci.2007.05.004.
- Fabiano, B., I. Parentini, A. Ferraiolo, and R. Pastorino. 1995. “A Century of Accidents in the Italian Industry: Relationship with the Production Cycle.” Safety Science 21 (1): 65–74. doi:10.1016/0925-7535(95)00043-7.
- Farina, E., M. Giraudo, G. Costa, and A. Bena. 2018. “Injury Rates and Economic Cycles in the Italian Manufacturing Sector.” Occupational Medicine 68: 459–463. doi:10.1093/occmed/kqy102.
- Ferguson, J., M. McNally, and R. Booth. 1985. “Accidental Injuries Among Naval Personnel by Occupation, Duty Status, and Pay-Grade.” Accident Analysis and Prevention 17 (1): 79–86. doi:10.1016/0001-4575(85)90011-9.
- Fortin, B., P. Lanoie, and C. Laporte. 1996. “Unemployment Insurance and the Duration of Workplace Accidents.” Canadian Journal of Economics 29: 17–24. doi:10.2307/135953.
- Fotta, B., and G. Bockosh. 2000. “The Aging Workforce: An Emerging Issue in the Mining Industry.” National Safety for Occupational Safety and Health (NIOSH), Mining Safety and Health Research. https://www.cdc.gov/niosh/mining/works/coversheet1199.html.
- Giraudo, M., A. Bena, M. Mosca, E. Farina, R. Leombruni, and G. Costa. 2018. “Differences in Work Injury Risk Between Immigrants and Natives: Changes Since the Economic Recession in Italy.” BMC Public Health 19 (1): 836. doi:10.1186/s12889-019-7178-2.
- Greer, S. L. 2018. “Labour Politics as Public Health: How the Politics of Industrial Relations and Workplace Regulation Affect Health.” European Journal of Public Health 28 (3): 34–37. doi:10.1093/eurpub/cky163.
- Hall, A. 2016. “Trust, Uncertainty and the Reporting of Workplaces Hazards and Injuries.” Health, Risk & Society 18 (7–8): 427–448. doi:10.1080/13698575.2016.1264576.
- Hämäläinen, P., K. L. Saarela, and J. Takala. 2009. “Global Trend According to Estimated Number of Occupational Accidents and Fatal Work-Related Diseases at Region and Country Level.” Journal of Safety Research 40 (2): 125–139. doi:10.1016/j.jsr.2008.12.010.
- Hartwig, R. P., W. J. Kahley, T. E. Restrepo, and R. C. Retterath. 1997. “Workers’ Compensation and Economic Cycles: A Longitudinal Approach.” Proceedings of the Casualty Actuarial Society, 84, 660–700. https://www.casact.org/sites/default/files/database/proceed_proceed97_97660.pdf
- Haslam, R., S. Hide, A. Gibb, D. Gyi, T. Pavitt, S. Atkinson, and A. Duff. 2005. “Contributing Factors in Construction Accidents.” Applied Ergonomics 36: 401–415. doi:10.1016/j.apergo.2004.12.002.
- Hausman, J. A. 1978. “Specification Tests in Econometrics.” Econometrica 46: 1251–1271. doi:10.2307/1913827.
- Ivascu, L., and L. I. Cioca. 2019. “Occupational Accidents Assessment by Field of Activity and Investigation Model for Prevention and Control.” Safety 5 (1): 12. doi:10.3390/safety5010012.
- Jeong, B. 1999. “Comparisons of Variables Between Fatal and Nonfatal Accidents in Manufacturing Industry.” International Journal of Industrial Ergonomics 23: 565–572. doi:10.1016/S0169-8141(98)00020-1.
- Khanzode, V., J. Maiti, and P. Ray. 2011. “A Methodology for Evaluation and Monitoring of Recurring Hazards in Underground Coal Mines.” Safety Science 49 (8–9): 1172–1179. doi:10.1016/j.ssci.2011.03.009.
- Khanzode, V. V., J. Maiti, and P. K. Ray. 2012. “Occupational Injury and Accident Research: A Comprehensive Review.” Safety Science 50: 1355–1367. doi:10.1016/j.ssci.2011.12.015.
