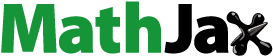
ABSTRACT
This study explores the nexus between livelihood goals (LGs) and livelihood strategy (LS) selection preferences. We construct a measurement of three livelihood goals (survival, security, and self-respect goals) by combining qualitative and quantitative analysis and classify livelihood strategies into three groups (agricultural, non-agricultural, and diversification) based on household income sources. The multinomial logit model with fixed effects empirically estimates the relationship between livelihood goal indices and livelihood strategy selection with panel data (2010–2018) from the China Family Panel Studies surveys (CFPS). The results show that households with survival goals are more likely to choose the agricultural and diversification strategies and less likely to select the non-agricultural strategy. Households with security and self-respect goals are more likely to choose the non-agricultural strategy and are less likely to select the agricultural strategy. Families with a higher self-respect goal level are more flexible in livelihood strategy selection. The livelihood strategy selection preference is consistent in different regions of rural China when households pursue survival and security maximization. From the low-level survival goal to the high-level self-respect goal, consistent with a gradual transition from a low-income agricultural strategy to the high-income non-agricultural strategy; the diversification strategy is the transformed livelihood strategy in this process.
I. Introduction
Chinese people’s life trajectories have significantly changed with the changed employment conditions during the COVID-19 pandemic. To lower future risk and uncertainties, many rural households changed their livelihood strategy (LS), such as choosing a more stable job, searching for a part-time job, or starting a small business (Zhao, Guo, and Peng Citation2022), reflecting their asset reallocation changes under limited structural constraints and the dynamic environment.
A large body of literature has analysed the determinants of LS selection based on the Sustainable Livelihood Approach (SLA) framework proposed by the Department for International Development (DFID) in 1999. The SLA framework links macro and micro factors on what households have rather than what they lack (Fahad et al. Citation2023) and shows the dynamic relationship between vulnerability, transforming structure and process, livelihood assets, strategies, and outcomes (Sarker et al. Citation2019). Under deteriorating climate change and a complicated social, economic, and political environment, there are three literature strands on factors influencing households’ LS selection.
The first strand focuses on the influence of vulnerability, particularly the effect of natural environmental factors on households’ LS selection. Most studies focus on farmers’ perceptions of climate change and their adaptive LSs (Démurger, Fournier, and Yang Citation2010; Funk et al. Citation2020; Musumba et al. Citation2022). For example, Funk et al. (Citation2020) reveal that most rural households understand climate change, but their adaptive strategies differ considerably in different regions in India. Musumba et al. (Citation2022) find that lowering LS’s diversity is an adaptation choice to deal with drought in rural Africa.
The second strand of studies focuses on the impact of the transforming structure and processes on households’ LS selection, specifically on how socio-economic factors influence households’ LS choices (Helmy Citation2020; Liang, Lin, and Lin Citation2022; Neef et al. Citation2018). For example, Neef et al. (Citation2018) find that social norms and cultural values influence households’ LS adaptation choices, and the diverse recognition of livelihood opportunities and climate-related risks brings about widely different LS adaptation decisions even in a much localized cultural context. Helmy (Citation2020) focuses on the influence of wealth and the urban-rural divide on the LS revolution in Egypt and finds that poor rural households depend more on informal LS and exhibit higher diversification.
The third literature strand emphasizes the importance of household characteristics, mainly on how households’ asset endowments affect their LS decision (Démurger, Fournier, and Yang Citation2010; Huang et al. Citation2021; Jiao, Pouliot, and Walelign Citation2017). Existing studies focus on the influence of different types of livelihood assets, such as formal and informal credit for financial assets (Felkner et al. Citation2022), natural assets (e.g. land access) (Jian, Mishra, and Zhu Citation2019), and social assets (Baird and Gray Citation2014), on households’ LS selection. For example, Huang et al. (Citation2021) find that, in Sa Pa, Vietnam, financial capital is the key to promoting a tourism livelihood, but it impedes agricultural households from participating in other livelihood activities.
Few studies discuss the linkage between livelihood goals (LGs) and LS selection, focusing mainly on the sub-levels of LGs – livelihood objectives (Berkhout et al. Citation2010; George and Ngaruko Citation2017; Jakoby et al. Citation2014). For example, Berkhout et al. (Citation2010) find that, in northern Nigeria, farmers’ production behaviours influence efficiency levels, and differences in intended behaviours come from the farmers’ heterogeneous goals. Jakoby et al. (Citation2014) reveal that farmers’ objectives and trade-offs between them affect their evaluation of land management strategies, producing structurally different outcomes in strategy choice. George and Ngaruko (Citation2017) discover that farmers’ varying objectives influence their investment in an irrigation scheme in the Lake Victoria water basin. However, despite these findings, there is no consensus on how LGs are measured and how households’ LGs influence their LS selection in rural China by on-farm and off-farm rural households.
The primary objective of this study is to explore the nexus between households’ LGs and their LS selection using data from the 2010–2018 China Family Panel Studies. The contributions of this study to the literature on LS selection are threefold. First, this study integrates LGs into the SLA framework to comprehensively understand the relationship between LGs, livelihood assets, and LS selection. Previous studies emphasize the role of LGs in LS selection (George, Fidelman, and Smith Citation2017), but no studies have developed an integrated framework to understand the roles of LGs in LS selection. Second, unlike previous studies using a survey to investigate farmers’ production objectives (Berkhout et al. Citation2010), or using proxy variables, such as risk attitudes (Jakoby et al. Citation2014) or collective action (George and Ngaruko Citation2017), to represent farmers’ objective preferences, we construct a measurement for unobservable LGs in four steps. In the first step, we classify household goals into three groups: survival, security, and self-respect goals and extract critical indicators for each type of LG from the literature (Dzanku Citation2018; Start and Johnson Citation2004; Tambe Citation2022). In the second step, we identify the key indicators through principal component analysis (PCA). In the third step, we use the multinomial logit (MNL) regression model to select usable indicators to explore the relationship between the identified key indicators and different types of LS. Indicators that pass these three steps are used to calculate the LG indices in the fourth step. The third contribution is that, in addition to considering farmers’ production behaviour, we include rural households in non-farm sectors in our analysis. Previous studies considered only farmers engaged in agricultural activities and analysed how their goals (or objectives) influence their production behaviour (Berkhout et al. Citation2010; George and Ngaruko Citation2017; Jakoby et al. Citation2014) but ignored rural residents engaged in non-farm activities. However, households involved in different livelihood activities could have heterogeneous LGs, significantly influencing their LS selection. Furthermore, off-farm workers are the main Chinese rural residents (Zhang et al. Citation2018). We include this group in our study to provide a more comprehensive understanding and outlook of Chinese rural residents’ livelihood position.
