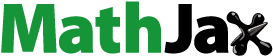
ABSTRACT
This study examines the explanatory power of the CAPM and downside risk asset pricing models (the downside beta and the realized semibeta models) for the next-month firm-level cross-sectional stock return variation in the Australian stock market. We show that the CAPM beta, downside beta, semibeta BetaNP, and semibeta BetaNN negatively predict future stock returns, which is inconsistent with the findings in the original study by Bollerslev, Patton, and Quaedvlieg (2022). The BetaNN measures the individual stock movement in the same direction as the downward stock market, while BetaNP measures individual stock downward with the upward stock market. These findings are robust, not subsumed by conventional cross-sectional asset pricing factors, and consistent with the existing Australian downside risk study.
I. Introduction
In the long history of asset pricing studies, academics and practitioners endeavour to measure the risk – return relationship appropriately. For example, Sharpe (Citation1964) and Lintner (Citation1965) independently propose the capital asset pricing model (CAPM), one of the most widely used asset pricing models. However, the CAPM’s pervasive usage does not mean that it is flawless. Early empirical studies have found that the CAPM fails to explain cross-sectional stock return variation (Black, Jensen, and Scholes Citation1972; Fama and MacBeth Citation1973). A plausible reason for this failure is one of its underlying assumptions that investors care only about mean – variance optimization, which can be partially remedied by using the mean – semivariance framework to capture the investor’s asymmetric treatment of upside and downside variance (Markowitz Citation1959).
In response, researchers have developed various downside risk measurement models to remedy the empirical weaknesses of CAPM. Along this path, we utilize the latest downside risk model in this study – Bollerslev, Patton, and Quaedvlieg’s (Citation2022) realized semibeta model to test whether it can predict cross-sectional stock returns in the Australian market.
Bawa (Citation1975) proposes the lower partial moment (LPM) framework, which can measure downside risk. The downside beta measurement, a theoretical extension based on LPM, has the advantage of better capturing the asymmetric price information in the market and can be applied to non-normal distributions (Bawa and Lindenberg Citation1977). Ang, Chen, and Xing (Citation2006) apply a downside beta model based on Gul’s (Citation1991) disappointment aversion theory, in which agents weigh more on loss than on gains. They find that the US stock investors receive a downside risk premium, and the downside beta can explain the cross-sectional US stock return variation in contemporaneous periods and predict future stock returns.
Early international downside beta empirical studies confirm Ang, Chen, and Xing’s (Citation2006) findings. Lettau, Maggiori, and Weber (Citation2014) extend the downside beta studies into multi-asset class markets beyond Ang, Chen, and Xing’s (Citation2006) seminal study, which focuses on the stock market only. Alles and Murray (Citation2013) prove that downside beta and coskewness capture different downside risk information in emerging Asian markets. Yet, subsequent empirical works do not find consistent outcomes of the downside beta model. Recent studies illustrate that the superior explanatory power of downside beta in the original US study may be sample- or time-specific (Atilgan et al. Citation2018; Atilgan, Demirtas, and Gunaydin Citation2020; Levi and Welch Citation2021).
Inspired by the latest realized semibeta model from Bollerslev, Patton, and Quaedvlieg (Citation2022), we propose that the mixed empirical evidence about whether the downside beta model has explanatory power to the cross-sectional stock return can be explained by the semibeta model. The semibeta model decomposes the CAPM beta into four semibetas based on the covariance between individual stock returns and market returns, instead of dividing CAPM beta into two betas only – upside and downside betas. The downside beta can be loosely dissected into BetaNN (measuring the individual stock movement in the same direction as the downward stock market) and BetaPN (measuring individual stock upward with the downward stock market), as BetaNN and BetaPN collectively measure all the stocks in a downward stock market. This finer dissection enables semibetas to convey more price information omitted by downside beta alone. The seminal US semibeta model study supports our proposition and reveals that BetaNN predicts future stock return positively, while BetaPN negatively. The inversely signed predictions between BetaNN and BetaPN presumably explain the reason for mixed empirical evidence with downside beta, as the switching sign of the predictive relationship about downside beta is determined by a stronger BetaNN or BetaPN for stocks.
Among downside beta studies, Alles and Murray’s (Citation2017) Australian study demonstrates a unique finding – both CAPM and downside betas report a negative coefficient estimate when explaining Australian cross-sectional stock return variation in the contemporaneous period, contrasting with the original US findings. They conclude that the unique result is attributed to the small and concentrated Australian stock market, with investors receiving excess returns (losses) in upturn (downturn) years.
Motivated by the conflicting empirical evidence regarding the downside beta model’s explanatory power worldwide and the unique findings from Alles and Murray’s (Citation2017) Australian study, we raise two research questions. Firstly, we test whether Alles and Murray’s findings suffer from sample-specific issues, as other researchers point out similar concerns for US studies. Secondly and more importantly, we test whether Bollerslev, Patton, and Quaedvlieg’s (Citation2022) semibeta model provide additional information and whether synergy exists between the two models in the Australian stock market.
Based on free-float market capitalization, the Australian stock market is the eighth largest globally and the second largest in Asia-Pacific (Bradrania and Veron Citation2023). The difference between the US and Australian stock markets is pervasive. The stock market composition is different, even though the US and Australian markets share a similar legal system, a high-level institutional investor engagement environment, and open financial markets (Chan et al. Citation2010; Zhong Citation2022). However, the Australian stock market has distinct features from the US market; the Australian market is smaller and contains fewer stocks (Chen et al. Citation2022; Gharghori, Lee, and Veeraraghavan Citation2009). Its listed stocks are concentrated in a few pillar industries, and the stock market is less diversified. High intensity of large capitalization stocks is observed in the energy, raw material, and finance sectors, in contrast to the high technology sector in the US (Alles and Murray Citation2017; Chan et al. Citation2010; Faff Citation2001). The tax system is also different between the US and Australia. The imputation tax system makes Australian firms prone to issue dividends more than US ones in exchange for tax benefits (Chan et al. Citation2010), leading to pervasively low stock prices in the Australian stock market.
