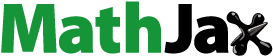
ABSTRACT
We investigate whether the impacts of financial development and liberalization on economic growth vary across different stages of development, which remains unaddressed in the literature on the finance–growth nexus. In the analysis, a comparative meta-analysis was performed for studies of advanced, developing, and emerging market economies, using 6,135 estimates extracted from a total of 379 previous works. The results have significant implications for studies of the finance–growth nexus. In particular, the impacts of financial development and liberalization on economic growth do not vary across different stages of development.
I. Introduction
Whether financial development and liberalization contribute to economic growth has long been one of the most frequently debated subjects among economists, including Joseph Alois Schumpeter (Citation1911), who, in his monumental work of economic development theory, claimed that credit extended to the entrepreneur for purposes of innovation is an element of economic development (Schumpeter Citation1911). In fact, there are a massive number of empirical studies that have examined the finance–growth nexus. Their subjects, however, are limited to certain countries and regions. Moreover, although this topic has considerable significance in the field of the relationship among economic growth and financial development as well as financial liberalization, there is no theoretical model for the financial growth-enhancing effects by stage of economic development.
If we take these situations into account, it is desirable to derive a conclusion regarding the finance–growth nexus from former empirical studies by meta-analysis. Arestis et al. (Citation2015), Valickova et al. (Citation2015), as well as Bumann et al. (Citation2013) are the pioneer studies of meta-analysis in the field of the effect of financial development on economic growth, and other subsequent articles have been published on this subject. More recently, Ono and Iwasaki (Citation2022) performed a meta-analysis of the effect on economic growth of financial development and liberalization in European emerging markets. However, the growth-enhancing effects of finance in different stages of economic development have not been comprehensively investigated in previous meta-analysis studies.
In this study, we apply a meta-analysis to examine the financial growth-enhancing effects for studies of advanced, developing, and emerging market economies. The most ideal method is to synthesize the interaction terms of variables that are the proxies of economic growth with those of financial development, using meta-analysis. However, no former studies introduce the above-mentioned variables. Therefore, as the second-best solution, in this paper, we will attempt to synthesize and compare reported estimates of the growth-enhancing effects of financial development and liberalization in advanced economies, developing economies, and post-communist emerging markets, using the advanced techniques of meta-analysis.Footnote1
This classification of economies has significance in the following aspects. First, the categories of advanced and developing economies differentiate between the economic development stages of ordinary market economies. Furthermore, the category of post-communist emerging markets allows us to evaluate the effects of the economic transition to a market economy. As Ono and Iwasaki (Citation2022) indicate, there would be no doubt that people’s demand for goods and services was not fully satisfied in the initial stage of the economic transition, as compared to a later time. For this reason, in the initial stage of transition, there were relatively large numbers of highly profitable businesses, and business’s demand for funds could potentially be quite high as well. On the other hand, unlike during the time of a planned economy, financial institutions were able to decide on their own to lend funds to highly profitable businesses.
In this paper, we will test the following (null) hypothesis through a comparative meta-analysis of 6,135 estimates extracted from a total of 379 previous works:
Hypothesis:
The effect size of financial development and liberalization on economic growth does not vary among advanced economies, developing economies, and post-communist emerging markets.
Meta-synthesis of and testing for publication selection bias and genuine empirical evidence in the collected estimates indicated that the effect size of finance on growth may differ among the three study types. The observed disparity, however, is not significantly large, and meta-regression analysis showed that no statistically significant differences among the three could be detected when controlling for a set of study conditions simultaneously.
The remainder of this paper is organized as follows. Section II describes the data and methodology. Section III synthesizes estimates collected from the selected studies. Section IV tests for publication selection bias. Section V performs a meta-regression analysis (MRA) of literature heterogeneity. Section VI summarizes the major findings and concludes the paper.
II. Data and methodology
As the initial stage of testing the hypothesis, this section explains the procedure for selecting literature. It overviews research works included in the meta-analysis and then describes the methodology of meta-analysis.
Procedure for selecting literature and overview of selected studies for meta-analysis
To discover studies that empirically examined the growth-promoting effect of finance in advanced economies, developing economies, and post-communist emerging markets, we searched for relevant literature by accessing EconLit, Web of Science, and major academic press websites.Footnote2 In utilizing these electronic databases, we carried out an AND search of paper titles, using ‘finance’ or ‘financial’ and ‘growth’ as keywords. This title search yielded nearly 2,950 hits on EconLit and Web of Science and more than 630 additional hits on academic press websites. After eliminating duplication among the literature found through the mechanical searches, we found that, at a minimum, the literature in this field of study consisted of more than 2,850 works published in English.
Needless to say, the above 2,850 or more publications include numerous studies intended for purposes other than the empirical analysis of the effect of financial development and liberalization on macroeconomic growth. Therefore, as a second step, we looked closely at the content of each study to inspect whether it examined the finance–growth nexus in the target economies and, if so, whether it included estimates that can be subject to our meta-analysis. This narrowed the literature list to a total of 379 works, from the pioneering studies by Akinboade (Citation2000), Allen and Ndikumana (Citation2000), Bekaert et al. (Citation2001), Reisen and Soto (Citation2001), and Tsangarides (Citation2002) to the latest research outcomes by Asante et al. (Citation2023), Dahmani et al. (Citation2023), Kassi et al. (Citation2023), Li and Wye (Citation2023), Mtar and Belazreg (Citation2023), Nsor-Ambala and Amewu (Citation2023), and Öncel et al. (Citation2023).Footnote3 As shown in , of the 379 selected works, 81 papers deal with advanced economies, while 299 and 46 works treat developing economies and post-communist emerging markets, including China, respectively. This means that 43 publications cover two of three study types, and one publication covers all three.