The rest of the paper is structured as follows. Section II presents the conceptual framework of the LSs and LGs. Data collection, measurements, and empirical models are discussed in Section III. Section V discusses the descriptive results, validity test results, and empirical results. Section VI concludes the paper with policy implications.
II. Conceptual framework
The theory of reasoned goal pursuit (TRGP) illustrates that goals motivate people’s behaviour in a given behavioural domain, intensify their motivation for achievement, and affect goal-holders’ productivity and satisfaction (Icek and Kruglanski Citation2019). LGs are the main base of livelihood asset allocation and are critical to the orientation of LS selection and implementation (George, Fidelman, and Smith Citation2017). Exploring how households’ LGs influence their LS selection can help us unpack the links between rural households’ inner motivation and their LS decisions, facilitating the implementation of a rural revitalization strategy in the post-Covid era.
shows the relationships between livelihood assets, LGs, LS selection, and livelihood outcomes based on the SLA framework. First, households’ LGs are decided by internal and external factors. Internal factors refer to livelihood assets that households possess and can access. Livelihood assets, allocation approaches, and efficiencies decide what LG households can realize (Díaz-Montenegro, Varela, and Gil Citation2018; Fahad et al. Citation2023; Sun et al. Citation2023). More assets (kinds and stocks) can enhance households’ portfolios to cope with risk and vulnerability (Jiao, Pouliot, and Walelign Citation2017; Sarker et al. Citation2019) and pursue higher LGs. External shocks (e.g. vulnerability, transforming the structure, and process) can directly influence households’ goal-setting or indirectly influence their goal-setting by influencing their livelihood assets (Wale, Andrias Nkoana, and Mkuna Citation2022). For example, Bai et al. (Citation2020) find that exposure to snow significantly affects households’ ability to realize livelihood objectives on the Mongolian Plateau.
Second, households’ LGs influence their LS selection. LGs are heterogeneous and comprise various objectives that may be parallel or exclusive, leading households to choose a different LS, even in a similar social environment. Farmers’ most common exclusive dual objectives are income growth and stability (Díaz-Montenegro, Varela, and Gil Citation2018). However, under different living standards, these two objectives could comprise different LGs, and households could adopt disparate LS to realize them. In most studies on households’ decisions or choices, utility maximization is the assumed goal that families pursue (Ding and Abdulai Citation2020; Jian, Mishra, and Zhu Citation2019). Therefore, following the work of Berkhout et al. (Citation2011), Chambers and Ghildyal (Citation1984), George and Ngaruko (Citation2017), and Gordon et al. (Citation2001), we classify household goals into three groups: survival, security, and self-respect goals, where households pursue survival maximization, asset accumulation maximization, and self-respect maximization, respectively. Instead of being mutually exclusive, households’ goals can be hierarchical and exist simultaneously (Jakoby et al. Citation2014). Therefore, we assume the three LGs can coexist or be upgraded when a lower-level LG is achieved.
Third, households’ LGs are dynamic and can go up or down in the next round regardless of whether they achieve them, thus encouraging them to reallocate their livelihood assets or improve their allocation efficiency (Sun et al. Citation2023). Further, Gordon et al. (Citation2001) show that different LS are generated based on different LGs, which can also change the LS and surrounding circumstances. Therefore, households adjust or keep their LGs based on the obtained livelihood outcomes, determining the LS selection at the next round and bringing about a dynamic livelihood trajectory.
III. Methodology
Data
We use data from the China Family Panel Studies (CFPS). Launched in 2010, the CFPS is a nationally representative, biennial longitudinal survey of Chinese communities, families, and individuals by the Institute of Social Science Survey (ISSS) of Peking University, China. CFPS covers 25 provinces/municipalities in China, representing about 95% of the Chinese population. It comprises four datasets: children, adults, households’ economic condition, and household demographics. The adult dataset covers individual health, education level, work, and migration experience. The household economic dataset includes households’ economic activity, income and expenditure, assets, and regional location. Using these national panel datasets, we minimize post hoc rationalization of the indicators of LGs.
We first merge adult and household datasets based on the respondents’ IDs and keep the samples with complete data for each year. Second, we combine five cross-section datasets (2010–2018) into a panel dataset to control for unobserved time-invariant heterogeneity variables. These two steps allow us to select indicators of LGs, LSs, and the control variables. Third, we drop urban samples because our analysis is of rural households. Finally, we delete samples with missing values in the dependent and independent variables. This process results in 25,948 rural samples for analysis in this study.
Measurement of livelihood goals
In the following two sub-sections, we discuss the procedures for constructing the measurement of LGs and the indicators selected to construct the three LGs.
Measurement of the livelihood goals
Following the studies by Liu et al. (Citation2020), Matter, Boillat, and Ifejika Speranza (Citation2021), and Zhou et al. (Citation2021), we construct the LGs indicators in four steps. First, because LGs are unobservable, we extract potential indicators that can be observed from the description for each kind of livelihood objective in previous seminal studies, such as Berkhout et al. (Citation2011), Jakoby et al. (Citation2014) and Start and Johnson (Citation2004). Second, to improve the indicators’ validity, we identify the key indicators using the Kaiser-Meyer-Olkin (KMO) test and PCA. KMO tests the proportion of variance in ratings attributable to underlying factors, and PCA with varimax rotation extracts the underlying factors (Speranza, Chinwe, and Rist Citation2014). Indicators are selected if they satisfy: (1) the KMO value is above 0.5; (2) the cumulative proportion of the indicator is over 50% in PCA; and (3) the loading value after rotation of each indicator is over 0.5 (Matter, Boillat, and Ifejika Speranza Citation2021). The preliminary and PCA assessments are reiterated until subsequent results show no further improvement. Third, we use the MNL regression model to select indicators significantly influencing household LS selection. Finally, we use PCA to calculate the LG index for each type of LG (see EquationEquation (1)(1)
(1) ), where
is the number of retained factors;
is the variance contribution of the factor
, and
is the factor score of factor
.
refers to three LG indices: the survival, security, and self-respect goal index.
Potential indicators of livelihood goals
According to Chambers and Ghildyal (Citation1984) and Gordon et al. (Citation2001), the survival goal is based on stable subsistence, including the desire of households for sufficient basic living needs, such as food, clothing, and shelter (Ibrahim Citation2022). Subsistence households have limited access to finance and use low inputs and traditional farming methods to eke out a livelihood (Tambe Citation2022). Those households are always vulnerable, poor, and struggling for subsistence (Kundu and Das Citation2021). Therefore, poverty is the first indicator used to measure the survival goal index. In addition, the poorer a family, the greater the proportion of total spending on food (Xiaohua Citation2018). Engel’s Law suggests that the share of food expenditure of total expenses declines as income rises. Thus, the Engel coefficient is selected as the second indicator to measure the survival goal index. Previous studies show that lower-income households have a higher diversification index than higher-income households (Djido and Shiferaw Citation2018). The diversification index assesses households’ concern for survival under unexpected external events. Therefore, it is the third indicator in measuring the survival goal index (see ).