The different stock market structure, tax system, economic nature, and unique location make the Australian stock market perform divergently to the US. Presumably, a more concentrated and less diversified stock market combined with large firms dominating environment suggests that the stock market has a higher tendency to be affected by large capitalization stocks, making the Australian stock market a valuable candidate to test the robustness of US semibeta evidence. Other studies also confirm that the Australian market shows unique results from other countries. For example, Frazzini and Pedersen (Citation2014), in their seminal ‘Betting Against Beta’ study, reveal that the profitable BAB strategy fails in the Australian stock market. Some existing cross-sectional asset pricing factors behave differently in Australia than within the US, such as factors in Fama-French three- and five-factor models (Durand et al. Citation2016; Elliot et al. Citation2018; Gaunt Citation2004).
Our study provides new empirical evidence regarding the explanatory power of the semibeta model to predict stock returns in the Australian stock market. In this stock market, we propose measuring the monthly semibeta based on the daily returns in both the past 12 months and the current month, generating similar results. From a domestic perspective, our study confirms and extends the unique findings that CAPM beta and downside beta negatively predict future stock returns into the semibeta model (Alles and Murray Citation2017). From an international perspective, our study serves as an out-of-sample test to the original US study by Bollerslev, Patton, and Quaedvlieg (Citation2022). We also provide further insight into how the semibeta model performs in the stock market with different features.
The results of our study illustrate that downside beta, semibeta BetaNP, and semibeta BetaNN consistently and negatively predict the next-month firm-level stock returns. These results are an extension of Alles and Murray’s (Citation2017) Australian study. Our results, which differ from the existing semibeta model studies by Bollerslev, Patton, and Quaedvlieg (Citation2022) and the Korean study by Chu (Citation2022), support the finding of Alles and Murray (Citation2017) that the Australian stock market has unique characteristics that differ from other markets.
The behavioural finance studies provide alternative assumptions regarding irrational investors, and the psychological bias may affect their investment decision-making process. Existing studies offer intuitive mechanisms to show how they affect the prediction of stock returns, and the mechanisms include investor sentiment, overconfidence, and demand for lottery-like stocks (Bali et al. Citation2017; Han, Li, and Li Citation2020; Long et al. Citation2022; Shi, Ausloos, and Zhu Citation2022; Van Eyden et al. Citation2023). Inspired by behavioural finance studies, we test whether these mechanisms affect the prediction by the semibeta model.
The remainder of this study is structured as follows. In Section 2, we detail how we extract the sample data; how we calculate various kinds of betas; and which are the control variables from cross-sectional asset pricing studies. In Section 3, we report the benchmark results from the Fama – MacBeth regression, and extensive robustness checks and subsample tests. Section 4 concludes.
II. Data and methodology
Data
This study focuses on the Australian stock market,Footnote1 particularly the Australian Securities Exchange (ASX), which is the largest stock exchange in Australia. As in mainstream studies that focus on ordinary stocks only (Alles and Murray Citation2013, Citation2017; Ang, Chen, and Xing Citation2006; Bollerslev, Patton, and Quaedvlieg Citation2022), we focus on all the currently listed ordinary stocks in the ASX. Our sample covers from 1 January 1985 to 31 January 2022. After detailed data screening,Footnote2 1,452 individual stocks with more than 4.4 million daily observations remain. Daily frequency data such as the total return index of individual stocks, the close price, the trading volume, and the market capitalization are derived from Refinitiv DataStream. We use the MSCI Australia index as a proxy for the market portfolio sourced from DataStream and measured in the Australian dollar. The risk-free rate is proxied by the 3-month overnight index swap (OIS) rate from the SIRCA SPPR and DataStream databases.
Methodology
Three kinds of betas are considered in this study: the CAPM beta, the downside and upside betas, and the four semibetas. Following Bollerslev, Patton, and Quaedvlieg (Citation2022), all the betas are calculated based on the realized variance framework, providing a more accurate volatility measurement and delivering more precise beta estimations. The combination of three kinds of beta offers a comprehensive view of the Australian stock market’s cross-sectional risk – return relationship from different angles. The two kinds of conventional beta models enable us to re-examine the unique findings in the existing Australian studies, while the semibeta model allows us to explore the root of unique findings more intuitively.
We follow Ang, Chen, and Xing (Citation2006) and Alles and Murray (Citation2017) to choose the daily log excess return in the past 12 months for the calculation of CAPM beta and downside and upside betas. For firm-level stocks at month , the current month beta is measured based on the past 12 months’ daily log excess returns from month
to month
. For the semibeta model, we provide semibetas measured in the past 12 months and based on the current month. In addition, we measure daily log stock excess returns based on the daily total return index to capture the dividend reinvestment concern for long-term holding.
The CAPM beta is measured in the realized volatility framework as follows:
where denotes the daily log excess return (measured as the difference between the individual stock return and the risk-free rate) of stock
on the
trading day of month
;
denotes the number of trading days of stock
in month
; and
denotes the daily log market excess return on the
trading day of month
.
Our downside beta model follows Bawa and Lindenberg (Citation1977) and is widely used in subsequent studies (Alles and Murray Citation2017; Ang, Chen, and Xing Citation2006). Our study chooses the average market excess return for the past 12 months as the threshold for the month , following Ang, Chen, and Xing (Citation2006). Other studies employ various thresholds, such as zero return or risk-free rate (Alles and Murray Citation2017). The choice of thresholds, however, will not materially change the results (Ang, Chen, and Xing Citation2006). The downside and upside betas are measured as follows:
where denotes the threshold value
on the
trading day of month
.
