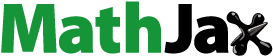
ABSTRACT
Findings in the traffic safety literature suggest that people value traffic risk reductions less when framed as a public good (e.g. infrastructure improvements) compared to when framed as a private good (e.g. personal safety equipment). This study contributes to this literature by reporting empirical evidence for the importance of controlling for the attributes of the goods used in such valuations. We focus on risks faced by vulnerable road users such as cyclists and pedestrians and compare valuations across private and public goods that vary in their attributes. When the goods are of an identical nature, we find no significant difference in valuations, resolving the controversy in previous findings. We find significant effects on valuations from using private or public provision, from offering the good as voluntary or mandated in use, and from changing the framing of the good between the private and the public versions. This adds further weight to the importance of controlling for many attributes.
I. Introduction
Empirical studies of willingness to pay (WTP) for traffic safety measures show that a reduction in the risk of traffic accidents (henceforth risk reduction) often is valued higher when the safety measure is framed as a private good than when it is framed as a public good (e.g. H. Andersson and Lindberg Citation2009; Hultkrantz, Lindberg, and Andersson Citation2006; Lindhjelm et al. Citation2011; Svensson and Vredin Johansson Citation2010). This result is contrary to theoretical expectations since valuations of public goods may include altruism, something that is not as much part of private goods evaluations. The excess of private valuations over public ones has been shown to be as high as 80% and 160% (e.g. H. Andersson and Lindberg Citation2009; Hultkrantz, Lindberg, and Andersson Citation2006; Svensson and Vredin Johansson Citation2010). Thus, if private valuations are used to estimate WTP for public good provisions it can lead to substantial biases. Most previous studies of these valuation differences frame the private and public goods using only vague descriptions. This can introduce perception differences across respondents and consequently confound elicited WTP. Furthermore, in those studies where the good is more clearly specified, the good is not the same and thus not fully comparable between the public and the private framing, again confounding WTP. In this article, we focus on attribute differences between pure private and pure public goods with an aim to identify some sources of valuation differences.
We make a twofold contribution to the literature. First, we introduce a traffic safety measure which is of an identical nature across the private-public dimension, thus minimizing affective reactions as pointed out by Gyrd-Hansen (Citation2016). This allows us to test whether the private-public valuation differences in previous studies are due to framing differences. The good we use is a downloadable mobile phone app that connects to sensors that are assumed available in all motor vehicles. Second, while keeping the nature of the good constant, we vary how the good is financed and provided (i.e. developed and produced), thus bridging the gap between the pure private and the pure public good in steps. We investigate the differences in WTP due to private and public financing, as well as private and public provision. All versions of the public good are offered with uniform tax financing, thus maintaining non-excludability. The pure private good is at one end of the spectrum with excludability in use, private payments for access, and private provision. The pure public good is at the other end of the spectrum with non-excludability, uniform tax financing, and public provision. It can also be argued that a downloadable app is non-rival since the marginal cost for additional downloads is negligible, but this applies both to the private and the public versions. An additional attribute we investigate is mandatory use, which ties in with the attributes of many fixed public installations in traffic safety, such as signals and sensors. These types of public goods have commonly been used in the literature showing the excess of private over public valuations.
Our respondents are sampled from an online survey population in Sweden. As has been the case in many countries, the electrification of bicycles and scooters and the switch from public transit to the use of bicycles and scooters during the COVID-19 pandemic have led to more diverse blends of experienced and non-experienced, and fast and slow users of roads, pavements, and sidewalks. This has spurred much concern for the safety of vulnerable road users at both national and local government levels (Holmberg and Gustavsson Citation2020). Despite the magnitude and severity of accidents among vulnerable road users, there are only a few studies of the WTP for safety measures for pedestrians and cyclists. This study therefore also contributes to the understanding of this aspect of traffic risk reductions.
We find that our good, the mobile phone app, results in the same WTP for its private form and its public form. We do find that the fixed public installation is valued higher than any other good, whether private or public. We also find that introducing private provision or mandated use to the public app lowers WTP. These findings all point to the importance of controlling for the attributes of the goods. Previous findings that private goods are valued higher than public goods may be explained by lack of such controls.
II. Background and empirical evidence
Based on classical utility maximization choice theory, the same risk reduction framed as a public of private good should be valued the same. However, if individuals are altruistic and care about the safety of others as well as for themselves, the public investment could be valued higher than the private safety device (Jones-Lee Citation1976; Mishan Citation1971; Needleman Citation1976; Viscusi, Magat, and Forrest Citation1988). In the context of traffic, several survey studies have found valuations of private goods to be higher than public goods, rejecting such altruistic predictions over safety access (H. Andersson and Lindberg Citation2009; de Blaeij et al. Citation2003; Hultkrantz, Lindberg, and Andersson Citation2006; Johannesson, Johansson, and O’Connor Citation1996; Svensson and Vredin Johansson Citation2010). On the other hand, studies with a health focus have found that public settings generate higher valuations than private ones. An essential explanation for why public valuations may not exceed, and perhaps even fall below, private ones has been explored focusing on payment vehicles. If altruism is expressed not just over the safety effect but over the full utility effect on others, including financial consequences, the latter may lead to no or even a negative impact on valuation of public investments when financed through uniform taxes (Bergstrom Citation1982, Citation2006; Johannesson, Johansson, and O’Connor Citation1996; Johansson Citation1994; Jones-Lee Citation1991, Citation1992). However, the magnitude of the excesses of private over public values in many studies cannot be solely attributed to altruism over financial consequences.