Table 1. Number of selected works and descriptive statistics of the partial correlation coefficients, t-test, and Shapiro–Wilk normality test of collected estimates and univariate comparative analysis of three study types.
For the present study, we adopted an eclectic coding rule, in which we do not necessarily limit selection to one estimate per study; instead, multiple estimates are collected from the selected studies if and only if we can recognize notable differences from the viewpoint of empirical methodology in at least one item of the data type, regression equation, estimation period, estimator, composition of independent variables, and so forth. As a result of extracting estimates according to this coding policy, we obtained a total of 6,135 estimates from the 379 selected works. As reported in , among the 6,135 estimates, 1,396 and 4,232 present empirical results for advanced economies and developing economies, respectively. The remaining 507 are estimates obtained from studies of emerging markets.
Methodology of meta-analysis
This subsection provides a brief description of the meta-analysis methodology performed in this paper.
As stated in the introduction, we are interested in the regression coefficient capturing the impact of financial development on macroeconomic growth. Nevertheless, as Valickova et al. (Citation2015) pointed out, different research works use different units of measurement; hence, the estimates are not directly comparable. To summarize and compare the results from various studies, we need standardized effect sizes. The partial correlation coefficient (PCC) serves this aim.
A PCC is a unitless measure of the association of a dependent variable and the independent variable in question when other variables are held constant. It ranges between −1.0 and 1.0. When tk and dfk denote the t value and the degree of freedom of the k-th estimate, respectively, the PCC (rk) is calculated with the following equation:
We synthesize PCCs using the meta fixed-effect model and the meta random-effects model; according to the Cochran Q test of homogeneity and I2 and H2 heterogeneity measures, we adopt the synthesized effect size of one of these two models. In addition to this traditional synthesis method, we also utilize the unrestricted weighted least squares average (UWA) approach proposed by Stanley and Doucouliagos (Citation2017) and Stanley et al. (Citation2017) as a new synthesis method. The UWA is less subject to influence from excess heterogeneity than is the fixed-effect model. The UWA method regards as the synthesized effect size a point estimate obtained from the regression that takes the standardized effect size as the dependent variable and the estimation precision as the independent variable. Specifically, we estimate EquationEq. (2)(2)
(2) , in which there is no intercept term, and the coefficient, α, is utilized as the synthesized value of the PCCs:
where is a residual term. In theory, α in EquationEq. (2)
(2)
(2) is consistent with the estimate of the meta fixed-effect model.
Furthermore, Stanley et al. (Citation2017) proposed conducting a UWA of estimates, the statistical power of which exceeds the threshold of 0.80. They called this estimation method the weighted average of the adequately powered (WAAP). They stated that WAAP synthesis has less publication selection bias than the traditional random-effects synthesis. We adopt the WAAP estimate as the best synthesis value whenever available. Otherwise, the traditional synthesized effect size is used as the second-best reference value.
As the second stage of meta-analysis, we examine publication selection bias using a funnel plot, by conducting a goodness-of-fit test of proportional distribution, and by performing an MRA test procedure consisting of a funnel-asymmetry test (FAT), a precision-effect test (PET), and a precision-effect estimate with standard error (PEESE), which were proposed by Stanley and Doucouliagos (Citation2012) and have been used widely in previous meta-studies.
A funnel plot is a scatter plot with the effect size (in the case of this paper, the PCC) on the horizontal axis and the precision of the estimate (the inverse of the standard error 1/SE) on the vertical axis. In the absence of publication selection bias, effect sizes reported by independent studies vary randomly and symmetrically around the true effect size. Moreover, according to the statistical theory, the dispersion of effect sizes is negatively correlated with the precision of the estimate. Therefore, the shape of the plot must look like an inverted funnel. In other words, if the funnel plot is not bilaterally symmetrical but is deflected to one side, then an arbitrary manipulation of the study area in question is suspected, in the sense that estimates in favour of a specific conclusion (i.e. estimates with an expected sign) are more frequently published.
The goodness-of-fit test examines the proportional distribution of the reported estimates. The test is performed based on either the assumption that the true effect size is zero or the assumption that the selected meta-synthesis value approximates the true effect. By conducting this univariate test, we consider whether the estimates in question are distributed evenly around the true effect size.
The FAT–PET–PEESE procedure has been developed to test publication selection bias and the presence of genuine evidence in a more rigid manner: FAT can be performed by regressing the t value of the k-th estimate on the inverse of the standard error (1/SE) using the next EquationEquation (3)(3)
(3) , thereby testing the null hypothesis that the intercept term
is equal to zero:
where k is the error term.Footnote4 When the intercept term
is statistically significantly different from zero, we can interpret that the distribution of the effect sizes is asymmetric.