Bollerslev, Li, Patton, and Quaedvlieg (Citation2020) develop a semicovariance framework based on the in-fill asymptotic theory. Furthermore, Bollerslev, Patton, and Quaedvlieg (Citation2022) reveal that in a fixed sample return period of , the realized semibetas are equal to the true latent semibetas when the sample is infinitely segmented. We use a double-letter system to label the four semibetas: the first letter represents the status of the individual stock; and the second represents the market. For instance,
(
) denotes that individual stock has a positive correlation when the market is in upturn (downturn) while
denotes that individual stock moves downward with the market in upturn; and
denotes that the individual stock moves upward with the market in a downturn. Given the definition of realized semibetas, it is intuitive to find that
is approximately equal to
if we ignore the difference in the denominators of models. The downside beta covers all stocks in a downward moving market, including individual stocks, both moving upward and downward. As a result, the term ‘realized semibeta’ conceptually refers to the further division of the CAPM beta into four semibetas, based on the market movement with individual stocks in the same direction and the reverse direction. The four different semibetas are measured as follows:
assuming = max (
) and
= min (
),
and
. Both
and
should maintain a positive number while
and
should be negative, as the latter group measures individual stocks that negatively correlate with market movement and are always negative. We can divide CAPM beta into four semibetas: these four semibetas conceptually are an extended decomposition of the downside and upside betas. The relationship across all three kinds of betas can be expressed by the following equation:
We also introduce several filters to reduce the sample’s noise. For example, we require that each firm-level stock have at least 125 valid trading days in the past 12 months, which aligns with existing downside risk studies (Alles and Murray Citation2017; Levi and Welch Citation2021). As well as these minimum return requirements, we introduce another filter: each beta needs at least 22 observations in the past 12 months.
Variables
The dependent variable used in the study is (log returns of each stock), and the main independent variables are Beta (CAPM beta), BetaN (downside beta), BetaP (upside beta), and BetaPP, BetaPN, BetaNP, BetaNN (semibetas). However, the long history of cross-sectional studies from the early 1980s has identified that the CAPM cannot fully explain the cross-sectional risk – return relationship (academically referred to as ‘market anomalies’). These anomalies have been extensively investigated in the Australian stock market and reveal mixed evidence (Durand et al. Citation2016; Gharghori, Lee, and Veeraraghavan Citation2009). Therefore, we need to add those firms’ unique characteristics as control variables in our regression analysis to check if they subsume the explanatory power of semibetas in the Australian market.
The control variables used in this study are SIZE (size factor), BM (book-to-market factor), MOM (momentum factor), REV (short-term reversal factor), IVOL (idiosyncratic volatility factor), RV (realized variance factor), ILLIQ (illiquidity factor), CSK (coskewness), and CKT (cokurtosis). shows the details of the control variables.
Table 1. Control variables.
III. Empirical results
We follow the conventional cross-sectional asset pricing studies by presenting results from the Fama and MacBeth (Citation1973) OLS (Ordinary Least Squares) regression in this section.
Descriptive statistics
Panels A and B of present the monthly time-series averages of the cross-sectional summary statistics and the correlations of all variables for the sample period from 1 January 1985 to 31 January 2022. Individual monthly stock returns are continuously compounded returns, and we only include months with non-missing independent variables.
Table 2. Summary statistics and correlations.
Based on Panel A of , follows a near bell-shaped return distribution, slightly right skewed and with a high peak. The CAPM beta has a right tail and a flatter peak, with a minimum value of −0.802, a maximum value of 2.016 and a kurtosis of 0.589. BetaN (skewness of 0.193) and BetaP (skewness of 0.032) are slightly right skewed; both maintain a flat shape and report low kurtosis. All statistic figures among the CAPM beta, BetaN and BetaP are close to each other, indicating that these three betas convey similar information. In contrast, the four semibetas present two distinct sets of patterns. For example, the trends between BetaPP and BetaNN are very close, as well as between BetaPN and BetaNP. Their means, standard deviations, minimum, and maximum values are similar, as are their skewness and kurtosis. A comparison between the four semibetas and the CAPM beta shows that semibetas have a lower standard deviation and a narrower spread, and the semibetas also show a lower spread than BetaN and BetaP.
Panel B of reports the average time-series correlation between the dependent variable and all independent variables. The correlations between
and all independent variables are low, with BetaN having the highest correlation value at −0.115. BetaN (0.883) and BetaP (0.855) are highly correlated with the CAPM beta and move in the same direction. These high correlations reinforce the finding in Panel A that BetaN and BetaP deliver similar information to that of the CAPM beta. In comparison, all four semibetas have a low to moderate correlation with the CAPM beta; the highest correlation (0.591) is obtained between BetaNN and the CAPM beta. A similar correlation exists between the semibetas and BetaN and BetaP, except that BetaNN has the highest correlation of 0.708 with BetaN. The correlations within semibetas are moderate to high, with the highest two being 0.796 (BetaPP and BetaNN) and 0.811 (BetaNP and BetaPN). These results confirm that BetaPP and BetaNN, as well as BetaPN and BetaNP, report similar statistical figures in Panel A. The moderate correlations between the semibetas and the CAPM beta suggest that each semibeta likely holds different information than the CAPM beta.Footnote3
Both panels of reveal that upside and downside betas (BetaN and BetaP) are closely linked to the CAPM beta since they have high correlations and similar statistical figures. In comparison, the semibetas exhibit a lower correlation to both CAPM beta and upside and downside betas, which suggests that the dissection of CAPM beta into four semibetas may convey additional price information.
Fama–MacBeth regressions
We then perform the univariate and multivariate Fama – MacBeth OLS regressions in testing how each kind of beta predicts the next-month firm-level stock returns. In addressing the heteroscedasticity and autocorrelation issue about the overlapping monthly beta calculation, we report the Newey – West t-statistic with 12 lags.
The Fama – MacBeth regression includes a two-stage regression design for panel data analysis. For each month, we run a cross-sectional regression:
Based on the regression results, we take the time-series average of s over all the months in the sample.Footnote4 Our dependent variable is the logreturns in month
, while all the independent variables are measured in month
. In addition, we winsorize all independent variables at 1% and 99% each month to exclude extreme values in the independent variables, following Atilgan, Demirtas, and Gunaydin (Citation2020), and Ang, Chen, and Xing (Citation2006). Winsorization rules out outliers that would vastly increase the volatility and further affect our regression analysis.
Benchmark result
presents the benchmark multivariate Fama – MacBeth regression results. To test whether the new measures provide any information beyond these existing measures of downside risk and to test whether adding these two variables will subsume the explanatory power of betas, we also add two higher-order downside risk measurement variables, coskewness (CSK) and cokurtosis (CKT) (Ang et al. Citation2006; Christoffersen et al. Citation2021; Dittmar Citation2002; Harvey and Siddique Citation2000; Kraus and Litzenberger Citation1976). Existing studies propose that higher moment measurements can better capture asymmetric information than betas (Alles and Murray Citation2013, Citation2017; Doan et al. Citation2014). The third and fourth moments could capture information that cannot be captured by the first and second moments when measuring betas.