Gyrd-Hansen, Kjaer, and Nielsen (Citation2016) address this issue by comparing valuations based on differences in payment vehicles. In one public good condition, they frame the payment vehicle as tax financing, implying that any expressed WTP applies to all, potentially triggering altruism over financial consequences. In another public good condition, the payment vehicle was framed so each person pays only what they themselves bid, thereby avoiding any altruism over financial consequences. They find a lower WTP in the former condition, and evidence that respondents believe that others will bid lower than themselves. This is consistent with the presence of financial altruism and an underestimation of public valuations.
Furthermore, Bergstrom (Citation2006) demonstrates that, under a wide set of assumptions, for a consumer of a public good the social value, i.e. the value that includes altruism, is the same as the private value. One of these assumptions is first-best taxation, meaning that individual tax contributions equal individual private benefits, resulting in zero net benefits. Beeson et al. (Citation2019) relax this assumption and find that under uniform taxation, WTP values become distorted, potentially leading to over- or under-provision of the public good, depending on the relative strengths of altruism. The joint existence of safety altruism and financial altruism is often referred to as pure altruism, encompassing all utility effects for others.
Svensson and Vredin Johansson (Citation2010) investigate whether pro- or anti-government attitudes further affect WTP for private versus public goods. They report evidence supporting this, but most effects have low significance levels (p < 0.10) with two exceptions (p < 0.05): in a within-sample survey, respondents who agree that individuals should bear the cost for risk reduction express a WTP for the private good significantly higher than their WTP for the public good. A similar pattern emerges for respondents who agree with the statement that public providers should not be involved in private markets. In a related study, Hultkrantz, Lindberg, and Andersson (Citation2006) investigate the impact of respondent confidence levels, bid levels in a dichotomous choice format, and provision points on the private-public valuation disparity, and find that none of these factors affect the observed difference in valuations. Hultkrantz, Lindberg, and Andersson (Citation2006, 155) note that ‘ … respondents may perceive a publicly funded measure as an “impure public good” providing benefits that are more valuable to other citizens than to themselves; or they may be suspicious of the quality of services provided by public agencies’. Viscusi, Magat, and Forrest (Citation1988) also suggest the presence of preferences based on private or public provision.
However, most previous studies present the private and public framing without clearly specifying the underlying good used to deliver the traffic safety improvement. For example, the private good can be defined as ‘a safety device’ (e.g. H. Andersson and Lindberg Citation2009; Hultkrantz, Lindberg, and Andersson Citation2006; Svensson and Vredin Johansson Citation2010) and the public good as a ‘road traffic safety programme’ (e.g. Hultkrantz, Lindberg, and Andersson Citation2006), a ‘public road safety investment’ (e.g. Svensson and Vredin Johansson Citation2010) or a ‘safety program’ (e.g. H. Andersson et al. Citation2013). Gyrd-Hansen, Kjaer, and Nielsen (Citation2016, 185) state that ‘It is a challenge to present a public and a private good holding all other characteristics constant in order to avoid affective reactions’. In the area of health, it has been shown to be easier to find private and public goods that only vary through the payment vehicle. For example, Gyrd-Hansen (Citation2016) examines valuations of private and public ambulance helicopter services and finds that the WTP for the public provision exceeds the WTP for the private alternative. Similarly, Pedersen, Gyrd-Hansen, and Kjaer (Citation2011) find higher valuations for prostate cancer screenings when made publicly accessible rather than privately accessible.
III. Study design
In this study, we contribute to the traffic risk literature by investigating if the higher valuation of the private compared to the public good is due to the goods being vaguely defined and quite different across the private and public settings. We introduce a good that is the same across the private and public settings. This good is a downloadable mobile phone app (hereafter app) that connects to instruments in cars to alert drivers of the presence of pedestrians and cyclists. The app scenario is inspired by, but not identical to, technologies that were demonstrated and tested within the InDev, PROSPECT and XCYCLE projects funded by the EU HORIZON 2020. The payment vehicle of the public good is a uniform tax, thus making the good non-excludable. One can also argue that a downloadable app is non-rival due to the marginal cost being negligible, but this applies to both the private and the public versions. The public good is also provided, i.e. developed and produced, by a public agency, the Swedish Transport Administration. Following Hultkrantz, Lindberg, and Andersson (Citation2006) we test whether private provision results in higher valuations, possibly motivated by a lack of trust in the quality provided by the public sector. In addition, to facilitate comparisons to previous traffic safety studies that frame the public good as a traffic safety programme, sometimes providing examples such as street light improvements and improved signage (Gyrd-Hansen, Kjaer, and Nielsen Citation2016), we also include a public good requiring a fixed installation investment: sensors at intersections between cars and bicycles. Based on the literature, we expect that the WTP for this good will be lower than for the private app. One important attribute of this fixed installation is that pedestrians and cyclists cannot avoid using it. Because of this, we also introduce a variant of the app which is mandatory in use to test whether this lowers WTP. This comprehensive approach allows for a nuanced exploration of factors influencing valuations in the context of traffic safety.
To elicit WTP, we show respondents five monetary amounts: SEK 0, 10, 25, 50, and 100, and they are asked to choose the amount that is the most that they would be willing to pay. This is the same as a series of five dichotomous choice tasks presented simultaneously, thus giving us more precise information than a single dichotomous choice task. Gyrd-Hansen (Citation2016) compares using payment cards to open-ended elicitation and finds no significant differences. Hultkrantz, Lindberg, and Andersson (Citation2006) compare a dichotomous choice approach to a provision point and find no significant difference. Additional support for using payment cards is given by demonstrations that dichotomous choice mechanisms lead to less hypothetical bias than open-ended elicitations (Harrison and Rutström Citation2008). Another mechanism we include which further weakens possible hypothetical biases in responses is controlling for how certain respondents are regarding their reported WTP (Blumenschein et al. Citation1998; Johannesson, Liljas, and Johansson Citation1998). Contingent valuation methods, despite the risk of hypothetical biases, serve an important purpose in circumstances where it is not possible to observe choices in real settings.