Table 1. The indicators of livelihood goals from the literature review.
When households reach a stable, sufficient subsistence state, they may upgrade to the security goal. Households with the security goal mainly focus on accumulating assets that are easily sold, divisible, and secure against price fluctuations to realize a more perpetuating livelihood (Tambe Citation2022), such as land, shelter, and durables (Start and Johnson Citation2004). From this perspective, the proportion of households’ land, house, and durable assets in their total net assets are used to measure households’ security goal level.
The self-respect goal is based on independence and choice, which reflects self-confidence and esteem in coping with and adapting to potential threats and opportunities (Start and Johnson Citation2004). Unlike the security goal, intangible social capital is the most emphasized asset influencing the self-respect goal (Gordon et al. Citation2001). Social capital can efficiently leverage other livelihood assets (Fahad et al. Citation2023) and strengthen households’ livelihood resilience (Speranza, Chinwe, and Rist Citation2014). Therefore, social capital is the lead indicator to measure the self-respect goal level. We use the proportion of social spending in household total expenditure and households’ self-reported social status to reflect a household’s social capital level. In addition, more business assets can improve households’ self-perceived well-being more than for those who work for others (Tambe Citation2022), and that helps them move to a more highly remunerated LS (Jiao, Pouliot, and Walelign Citation2017). Households at the self-respect stage can invest more in personal and family welfare, such as purchasing commercial insurance or a pension. Therefore, the proportion of business assets in a household’s total net assets and welfare spending in total expenditure are indicators of the self-respect goal.
Measurement of livelihood strategy
LS selection is very diverse in terms of time, space, and family. To deconstruct this differentiation, it is necessary to explore LS classification. We classify LS based on households’ income from various income-generating activities (Jian, Mishra, and Zhu Citation2019), including wages, self-employment, property income, transfer income, agricultural income (crops and livestock), and other income (see Appendix ). Consistent with previous studies (Liu et al. Citation2020; Zhou et al. Citation2021), we categorize LS as agricultural, non-agricultural, and diversification strategies. Specifically, the agricultural strategy means over 75% of family income comes from agricultural activities. The non-agricultural strategy means over 75% of family income comes from non-agricultural activities. The diversification strategy means that a household engages in more than one livelihood activity, but no activity brings in over 75% of total income.
Methods
In livelihood studies, the multinomial logit (MNL) is the most common method to analyse factors influencing LS selection (Huang et al. Citation2021). Based on the utility theory, household chooses an LS
from a set of livelihood strategies
when
The unobserved-heterogeneity model for both the fixed effects and random effects estimator can be written in utility-maximization form as:
The response probabilities of the MNL model for household
with
choose LS
at time
are:
where refers to the probability that household
chooses LS
at time
; and
are households’ livelihood goal indices at time
. The three livelihood goal indices exist at the same time.
refers to a vector of control variables influencing LS selection at time
. The effects of LGs on LS selection are captured by
. LS is dynamic, and the previous LS can influence the current LS selection because of changes in external or internal shocks, transformation, and opportunities (Jian, Mishra, and Zhu Citation2019; Jiao, Pouliot, and Walelign Citation2017; Walelign et al. Citation2017). Finally, EquationEquation (3)
(3)
(3) can be rewritten as:
In the panel data, compared with the random effects (RE) model, the fixed effects (FE) model can control time-invariant differences and minimize bias from omitted time-invariant factors. Therefore, we chose the FE model to estimate the relationships between LGs and LS selection. To identify the consistency of the estimates, we use the RE model, the correlated random effects (CRE) model, and further sub-sample analysis in different economic regions and years for a robustness check. Unlike the RE model, the CRE model relaxes the assumption that the observed variables are uncorrelated with the unobserved (latent) variables, so it can minimize time-invariant unobserved heterogeneity in the fixed effects model and control the incidental parameters problem (Wooldridge Citation2019). In this case, standard errors are clustered at the household level to reduce bias from heteroscedasticity and serial correlation. Further, we split samples into different economic regions and years and separately estimate the relationship between households’ LGs and LS selection.
Selection bias and unobserved attributes could produce inconstant estimates. Therefore, we use the two-step instrumental probit regression model (IV probit) to address the endogeneity problem (Dzanku Citation2018). Specifically, we divide three types of LS into three dummy variables and used the IV probit regression to estimate the relationships between LG indices and each kind of LS. In this estimation, we add time-variant and location-variant variables to minimize bias. The instrumental variables we use in this study have two parts. First, average values of LG indices in the same county are considered. Farmers’ behaviour is influenced by their social interactions (Wuepper et al. Citation2023). Therefore, other people’s goals would influence households’ goal-setting but do not affect their LS selection. Secondly, households’ LG indices in the previous term are considered. Goal-setting is dynamic, indicating that the goal achieved influences goal-setting for the near future (Icek and Kruglanski Citation2019). Therefore, households’ goal in the previous term affects their goal-setting in the next round but does not influence their LS selection in the next round. Therefore, the selected instrumental variables are households’ LG index in the previous term and the average value of LG indices in the same county in the current and the previous term.
IV. Results and discussion
Descriptive results
Appendix presents the descriptive statistics of LG indicators and Chinese rural households’ characteristics. Appendix and present the LS distribution for rural China. Overall, the non-agricultural strategy was the primary LS in rural China (47.74%), followed by the diversification strategy (38.67%). In 2010, the diversification strategy was the most popular choice (44.02%), followed by the non-agricultural strategy (36.20%). From 2012 to 2018, the preference reversed to the non-agricultural strategy.
Validity tests results
This section discusses the validity tests of the indicators used to construct LGs measurement, including the PCA and MNL analysis results. The indicators are valid and may be used to calculate LG indices only when they pass the PCA analysis and significantly affect households’ LS selection.
Principal component analysis results
presents the selected indicators of LGs based on PCA. Following Matter, Boillat, and Ifejika Speranza (Citation2021), indicators with KMO values over 0.5 and cumulative proportions over 50% in PCA are kept. Two extracted indicators reflect 53.4% of a household’s survival goal (KMO = 0.50), so poverty and the Engel coefficient are used to measure a household’s survival goal level. The cumulative proportion is highest for the indicators measuring the security goal after deleting durable assets (KMO = 0.50, cumulative proportion = 56.6%). Therefore, the proportions of land assets and residences are kept to calculate the security goal index. As the four indicators of the self-respect goal satisfy the cumulative proportion and KMO value (KMO = 0.50, the cumulative proportion = 54.2%), all four indicators (social spending, commercial insurance, business assets, and social status) are kept to measure a household’s self-respect goal for further selection.