Even if there is publication selection bias, a genuine effect may exist in the available empirical evidence. Stanley and Doucouliagos (Citation2012) proposed examining this possibility by testing the null hypothesis that the coefficient is equal to zero in EquationEq. (3)
(3)
(3) . The rejection of the null hypothesis implies the presence of genuine empirical evidence.
is the coefficient of precision; therefore, it is called a PET.
Moreover, Stanley and Doucouliagos (Citation2012) also stated that an estimate of the publication selection bias-adjusted effect size can be obtained by estimating the following EquationEquation (4)(4)
(4) , which has no intercept. If the null hypothesis of
is rejected, then a non-zero true effect does actually exist in the literature, and the coefficient
can be regarded as its estimate.
This is the PEESE approach.Footnote5
To test the robustness of the regression coefficients obtained from the above FAT–PET–PEESE procedure, we estimate EquationEqs. (3)(3)
(3) and (Equation4
(4)
(4) ) using not only the unrestricted WLS estimator, but also the WLS estimator with bootstrapped standard errors, the cluster-robust WLS estimator, and the unbalanced panel estimator. In addition to these four models, we also run an instrumental variable (IV) estimation with the inverse of the square root of the number of observations used as an instrument of the standard error because ‘the standard error can be endogenous if some method choices affect both the estimate and the standard error. Moreover, the standard error is estimated, which causes attenuation bias in meta-analysis’ (Cazachevici, Havranek, and Horvath Citation2020, 5).
Furthermore, as pointed out by Bajzik et al. (Citation2020) and Zigraiova et al. (Citation2021), the FAT–PET–PEESE approach implicitly assumes that publication selection bias is linearly proportional to the size of the standard error, which might not be practical in some cases. To deal with the possible nonlinear relationship between the two, some advanced techniques have been developed recently. They include the Top 10 approach, proposed by Stanley et al. (Citation2010), who discovered that discarding 90% of the published findings greatly reduces publication selection bias and is often more efficient than conventional summary statistics; the selection model, developed by Andrews and Kasy (Citation2019), which tests for publication selection bias using the conditional probability of publication as a function of a study’s results; the endogenous kinked model, innovated by Bom and Rachinger (Citation2019), which presents a piecewise linear meta-regression of estimates of their standard errors, with a kink at the cut-off value of the standard error below which publication selection is unlikely; and the p-uniform method, introduced by van Aert and van Assen (Citation2021), which is grounded on the statistical theory that the distribution of p-values is uniform conditional on the population effect size. In this paper, we apply these four techniques to provide alternative estimates of the publication selection bias-corrected effect size and compare them with the WAAP and PEESE estimates for a robustness check.
As the final step of meta-analysis, we conduct a meta-regression analysis (MRA) to explore the factors causing heterogeneity among selected studies to test the robustness of the results obtained from the meta-synthesis and the test for publication selection bias. More concretely, we estimate a meta-regression model:
where yk is the PCC (i.e. rk) of the k-th estimate, β0 is the constant, xkn denotes a meta-independent variable that captures the relevant characteristics of an empirical study and explains its systematic variation from other empirical results in the literature, sek is the standard error of the PCC, βn denotes the meta-regression coefficient to be estimated, and ek is the meta-regression disturbance term.
As pointed out in Iwasaki et al. (Citation2020) and Ono and Iwasaki (Citation2022), there is no clear consensus among meta-analysts about the best model for estimating EquationEq. (5)(5)
(5) . Hence, to check the statistical robustness of coefficient βn, we perform an MRA using the following six estimators: (1) the cluster-robust weighted least squares (WLS), which clusters the collected estimates by study, computes robust standard errors, and is weighed by the inverse of the standard error as a measure of estimate precision; (2) the cluster-robust WLS weighed by the degrees of freedom to account for sample-size differences among the studies; (3) the cluster-robust WLS weighed by the inverse of the number of estimates in each study to avoid the domination of the results by studies with large numbers of estimates; (4) the multi-level mixed-effects RLM estimator; (5) the cluster-robust random-effects panel GLS estimator; and (6) the cluster-robust fixed-effects panel LSDV estimator. We report either a random-effects model or a fixed-effects model, according to the Hausman test of model specification.
As Havranek and Sokolova (Citation2020) and Zigraiova et al. (Citation2021) argued, MRA involves the issue of model uncertainty, in the sense that the true model cannot be identified in advance. In addition, there is a high risk that the simultaneous estimation of multiple meta-independent variables could lead to multicollinearity. Accordingly, we estimate the posterior inclusion probability (PIP) and t value of each meta-independent variable other than the variables needed for hypothesis testing and the standard error of PCCs using the Bayesian model averaging (BMA) estimator and the weighted-average least squares (WALS) estimator, respectively, adopting a policy of employing variables for which the estimates have a PIP of 0.50 or more in the BMA analysis and a t value of 1.00 or more in the WALS estimation as selected moderators in EquationEq. (5)(5)
(5) .
III. Meta-synthesis
A meta-analysis of economic literature is ordinarily composed of three steps: (1) meta-synthesis of the collected estimates, (2) testing for publication selection bias and the presence of genuine empirical evidence, and (3) MRA of literature heterogeneity.Footnote6 We follow this standard procedure in testing the hypothesis. Hence, as the first step of meta-analysis, this section synthesizes the PCCs of the 6,135 estimates introduced in the preceding section.