The survey
The data used in this study come from a contingent valuation survey conducted in April – May 2020, which was first tested on both a small focus group and a pilot sample of students in November 2019. The full survey is presented in Appendix A online. The sampling procedure resembles that of several recent studies in this field; see e.g. Olofsson et al. (Citation2019b, Citation2019a). The data were collected from a sample of the web panel Userneeds,Footnote1 aged 18–80. To gather a sample of 1,022 responses, a total of 7,448 members were invited to answer the survey. This corresponds to a response rate of 14%, which, while not being unusually low for online surveys, still implies that caution should be applied when drawing inference outside of the sample.Footnote2 The sample is representative for the Swedish adult population by gender, age, and geographical region; see Table B1 in Appendix B online.
After a short introduction that presents the aim of our study, the survey collects a few demographic characteristics of the respondents (age, gender, and geographic location) and their perceived risk as a cyclist or pedestrian of being in a traffic accident that is severe enough that a visit to the emergency room is necessary. The main research questions collect information about the respondents’ willingness to pay for a traffic safety measure that would reduce by half the risk to be involved in a traffic accident that would cause a visit to the emergency room as a cyclist or pedestrian.
The survey has five different scenarios, labelled A-E, with the same risk reduction (),Footnote3 where the first four (A-D) are all based on the app. A is the private good, B is the public good with public provision and voluntary use, C introduces private provision of the public good, and D introduces mandatory use but maintains public provision. Last, scenario E is a public good with fixed installation of sensors. It is by its nature mandatory to use and provided by a public organization. All public goods are financed through uniform taxes.
Table 1. Survey scenarios by attribute.
All respondents answered questions about scenario B, i.e. the base public app, and one other scenario (i.e. A, C, D, or E), which was randomly allocated. To control for possible order effect, we also randomized if the respondent got scenario B first or second. Since each respondent answered questions about two scenarios, our data have a panel structure, which facilitates panel data estimations. The use of a common scenario allows us to anchor the covariance of the errors across scenarios in the estimation models.
Since respondents often find it difficult to understand a change in small probabilities (Hammitt and Graham Citation1999), additionally to the relative numbers, we also inform the respondents about how the risk reduction would influence the absolute number of accidents in a small town (with 10,000 inhabitants), i.e. ‘ … if all citizens would use the mobile phone app, the number of accidents among unprotected road users in a town with 10,000 inhabitants would decrease on average from 20 to 10 per year’. This type of information has been suggested to be a good complement to understanding change in probabilities (Calman and Royston Citation1997).
Regardless of whether the payment vehicle is a market price or a uniform tax, we present the respondents with five different monthly payment values (SEK 0, 10, 25, 50 and 100) and ask them to indicate which one is the maximum value that they would payFootnote4; see Box 1, which shows scenario B. Since answers can be influenced by the duration of payment (H. Andersson et al. Citation2013), we also show the respondents the annual equivalent of each monthly payment. When the payment vehicle is a tax, it is stated that the cost of the traffic safety measure is going to be equally divided among all taxpayers, i.e. a uniform tax.
Box 1. Willingness to pay question for scenario B
This proposal means that you will have the opportunity to instal an app in your mobile phone that is paid for with tax money. The app is provided by the Swedish Transport Administration. It is voluntary to use the app and you, and anyone else who wants to, will be able to download it without additional payment. The cost is shared equally between you and all other taxpayers and is paid through a tax increase. It will not be possible to buy a similar app from private companies.
How much the tax is going to be increased depends on several factors that are still unknown. We currently assume that all levels below are equally likely. Assume that the proposed intervention will be implemented if enough people answer yes to the tax increase that ultimately applies.
Mark the largest tax increase you would vote yes to
∘ 0 SEK per month (0 SEK per year)
∘ 10 SEK per month (120 SEK per year)
∘ 25 SEK per month (300 SEK per year)
∘ 50 SEK per month (600 SEK per year)
∘ 100 SEK per month (1200 SEK per year)
To reduce the potential problem of hypothetical bias we include a cheap talk script reminding them about their budget constraint and that it is easy to exaggerate the willingness to pay by stating: ‘It is easy to exaggerate when answering these kinds of questions, therefore, think about what you really want to pay and can afford. Keep in mind that there are many other things you would like that also cost money’. This type of cheap talk is commonly used and has been shown to have the potential to reduce hypothetical bias (e.g. Cummings and Taylor Citation1999; Johnston et al. Citation2019; Loomis Citation2014). Furthermore, given that certainty calibration has been shown to reduce the problem of hypothetical bias (e.g. Blumenschein et al. Citation2008; Ryan et al. Citation2017) and give more consistent results (e.g. Svensson Citation2009), we also ask the respondents how certain they are that they would buy the good in the private scenarios or vote in favour in the public scenarios. However, only using the certain respondent can also influence the representativeness of the data (Gyrd-Hansen Citation2016) and, therefore, we estimate our models both with and without certainty calibration.
To test for the influence of financial altruism, as proposed by Johansson (Citation1994) and Gyrd-Hansen, Kjaer, and Nielsen (Citation2016), we ask the respondents about their perception of others’ WTP. Financial altruism would only apply if the respondent believed that the other respondents value the good less.
Furthermore, we ask the respondents about their beliefs that our study findings will influence policy (project consequentiality) and their beliefs that the payment as stated will have to be made if the policy is implemented (payment consequentiality) (Zawojska, Bartczak, and Czajkowski Citation2019). To increase the saliency of policy consequentiality, we clearly state that our research is financed by the Swedish Transport Administration, i.e. the government agency responsible for national traffic-safety programmes in Sweden.