Table 2. Validity tests of livelihood goals’ indicators: The principal component analysis results.
Multinomial logit results
The principal hypothesis of this study is that LGs influence households’ LS selection. Thus, the LGs indicators should relate to the LS. We use pooled MNL estimation and the fixed effects MNL model to identify the relationship between the indicators and households’ LS selection (see ).
Appendix shows that poverty and the Engel coefficient are critical indicators in measuring the survival goal index. These two indicators significantly affect LS selection at the 5% confidence level. Households’ proportions of land and residence assets, comprising the security goal, significantly influence households’ LS selection at the 1% confidence level, showing that these indicators are essential in measuring the security goal index. However, one of the four indicators of the self-respect goal, commercial insurance, is insignificant in the pooled MNL estimation and the fixed effects MNL model. Thus commercial insurance is excluded. The self-respect goal index can be calculated by the proportions of social spending, business assets, and households’ social status. We then calculate the survival, security, and self-respect goal indices based on the selected indicators using EquationEquation (1)(1)
(1) .
Empirical results
The impact of livelihood goals on livelihood strategy selection
Given that the coefficients estimated by the MNL model cannot be explained directly, following previous studies (Funk et al. Citation2020; Jian, Mishra, and Zhu Citation2019), we calculate the marginal effects of these factors to provide a better understanding of how households’ LGs affect their LS selection. Specifically, we calculate the marginal effects of the three LG indices on the three types of LS based on the estimates of the fixed effects MNL model (see ). shows that households struggling to optimize subsistence tend to choose the diversification strategy, followed by the agricultural strategy. When families have a 1% higher probability of pursuing the survival goal, the likelihood of selecting the diversification and agricultural strategies increases by 7.3% and 2.5%, respectively. Comparably, rural households in the survival stage have a 9.8% lower willingness to be involved in the non-agricultural strategy. Our results indicate that households prefer the lower-risk or risk-spreading strategies when struggling on the edge of subsistence. Consistent with previous studies, this result indicates diversifying households’ assets into the agricultural and non-agricultural sectors enables families to balance expected returns and risk exposure given their constraints (Gautam and Andersen Citation2016).
Table 3. Marginal effects of livelihood goal indices on livelihood strategy selection: The fixed effects multinomial logit model result.
The non-agricultural strategy is chosen when households have a higher propensity to pursue the security goal. If families have a 1% higher likelihood of following the security goal, the possibility of choosing the non-agricultural strategy rises by 16.7%. The tendency to be involved in the agricultural and diversification strategies decreases by 11.7% and 5%, respectively. Consistent with a previous study on the labour productivity gap between the farm and non-farm sectors (Djido and Shiferaw Citation2018), shows that the non-agricultural strategy dominates with the highest income-generating ability. This result echoes the findings of Ding and Abdulai (Citation2020), who found the income-increasing space of the agricultural strategy is constrained by farm size. In comparison, non-agricultural livelihood activities have fewer constraints in diversifying and growing income. Combined with the result in , this result indicates that the higher income-generating strategy is chosen when households transit from pursuing survival maximization to security maximization.
A similar choice happens with households in the self-respect stage. With a 1% higher level in the self-respect goal index, Chinese rural households have a 2.4% higher probability of choosing the non-agricultural strategy and a 1.1% lower possibility of engaging in the agricultural strategy. However, a higher self-respect goal index does not significantly influence their willingness to select the diversification strategy. Comparing the marginal effects of the survival goal and security goal indices, the marginal effects of the self-respect goal index on LS selection are lower, indicating that households are more flexible in LS choice when they focus on realizing the self-respect goal.
The marginal effects of the three LG indices on LS selection are shown in . It demonstrates that, with an increasing survival goal index, the probability of choosing the diversification strategy increases more dramatically than for the agricultural strategy. Conversely, the possibility of selecting the non-agricultural strategy decreases. The opposite trend shows when households shift to the security goal; the marginal effects of choosing the agricultural or diversification strategies tend to converge with a rise in the security goal index. Comparably, with an increase in the self-respect goal index, the marginal effects of selecting the non-agricultural strategy increase steadily. In contrast, the marginal effects of choosing the agricultural and diversification strategies gradually decrease.
Figure 3. The probabilities of different livelihood strategy selections with changes in livelihood goal indices.
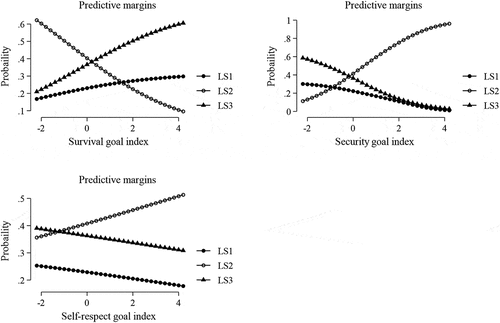
Overall, from the high survival goal index to the high self-respect goal index, the potential choice of LS transits from the agricultural strategy to the non-agricultural strategy. In this process, the diversification strategy is households’ transformation choice. This result echoes a previous study that off-farm transition is the most common outcome in rural China (Zhu, Sun, and Song Citation2022). Our findings are also consistent with Baird and Gray (Citation2014), who find that diversification is the main means to increase income, reduce risk, and transit livelihoods, especially for households in low living states.
Other variables also influence the LS selection. At an individual level, household heads’ age and gender influence households’ LS choice. shows that older household heads prefer the diversification strategy and are less likely to choose the non-agricultural strategy. However, different from previous studies, that male household heads have a stronger motivation and ability to engage in non-farm activities (Rahut, Abdul Mottaleb, and Ali Citation2018); female household heads have a higher probability of engaging in the diversification strategy (Helmy Citation2020), we show that female household heads more likely to choose the non-agricultural strategy than male. A possible reason could be the possessed assets differences between male and female heads. A household led by a female leader has less land, residence assets, and weaker social networks than a male (Wondimu, Delelegn, and Dejene Citation2022). This asset-lacking state encourages female household heads to pursue more lucrative job opportunities away from home (Sarah Citation2019).