First, let us look at the distribution of the collected estimates. In addition to the number of selected works, provides descriptive statistics of the estimates and the results of the t mean comparison test and Shapiro–Wilk normality test for all studies and by study type. shows the corresponding kernel density estimations. According to , both the mean and median for the category ‘all studies’ are positive, and according to the t-test, the null hypothesis that the mean is zero is rejected at the 1% statistical significance level.
Figure 1. Kernel density estimation of collected estimates.
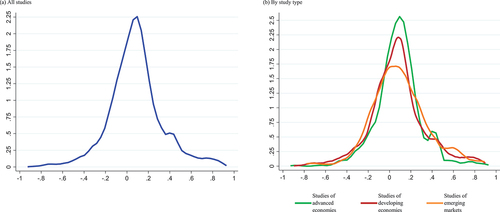
In addition, Panel (a) of displays a kernel density estimation biased in the positive direction. These results reveal that the empirical results reported in the 379 selected studies demonstrate that, as a whole, financial development and liberalization do contribute to economic growth in target economies.
Further, the mean and median of estimates extracted from the studies of advanced economies account for 0.090 and 0.085, respectively, slightly exceeding those from the studies of developing economies (0.077 and 0.068, respectively), while the mean and median of estimates reported in the studies of emerging markets are equal to 0.096 and 0.066, respectively. In other words, the relationship among the three study types differs significantly depending on whether one refers to the mean or the median. In fact, as reported in , the analysis of variance (ANOVA) does not reject the null hypothesis that there is no difference among the three study types (F = 2.17, p = 0.1148). At the same time, however, the Kruskal–Wallis rank-sum test strongly rejects it (χ2 = 9.383, p = 0.0092). Panel (b) of shows no significant differences in the distribution of the estimates for the three study types. This may be the reason it is difficult to reach a clear judgement based on descriptive statistics alone.
In light of the above considerations, we turn next to the results of meta-synthesis. Column (a) of reports the synthesis results using a meta fixed-effect model and a meta random-effects model, while Column (b) shows the heterogeneity test and measures. As shown in Column (b), the Cochran Q test of homogeneity rejects the null hypothesis at the 1% significance level, and the I2 and H2 statistics indicate the presence of heterogeneity in all study types. Accordingly, we have adopted the estimates of the random-effects model in Column (a) as reference values of the traditional method.
Table 2. Synthesis of collected estimates.
Column (c) of shows the results of the unrestricted weighted least squares averaging (UWA) and the weighted average of the adequately powered (WAAP) approach proposed by Stanley and Doucouliagos (Citation2017) and Stanley et al. (Citation2017) as a new synthesis method. Although, in theory, the UWA synthesis generated the same point estimate as that of the traditional fixed-effect model, the t value of the former notably falls below that of the latter. This suggests that the UWA method is less influenced by excess heterogeneity than the fixed-effect model.
In addition, three of four cases successfully synthesized collected estimates using the WAAP method. Hence, we adopt the WAAP estimates of 0.072, 0.072, and 0.053 as the best synthesis values for all studies, studies of developing economies, and studies of emerging markets, respectively, while the random-effects estimate of 0.085 is utilized as the reference synthesis value for studies of advanced economies.
The above meta-synthesis results imply that financial development and liberalization in advanced economies outperform those in developed economies from the viewpoint of the size of their effect on economic growth in terms of PCC. In this sense, emerging markets have fallen behind both advanced and developing economies; therefore, the null hypothesis of no difference among the three development stages is rejected. Are these synthesis results not affected by publication selection bias? Can we regard them as true effect sizes? The next section examines these issues.
IV. Test of publication selection bias
As the second step of meta-analysis, we test for publication selection bias and the presence of genuine empirical evidence using a funnel plot and the goodness-of-fit test of proportional distribution, as well as the MRA test procedure combining the funnel-asymmetry test (FAT), the precision-effect test (PET), and the precision-effect estimate with standard error (PEESE).
The funnel plot presented in Panel (a) of shows that the estimates extracted from all 379 studies form a distribution with an inverted funnel shape, suggesting that strong manipulation of the publication selection of empirical evidence is unlikely to exist in the selected literature. As shown in panels (b) through (d) of the figure, this is mostly true even when the estimates are split by study type. This finding is backed up by a goodness-of-fit test that examines whether collected estimates are distributed proportionally around the true effect under the assumption that the true effect size is close to the selected synthesis value reported in . In fact, according to , in the case of all studies, if we assume that the true effect size is zero, as illustrated by the dotted line in Panel (a) of , then the ratio of positive and negative estimates would be 4011:2124, and the null hypothesis that the ratio of positive to negative estimates is equal is rejected at the 1% significance level (z = 24.092, p = 0.000).
Figure 2. Funnel plot of partial correlation coefficients.