Following Svensson and Vredin Johansson (Citation2010) who suggest that the respondents’ attitudes about the desirability and performance of public and private provision of services may explain the valuation disparities, we also ask the respondents about several of their attitudes.Footnote5 The survey ends with questions about the respondent’s experience of accidents, self-risk assessment, socioeconomic and demographic characteristics.
The definition and descriptive statistics of all variables used in this study are reported in Table B2 in Appendix B online. Approximately, 15% of respondents believe that our study findings may affect the implementation of traffic safety interventions (as shown by the variable Project cons. in Table B2). Only 14% of respondents believe that, if an intervention is implemented, the amount they will have to pay will be influenced by our study (as shown by the variable Payment cons). Table B3 shows that the demographic characteristics of respondents across the scenarios are not statistically significantly different (p < 0.01).
IV. Theory
A general utility function
An important factor behind valuation disparities is the types of altruism that have been discussed in both the theory and empirical literature. We consider, with a slight abuse of the framework of Jones-Lee (Citation1992),Footnote6 a society with individuals, each individual i having differentiable utility functions of the following general form:
where and
are the one-year ahead probability of being injured in an accident and wealth, respectively, of an individual i.
is strictly increasing in
and
, and non-decreasing in
and
, where j = 1 …
and j ≠ i, with partial derivatives denoted as
,
,
and
for all i, j.
With this general formulation, we consider three polar cases of the utility function of individual i, as defined by Jones-LeeFootnote7,Footnote8
a. Pure self-interest, when:
b. Pure altruism, when:
c. Safety-focused altruism, when:
A decision model
We now turn to the choice problem that respondents were presented with in this study. For this purpose, we reduce the agents whose safety and wealth are considered in the respondent’s utility function to self (s) and others (o). In addition, we amend the arguments with a vector Y of individual-specific variables, such as socio-economic and attitude variables. As described further below, respondents are asked about their maximum WTP, framed as the compensating variation (τ) for a traffic accident risk reducing intervention in a specific funding and provision context. Assuming that they maximize their subjective expected utility (Seu), they are asked to express τ in the following equation:
where and
are the subjective baseline accident probabilities of self and others, respectively,
and
are the given risk reductions from the safety intervention, which are given exogenously in the scenario descriptions and are the same for all individuals that participate.
is a vector of scenario-specific attributes associated with the safety intervention and Y is a vector of individual-specific covariates.
and
are the respondent’s subjective probability that the measure will be provided for self or others, respectively, and
and
are the respondent’s subjective probability that self or others, respectively, will have to pay the cost.
Except for in the standard private good case (scenario A in ), provision is not certain but is contingent on enough people being willing to pay τ. Therefore, all changes of risk are multiplied by or
and all changes of cost are multiplied by
or
. These probabilities all depend on
and may be less than one. In the case of mandatory use of public good solutions, i.e. scenarios D and E, EquationEquation (5)
(5)
(5) simplifies by the condition
. For all tax financed alternatives,
.
Thus, the decision model for a representative respondent sets the Seu of the proposed safety intervention equal to the utility of the current traffic risk and wealth. Both Seu and u are functions of the individual-specific variables, Y, which do not change with the safety intervention. Seu is also a function of Z, i.e. the attributes that are associated with each specific traffic safety.
An individual who only exhibits safety altruism would have a positive third term in the Seu expression in EquationEquation (5)(5)
(5) and a zero for the fourth term. An individual who exhibits both safety and financial altruism, i.e. pure altruism, would have a negative fourth term. Thus, for an individual who is a pure altruist, altruism matters less to her decisions than if the individual is only a safety altruist.
In summary, the type of altruism that characterizes respondents will affect the relationship between private and public values. Safety altruism tends to increase WTP for the public good, while financial altruism tends to decrease WTP for the public good. However, the empirical literature also expresses the possibility that other factors also affect the disparity in valuations.
V. Hypotheses and descriptive results
Hypotheses
We have four hypotheses regarding the effects of the scenarios on WTP assuming the same risk reduction.
Hypothesis 1 (H1):
Public good versus private good valuation differences
Scenario A, the private good, is separately compared to each of scenarios B, D and E. Scenario B keeps all attributes, except for payment vehicle and provision, the same as scenario A. Scenario D is the same as scenario B, except that its use is mandatory. Scenario E, the public fixed installation of sensors, also keeps the payment vehicle and provision the same as in B. Theory predicts equality based on the simultaneous presence of both safety altruism (increasing WTP for the public good) and financial altruism (decreasing WTP for the public good).
Hypothesis 2 (H2):
Framing
Scenarios E and D both share the attributes of tax financing, non-excludability, public provision, and mandated use. The only difference between the two scenarios is the safety measure per se, i.e. a mobile phone app in scenario D versus infrastructure in scenario E.
Hypothesis 3 (H3):
Mandatory use
Scenarios D and B differ in only one aspect, namely that in D the use of the app is mandatory while in B it is voluntary. Thus, a result where (
) indicates that respondents are in favour of (are not in favour of) a safety solution that requires mandatory use.
Hypothesis 4 (H4):
Public versus private provision
Scenarios B and C differ only in that C uses private provision while B uses public provision (by the Swedish Transport Administration). (
) indicates that respondents value a safety device provided by a private company higher (lower) than the same device provided by a public institution.
Descriptive results
shows some descriptive statistics of the stated WTP. The proportion of responses that are SEK 0 ranges between 30% in scenario E and 50% in scenario C. For both the full sample, i.e. including the zero bids, and the sample without the zero bids, the mean WTP is lowest for scenario C, which is the public good with private provision. For the full sample, the infrastructure scenario E has the highest WTP, with an average WTP of SEK 15.21 and the lowest proportion of SEK 0 responses, followed by scenario B, with an average WTP of SEK 12.01 and the next lowest proportion of SEK 0 responses. While these differences are significant, we also notice that the central tendencies of the distributions, as captured by the median values, are all the same (SEK 10). Thus, the differences across scenarios are generated towards the tails of the distributions.