Table 3. Benchmark Fama – MacBeth multivariate OLS regression.
We purposely add a minus sign to BetaPN and BetaNP and convert them into positive numbers to better compare the risk – return relationship in the regression results hereafter following Bollerslev, Patton, and Quaedvlieg (Citation2022). presents the multivariate Fama – MacBeth regression results of each kind of betas by controlling the CSK and CKT. Column (2) indicates that when testing CSK and CKT together, none reports a significant coefficient. When testing the CAPM beta (column 3) against CSK and CKT, the result shows that CSK and CKT are both insignificant. Once we focus on the downside and upside beta (column 5) or four semibetas jointly (column 7), CSK and CKT report significant negative coefficients. Although the results illustrate that CSK and CKT may convey price information that downside risk measurements fail to capture, they do not show that higher moment measurements subsume the explanatory power of various betas. A latest Australian study also supports that higher moment measurements convey additional asymmetric information than downside risk ones (Ahadzie and Jeyasreedharan Citation2023).
also tests whether conventional cross-sectional asset pricing factors subsume the explanatory power of all kinds of betas. We find that the coefficients of the CAPM beta, downside beta, upside beta, and four semibetas remain almost the same after adding a full list of control variables. Considering the correlations between semibetas (around 0.8) that might affect our multivariate regression results, we report Fama – MacBeth regression for a single semibeta with control variables in Table A1 in the online appendix. BetaPP and BetaPN report insignificant coefficients in Table A1, while the coefficients for BetaNP and BetaNN do not change much. The difference in results illustrates that the difference is derived from the correlations within four semibetas rather than the correlations between semibetas and control variables. As a result, only BetaNP and BetaNN have stable predictive power, as both report consistent regression results and are unaffected by the high correlations within four semibetas.
Among all the control variables, BM, MOM, RV, and ILLIQ coefficient estimates show consistent signs among columns (8) to (11) in . MOM and ILLIQ show opposite signs compared to the cross-sectional asset pricing literature in column (11). The average R2 increases when shifting from downside and upside betas to four semibetas, indicating that the semibeta model has a higher explanatory power.
One interesting findings relate to the signs for coefficient estimates of various kinds of betas. Recall that we observe a negative relationship between future stock returns with CAPM beta, downside beta, BetaNP, and BetaNN in univariate regression; a consistent negative coefficient exists across multivariate regression. Using current-month beta to predict next-month firm-level stock returns extend the unique Alles and Murray (Citation2017) finding, consistent with Ahadzie and Jeyasreedharan’s (Citation2023) results. For semibetas, BetaNP and BetaNN report negative coefficients and consistent results in univariate and multivariate regressions. These results contrast with existing semibeta studies (Bollerslev, Patton, and Quaedvlieg Citation2022; Chu Citation2022).
Hypothesis testing
The Fama – MacBeth regression results prove the statistical significance of all three measures of betas. We propose two hypotheses to test the economic meaning of whether different models convey different price information. Suppose downside beta and semibeta models deliver different information. In that case, investors can achieve profitable stock trading strategies based on targeting specific beta(s). Given that all downside beta and semibeta BetaNP and BetaNN coefficients are negative in our benchmark results, investors could construct quintile beta sorting stock portfolios based on any of them. A self-financed trading strategy could be taking a short position with the highest quintile beta portfolio (lowest return) and taking a long position with the lowest quintile beta portfolio (highest return). An investor could receive the difference in return as their profit.
The first hypothesis tests whether the semibeta model conveys additional information compared to the traditional CAPM. If both models convey the same information, the semibeta model risk premiums should hold as follows:
The second hypothesis compares the information conveyed between the downside beta and the semibeta model. As the semibeta model is a conceptual extension of the conventional downside beta model, the model is expected to deliver more information for asset pricing purposes.
reports the results for hypotheses 1 & 2 under the benchmark results scenario. We deliberately add a negative sign to and
to convert it back to its original negative value. We perform the Chi-square test for both hypotheses and report their Chi-square statistic value,
-value, and degree of freedom in . Based on the result, we can reject the
and
both at least at the 5% level. In other words, the semibeta model conveys additional information compared to the CAPM and downside beta models.
Table 4. Hypothesis testing.
Robustness checks
In previous sections, we analyse the explanatory power of three kinds of betas using various current months’ betas to predict the following month’s firm-level stock returns in the Australian stock market. Our counter-theoretical benchmark results are consistent with the existing Australian study but differ from those for other markets. To ease the sample- or time-specific issue that might lead to inconsistent results, we perform extensive sensitive checks to see whether our benchmark results hold.
We first explore whether the change in filters used to calculate betas will materially affect our benchmark results. Specifically, the following changes in data filtering are considered: removing the winsorization; requiring fewer beta observations; requiring more valid daily stock observations in the past 12 months; and relying on an additional filter based on trading volume.Footnote5 All report statistically equivalent results.Footnote6
Galagedera, Maharaj, and Brooks (Citation2008) reveal that different beta formation periods affect the beta’s explanatory power of the Australian industry portfolios based on the wavelet timescales technique. We address their findings at individual stock levels and calculate betas based on different formation periods. Monthly betas that are calculated based on the current month’s daily returns, following Bollerslev, Patton, and Quaedvlieg (Citation2022) and Chu (Citation2022), generate similar results with benchmark results, except that CAPM beta is insignificant from 0.Footnote7 For betas calculated based on longer horizons, such as in the past 36 or 60 months, both downside and upside betas and four semibetas report a result similar to the benchmark results.Footnote8 Neither the extension nor shortening of the beta formation period affects our cross-sectional stock return results in the Australian stock market.
Cakici, Zaremba, Bianchi, and Pham (Citation2021) propose that small-cap stocks may drive many anomalies within the Australian stock market. Alles and Murray (Citation2017) perform their study based on ASX 200 constituents, which naturally remove the small-cap stocks. We examine whether removing small-cap stocks changes our benchmark results.
illustrates the Fama – MacBeth regression result for ordinary stocks in the Australian stock market by removing small-cap stocks and further restricting them to the Top 200 each month.Footnote9 The ASX 200 was formed in April 2000, later than our sample starting month (January 1985). As a result, we choose to construct our own ASX 200 by ranking the stocks by market capitalization each month. Admittedly, this does not produce an identical ASX 200 index in the real world. However, maintaining a consistent ASX 200 list is more important in our study for understanding the Australian market.