- Kletz, T. A. 1993. “Accident Data—The Need for a New Look at the Sort of Data That are Collected and Analysed.” Safety Science 16 (3–4): 407–415. doi:10.1016/0925-7535(93)90061-H.
- Ko, K., J. Mendeloff, and W. Gray. 2010. “The Role of Inspection Sequence in Compliance with the US Occupational Safety and Health Administration’s (OSHA) Standards: Interpretations and Implications.” Regulation & Governance 4 (1): 48–70. doi:10.1111/j.1748-5991.2010.01070.x.
- Kossoris, M. 1938. “Industrial Injuries and the Business Cycle.” Monthly Labor Review 61: 579–595.
- Kubicek, A., R. Bhanugopan, and G. O’Neill. 2019. “How Does Cultural Intelligence Affect Organisational Culture: The Mediating Role of Cross-Cultural Role Conflict, Ambiguity, and Overload.” The International Journal of Human Resource Management 30 (7): 1059–1083. doi:10.1080/09585192.2017.1282535.
- Lafuente, E., and V. Daza. 2019. “Work Inspections as a Control Mechanism for Mitigating Work Accidents in Europe.” Tec Empresarial 14 (1): 26–37. doi:10.18845/te.v14i1.4953.
- Leigh, J. P. 1989. “Firm Size and Occupational Injury and Illness Incidence Rates in Manufacturing Industries.” Journal of Community Health 14 (1): 44–52. doi:10.1007/BF01324438.
- Leigh, J., H. Mulder, G. Want, N. Farnsworth, and G. Morgan. 1990. “Personal and Environmental Factors in Coal Mining Accidents.” Journal of Occupational Accidents 13: 233–250. doi:10.1016/0376-6349(90)90023-O.
- Lenaerts, K., W. Waeyaert, D. Gillis, I. Smits, and H. Hauben. 2022. Occupational Safety and Health in Digital Platform Work: Lessons from Regulations, Policies, Actions and Initiatives. Bruxelles: EU Publishing Services. doi:10.2802/236095.
- Liu, K. 2019. “Regulating Health and Safety at the Workplace: Prescriptive Approach Vs Goal-Oriented Approach.” Safety Science 120: 950–961. doi:10.1016/j.ssci.2019.08.034.
- Lombardi, M., M. Fargnoli, and G. Parise. 2019. “Risk Profiling from the European Statistics on Accidents at Work (ESAW) accidents′ Databases: A Case Study in Construction Sites.” International Journal of Environmental Research and Public Health 16: 4748. doi:10.3390/ijerph16234748.
- Lopez, M. A. C., D. O. Ritzel, I. Fontaneda, and O. J. G. Alcantara. 2008. “Construction Industry Injuries in Spain.” Journal of Safety Research 39 (5): 497–507. doi:10.1016/j.jsr.2008.07.006.
- Maiti, J., and A. Bhattacherjee. 1999. “Evaluation of Risk of Occupational Injuries Among Underground Coal Mine Workers Through Multinomial Logit Analysis.” Journal of Safety Research 30: 93–101. doi:10.1016/S0022-4375(99)00003-1.
- Maiti, J., A. Bhattacherjee, and S. Bangdiwala. 2001. “Loglinear Model for Analysis of Cross-Tabulated Coal Mine Injury Data.” Injury Control and Safety Promotion 8: 229–236. doi:10.1076/icsp.8.4.229.3335.
- Maiti, J., S. Chatterjee, and S. Bangdiwala. 2004. “Determinants of Work Injuries in Mines – an Application of Structural Equation Modelling.” Injury Control and Safety Promotion 11 (1): 29–37. doi:10.1076/icsp.11.1.29.26305.
- Nichols, T. 1991. “Industrial Injuries in British Manufacturing Industry and Cyclical Effects: Continuities and Discontinuities in Industrial Injury Research.” The Sociological Review 39: 131–139. doi:10.1111/j.1467-954X.1991.tb02972.x.
- Picchio, M., and J. C. van Ours. 2017. “Temporary Jobs and the Severity of Workplace Accidents.” Journal of Safety Research 61: 41–51. doi:10.1016/j.jsr.2017.02.004.