Table 2. Willingness to pay (WTP) in SEK for reduced risk of accident by provision scenario.
reports the tests of pairwise comparisons of equality of the full sample distributions based on Wilcoxon rank-sum tests.Footnote9 There is a statistically significant difference in WTP between scenario E and scenarios A, C and D (columns 1–3). All these four scenarios were randomly allocated to subsamples of 25% of the respondents, while scenario B was given to all respondents. WTP for each of the scenarios C, D, and E is significantly different from WTP for scenario B (p < 0.01), but the WTP for scenario A is not (column 4).
Table 3. Tests of equality of distributions for WTP in the different scenarios.
Based on the results of these unconditional tests, we cannot reject hypothesis H1 for scenario B; the WTP for the public good is equal to the WTP for the private good. We also cannot reject H1 for scenario D where the use of the public good is mandatory. The only exception is scenario E that has a higher WTP than A. This finding is contrary to most of the literature that motivates our study. Similarly to the tests in the previous literature, the comparison of WTP between scenarios A and E is confounded by the fact that the two goods are different. We reject H2, that the WTP for public goods is not affected by framing; the WTP for Scenario E is significantly higher than the WTP for scenario D. We reject H3, that the WTP does not depend on mandatory use requirements; the WTP for scenario D is lower than the WTP for B. Finally, we also reject H4 that private and public provision are valued the same; the WTP for C is lower than the WTP for B.
In summary, we do not find support for what has been reported in the literature that private goods are valued higher than public goods when it comes to traffic risk. We find that valuations vary with several attributes of the public good: the framing, mandated use, and private provision.
VI. The econometric framework
The outcome variable
Our outcome variable is the respondents’ willingness to pay for a safety measure that would reduce by half the risk of a cyclist or pedestrian to be involved in a traffic accident, severe enough to cause a visit to the emergency room. Given our design of the survey, the exact value of the maximum WTP is not directly observable since we present respondents only with a finite number of possible values, i.e. a discrete price list of SEK 0, 10, 25, 50, and 100. Thus, respondents who state that the most they would pay is SEK 10, could have in mind that they would be willing to pay at least 10, but not as much as SEK 25. Thus, the SEK 10 response is censored in the sense that we cannot observe the true maximum, all we know is that the true value lies in the interval SEK [10–24]. Similarly, if the individual responds SEK 25 or SEK 50, we know that the true WTP must lie in the intervals SEK [25–49] or SEK [50–99], respectively. Responses such as these are therefore interval censored. A response of SEK 100 at the upper end of the price list is right-censored, since the true, but latent, WTP could be higher than SEK 100. A response of SEK 0 at the lower end of the price list is both left-censored and interval-censored. The upper endpoint for the SEK 0 response is SEK 9, and the lower endpoint is some value below SEK 0 reflecting that latent valuations could be negative.Footnote10
Basic setup
The proposed model of the continuous latent outcome variable, , can be stated as
where is a vector of socio-demographic variables with a corresponding coefficient vector
,
is a vector of attitude variables with a corresponding coefficient vector
,
is a vector of variables reflecting risk assessments and accident experiences with a corresponding coefficient vector
,
is a vector of variables reflecting consequentiality, usage and WTP of others with a corresponding coefficient vector
, and
is a vector of dummy variables representing the scenarios with a corresponding coefficient vector
. Given our payment card design, the latent
may fall within a closed interval with two fixed endpoints (interval-censored data)
where is the lower endpoint and
is the upper endpoint of the interval. Alternatively, the latent
may fall within an interval that has a fixed upper endpoint and an open (possibly infinite) lower endpoint (left- and interval-censored data)
or an interval that has a fixed lower endpoint and an unknown upper endpoint (right-censored data).
We assume that are i.i.d. with a normal distribution.Footnote11 The likelihood contribution of each observation is dependent on the conditional probability that
is in an interval defined by one of EquationEquations (7)
(7)
(7) , (Equation8
(8)
(8) ) or (9).
Using the short-hand , the log likelihood is
VII. Results
Main hypotheses tests
We estimate several specifications of our model (6), differing in which covariates are included. Focusing on the key scenario variables, does not give the exact coefficient estimates for all these covariations. The ones included in each specification are indicated by a ‘yes’ in . Scenario B is the reference scenario in all specifications. The full estimated models are shown in Table B4 in Appendix B online.
Table 4. Panel interval regression with scenario dummies.
Regardless of the model specification, the estimated WTP for scenarios C and D are consistently lower compared to scenario B, while the WTP for scenario E consistently shows higher estimates. This supports our unconditional hypotheses tests in . First, for H1 we verify that WTP in scenario A is not significantly different from WTP in scenario B. Wald tests further confirm that scenario D is also not significantly different from A (chi2 = 2.76, p = 0.097), but scenario E is (chi2 = 14.49, p = 0.000) and it is again positive. Second, for H2, using Wald tests, we confirm the unconditional test that E is valued higher than D (chi2 = 29.96, p = 0.000), which is evidence of framing effects. Third, for H3 we confirm that scenario D is valued significantly lower than scenario B: respondents have a negative valuation of mandatory use of the app, however as mentioned above, this is not a decline that is large enough to make D different from the private good A. This negative effect of mandatory use implies that the coefficient estimated for scenario E, the infrastructure good with mandatory use, underestimates the framing effect. Finally, for H4, we confirm that scenario C is valued significantly lower than scenario B, thus concluding that public provision is preferred to private provision.
Our findings suggest that there is no difference in WTP between scenarios featuring a public good and a private good when controlling for the impact of framing. This finding is consistent with the presence of both safety and financial altruism within the domain of public goods. Safety and financial altruism affect the expected utility of the decision-maker in opposite directions, and can cancel each other out under commonly valid assumptions, as presented by Bergstrom (Citation2006).