Table 5. Fama – MacBeth OLS regression with market capitalization restrictions.
Columns (1) to (6) in focus on the joint testing of the downside and upside betas, while columns (7) to (12) report the regression results for the semibeta models. All betas report consistent significant coefficients that are similar to benchmark results. We observe minor changes in the significant level for coefficient estimation in SIZE, REV, IVOL, and ILLIQ. The overall results suggest that the small-cap stocks have explanatory power of certain market anomalies but do not have a material effect on the explanatory power of various kinds of betas.
reports the results of subsample robust checking based on different periods. Columns (1) to (8) report subsample checking results based on the economic cycle, while columns (9) to (12) explore the difference between pre- and post-COVID periods. We follow Wen, Li, Chen, and Singh (Citation2023) in defining each economic cycle as lasting for a decade. In doing so, we separate each financial crisis into one economic cycle, and this gives us four cycles from January 1985 to the present. The quarterly adjusted GDP percentage change figures from the Australian Bureau of Statistics support this assumption. We observe an upward trend in quarterly GDP, except for major declines in 1991, 2000, 2008 and 2020. Quarters of declining GDP are associated with well-known domestic and international financial crises. For COVID subsample checking, we set January 2020 as the starting point.
Table 6. Fama – MacBeth OLS regression with subsample tests.
Columns (1) to (4) of report that downside and upside betas have consistent results across economic cycles. In addition, columns (5) to (8) report the regression results for four semibetas; all semibetas show consistent results similar to benchmark results. Control variables generally show time-varying natures, with decreasing average R2 as time moves to the present. The unstable coefficients of control variables and R2 presumably relate to fewer observations available in the early periods.
Columns (9) and (10) of show that downside and upside betas maintain explanatory power during pre- and post-COVID periods. However, semibeta models perform differently post-COVID, as BetaPP report a less significant coefficient, BetaPN reports insignificant coefficients, and the rest are intact. In comparison, BetaNP and BetaNN still report similar results as in the benchmark one. Intuitively, the limited length of monthly observations (25 months) does not hinder the explanatory power of BetaNP and BetaNN.
We also find that both downside and upside beta models and four semibetas have consistent explanatory power within and outside the global financial crisis (GFC) periods, and report similar results compared with benchmark results.Footnote10 In the upturn and downturn years’ regression analysis constructed following Alles and Murray (Citation2017), we observe results identical to the benchmark results in both upturn and downturn periods, even though we choose different threshold values in distinguishing the upturn and downturn periods.Footnote11 Instead of predicting the stock return in one month only, we also examine the long-term predictability of betas to reveal more insights. We find that the BetaNP and BetaNN have stable predictive power to stock returns in the future 3, 6, 9, and 12 months, as well as BetaN and BetaP.Footnote12
Results comparison
We observe similar coefficient estimations for all the betas between benchmark results and the subsequent robustness checks, confirming our results are sound. The negative coefficients of CAPM and downside betas align with existing Australian downside beta studies (Ahadzie and Jeyasreedharan Citation2023; Alles and Murray Citation2017). Though the negative relationship observed in the Australian stock market seems counter-intuitive, it is not uncommon as similar findings are reported in several other regions (Atilgan et al. Citation2018). According to the regression results for the semibeta model, we conclude that the negative coefficient estimations for downside beta are primarily driven by the negative coefficient observed with semibeta BetaNN. Different from existing semibeta studies, we are the first to report negative relationships between BetaNP (BetaNN) and stock returns in a cross-sectional setting. Both the US (Bollerslev, Patton, and Quaedvlieg Citation2022) and Korean (Chu Citation2022) studies show that BetaNN has a positive relationship with stock returns, while BetaNP does not.
The contrasting findings in the Australian stock market raise concerns about applying asset pricing models’ predictive power in regions outside the US, especially in markets with different structures and risk exposures. By addressing these differences and exploring some possible explanations, we show that for both practitioners and researchers achieving a better and deeper understanding of the local market is crucial.
Plausible explanations from the behavioural finance perspective
Our empirical results show that CAPM beta, downside beta, BetaNP, and BetaNN all significantly and negatively predict future firm-level stock returns within the Australian stock market. These intriguing negative relationship findings contradict what the classical finance theory suggests, as the results imply that investors who hold riskier assets (higher betas) fail to be rewarded with higher returns.
The existing behavioural finance studies provide several possible explanations for the negative risk – return relationship observed worldwide, which generally states their psychological bias influences investors’ decision-making process. Inspired by this strand of literature, we test three mechanisms and test whether they can explain the negative risk – return relationship in our study; the three effects are left tail risk, lottery stocks demand, and investor sentiments.
Atilgan, Bali, Demirtas, and Gunaydin (Citation2020) reveal the left tail anomaly that stocks with high extreme downside risk have a negative relationship with the future stock returns cross-sectionally in the US and international stock markets. They conclude that this anomaly is related to investors underestimating the persistence of downside tail risk of stocks, hence accepting overpriced high downside risk stock and leading to a lower return. Recall that a similar negative risk – return relationship is observed in Alles and Murray’s (Citation2017) and our studies, and it is reasonable to test whether the negative risk – return relationship is driven by left tail risk (extreme downside risk) or downside beta/semibetas (systematic downside risk).
We follow Atilgan, Bali, Demirtas, and Gunaydin (Citation2020) and use value at risk (VaR) to measure the left tail risk cross-sectionally in Australia. The VaR is calculated as the first percentile of daily stock return in the past 12 months. We then construct univariate quintile portfolios based on individual stocks’ VaR and examine the portfolios’ excess return. Table A8 reports the results, and we fail to observe a decrease in portfolio return as VaR increases. Hence, our study’s negative risk – return relationship is not driven by extreme downside risk and cannot be explained by the left tail anomaly.