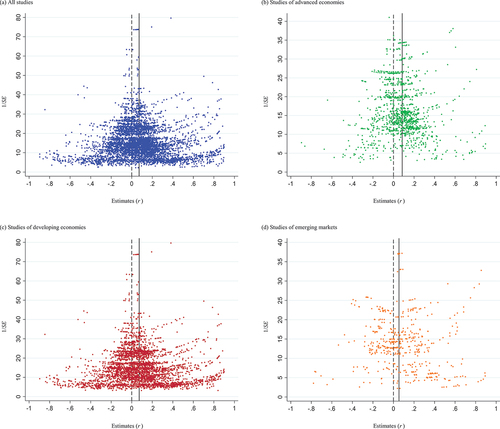
Table 3. Univariate test of publication selection bias.
Meanwhile, if we assume that the WAAP synthesis value depicted by the solid line approximates the true effect, then the distribution of the collected estimates would be 3032:3103 on the left and right sides of the threshold of 0.072, and, accordingly, the null hypothesis cannot be rejected (z = 0.907, p = 0.365). These results imply that the probability of publication selection bias is low in this research domain if the meta-synthesis result captures the true effect. The same conclusion can be applied to the three individual study types.
As discussed above, visual examinations using a funnel plot in and the univariate test in demonstrate consistent results. However, the shape of the funnel plot that seems to peak at or near zero may indicate the presence of a potential measurement error and a resulting attenuation bias in previous studies that could drive the reported results to zero. This observation implies the need to check for the presence of publication selection bias more rigidly and to deal with possible endogeneity between publication selection bias and the standard error. Therefore, we should rely on the methodologically stricter FAT–PET–PEESE procedure for final judgement.
shows the results of the FAT–PET–PEESE procedure for all studies. According to Panel (a) of the table, the null hypothesis that the intercept (γ0) is zero is rejected in three of five models, indicating that publication selection bias is likely to have taken place in the 379 selected research works. Hence, the FAT result negates the intuitive comprehension obtained from the funnel plot in and the univariate test result in .
Table 4. Meta-regression analysis of publication selection: all studies.
Even if publication selection bias is present, however, the collected estimates may contain genuine empirical evidence. Panel (a) of shows that the null hypothesis that the coefficient (γ1) of the inverse of the standard error (1/SE) is zero is rejected in all five models by PET, proving that the collected estimates do contain empirical evidence. Furthermore, as seen in the results of the PEESE approach, shown in Panel (b) of the table, the coefficients (γ1) of 1/SE are estimated to be statistically significant in five models; therefore, the true value should be in a range from 0.0564 to 0.0695 in terms of PCC. These results lead us to conclude that financial development and liberalization have a positive effect on economic growth in target economies as a whole in line with the meta-synthesis results in .
As pointed out in Section II, the FAT–PET–PEESE method implicitly assumes a linear relationship between the standard error and publication selection bias, which may not be realistic in the case of this study. Therefore, as a robustness check, we performed alternative estimations of the publication selection bias-corrected effect size using four models. shows the results. Although the estimates vary depending on the method used, all four models demonstrate the existence of a statistically significant and positive effect of finance on growth consistent with the PEESE estimates in .
Table 5. Alternative estimates of publication selection bias-corrected effect size: all studies.
Taking the above results for all studies as the benchmark, we also performed the FAT–PET–PEESE procedure and the alternative estimations of publication selection bias-corrected effect size separately by study type. summarizes the results together with those for all studies. As reported in this table, the FAT did not identify publication selection bias in all three study types. Furthermore, the PET proved the presence of genuine empirical evidence, and the PEESE approach and the alternative estimation methods produced non-zero publication selection-adjusted effect sizes for all three cases. These PEESE and alternative estimates indicate that the true effect size of finance differs among the three study types and, consequently, reject the hypothesis.
Table 6. Summary of publication selection bias test.
V. Meta-regression analysis
The findings obtained from the meta-synthesis of and the testing for publication selection bias in the collected estimates, however, fail to account for differences in study conditions across the selected research works. Therefore, we need to check the robustness of the findings in the previous sections by examining whether they are replicable when various aspects of heterogeneity in the literature are controlled for. Hence, as the final step of meta-analysis, this section examines the possible influence of literature heterogeneity on the empirical results of selected works by estimating a multivariate meta-regression model.
To this end, we take the PCCs of the collected estimates as the dependent variable, while we initially employ a total of 30 variables as meta-independent variables. These 30 variables capture the differences in types of financial variables, number of countries studied, data type, estimation period, estimator, presence of control for endogeneity, attributes of financial variables, and selection of control variables in addition to the variables of study type that aim to test the hypothesis and standard errors of PCCs.Footnote7
To tackle the issues of model uncertainty and multicollinearity that may arise from the use of a multitude of moderators, following the example of Havranek and Sokolova (Citation2020) and Zigraiova et al. (Citation2021), we first conducted a Bayesian model averaging (BMA) analysis and a weighted-average least squares (WALS) estimation, taking the studies of developing economies, studies of emerging markets, and standard errors of PCCs as focus regressors and the other meta-independent variables as auxiliary regressors. As a result of this selection process, 11 variables having both a posterior inclusion probability (PIP) of 0.50 or more in the BMA analysis and a t value of 1.00 or more in the WALS estimation are identified as robust moderators against model uncertainty and multicollinearity.Footnote8
We then regressed the PCCs of collected estimates on three focus regressors and the 11 selected moderators from bank credit to GDP to financial crisis using five different models. The estimation results are reported in . As shown in this table, estimates are sensitive to the choice of estimator. Therefore, we assume that meta-independent variables that are statistically significant and have the same sign in at least three of five models constitute robust estimates.