Apart from our hypotheses tests, we also find empirical evidence for the relationship between other explanatory variables and the WTP; see Table B4 in Appendix B online. Of particular interest are the estimates of the attitudinal variables (model 8), which suggest a positive statistically significant (p < 0.01) association between Safety tax and Tax attitude and WTP. Consequently, participants who favour tax increases to address traffic safety concerns and those who believe that current taxes are not excessively high express higher WTP. This aligns with previous findings (Svensson and Vredin Johansson Citation2010) that show that negative attitudes to higher taxes result in a lower valuation of public goods. We do not find any significant differences in valuations based on demographics (age, gender, income, urban residence, or children in the family). A few of our other covariates show a significant positive effect on WTP: having experienced a car accident, believing that cyclists or pedestrians would download such a safety app, and believing that others have a lower WTP than self. These results validate our data as coming from respondents who pay some attention to their responses since they are consistent with what one would expect.
Robustness tests
Results of several robustness tests are reported in Appendix C online. First, in section C1 we control for order effects in the presentation of scenarios by estimating the model with only the first answer from each respondent. As can be expected, precision is lower, and we can no longer find a significant effect from mandatory use requirements. All other results stand. Second, in section C2 we drop respondents who are not certain of their WTP response, resulting in a larger proportion of SEK 0 responses in scenario B. This translates into a negative estimated value for scenario B in the interval regression model. The marginal effects of the other scenarios are not strongly affected, so the rank ordering of the scenarios is unchanged. Third, in section C3 we exclude respondents who answered ‘don’t know’ to explanatory variables, resulting in no significant changes of the results. The only difference is that the estimate of Tax attitudes is no longer statistically significant. Fourth, in section C4 we exclude respondents who completed the entire questionnaire in less than 4 min (compared to a median duration of 7 min and 45 s), resulting in only minor changes, with the main conclusions still supported. Finally, in section C5, we control for transportation mode, resulting in no significant change of the results. In summary, the robustness tests confirm that our core results are stable.
VIII. Conclusions
This study is motivated by controversial findings in previous empirical studies of traffic accident risk reductions where private goods have been reported as being valued higher than public goods. Using data from an online contingent valuation survey in Sweden, we investigate whether such controversial findings may arise out of the difficulty of presenting private and public goods that are sufficiently identical. We introduce a good that is consistently featured across the private and public presentations, namely a mobile phone app that sends signals to sensors in cars, warning these about the proximity of the cyclist or pedestrian. The main difference between the private and the public good is that the latter is financed through uniform taxes, thus making it non-excludable.
We cannot reject our hypothesis that the private and public goods are valued the same. We do find that introducing private provision of the public app lowers the WTP. From this, we can conclude that the lower public valuation in the literature is not due to the provision agency being public. Further, we find that a public good that is a fixed infrastructure installation leads to higher WTP than the private good. Thus, the use of such installations as public goods in the literature does not explain the lower valuations of public goods reported there. One attribute of such public installations is that they are mandatory, and we find that a mandatory app is valued less than a voluntary app, even when tax financed. Thus, we cannot rule out that mandatory use is an attribute that contributes to the lower valuations of public goods in the literature. However, we find that when the public good is not of an identical nature to the private one, but rather is framed as a fixed installation, it is valued higher than the private good despite that it is mandated in use.
Our results show that using the same goods across the two settings removes the controversial valuations. This also supports Bergstrom’s (Citation2006) hypothesis that in the presence of altruism over the access to the good as well as over the financial costs, the valuations should be similar. Our findings suggest that the selection of goods’ attributes is critical in WTP studies.
Our findings suggest that transferring values for a risk reduction based on a specific good and setting to another context might lead to misleading conclusions when evaluating safety interventions. For example, if one were to use the estimates for WTP reported in the previous literature comparing private and public WTP to evaluate a possible sensor installation in important cyclist crossings, it is unclear if the higher private value or the lower public value should be used. As we demonstrate here, the specifics of the attributes of the traffic safety solution importantly impact the valuations. Further research may explore a wider range of possible safety measures both within and between the private and the public good domains.
Limitations and future research
Our findings are limited to populations that match our online sample, although we have shown that the demographics of our sample matches the Swedish population. Our use of payment cards as the payment vehicle is a potential limitation, although it is preferable to open-ended or dichotomous choice methods. Following the literature, we also did not control for risk attitudes of the respondents, and this factor may be explored in future research. Finally, using a contingent valuation method runs the risk of hypothetical bias, although we employed several standard methods for minimizing this: controlling for certainty in responses, using the payment card method, and including cheap talk scripts.
Supplemental Material
Download Zip (126.5 KB)Acknowledgements
We thank the anonymous reviewers, the Editor, Henrik Andersson, Maria Bratt Börjesson, Gunnar Isacsson, Mikael Svensson, Maria Vredin, participants at Swedish National Road and Transport Research Institute (VTI) seminar, the Society for Benefit Cost Analysis 2021 Annual Conference, and the European Conference 2021 of the Society for Benefit-Cost Analysis for valuable comments on a previous version of the article.
Disclosure statement
No potential conflict of interest was reported by the author(s).
Supplementary material
Supplemental data for this article can be accessed online at https://doi.org/10.1080/00036846.2024.2386844
Additional information
Funding
Notes
1 Userneeds web panel is since 2022 part of Norstat web panel https://norstat.co/company/. Our sampling was managed by the survey company Attityd Karlstad AB.
2 For a discussion on how to judge quality of such surveys see Survey Society of the Swedish Statistical Association (Citation2015).
3 The pilot study only included four different scenarios: A, B, C and E. In the main survey, we added scenario D with mandatory use.