Australian studies reveal two possible behavioural finance explanations for the negative risk – return relationship: lottery stocks demand (Bradrania and Veron Citation2023; Zhong and Gray Citation2016) and investor sentiment (Akhtar et al. Citation2011; Tiwari et al. Citation2022). The former explanation indicates that the unusual demand for lottery stocks (with a high possibility of a sizable future stock price increase) drives up the stock price and lowers the return. The latter explanation proposes that investor sentiments negatively affect stock prices when the consumer sentiment index is lower than in the previous period.
Inspired by Bradrania and Veron (Citation2023), we use the MAX factor to measure the investor demand for lottery-like stocks and test whether it also explains our findings. However, we fail to find that the MAX effect has explanatory power to explain the negative risk – return relationship in the Australian stock market. Our study uses monthly and yearly MAX factors; the MAX (M) factor is constructed by the highest daily return in the previous month, while MAX (Y) refers to the highest daily return in the previous years. The MAX sorted quintile portfolios fail to exhibit a decreasing return trend in Panel A of Table A9 in the online appendix.
Following Akhtar, Faff, Oliver, and Subrahmanyam (Citation2011), we use the Westpac-Melbourne Institute consumer sentiment index to proxy for Australia’s consumer sentiment. The index is issued monthly, and we focus on the change of direction of the current month’s index compared with the previous month. Hence, we divide the whole sample into two subsample periods: confidence increased (when current month consumer sentiment is higher than the previous month), and confidence decreased (when current month consumer sentiment is lower than the previous month). The Fama – MacBeth regression in Table A10 reports similar results for both periods, suggesting that consumer sentiment cannot explain our study’s negative risk – return relationship.
In short, we find none of the three behavioural finance effects can explain the unique finding of a negative risk – return relationship in the Australian stock market in our study. However, other approaches in the behavioural finance studies might shed more light on the negative risk – return relationship in Australia.
IV. Conclusion
This study extends Alles and Murray’s (Citation2017) firm-level downside risk Australian stock market study, by predicting future stock returns through an extended sample and newly developed realized semibeta models by Bollerslev, Patton, and Quaedvlieg (Citation2022). We observe unique findings that both CAPM and downside beta report consistent negative coefficients, which extends Alles and Murray’s findings. The realized semibeta models report that BetaNP (which measures the individual stock move in the opposite direction from the upward stock market) and BetaNN (which measures the individual stock move in the same direction as the downward stock market) negatively predict next-month firm-level stock returns contradicting to the classical finance theory. These results differ from existing semibetas studies (Bollerslev, Patton, and Quaedvlieg Citation2022; Chu Citation2022). Firstly, none of the studies reported BetaNN with a negative coefficient estimate. However, our findings are robust and consistent with the existing downside beta study by Alles and Murray (Citation2017), whose findings show that the downside beta has a negative relationship with the stock return in the downturn market, which is conceptually the same as the BetaNN measurement. Secondly, BetaNP has not previously been shown to have explanatory power outside Australia.
The semibeta model dissects the covariation measured by CAPM beta into four parts, which provide an alternative way to re-examine the various market anomalies. This study identifies that the negative relationship between downside beta and stock returns observed in the existing Australian studies is derived from the negative relationship between semibeta BetaNN and stock returns. Our findings also raise a concern that the predictive power of asset pricing models may behave differently in regional markets, of which practitioners and researchers should be aware.
We provide several plausible explanations based on behavioural finance and attempt to understand why Australian investors do not receive downside risk premiums. We find that none of the mechanisms can explain the negative risk – return relationship. And we leave the exploration of this intriguing puzzle for further studies. In the meantime, future international semibetas studies are also required to shed more light on the explanatory power of semibetas in different stock markets.
Supplemental Material
Download (43.5 KB)Disclosure statement
No potential conflict of interest was reported by the author(s).
Supplemental material
Supplemental data for this article can be accessed online at https://doi.org/10.1080/00036846.2024.2337809.
Notes
1 Although there are other stock exchanges in Australia, such as the Sydney Stock Exchange (SSX) and the National Stock Exchange (NSX), the Australian Securities Exchange (ASX) is the dominant stock exchange in terms of the number of firms and total market capitalization.
2 Firstly, we define and remove any day as a non-trading day if more than 90% of stocks have zero returns on that day. Secondly, we remove any stock in certain months if more than 80% of the trading days have a zero return. Finally, we also remove trading days for all stocks if the total return index is below 0.1, which is an unrealistic value if the stock is still actively traded (Ince and Porter Citation2006; Karolyi, Lee, and van Dijk Citation2012).
3 In addressing the few high correlations within the independent variables and the concern about possible multicollinearity issue, we perform the Variance Inflation Factor (VIF) test. The results show that no single VIF score is greater than 10.
4 We lose one-month data as we are running a predictive regression starting from month rather than from month
.
5 We compare the monthly individual stock trading volume between the current and previous period, and remove any stock showing same trading volume in the two periods.
6 Refer to Table A2 in online Appendix for details.
7 The four semibetas regression from 1 January 1985 to 31 January 2022 (based on overlapping monthly betas that are constructed based on the past 12 months) have 143,791 monthly observations. In comparison, the monthly betas based on current month daily return report 162,346 monthly observations. For further information refer to Table A3 in the online Appendix.
8 Refer to Table A4 in the online Appendix for details.
9 We remove the bottom 20% stocks each month based on market capitalization before picking the top 200 stocks. This procedure ensures that we do not choose the small-cap stocks when the monthly total stock number is less than 200.
10 Refer to Table A5 in the online Appendix for details.
11 Refer to Table A6 in the online Appendix for details.
12 Refer to Table A7 in the online Appendix for details.
References
- Ahadzie, R. M., and N. Jeyasreedharan. 2023. “Higher-Order Moments and Asset Pricing in the Australian Stock Market.” Accounting & Finance 64 (1): 75–128. https://doi.org/10.1111/acfi.13135.
- Akhtar, S., R. Faff, B. Oliver, and A. Subrahmanyam. 2011. “The Power of Bad: The Negativity Bias in Australian Consumer Sentiment Announcements of Stock Returns.” Journal of Banking & Finance 35 (5): 1239–1249. https://doi.org/10.1016/j.jbankfin.2010.10.014.
- Alles, L., and L. Murray. 2013. “Rewards for Downside Risk in Asian Markets.” Journal of Banking & Finance 37 (7): 2501–2509. https://doi.org/10.1016/j.jbankfin.2013.02.006.