Table 7. Meta-regression analysis with selected moderators.
From the above standpoint, we confirm that, while three selected moderators consisting of bank credit to GDP, number of target countries, and cross-sectional data were given robust estimates, the variables of studies of developing economies and studies of emerging markets were estimated to be insignificant in all five models.Footnote9 These results indicate that there is no statistical difference among the three study types in terms of the effect size of financial variables measured by PCC, ceteris paribus. Thereby, we judge that the MRA results in cannot reject the hypothesis and, thus, do not support the meta-synthesis results and the test results for publication selection bias.
VI. Conclusions
In this study, we attempted to answer the question of whether the growth-promoting effects of finance differ at different stages of development through a comparative meta-analysis of advanced, developing, and post-communist emerging market economies. This has not been comprehensively investigated in previous meta-analysis studies. As a result, the meta-synthesis and the testing for publication selection bias and genuine empirical evidence performed in Sections III and IV, subject to 6,135 estimates, reported that 379 precedent-setting research works rejected the null hypothesis that the effect size of financial development and liberalization on economic growth does not vary among advanced economies, developing economies, and emerging markets.
According to the standards of Doucouliagos (Citation2011) regarding the evaluation of PCCs in macroeconomics research, however, the selected synthesis values of collected estimates in do not exceed the ‘small’ scale threshold, meaning that, regardless of the difference in the development stage, the growth-promoting effect of finance is quite limited.Footnote10 The same is true for the results of the publication selection bias test and the alternative estimations of the publication selection bias-corrected effect size reported in . Perhaps it is due to the negligible disparities in effect size among the three study types that the MRA of literature heterogeneity in could not detect a statistically significant difference among them when a series of study conditions were simultaneously controlled for.
The aforementioned results have significant implications for studies of the finance–growth nexus. First, the impacts of financial development and liberalization on economic growth do not vary across different stages of development. In other words, the results do not suggest that the financial growth-enhancing effects for low-income economies are larger, which are often said to have advantages in catching up to advanced economies, using technologies that already exist.Footnote11 Nor do they suggest that developed economies enjoy higher financial growth-prompting effects as compared with developing countries, which could be economically subordinate to economic powerhouses.
Moreover, the financial growth-enhancing effects for post-communist emerging markets do not significantly differ from those of the other economies. Although there could be relatively large numbers of highly profitable businesses in the initial stage of transition, the financial growth-promoting effects are not significantly larger than those of other economies.Footnote12 This could suggest that newly established banks and financial markets did not contribute sufficiently to economic growth.
The number of studies on the finance–growth nexus for emerging markets is relatively limited as compared with those of developing and advanced economies. Specifically, our literature search identified 46 studies that provide empirical evidence of the effects of financial development and liberalization on economic growth for emerging markets, whereas this paper used 299 and 81 studies of developing and advanced economies for the meta-analysis, respectively. This could be a limitation of our study; thus, it is hoped that global financial research will advance further, especially with regard to post-communist emerging economies.
Supplemental Material
Download PDF (277.3 KB)Supplemental data
Supplemental data for this article can be accessed online at https://doi.org/10.1080/00036846.2023.2289921.
Disclosure statement
No potential conflict of interest was reported by the author(s).
Additional information
Funding
Notes
1 Post-communist emerging markets are, specifically, Albania, Armenia, Azerbaijan, Belarus, Bosnia and Herzegovina, Bulgaria, China, Croatia, the Czech Republic, Estonia, Georgia, Hungary, Kazakhstan, Kyrgyzstan, Latvia, Lithuania, Moldova, Montenegro, North Macedonia, Poland, Romania, Russia, Serbia, Slovakia, Slovenia, Tajikistan, Turkmenistan, Ukraine, and Uzbekistan.
2 The following academic press websites were used in this literature search: Emerald Insight, Oxford University Press, Sage Journals, Science Direct, Springer Link, Taylor and Francis Online, and Wiley Online Library. The search of academic press websites was conducted for the most recent studies, published since January 2022, to supplement the results of the EconLit and Web of Science searches. The final search of literature was conducted in February 2023.
3 A bibliography of these 379 selected works is available as an Online Supplement. It is inevitable that our literature search would have missed some papers published in newly established journals, in particular Bibi and Sumaira (Citation2022) and Sumaira and Bibi (Citation2022). We thank a reviewer for his/her suggestion regarding this point.
4 Eq. (3) is an alternative to the following meta-regression model that takes the effect size as the dependent variable and the standard error as the independent variable.
, (3b).
More specifically, Eq. (3) is obtained by dividing both sides of the equation above by the standard error. The error term in Eq. (3b) does not often satisfy the assumption of being i.i.d. (independent and identically distributed). In contrast, the error term in Eq. (3),
, is normally distributed; thus, it can be estimated by OLS. Publication selection bias also can be detected by estimating Eq. (3b) using the WLS estimator with the inverse of the squared standard error
as the analytical weight, thereby testing the null hypothesis of γ0 = 0.
5 We can see that coefficient γ1 in Eq. (4) may become the estimate of the publication selection bias-adjusted effect size in light of the fact that the following equation is obtained when both sides of Eq. (4) are multiplied by the standard error.