4 During the spring 2020 when the survey was answered, EUR 1 was about SEK 10.5. In the pilot study, we used an open-ended format for the WTP question, which informed us about the range of probable payment.
5 Subhan et al. (Citation2021) find that the intention to pay for a traffic-safety programme in a sample of car drivers in Pakistan is affected by, among others, attitudes and the perceived fairness and effectiveness of the programme.
6 Notice that this formulation is general. It does not specify whether preferences over others’ safety and wealth are linked to others’ true utility functions, beliefs over others’ utility functions or ‘warm glow’ preferences.
7 A fourth special case pointed out by Jones-Lee (Citation1992) is pure paternalism, i.e. when an individual has the same safety-wealth marginal rate of substitution for others as for herself.
8 Notice that these definitions of ‘pure altruism’ relate to marginal utilities. An empirical measurement of the ‘degree of altruism’, or ‘impure altruism’ needs to cope with the issue of disentangling altruism and diminishing marginal utility when wealth endowment is unequal (Gauriot, Heger, and Slonim Citation2020).:
9 Both a Shapiro–Wilk test and a joint test of skewness and kurtosis indicate that valuations in each scenario are not consistent with normal distribution, and hence motivates using Wilcoxon tests.
10 Latent negative valuations could reflect a strong negative value of tax funding or public provision, for instance.
11 We use xtintreg command in Stata 18. This facilitates maximum likelihood estimation of interval response data, assuming normally distributed errors, which is the most common assumption in maximum likelihood approaches to this type of data.
References
- Andersson, H., J. K. Hammitt, G. Lindberg, and K. Sundström. 2013. “Willingness to Pay and Sensitivity to Time Framing: A Theoretical Analysis and an Application on Car Safety.” Environmental & Resource Economics 56 (3): 437–456. https://doi.org/10.1007/s10640-013-9644-0.
- Andersson, H., and G. Lindberg. 2009. “Benevolence and the Value of Road Safety.” Accident Analysis & Prevention 41 (2): 286–293. https://doi.org/10.1016/j.aap.2008.11.009.
- Beeson, M., S. Chilton, M. Jones-Lee, H. Metcalf, and J. Seested Nielsen. 2019. “Can a ‘Veil of ignorance’ Reduce the Impact of Distortionary Taxation on Public Good Valuations?” Journal of Risk and Uncertainty 58 (2–3): 245–262. https://doi.org/10.1007/s11166-019-09306-4.
- Bergstrom, T. C. 1982. “When is a man’s Life Worth More Than His Human Capital?” In The Value of Life and Safety, edited by M. W. Jones-Lee, 3–26. Amsterdam: North-Holland.
- Bergstrom, T. C. 2006. “Benefit-Cost in a Benevolent Society.” The American Economic Review 96 (1): 339–351. https://doi.org/10.1257/000282806776157623.
- Blumenschein, K., G. Blomquist, M. Johannesson, N. Horn, and P. Freeman. 2008. “Eliciting Willingness to Pay without Bias: Evidence from a Field Experiment.” The Economic Journal 118 (525): 114–137. https://doi.org/10.1111/j.1468-0297.2007.02106.x.
- Blumenschein, K., M. Johannesson, G. C. Blomquist, B. Liljas, and R. M. O’Conor. 1998. “Experimental Results on Expressed Certainty and Hypothetical Bias in Contingent Valuation.” Southern Economic Journal 65 (1): 169–177. https://doi.org/10.1002/j.2325-8012.1998.tb00136.x.
- Calman, K. C., and G. H. Royston. 1997. “Personal Paper: Risk Language and Dialects.” BMJ 315 (7113): 939–942. https://doi.org/10.1136/bmj.315.7113.939.
- Cummings, R. G., and L. O. Taylor. 1999. “Unbiased Value Estimates for Environmental Goods: A Cheap Talk Design for the Contingent Valuation Method.” The American Economic Review 89 (3): 649–665. https://doi.org/10.1257/aer.89.3.649.
- de Blaeij, A., R. J. G. M. Florax, P. Rietveld, and E. Verhoef. 2003. “The Value of Statistical Life in Road Safety: A Meta-Analysis.” Accident Analysis & Prevention 35 (6): 973–986. https://doi.org/10.1016/S0001-4575(02)00105-7.
- Gauriot, R., S. A. Heger, and R. Slonim. 2020. “Altruism or Diminishing Marginal Utility.” Journal of Economic Behavior and Organization 180:24–48. https://doi.org/10.1016/j.jebo.2020.09.030.
- Gyrd-Hansen, D. 2016. “The Role of Payment Vehicle in Non-Market Valuations of a Health Care Service: Willingness-To-Pay for an Ambulance Helicopter Service.” Health Economics, Policy, and Law 11 (1): 1–16. https://doi.org/10.1017/S1744133115000018.
- Gyrd-Hansen, D., T. Kjaer, and J. S. Nielsen. 2016. “The Value of Mortality Risk Reductions. Pure Altruism – a Confounder?” Journal of Health Economics 49:184–192. https://doi.org/10.1016/j.jhealeco.2016.07.002.
- Hammitt, J. K., and J. D. Graham. 1999. “Willingness to Pay for Health Protection: Inadequate Sensitivity to Probability?” Journal of Risk and Uncertainty 18 (1): 33–62. https://doi.org/10.1023/A:1007760327375.
- Harrison, G. W., and E. E. Rutström. 2008. “Experimental Evidence on the Existence of Hypothetical Bias in Value Elicitation Methods.” In Handbook of Experimental Economics Results, edited by C. R. Plott and V. L. Smith, 752–767. Vol. 1. Amsterdam: North-Holland.
- Holmberg, R., and J. Gustavsson. 2020. Kommunernas trafiksäkerhetsarbete för oskyddade trafikanter. Swedish Transport Administration, Trafikverket TRV 2018/26243.