- Alles, L., and L. Murray. 2017. “Asset Pricing and Downside Risk in the Australian Share Market.” Applied Economics 49 (43): 4336–4350. https://doi.org/10.1080/00036846.2017.1282143.
- Amihud, Y. 2002. “Illiquidity and Stock Returns: Cross-Section and Time-Series Effects.” Journal of Financial Markets 5: 31–56.
- Andersen, T. G., T. Bollerslev, F. X. Diebold, and H. Ebens. 2001. “The Distribution of Realized Stock Return Volatility.” Journal of Financial Economics 61: 43–76.
- Ang, A., J. Chen, and Y. Xing. 2006. “Downside Risk.” The Review of Financial Studies 19 (4): 1191–1239. https://doi.org/10.1093/rfs/hhj035.
- Ang, A., R. J. Hodrick, Y. Xing, and X. Zhang. 2006. “The Cross-Section of Volatility and Expected Returns.” The Journal of Finance 61 (1): 259–299. https://doi.org/10.1111/j.1540-6261.2006.00836.x.
- Atilgan, Y., T. G. Bali, K. O. Demirtas, and A. D. Gunaydin. 2018. “Downside Beta and Equity Returns Around the World.” The Journal of Portfolio Management 44 (7): 39–54. https://doi.org/10.3905/jpm.2018.1.080.
- Atilgan, Y., T. G. Bali, K. O. Demirtas, and A. D. Gunaydin. 2020. “Left-Tail Momentum: Underreaction to Bad News, Costly Arbitrage and Equity Returns.” Journal of Financial Economics 135 (3): 725–753. https://doi.org/10.1016/j.jfineco.2019.07.006.
- Atilgan, Y., K. O. Demirtas, and A. D. Gunaydin. 2020. “Downside Beta and the Cross Section of Equity Returns: A Decade Later.” European Financial Management 26 (2): 316–347. https://doi.org/10.1111/eufm.12258.
- Bali, T. G., S. J. Brown, S. Murray, and Y. Tang. 2017. “A Lottery-Demand-Based Explanation of the Beta Anomaly.” Journal of Financial and Quantitative Analysis 52 (6): 2369–2397. https://doi.org/10.1017/S0022109017000928.
- Banz, R. W. 1981. “The Relationship Between Return and Market Value of Common Stocks.” Journal of Financial Economics 9: 3–18.
- Bawa, V. S. 1975. “Optimal Rules for Ordering Uncertain Prospects.” Journal of Financial Economics 2 (1): 95–121. https://doi.org/10.1016/0304-405X(75)90025-2.
- Bawa, V. S., and E. B. Lindenberg. 1977. “Capital Market Equilibrium in a Mean-Lower Partial Moment Framework.” Journal of Financial Economics 5 (2): 189–200. https://doi.org/10.1016/0304-405X(77)90017-4.
- Black, F., M. C. Jensen, and M. Scholes. 1972. The Capital Asset Pricing Model: Some Empirical Tests. New York: Praeger.
- Bollerslev, T., J. Li, A. J. Patton, and R. Quaedvlieg. 2020. “Realized semicovariances.” Econometrica 88 (4): 1515–1551. https://doi.org/10.3982/ECTA17056.
- Bollerslev, T., A. J. Patton, and R. Quaedvlieg. 2022. “Realized Semibetas: Disentangling “Good” and “Bad” Downside Risks.” Journal of Financial Economics 144 (1): 227–246. https://doi.org/10.1016/j.jfineco.2021.05.056.
- Bradrania, R., and J. F. Veron. 2023. “The Beta Anomaly in the Australian Stock Market and the Lottery Demand.” Pacific-Basin Finance Journal 77:101903. https://doi.org/10.1016/j.pacfin.2022.101903.
- Cakici, N., A. Zaremba, R. J. Bianchi, and N. Pham. 2021. “False Discoveries in the Anomaly Research: New Insights from the Stock Exchange of Melbourne (1927-1987).” Pacific-Basin Finance Journal 70:101675. https://doi.org/10.1016/j.pacfin.2021.101675.
- Chan, H., X. Chang, R. Faff, and G. Wong. 2010. “Financial Constraints and Stock Returns — Evidence from Australia.” Pacific-Basin Finance Journal 18 (3): 306–318. https://doi.org/10.1016/j.pacfin.2010.02.004.
- Chen, X., B. Li, C. Andrew, and Worthington. 2022. “Economic Uncertainty and Australian Stock Returns.” Accounting & Finance 62 (3): 3441–3474. https://doi.org/10.1111/acfi.12892.
- Christoffersen, P., M. Fournier, K. Jacobs, and M. Karoui. 2021. “Option-Based Estimation of the Price of Coskewness and Cokurtosis Risk.” Journal of Financial and Quantitative Analysis 56 (1): 65–91. https://doi.org/10.1017/S002210902000023X.
- Chu, P. K. 2022. “Semibeta Asset Pricing in the Korean Stock Market.” Finance Research Letters 50:103245. https://doi.org/10.1016/j.frl.2022.103245.
- Dittmar, R. F. 2002. “Nonlinear Pricing Kernels, Kurtosis Preference, and Evidence from the Cross Section of Equity Returns.” The Journal of Finance 57 (1): 369–403. https://doi.org/10.1111/1540-6261.00425.
- Doan, M. P., C.-T. Lin, M. Chng, and D. Gallagher. 2014. “Higher Moments and Beta Asymmetry: Evidence from Australia.” Accounting and Finance 54 (3): 779–807. https://doi.org/10.1111/acfi.12022.
- Durand, R. B., M. Limkriangkrai, D. Chai, and D. Gallagher. 2016. “The Australian Asset-Pricing Debate.” Accounting and Finance 56 (2): 393–421. https://doi.org/10.1111/acfi.12097.
- Elliot, B., P. Docherty, S. Easton, and D. Lee. 2018. “Profitability and Investment-Based Factor Pricing Models.” Accounting and Finance 58 (2): 397–421. https://doi.org/10.1111/acfi.12217.
- Faff, R. 2001. “A Multivariate Test of a Dual-Beta CAPM: Australian Evidence.” Financial Review 36 (4): 157–174. https://doi.org/10.1111/j.1540-6288.2001.tb00034.x.