At the household level, the previous LS choice, family size, dependency ratio, and land status influence households’ LS selection. shows that LS selection has a lagged effect, families are more likely to adopt the same LS if they adopt agricultural or non-agricultural strategies in the last year but are less likely to keep the diversification strategy if they use it in the previous term. In addition, households prefer the non-agricultural strategy when they have a larger family. This could be because a large family creates higher livelihood resilience (Zhao et al. Citation2022). However, if they have more children to raise, their willingness to select the non-agricultural strategy is 9.5% lower. This result shows that a higher dependency ratio encourages households to adopt the lower-risk LS because the higher financial burden weakens households’ capability to deal with external risk (Speranza, Chinwe, and Rist Citation2014). Land status also influences households’ LS choices. Households’ willingness to adopt the agricultural strategy decreases when their land is expropriated by the government, indicating that land acquisition promotes households to transit their LS (Nguyen and Kim Citation2020). Households that proactively rent out their land are less likely to engage in the agricultural strategy but prefer the non-agricultural strategy.
Robustness tests
Appendix show that the RE and CRE regression results are consistent with the FE model: as the survival goal index rises, the possibility of choosing the agricultural and diversification strategies increases and the possibility of selecting the non-agricultural strategy decreases. As the indices of the security and self-respect goals rise, the probability of choosing the non-agricultural strategy increases and the likelihood of selecting the agricultural strategy decreases. However, different from the results of the full sample and the FE model, the results of the RE and CRE models reflect that households with a higher survival goal index are more likely to choose the agricultural strategy than the diversification strategy. A possible explanation is that households tend to maintain a specific farming scale and temporarily diversify their agricultural planting structure to achieve diversification (Zhu, Sun, and Song Citation2022), especially for subsistence farmers (Wang et al. Citation2020). Therefore, although non-farming diversification is a transformation strategy, households take years to reallocate assets to realize it. Hence, when we use the RE and CRE models that assume the individual effect is exogenous, the models could overestimate households’ willingness to select the diversification strategy.
We also present the results from different economic regions (see Appendix A2) and year (Appendix A3). shows, regardless of the regions where the households are, families with higher survival goal indices are more likely to choose the agricultural strategy and less likely to select the non-agricultural strategy. The reverse applies when families have a higher security goal index. More diverse situations transpire when households are at the self-respect goal stage: households in the northeast, coastal, middle reaches areas, and southwest China have a significantly higher possibility of choosing the diversification strategy. However, for households in northwest China, the possibility of adopting the non-agricultural strategy is higher than the diversification strategy when pursuing the self-respect goal. shows the detailed heterogeneous results of the relationship between LGs and LS selection in different study periods. Previous studies showed that diversification is the better choice for low-income people to mitigate vulnerability (Helmy Citation2020), and non-farm diversification can improve households’ well-being (Gautam and Andersen Citation2016). We show that, when households’ survival goal index is relatively low, they are more likely to choose the diversification strategy than other LS. However, a tougher subsistence level leads to the likelihood of adopting the agricultural strategy exceeding the probability of selecting the diversification strategy from 2010 to 2012. This result echoes the importance of the safety net role of agriculture (Wang et al. Citation2020). In line with the RE and CRE results, households with the security goal have a stronger and consistent preference for the non-agricultural strategy over the study period (2010–2018). Like the full sample results, households with a self-respect goal have more flexible choices in LS.
Endogeneity tests
To address the endogeneity problem, we set the three types of LS as three dummy variables and use the logit model to estimate the Rho value of each LS. Appendix shows that, for any LS, the Rho value is less than 0.1, indicating that we may use the pooled instrumental probit regression model to address any endogeneity.
shows that the IV probit results are consistent with the fixed effects MNL regression. Households with a higher survival goal index prefer to choose the agricultural and diversification strategies, but they are more willing to engage in the non-agricultural strategy as their security and self-respect goal indices rise. This result echoes the risk aversion preference of low-income farmers and shows that households with more resources and assets are more able to mitigate risk (Tesfaye and Tirivayi Citation2020). Wald tests for each type of LS indicate that livelihood goal indices are endogenous in LS selection. For each LS, the estimated results with instrumental variables (including the lagged LG indices, the average LG indices in the same county, and the corresponding lagged values) reject the weak IV hypothesis. Therefore, there is no weak IV problem. Furthermore, the Amemiya-Lee-Newey minimum Chi-square statistic is insignificant, indicating that these instrumental variables are valid and no overidentification problem exists. Therefore, the study’s results are robust and credible.
Table 4. Marginal effects of livelihood goal indices on livelihood strategy selection: Two-step instrumental probit regression results.
V. Conclusions, implications and limitations
Conclusions
LG plays a crucial role in LS selection, but how LG affects LS selection remains underexplored. Using CFPS data (2010–2018), we first incorporate LGs into the SLA framework based on the utility maximization theory to investigate the relationship between livelihood assets, LGs, and LSs. Next, we develop a measurement of LGs based on the literature review, PCA, and MNL analysis. Then, we explore the nexus between LGs and LS in rural China using the fixed effects MNL model. Finally, we use the RE and CRE models to check our results’ robustness.
The results showed that households’ LGs are heterogeneous and influence households’ LS selection. LGs can be classified as survival, security, and self-respect and are defined by seven indicators. Specifically, poverty and the Engel coefficient reflect the survival goal level of households. The security goal is characterized by accumulating tangible land and house assets. The self-respect goal is the highest LG; households’ social spending, business assets, and social status can be used to evaluate it. Furthermore, families with the survival goal are more likely to choose the diversification and agricultural strategies but less likely to select the non-agricultural strategy. In contrast, the non-agricultural strategy is the priority when households pursue security or self-respect maximization. Families with a higher level of the self-respect goal index are more flexible in LS selection. Further analysis shows that, regardless of the region, preferences are consistent in selecting the agricultural strategy in the survival goal stage and the non-agricultural strategy in the security goal stage. Likewise, households in the self-respect goal stage have more variety in LS selection. Diversification is the transformation strategy from the low-level survival goal to the high-level self-respect goal and from a low-income agricultural strategy to a high-income non-agricultural strategy. This trend gradually happens over time.
Policy implications
Our findings suggest that households’ LGs and LS have an inner nexus. The government can use this nexus to satisfy households’ diverse LGs, upgrade households’ LGs, and encourage households to choose more sustainable LS. Specifically, there are two approaches to combine households’ LGs with rural development programmes. First, our findings show that LGs can be measured by different indicators, highlighting that adjusting the indicators’ level could be an essential, efficient way to manage households’ living expectations. For example, the government could subsidize poor people to lower their food expenditure so that families have a lower survival goal index and stronger motivation to pursue higher LGs. Second, considering the relationship between LGs and LS, the government could encourage rural households to choose the LS to match their goals and abilities or integrate households’ LGs into local livelihood programmes. For example, for households living for survival maximization, the government could provide some transition positions to encourage them to distribute their assets towards on-farm or non-farm livelihood activities to gain higher revenue with lower risk than engaging purely in the agricultural strategy.