- Hultkrantz, L., G. Lindberg, and C. Andersson. 2006. “The Value of Improved Road Safety.” Journal of Risk and Uncertainty 32 (2): 151–170. https://doi.org/10.1007/s11166-006-8291-z.
- Johannesson, M., P.-O. Johansson, and R. M. O’Connor. 1996. “The Value of Private Safety versus the Value of Public Safety.” Journal of Risk and Uncertainty 13 (3): 263–275. https://doi.org/10.1007/BF00056156.
- Johannesson, M., B. Liljas, and P.-O. Johansson. 1998. “An Experimental Comparison of Dichotomous Choice Contingent Valuation Questions and Real Purchase Decisions.” Applied Economics 30 (5): 643–647. https://doi.org/10.1080/000368498325633.
- Johansson, P.-O. 1994. “Altruism and the Value of Statistical Life: Empirical Implications.” Journal of Health Economics 13 (1): 111–118. https://doi.org/10.1016/0167-6296(94)90007-8.
- Johnston, R. J., K. Boyle, W. Adamowicz, J. Bennett, R. Brouwer, T. A. Cameron, W. M. Hanemann, et al. 2019. “Contemporary Guidance for Stated Preference Studies.” Journal of the Association of Environmental and Resource Economists 4 (2): 319–405. https://doi.org/10.1086/691697.
- Jones-Lee, M. W. 1976. The Value of Life: An Economic Analysis. Chicago: University of Chicago Press.
- Jones-Lee, M. W. 1991. “Altruism and the Value of Other People’s Safety.” Journal of Risk and Uncertainty 4:213–219. https://doi.org/10.1007/BF00056126.
- Jones-Lee, M. W. 1992. “Paternalistic Altruism and the Value of Statistical Life.” The Economic Journal 102 (410): 80–90. https://doi.org/10.2307/2234853.
- Lindhjelm, H., S. Navrud, N. A. Braathen, and V. Biausque. 2011. “Valuing Mortality Risk Reductions from Environmental, Transport, and Health Policies: A Global Meta-Analysis of Stated Preference Studies.” Risk Analysis 31 (9): 1381–1407. https://doi.org/10.1111/j.1539-6924.2011.01694.x.
- Loomis, J. B. 2014. “Strategies for Overcoming Hypothetical Bias in Stated Preference Surveys.” journal of agricultural & resource economics 39 (1): 34–46.
- Mishan, E. J. 1971. “Evaluation of Life and Limb: A Theoretical Approach.” Journal of Political Economy 79 (4): 687–705. https://doi.org/10.1086/259784.
- Needleman, L. 1976. “Valuing Other People’s Lives.” Manch Sch Econ & Social Stud 44 (4): 309–342. https://doi.org/10.1111/j.1467-9957.1976.tb00143.x.
- Olofsson, S., U. G. Gerdtham, L. Hultkrantz, and U. Persson. 2019a. “Dread and Risk Elimination Premium for the Value of a Statistical Life.” Risk Analysis: An Official Publication of the Society for Risk Analysis 39 (11): 2391–2407. https://doi.org/10.1111/risa.13341.
- Olofsson, S., U. G. Gerdtham, L. Hultkrantz, and U. Persson. 2019b. “Value of a QALY and VSI Estimated with the Chained Approach.” The European Journal of Health Economics: HEPAC: Health Economics in Prevention and Care 20 (7): 1063–1077. https://doi.org/10.1007/s10198-019-01077-8.
- Pedersen, L. B., D. Gyrd-Hansen, and T. Kjaer. 2011. “The Influence of Information and Private versus Public Provision on Preferences for Screening for Prostate Cancer: A Willingness-To-Pay Study.” Health Policy 101 (3): 277–289. https://doi.org/10.1016/j.healthpol.2011.05.008.
- Ryan, M., E. Mentzakis, S. Jareinpituk, and J. Cairns. 2017. “External Validity of Contingent Valuation: Comparing Hypothetical and Actual Payments.” Health Economics 26 (11): 1467–1473. https://doi.org/10.1002/hec.3436.
- Subhan, F., S. Zhao, E. B. Diop, Y. Ali, and H. Zhou. 2021. “Public Intention to Pay for Road Safety Improvement: A Case Study of Pakistan.” Accident Analysis & Prevention 160:160. https://doi.org/10.1016/j.aap.2021.106315.
- Survey Society of the Swedish Statistical Association. 2015. “Judging Quality of Web Panel Surveys.” Accessed May 26, 2021. Report. https://statistikframjandet.se/survey/wp-content/uploads/2015/10/Webbpanelrapporten-eng.pdf.
- Svensson, M. 2009. “The Value of a Statistical Life in Sweden: Estimates from Two Studies Using the “Certainty Approach” Calibration.” Accident Analysis & Prevention 41 (3): 430–437. https://doi.org/10.1016/j.aap.2009.01.005.
- Svensson, M., and M. Vredin Johansson. 2010. “Willingness to Pay for Private and Public Road Safety in Stated Preference Studies: Why the Difference?” Accident Analysis & Prevention 42 (4): 1205–1212. https://doi.org/10.1016/j.aap.2010.01.012.
- Viscusi, W. K., W. A. Magat, and A. Forrest. 1988. “Altruistic and Private Valuations of Risk Reduction.” Journal of Policy Analysis and Management 7 (2): 227–245. https://doi.org/10.2307/3323826.
- Zawojska, E., A. Bartczak, and M. Czajkowski. 2019. “Disentangling the Effects of Policy and Payment Consequentiality and Risk Attitudes on Stated Preferences.” Journal of Environmental Economics & Management 93:63–84. https://doi.org/10.1016/j.jeem.2018.11.007.