(4b).
When directly estimating Eq. (4b), the WLS method, with as the analytical weight, is used.
6 This paper performs a meta-analysis in accordance with internationally established standard procedures (Iwasaki Citation2020; Stanley and Doucouliagos Citation2012) and the reporting guidelines published in Havránek et al. (Citation2020).
7 Appendix lists the names, definitions, and descriptive statistics of 30 meta-independent variables.
8 Results of the BMA analysis and WALS estimation are reported in Appendix .
9 Estimation results of a model with all moderators are reported in Appendix . As shown in the table, the study type variables show insignificant estimates, as in .
10 As the evaluation criteria of the correlation coefficient, Doucouliagos (Citation2011) proposed 0.104, 0.226, and 0.386 to be the lowest thresholds of small, medium, and large effects, respectively, as general standards in macroeconomic research (ibid., Table 3, p. 11). On the other hand, we must note that even small contributions to growth can accumulate to economically significant effects over longer periods.
11 The IMF (IMF Citation2000) argues that although human living conditions have improved greatly over the past 100 years, brought on by unprecedented technological and economic transformations, rising global prosperity has not benefited all countries and regions. Our results could reflect these situations.
12 EBRD (1997) claims that several post-communist emerging countries, including the Baltic states, suffered a banking crisis, which would support our results.
References
- Akinboade, O. A. 2000. “The Relationship Between Financial Deepening and Economic Growth in Tanzania.” Journal of International Development 12 (7): 939–950. https://doi.org/10.1002/1099-1328(200010)12:7<939:AID-JID668>3.0.CO;2-I.
- Allen, D. S., and L. Ndikumana. 2000. “Financial Intermediation and Economic Growth in Southern Africa.” Journal of African Economies 9 (2): 132–160. https://doi.org/10.1093/jae/9.2.132.
- Andrews, I., and M. Kasy. 2019. “Identification of and Correction for Publication Bias.” American Economic Review 109 (8): 2766–2794. https://doi.org/10.1257/aer.20180310.
- Arestis, P., G. Chortareas, and G. Magkonis. 2015. “The Financial Development and Growth Nexus: A Meta-Analysis.” Journal of Economic Surveys 29 (3): 549–565. https://doi.org/10.1111/joes.12086.
- Asante, G. N., P. O. Takyi, and G. Mensah (2023) The Impact of Financial Development on Economic Growth in Sub-Saharan Africa: Does Institutional Quality Matter? Development Studies Research, 10(1), 2156904 Article.
- Bajzik, J., T. Havranek, Z. Irsova, and J. Schwarz. 2020. “Estimating the Armington Elasticity: The Importance of Study Design and Publication Bias.” Journal of International Economics 127:103383. Article. https://doi.org/10.1016/j.jinteco.2020.103383.
- Bekaert, G., C. R. Harvey, and C. Lundblad. 2001. “Emerging Equity Markets and Economic Development.” Journal of Development Economics 66 (2): 465–504. https://doi.org/10.1016/S0304-3878(01)00171-7.
- Bibi, R., and Sumaira. 2022. “The Relationship Between Trade Openness, Financial Development and Economic Growth: Evidence from Generalized Method of Moments.” Journal of Environmental Science & Economics 1 (1): 58–66. https://doi.org/10.56556/jescae.v1i1.15.
- Bom, P. R. D., and H. Rachinger. 2019. “A Kinked Meta-Regression Model for Publication Bias Correction.” Research Synthesis Methods 10 (4): 497–514. https://doi.org/10.1002/jrsm.1352.
- Bumann, S., N. Hermes, and R. Lensink. 2013. “Financial Liberalization and Economic Growth: A Meta-Analysis.” Journal of International Money and Finance 33:255–281. https://doi.org/10.1016/j.jimonfin.2012.11.013.
- Cazachevici, A., T. Havranek, and R. Horvath. 2020. “Remittances and Economic Growth: A Meta-Analysis.” World Development 134:105021. Article. https://doi.org/10.1016/j.worlddev.2020.105021.
- Dahmani, M., M. Mabrouki, and A. B. Youssef. 2023. “The ICT, Financial Development, Energy Consumption and Economic Growth Nexus in MENA Countries: Dynamic Panel CS-ARDL Evidence.” Applied Economics 55 (10): 1114–1128. https://doi.org/10.1080/00036846.2022.2096861.
- Doucouliagos, H. 2011.“How Large is Large? Preliminary and Relative Guidelines for Interpreting Partial Correlations in Economics.” School Working Paper No. SWP 2011/5, School of Accounting, Economics and Finance, Faculty of Business and Law, Deakin University: Melbourne.
- EBRD (European Bank for Reconstruction and Development). 1997. “Transition Report 1997.” London. European Bank for Reconstruction and Development.
- Havranek, T., and A. Sokolova. 2020. “Do Consumers Really Follow a Rule of Thumb? Three Thousand Estimates from 144 Studies Say “Probably Not.” Review of Economic Dynamics 35:97–122. https://doi.org/10.1016/j.red.2019.05.004.