Limitations
As with any empirical study, there are limitations to this study. First, the LGs are measured from an ex-post perspective, which assumes households have realized their LGs. Future studies could improve the measurement of LGs by combining subjective descriptions with objective indicators. Furthermore, limited by the endogeneity analysis method for the categorical dependent variables with continuous endogenous variables in the panel dataset, we divide LS into three dummy variables to conduct the IV probit estimation separately. Although the result is consistent with the FE results, the marginal effects among the different dummy variables cannot be compared. Future studies could apply other methods to address the endogeneity problem and further identify the relationship between LGs and LS.
Disclosure statement
No potential conflict of interest was reported by the author(s).
References
- Arrondel, L., L. Bartiloro, P. Fessler, M. Haliassos, and P. Lindner. 2016. “How Do Households Choose to Allocate Their Wealth? Some Stylized Facts Derived from the Eurosystem Household Finance and Consumption Survey.” International Journal of Central Banking 12 (October): 1–45. http://econpapers.repec.org/RePEc:ijc:ijcjou:y:2016:q:2:a:4%0Ahttp://econpapers.repec.org/article/ijcijcjou/y_3a2016_3aq_3a2_3aa_3a4.htm.
- Baird, T. D., and C. L. Gray. 2014. “Livelihood Diversification and Shifting Social Networks of Exchange: A Social Network Transition?” World Development 60:14–30. https://doi.org/10.1016/j.worlddev.2014.02.002.
- Bai, H., Y. Yin, J. Addison, Y. Hou, L. Wang, and X. Hou. 2020. “Market Opportunities Do Not Explain the Ability of Herders to Meet Livelihood Objectives Over Winter on the Mongolian Plateau.” Journal of Arid Land 12 (3): 522–537. https://doi.org/10.1007/s40333-020-0122-6.
- Berkhout, E. D., R. A. Schipper, A. Kuyvenhoven, and O. Coulibaly. 2010. “Does Heterogeneity in Goals and Preferences Affect Efficiency? A Case Study of Farm Households in Northern Nigeria.” Agricultural Economics 41 (3–4): 265–273. https://doi.org/10.1111/j.1574-0862.2010.00449.x.
- Berkhout, E. D., R. A. Schipper, H. Van Keulen, and O. Coulibaly. 2011. “Heterogeneity in Farmers’ Production Decisions and Its Impact on Soil Nutrient Use: Results and Implications from Northern Nigeria.” Agricultural Systems 104 (1): 63–74. https://doi.org/10.1016/j.agsy.2010.09.006.
- Chambers, R., and B. P. Ghildyal. 1984. “To the Hands of the Poor: Water, Trees and Land.” 14. Rural Poverty and Resource. Discussion Paper Series.
- Démurger, S., M. Fournier, and W. Yang. 2010. “Rural Households’ Decisions Towards Income Diversification: Evidence from a Township in Northern China.” China Economic Review 21:S32–44. https://doi.org/10.1016/j.chieco.2010.05.007.
- Díaz-Montenegro, J., E. Varela, and J. M. Gil. 2018. “Livelihood Strategies of Cacao Producers in Ecuador: Effects of National Policies to Support Cacao Farmers and Specialty Cacao Landraces.” Journal of Rural Studies 63 (August): 141–156. https://doi.org/10.1016/j.jrurstud.2018.08.004.
- Ding, Z., and A. Abdulai. 2020. “An Analysis of the Factors Influencing Choice of Microcredit Sources and Impact of Participation on Household Income.” Journal of International Development 32 (4): 505–525. https://doi.org/10.1002/jid.3466.
- Djido, A. I., and B. A. Shiferaw. 2018. “Patterns of Labor Productivity and Income Diversification – Empirical Evidence from Uganda and Nigeria.” World Development 105:416–427. https://doi.org/10.1016/j.worlddev.2017.12.026.
- Dzanku, F. M. 2018. “Rational but Poor? An Explanation for Rural Economic Livelihood Strategy.” Journal of Agricultural Economics 69 (2): 365–381. https://doi.org/10.1111/1477-9552.12245.
- Fahad, S., H. Nguyen-Thi-Lan, D. Nguyen-Manh, H. Tran-Duc, and N. To-The. 2023. “Analyzing the Status of Multidimensional Poverty of Rural Households by Using Sustainable Livelihood Framework: Policy Implications for Economic Growth.” Environmental Science and Pollution Research 30 (6): 16106–16119. https://doi.org/10.1007/s11356-022-23143-0.
- Felkner, J. S., H. Lee, S. Shaikh, A. Kolata, and M. Binford. 2022. “The Interrelated Impacts of Credit Access, Market Access and Forest Proximity on Livelihood Strategies in Cambodia.” World Development 155:105795. https://doi.org/10.1016/j.worlddev.2021.105795.
- Funk, C., A. R. Sathyan, P. Winker, and L. Breuer. 2020. “Changing Climate - Changing Livelihood: Smallholder’s Perceptions and Adaption Strategies.” Journal of Environmental Management 259 (December 2018): 109702. https://doi.org/10.1016/j.jenvman.2019.109702.
- Gautam, Y., and P. Andersen. 2016. “Rural Livelihood Diversification and Household Well-Being: Insights from Humla, Nepal.” Journal of Rural Studies 44:239–249. https://doi.org/10.1016/j.jrurstud.2016.02.001.
- George, F., P. Fidelman, and T. F. Smith. 2017. “The Impacts of Environmental and Socio-Economic Stressors on Small Scale Fisheries and Livelihoods of Fishers in Ghana.” Applied Geography 89 (November 2016): 1–11. https://doi.org/10.1016/j.apgeog.2017.09.009.
- George, S., and D. Ngaruko. 2017. “The Link Between Farm Household Objectives and Investment in Irrigation Scheme: Implications for Collective Action Coordination Efficiency.” African Journal of Economic Review 5 (1): 110–125.
- Gordon, A., C. Conroy, S. Iyengar, Lobo, V., Rani, U., and Rao, G.B. 2001. Household Livelihood and Coping Strategies in Semi-Arid India : Synthesis of Macro- and Micro- Level Findings. Annex A of the Final Technical Report of NRSP Project, 7558.
- Helmy, I. 2020. “Livelihood Diversification Strategies: Resisting Vulnerability in Egypt.” 441. GLO Discussion Paper. www.econstor.eu.
- Huang, L., L. Yang, N. Thị Tuyến, N. Colmekcioglu, and J. Liu. 2021. “Factors Influencing the Livelihood Strategy Choices of Rural Households in Tourist Destinations.” Journal of Sustainable Tourism 30 (4): 875–896. https://doi.org/10.1080/09669582.2021.1903015.