- Fama, E. F., and K. R. French. 1992. “The Cross-Section of Expected Stock Returns.” The Journal of Finance 47: 427–465.
- Fama, E. F., and J. D. MacBeth. 1973. “Risk, Return, and Equilibrium: Empirical Tests.” The Journal of Political Economy 81 (3): 607–636. https://doi.org/10.1086/260061.
- Frazzini, A., and L. H. Pedersen. 2014. “Betting Against Beta.” Journal of Financial Economics 111 (1): 1–25. https://doi.org/10.1016/j.jfineco.2013.10.005.
- Galagedera, D. U. A., E. A. Maharaj, and R. Brooks. 2008. “Relationship Between Downside Risk and Return: New Evidence Through a Multiscaling Approach.” Applied Financial Economics 18 (20): 1623–1633. https://doi.org/10.1080/09603100701720435.
- Gaunt, C. 2004. “Size and Book to Market Effects and the Fama French Three Factor Asset Pricing Model: Evidence from the Australian Stock Market.” Accounting and Finance 44 (1): 27–44. https://doi.org/10.1111/j.1467-629x.2004.00100.x.
- Gharghori, P., R. Lee, and M. Veeraraghavan. 2009. “Anomalies and Stock Returns: Australian Evidence.” Accounting and Finance 49 (3): 555–576. https://doi.org/10.1111/j.1467-629X.2009.00298.x.
- Gul, F. 1991. “A Theory of Disappointment Aversion.” Econometrica 59 (3): 667–686. https://doi.org/10.2307/2938223.
- Han, X., K. Li, and Y. Li. 2020. “Investor Overconfidence and the Security Market Line: New Evidence from China.” Journal of Economic Dynamics and Control 117:103961. https://doi.org/10.1016/j.jedc.2020.103961.
- Harvey, C. R., and A. Siddique. 2000. “Conditional Skewness in Asset Pricing Tests.” The Journal of Finance 55 (3): 1263–1295. https://doi.org/10.1111/0022-1082.00247.
- Ince, O. S., and R. B. Porter. 2006. “Individual Equity Return Data from Thomson Datastream: Handle with Care!” Journal of Financial Research 29 (4): 463–479. https://doi.org/10.1111/j.1475-6803.2006.00189.x.
- Jegadeesh, N. 1990. “Evidence of Predictable Behavior of Security Returns.” The Journal of Finance 45: 881–898.
- Jegadeesh, N., and S. Titman. 1993. “Returns to Buying Winners and Selling Losers: Implications for Stock Market Efficiency.” The Journal of Finance 48: 65–91.
- Karolyi, G. A., K.-H. Lee, and M. A. van Dijk. 2012. “Understanding Commonality in Liquidity Around the World.” Journal of Financial Economics 105 (1): 82–112. https://doi.org/10.1016/j.jfineco.2011.12.008.
- Kraus, A., and R. H. Litzenberger. 1976. “Skewness Preference and the Valuation of Risk Assets.” The Journal of Finance 31 (4): 1085–1100. https://doi.org/10.1111/j.1540-6261.1976.tb01961.x.
- Lettau, M., M. Maggiori, and M. Weber. 2014. “Conditional Risk Premia in Currency Markets and Other Asset Classes.” Journal of Financial Economics 114 (2): 197–225. https://doi.org/10.1016/j.jfineco.2014.07.001.
- Levi, Y., and I. Welch. 2021. “Symmetric and Asymmetric Market Betas and Downside Risk.” The Review of Financial Studies 33 (6): 2772–2795. https://doi.org/10.1093/rfs/hhz108.
- Lintner, J. 1965. “The Valuation of Risk Assets and the Selection of Risky Investments in Stock Portfolios and Capital Budgets.” The Review of Economics and Statistics 47 (1): 13–37. https://doi.org/10.2307/1924119.
- Long, H., A. Zaremba, W. Zhou, and E. Bouri. 2022. “Macroeconomics Matter: Leading Economic Indicators and the Cross-Section of Global Stock Returns, 2022.” Journal of Financial Markets 61:100736. https://doi.org/10.1016/j.finmar.2022.100736.
- Markowitz, H. 1959. Portfolio Selection: Efficient Diversification of Investments. New Haven: Yale University Press.
- Sharpe, W. F. 1964. “Capital Asset Prices: A Theory of Market Equilibrium Under Conditions of Risk.” The Journal of Finance 19 (3): 425–442. https://doi.org/10.1111/j.1540-6261.1964.tb02865.x.
- Shi, J., M. Ausloos, and T. Zhu. 2022. “If Global or Local Investor Sentiments Are Prone to Developing an Impact on Stock Returns, Is There an Industry Effect?” International Journal of Finance & Economics 27 (1): 1309–1320. https://doi.org/10.1002/ijfe.2216.
- Tiwari, A. K., E. J. A. Abakah, C. O. Bonsu, N. K. Karikari, and S. Hammoudeh. 2022. “The Effects of Public Sentiments and Feelings on Stock Market Behavior: Evidence from Australia.” Journal of Economic Behavior and Organization 193:443–472. https://doi.org/10.1016/j.jebo.2021.11.026.
- Van Eyden, R., R. Gupta, J. Nielsen, and E. Bouri. 2023. “Investor Sentiment and Multi-Scale Positive and Negative Stock Market Bubbles in a Panel of G7 Countries.” Journal of Behavioural and Experimental Finance 38:100804. https://doi.org/10.1016/j.jbef.2023.100804.
- Wen, Y.-C., B. Li, X. Chen, and T. Singh. 2023. “Spillover Effects of the US Stock Market and the Predictability of Returns: International Evidence Based on Daily Data.” Applied Economics 55 (45): 5251–5266. https://doi.org/10.1080/00036846.2022.2138818.
- Zhong, A. 2022. “Institutional Trading in Stock Market Anomalies in Australia.” Accounting and Finance 62 (1): 893–930. https://doi.org/10.1111/acfi.12813.
- Zhong, A., and P. Gray. 2016. “The MAX Effect: An Exploration of Risk and Mispricing Explanations.” Journal of Banking & Finance 65:76–90. https://doi.org/10.1016/j.jbankfin.2016.01.007.