- Rasmussen, J. 1987. “Reasons, Causes and Human Errors.“ In New Technology and Human Error, edited by J. Rasmussen, K. Duncan, and J. Leplat, 293–303. UK: Wiley and Sons Ltd.
- Robinson, J. 1988. “The Rising Long-Term Trend in Occupational Injury Rates.” American Journal of Public Health 78: 276–281. doi:10.2105/AJPH.78.3.276.
- Salminen, S. 1993. “The Effect of Company Size on Serious Occupational Accidents.” In Advances in Industrial Ergonomics and Safety, edited by R. Nielsen and K. Jorgensen, 507–514. Vol. 5. London: Taylor & Francis.
- Salminen, S. 1998. “Why Do Small Company Owners Think Larger Companies Have Fewer Accidents?” Journal of Occupational Health & Safety – Australia and New Zealand 14 (6): 607–614.
- Sari, M., H. Duzgun, C. Karpuz, and A. Selcuk. 2004. “Accident Analysis of Two Turkish Underground Coal Mines.” Safety Science 42: 675–690. doi:10.1016/j.ssci.2003.11.002.
- Schulte, P. A., G. Delclos, S. A. Felknor, and L. C. Chosewood. 2019. “Toward an Expanded Focus for Occupational Safety and Health: A Commentary.” International Journal of Environmental Research and Public Health 16: 4946. doi:10.3390/ijerph16244946.
- Takala, J. 2019. “Burden of Injury Due to Occupational Exposures.” In Handbook of Disability, Work and Health. Handbook Series in Occupational Health Sciences, U. Bültmann and J. Siegrist edited by, Vol. 1. Cham: Springer. 10.1007/978-3-319-75381-2_5-1.
- Tassinari, A., R. E. Chesta, and L. Cini. 2020. “Labour Conflicts Over Health and Safety in the Italian COVID-19 Crisis.” Interface 12 (1): 128–138.
- Theorell, T. 2022. Handbook of Socioeconomic Determinants of Occupational Health. From Macro-Level to Micro-Level Evidence. Cham (Switzerland): Springer.
- Toch, M., C. Bambra, T. Lunau, V. D. W. KA, W. MI, N. Dragano, and E. TA. 2014. “All Part of the Job? The Contribution of the Psychosocial and Physical Work Environment to Health Inequalities in Europe and the European Health Divide.” International Journal of Health Services 44 (2): 285–305. doi:10.2190/HS.44.2.g.
- Ussif, A. A. 2004. “An International Analysis of Workplace Injuries.” Monthly Labor Review 127: 41–51.
- Verra, S. E., A. Benzerga, B. Jiao, and K. Ruggeri. 2019. “Health Promotion at Work: A Comparison of Policy and Practice Across Europe.” Safety and Health at Work 10: 21–29. doi:10.1016/j.shaw.2018.07.003.
- Virtanen, M., M. Kivimäki, M. Joensuu, P. Virtanen, M. Elovainio, and J. Vahtera. 2005. “Temporary Employment and Health: A Review.” International Journal of Epidemiology 34 (3): 610–622. doi:10.1093/ije/dyi024.
- Viscusi, W. 1986. “The Impact of Occupational Safety and Health Regulations, 1973-1983.” The Rand Journal of Economics 17 (4): 567–580. doi:10.2307/2555482.
- Vredenburgh, A. G. 2002. “Organizational Safety: Which Management Practices are Most Effective in Reducing Employee Injury Rates?” Journal Safety Research 33 (2): 259–276. doi:10.1016/S0022-4375(02)00016-6.
- Wadsworth, E., and D. Walters. 2014. “The Determinants of Workplace Health and Safety Practice in the UK.” Policy and Practice in Health and Safety 12 (2): 3–22. doi:10.1080/14774003.2014.11667801.
- Weil, D. 1996. “If OSHA is so Bad, Why is Compliance so Good?” The Rand Journal of Economics 27 (3): 618–640. doi:10.2307/2555847.
- Zambon, I., A. Piergentili, L. Salvati, D. Monarca, P. Matyjas-Łysakowska, and A. Colantoni. 2018. “Applied Research for a Safer Future: Exploring Recent Job Accidents in Agriculture, Italy (2012–2017).” Processes 6 (7): 87. doi:10.3390/pr6070087.