- Hua, Xiaobo, Jianzhong Yan, and Yili Zhang. 2017. “Evaluating the Role of Livelihood Assets in Suitable Livelihood Strategies: Protocol for Anti-Poverty Policy in the Eastern Tibetan Plateau, China.” Ecological Indicators 78: 62–74. https://doi.org/10.1016/j.ecolind.2017.03.009
- Ibrahim, S. S. 2022. “Livelihood Transition and Economic Well-Being in Remote Areas Under the Threat of Cattle Rustling in Nigeria.” GeoJournal, (1): 1–16. https://doi.org/10.1007/s10708-022-10583-x.
- Icek, A., and A. W. Kruglanski. 2019. “Reasoned Action in the Service of Goal Pursuit.” Psychological Review 126 (5): 774–786. https://doi.org/10.1037/rev0000155.
- Imai, K. S., and J. You. 2014. “Poverty Dynamics of Households in Rural China.” Oxford Bulletin of Economics and Statistics 76 (6): 898–923. https://doi.org/10.1111/obes.12044.
- Jakoby, O., M. F. Quaas, B. Müller, S. Baumgärtner, K. Frank, and J. Jones. 2014. “How Do Individual Farmers’ Objectives Influence the Evaluation of Rangeland Management Strategies Under a Variable Climate?” Journal of Applied Ecology 51 (2): 483–493. https://doi.org/10.1111/1365-2664.12216.
- Jian, Z., A. K. Mishra, and P. Zhu. 2019. “Identifying Livelihood Strategies and Transitions in Rural China: Is Land Holding an Obstacle?” Land Use Policy 80 (October 2018): 107–117. https://doi.org/10.1016/j.landusepol.2018.09.042.
- Jiao, X., M. Pouliot, and S. Z. Walelign. 2017. “Livelihood Strategies and Dynamics in Rural Cambodia.” World Development 97:266–278. https://doi.org/10.1016/j.worlddev.2017.04.019.
- Kundu, A., and S. Das. 2021. “Occupational Diversification as Livelihood Strategy Among the Agricultural Labour Households of West Bengal, India.” Management and Labour Studies 47 (1): 40–58. https://doi.org/10.1177/0258042X211026149.
- Liang, F., C. Lin, and S.-H. Lin. 2022. “Farmers’ Livelihood, Risk Expectations, and Homestead Withdrawal Policy: Evidence on Jinjiang Pilot of China.” International Journal of Strategic Property Management 26 (1): 56–71. https://doi.org/10.3846/ijspm.2022.16174.
- Liu, W., L. Jie, L. Ren, X. Jie, L. Cong, and L. Shuzhuo. 2020. “Exploring Livelihood Resilience and Its Impact on Livelihood Strategy in Rural China.” Social Indicators Research 150 (3): 977–998. https://doi.org/10.1007/s11205-020-02347-2.
- Matter, S., S. Boillat, and C. Ifejika Speranza. 2021. “Buffer-Capacity-Based Livelihood Resilience to Stressors—An Early Warning Tool and Its Application in Makueni County, Kenya.” Frontiers in Sustainable Food Systems 5 (July): 1–16. https://doi.org/10.3389/fsufs.2021.645046.
- Musumba, M., C. A. Palm, A. M. Komarek, P. K. Mutuo, and B. Kaya. 2022. “Household Livelihood Diversification in Rural Africa.” Agricultural Economics (United Kingdom), (October 2021): 1–11. https://doi.org/10.1111/agec.12694.
- Neef, A., L. Benge, B. Boruff, N. Pauli, E. Weber, and R. Varea. 2018. “Climate Adaptation Strategies in Fiji: The Role of Social Norms and Cultural Values.” World Development 107:125–137. https://doi.org/10.1016/j.worlddev.2018.02.029.
- Nguyen, Q., and D. C. Kim. 2020. “Reconsidering Rural Land Use and Livelihood Transition Under the Pressure of Urbanization in Vietnam: A Case Study of Hanoi.” Land Use Policy 99 (July): 104896. https://doi.org/10.1016/j.landusepol.2020.104896.
- Rahut, D. B., K. Abdul Mottaleb, and A. Ali. 2018. “Rural Livelihood Diversification Strategies and Household Welfare in Bhutan.” European Journal of Development Research 30 (4): 718–748. https://doi.org/10.1057/s41287-017-0120-5.
- Sarah, A. L. 2019. “Household Livelihood Diversification and Gender: Panel Evidence from Rural Kenya.” Journal of Rural Studies 69 (February 2017): 156–172. https://doi.org/10.1016/j.jrurstud.2019.03.001.
- Sarker, M. N. I., Cao, Q., M. Wu, Hossin, M. A., Alam, G. M. M., and R. C. Shouse. 2019. “Vulnerability and Livelihood Resilience in the Face of Natural Disaster: A Critical Conceptual Review.” Applied Ecology and Environmental Research 17 (6): 12769–12785. https://doi.org/10.15666/aeer/1706_1276912785.
- Speranza, I., U. W. Chinwe, and S. Rist. 2014. “An Indicator Framework for Assessing Livelihood Resilience in the Context of Social-Ecological Dynamics.” Global Environmental Change 28 (1): 109–119. https://doi.org/10.1016/j.gloenvcha.2014.06.005.
- Start, D., and C. Johnson. 2004. “Livelihood Options? The Political Economy of Access, Opportunity and Diversification.” Working Paper 233. chrome-extension://efaidnbmnnnibpcajpcglclefindmkaj/https://cdn.odi.org/media/documents/2470.pdf.
- Sun, F., L. Wang, S. Cao, and Y. Zhang. 2023. “How Farmland Circulation Affects Household Financial Vulnerability in China: The Chain Mediation Effect of Labor Transfer and Financial Literacy.” Frontiers in Sustainable Food Systems 7:7. https://doi.org/10.3389/fsufs.2023.1102189.
- Tambe, S. 2022. Teaching and Learning Rural Livelihoods: A Guide for Educators, Students, and Practitioners. Cham, Switzerland: Springer Nature. https://doi.org/10.1007/978-3-030-90491-3.
- Tesfaye, W., and N. Tirivayi. 2020. “Crop Diversity, Household Welfare and Consumption Smoothing Under Risk: Evidence from Rural Uganda.” World Development 125:104686. https://doi.org/10.1016/j.worlddev.2019.104686.
- Wale, E., M. Andrias Nkoana, and E. Mkuna. 2022. “Climate Change-Induced Livelihood Adaptive Strategies and Perceptions of Forest-Dependent Communities: The Case of Inanda, KwaZulu-Natal, South Africa.” Trees, Forests and People 8 (March): 100250. https://doi.org/10.1016/j.tfp.2022.100250.