- Havránek, T., T. D. Stanley, H. Doucouliagos, P. Bom, J. Geyer-Klingeberg, I. Iwasaki, W. R. Reed, K. Rost, and R. C. M. van Aert. 2020. “Reporting Guidelines for Meta-Analysis in Economics.” Journal of Economic Surveys 34 (3): 469–475. https://doi.org/10.1111/joes.12363.
- IMF (International Monetary Fund). 2000. World Economic Outlook. Washington, DC: International Monetary Fund.
- Iwasaki, I. 2020. “Meta-Analysis of Emerging Markets and Economies: An Introductory Note for the Special Issue.” Emerging Markets Finance & Trade 56 (1): 1–9. https://doi.org/10.1080/1540496X.2019.1620117.
- Iwasaki, I., X. Ma, and S. Mizobata. 2020. “Corporate Ownership and Managerial Turnover in China and Eastern Europe: A Comparative Meta-Analysis.” Journal of Economics and Business 111:105928. Article. https://doi.org/10.1016/j.jeconbus.2020.105928.
- Kassi, D. F., Y. Li, and Z. Dong. 2023. “The Mitigating Effect of Governance Quality on the Finance-Renewable Energy-Growth Nexus: Some International Evidence.” International Journal of Finance and Economics 28 (1): 316–354. https://doi.org/10.1002/ijfe.2423.
- Li, X. L., and C.-K. Wye. 2023. “The Effect of Investment in Education on China’s Economic Growth: The Role of Financial Development.” The Chinese Economy 56 (1): 69–87. https://doi.org/10.1080/10971475.2022.2058182.
- Mtar, K., and W. Belazreg. 2023. “On the Nexus of Innovation, Trade Openness, Financial Development and Economic Growth in European Countries: New Perspective from a GMM Panel VAR Approach.” International Journal of Finance and Economics 28 (1): 766–791. https://doi.org/10.1002/ijfe.2449.
- Nsor-Ambala, R., and G. Amewu. 2023. “Linear and Non-Linear ARDL Estimation of Financial Innovation and Economic Growth in Ghana.” Journal of Business and Socioeconomic Development 3 (1): 36–49. https://doi.org/10.1108/JBSED-09-2021-0128.
- Öncel, A., S. Saidmurodov, and A. Kutlar. 2023. “Financial Development, Export and Economic Growth: Panel Data Evidence from Commonwealth of Independent States.” The Journal of International Trade & Economic Development 1–28. (Early View). https://doi.org/10.1080/09638199.2022.2164045).
- Ono, S., and I. Iwasaki. 2022. “The Finance-Growth Nexus in Europe: A Comparative Meta-Analysis of Emerging Markets and Advanced Economies.” Eastern European Economics 60 (1): 1–49. https://doi.org/10.1080/00128775.2021.2006067.
- Reisen, H., and M. Soto. 2001. “Which Types of Capital Inflows Foster Developing-Country Growth?” International Finance 4 (1): 1–14. https://doi.org/10.1111/1468-2362.00063.
- Schumpeter, J. A. 1911. The Theory of Economic Development. Oxford: Oxford University Press.
- Stanley, T. D., and H. Doucouliagos. 2012. Meta-Regression Analysis in Economics and Business. London and New York: Routledge.
- Stanley, T. D., and H. Doucouliagos. 2017. “Neither Fixed nor Random: Weighted Least Squares Meta-Regression.” Research Synthesis Method 8 (1): 19–42. https://doi.org/10.1002/jrsm.1211.
- Stanley, T. D., H. Doucouliagos, and J. P. A. Ioannidis. 2017. “Finding the Power to Reduce Publication Bias.” Statistics in Medicine 36 (10): 1580–1598. https://doi.org/10.1002/sim.7228.
- Stanley, T. D., S. B. Jarrell, and H. Doucouliagos. 2010. “Could It Be Better to Discard 90% of the Data? A Statistical Paradox.” The American Statistician 64 (1): 70–77. https://doi.org/10.1198/tast.2009.08205.
- Sumaira, and R. Bibi. 2022. “Banking Sector Development and Economic Growth in South Asian Countries: Dynamic Panel Data Analysis.” Journal of Environmental Science & Economics 1 (1): 52–57. https://doi.org/10.56556/jescae.v1i1.10.
- Tsangarides, C. G. 2002. “On Cross-Country Growth and Convergence: Evidence from African and OECD Countries.” Journal of African Economies 10 (4): 355–389. https://doi.org/10.1093/jae/10.4.355.
- Valickova, P., T. Havranek, and R. Harvath. 2015. “Financial Development and Economic Growth: A Meta-Analysis.” Journal of Economic Surveys 29 (3): 506–526. https://doi.org/10.1111/joes.12068.
- van Aert, R. C., and M. van Assen 2021. “Correcting for Publication Bias in a Meta-Analysis with the P-Uniform Method.” Working paper, Tilburg University and Utrecht University.
- Zigraiova, D., T. Havranek, Z. Irsova, and J. Novak. 2021. “How Puzzling is the Forward Premium Puzzle? A Meta-Analysis.” European Economic Review 134:103714. Article. https://doi.org/10.1016/j.euroecorev.2021.103714.
Appendix
Table A1. Names, definitions, and descriptive statistics of meta-independent variables.
Table A2. Meta-regression analysis of model uncertainty and multicollinearity for the selection of moderators.
Table A3. Meta-regression analysis with all moderators.