- Walelign, S. Z., M. Pouliot, H. Overgaard Larsen, and C. Smith-Hall. 2017. “Combining Household Income and Asset Data to Identify Livelihood Strategies and Their Dynamics.” The Journal of Development Studies 53 (6): 769–787. https://doi.org/10.1080/00220388.2016.1199856.
- Wang, H., J. Fidrmuc, Q. Luo, and M. Luo. 2020. “Exploring the Determinants of On-Farm Transitions: Evidence from Rural China.” Applied Economics 52 (52): 5667–5686. https://doi.org/10.1080/00036846.2020.1770194.
- Wondimu, H., W. Delelegn, and K. Dejene. 2022. “What Do Female-Headed Households’ Livelihood Strategies in Jimma City, South West Ethiopia Look Like from the Perspective of the Sustainable Livelihood Approach?” Cogent Social Sciences 8 (1). https://doi.org/10.1080/23311886.2022.2075133.
- Wooldridge, J. M. 2019. “Correlated Random Effects Models with Unbalanced Panels.” Journal of Econometrics 211 (1): 137–150. https://doi.org/10.1016/j.jeconom.2018.12.010.
- Wuepper, D., S. Bukchin-Peles, D. Just, and D. Zilberman. 2023. “Behavioral Agricultural Economics.” Applied Economic Perspectives and Policy 1–12. https://doi.org/10.1002/aepp.13343.
- Xiaohua, Y. 2018. “Engel Curve, Farmer Welfare and Food Consumption in 40 Years of Rural China.” China Agricultural Economic Review 10 (1): 65–77. https://doi.org/10.1108/CAER-10-2017-0184.
- Zhang, L., Y. Dong, C. Liu, and Y. Bai. 2018. “Off-Farm Employment Over the Past Four Decades in Rural China.” China Agricultural Economic Review 10 (2): 190–214. https://doi.org/10.1108/CAER-11-2017-0212.
- Zhao, X., H. Chen, H. Zhao, and B. Xue. 2022. “Farmer Households’ Livelihood Resilience in Ecological-Function Areas: Case of the Yellow River Water Source Area of China.” Environment, Development and Sustainability 24 (7): 9665–9686. https://doi.org/10.1007/s10668-021-01827-w.
- Zhao, H., X. Guo, and N. Peng. 2022. “What Catalyzes the Proactive Recovery of Peasants from the COVID-19 Pandemic? A Livelihood Perspective in Ningqiang County, China.” International Journal of Disaster Risk Reduction 73 (March): 102920. https://doi.org/10.1016/j.ijdrr.2022.102920.
- Zhou, W., S. Guo, X. Deng, and X. Dingde. 2021. “Livelihood Resilience and Strategies of Rural Residents of Earthquake-Threatened Areas in Sichuan Province, China.” Natural Hazards 106 (1): 255–275. https://doi.org/10.1007/s11069-020-04460-4.
- Zhu, J., Y. Sun, and Y. Song. 2022. “Household Livelihood Strategy Changes and Agricultural Diversification: A Correlation and Mechanism Analysis Based on Data from the China Family Panel.” Land 11 (5): 5. https://doi.org/10.3390/land11050685.
Appendix
Section I Livelihood strategy classification
Figure A2. The probability of livelihood strategy selection within regions. (a) The probability of LS selection with survival goal index changes in different regions. (b) The probability of LS selection with security goal index changes in different regions. (c) The probability of LS selection with self-respect goal index changes in different regions. LS1, LS2, and LS3 refer to the agricultural, non–agricultural, and diversification strategies, respectively.
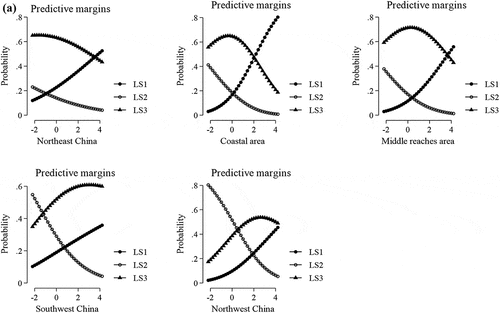
Figure A3. The probability of livelihood strategy selection within years. (a) the probability of LS selection with survival goal index change (2010–2018). (b) the probability of LS selection with security goal index change (2010–2018). (c) the probability of LS selection with self-respect goal index change (2010–2018). LS1, LS2, and LS3 refer to the agricultural, non-agricultural, and diversification strategies, respectively.
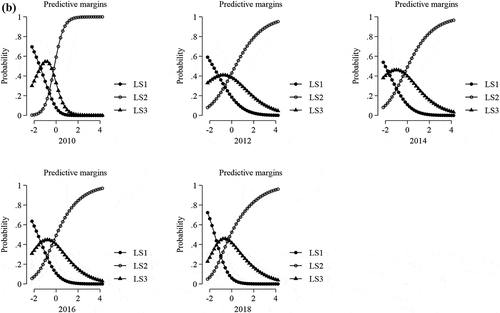
Table A1. Six income sources to classify livelihood strategies.
Section II Descriptive statistics
The descriptive statistics show that 15.6% of rural households were in poverty from 2010 to 2018 (see ). The average Engel Coefficient was 0.182, lower than the international poverty level. Chinese rural households’ asset allocation was extraordinarily different. The average percentage of residence value in total net assets is up to 45.9%. In addition, around one-quarter of assets are invested in land and commercial insurance. The percentage of durable assets accounts for only 7.2% of total net assets. shows that household business operation assets are almost three times total net assets. About half of families participate in formal or informal organizations and are satisfied with their social status, with 2.981 self-reported scores on a scale of 1-5.
Chinese rural households do not favour renting or lending out their land. Only 10.2% of rural households rent out their land, and only 5.7% have land acquisition. The average family size is 3.78 persons. However, around a quarter of the household members need to be raised by other families. Over half of the rural households live in the Coastal and Middle Reaches areas. The average age of household head is 50.04 years old, 62% are male, and 87.1% are married. Their average education level is 7.32 years, and they have an optimistic health condition (average self-reported score is 2.9).
Table A2. Descriptive statistics of the variables.
Table A3. Livelihood strategy distribution in different years.
Section III Empirical results
Table A4. Multinomial logit regression result of livelihood goal indicators on livelihood strategy selection.
Table A5. Margins effects of livelihood goal indices on livelihood strategy selection: the random effects multinomial logit regression result.
Table A6. Margins effects of livelihood goal indices on livelihood strategy selection: the correlated random effects regression result.
Table A7. Margins effects of livelihood goal indices on livelihood strategy selection: the logit model regression results for each livelihood strategy.