The advent of large language models (LLMs) such as ChatGPT and text-to-image generation systems like DALL-E developed by OpenAI has raised critical questions in education and art communities, urging us not only to reconceptualize our understanding of creativity, authorship, and human–machine relations, but also to examine their social and ethical implications for teaching and learning. Particularly because the data sets to train many generative artificial intelligence (AI) models are not made available to the public and their operating mechanisms are opaque to human reasoning, it is difficult to predict their potential harm to the field of art education. Anthropologist Hannah Knox (Citation2021) described that we are entering a different phase of challenges driven by these emerging technologies:
Here we have gone far beyond the digital divide, a problem essentially of access, into a situation where poverty, racism, nationalism, violence, misogyny and gross levels of capital accumulation are sustained by and supported by opaque informational infrastructures with powerful real-world effects. (p. 184)
Visual culture education has been emphasized in art education as contemporary society is saturated with visual images (Duncum, Citation2002). Postdigital conditions, in which emerging technologies increasingly permeate every dimension of our lives (Berry, Citation2014), complicate the ecosystem of imagery by inviting millions of images that are produced by generative AI models such as DALL-E, Stable Diffusion, and Midjourney every day; the landscape of visual culture is increasingly entangled with visuality created by both humans and nonhumans (Contreras-Medina & Marín, Citation2022; Manovich, Citation2017; Routhier et al., Citation2022; Sweeny, Citation2005). Therefore, it is important to develop effective art pedagogies that can empower students to engage critically with these emergent intelligences to understand how they shape the ways we see ourselves, others, and the world through imagery.
In this article, I explore social and ethical implications of generative AI models that need to be addressed in art education by investigating how DALL-E 2 visualizes existing power structures. Because OpenAI does not provide access to data sets for DALL-E 2, I attempt to examine inductively racialized assumptions and biases inscribed in the system through a method of critical content analysis suggested by new media artist and researcher Eryk Salvaggio (Citation2022). Then, I will discuss the implications of the findings for art teaching and learning.
Biases in Generative AI
The emergence of generative AI models has engendered ongoing debates and concerns. Scholars and artists have raised issues of authorship and copyright infringement (Aktay, Citation2022; Cetinic & She, Citation2022; Dehouche & Dehouche, Citation2023; Vartiainen & Tedre, Citation2023), as well as accusations of reinforcing biases against certain groups of people based on race, gender, class, and dis/abilities (Bianchi et al., Citation2022; Dehouche, Citation2021; Johnson, Citation2022). Bianchi et al. (Citation2022) stated that generative AI models repeat intersectional stereotypes of marginalized people and communities, which contribute to reifying social categories and justifying discrimination, hostility, and violence against them. For example, image-generation tools and face filters have portrayed women in a hypersexualized manner by underdressing them (Birhane et al., Citation2021; Heikkilä, Citation2022), and generative AI has also been “weaponized” against women by producing deepfakes and pornographic content that use images of women’s faces that were available online without their consent (Gebru, Citation2020, p. 6). Further, AI-generated outputs represent the imbalances of power relations by centering Western epistemologies, thereby “invisibilizing other ways of being” (Bianchi et al., Citation2022, p. 3). The fact that many generative AI models are mostly trained on U.S.-based data, generated texts and images inevitably conceive “American associations, biases, values, and culture” (Heikkilä, Citation2023, para. 16); they tend to exoticize non-White ethnicities, reifying White supremacy and privileges as social norms (Bianchi et al., Citation2022).
When it comes to DALL-E, its development has been possible through the Contrastive Language–Image Pretraining (CLIP) model, which is trained on 400 million text and image pairs taken from the internet. Although the CLIP model has expedited the process of labeling image data with text with no need for manual image–text classification by human annotators, Dehouche and Dehouche (Citation2023) pointed out that CLIP is prone to reproducing skewed and unfair stereotypes present in current media culture and society because online content inevitably contains biases.
In the field of art education, researchers and educators have strived to disrupt normalized ways of being and knowing by critically examining racialized assumptions in pedagogical practice and society through the lens of social justice (Acuff & Kraehe, Citation2022; Bae-Dimitriadis, Citation2023; Carpenter et al., Citation2021; Denmead, Citation2021; Dewhurst, Citation2019; Duncum, Citation2011; Keifer-Boyd et al., Citation2007). As previously discussed, however, the content current generative AI models produce tends to amplify existing biases and reify conventional social categorization, which in turn causes further marginalization and social injustice. Particularly, engaging with AI-generated images without being aware of their biased nature can lead students to passively absorb hidden ideologies behind AI and contribute to perpetuating systemic oppression, rather than empowering them to become agents for social change. In the following sections, therefore, I discuss how to engage with such biases and stereotypes through AI imagery using the critical content analysis method, which can be an effective pedagogy art educators can employ when discussing power structures of society. After describing the six steps of critical content analysis suggested by Salvaggio (Citation2022), I introduce how I applied the method to my experimentation with DALL-E 2 to examine its biases.
Critical Content Analysis
Through critical content analysis, Salvaggio (Citation2022) aimed to examine assumptions behind text-to-image generators by understanding how to read AI-generated images. According to him, AI images are “data patterns inscribed into pictures” that tell us the “stories about that dataset and the human decisions behind it” (Intro). Reading AI images requires human viewers to examine not just the image itself but what is involved in the image’s production, including the image-generating mechanisms that are created by AI models based on prompts supplied by human users. Due to the opacity of DALL-E 2 data sets, Salvaggio (Citation2022) suggested inductively tracing the hidden biases in the data sets by analyzing the images DALL-E 2 generates through the following six steps. He stated that doing this can help cultivate literacy and fluency in critical engagement with AI-generated output.
Create a sample set: The first step is to set up a sample set by generating multiple images using the same prompt to identify patterns emerging from generated images, which can aid understanding of assumptions embedded in the data.
Content analysis: Content analysis is used to describe what one sees. Salvaggio (Citation2022) suggested identifying strong connectivity between images while looking for “certain compositions/arrangements, color schemes, lighting effects, figures or poses, or other expressive elements” (sec. 2) that are prominent in the sample set. One can focus on emerging patterns, the strengths of the data set, what is missing, and its weaknesses. If an AI system does not create solid images with given prompts, it is possible that there are not enough data in the data set. For example, if Black women’s faces are distorted or unconvincing compared to White women’s faces, it can be hypothesized that Black women are underrepresented in the data set.
Open the hood: This step is to examine what exists in the data set. As mentioned earlier, because the data set of DALL-E 2 is not publicly available, one can look at the training data for other generative AI models, such as the Large-Scale Artificial Intelligence Open Network data set for Midjourney.
Interventions: The next step is to consider the possibilities of interventions. Although sparse data can cause weakness in the generated images, another reason can be external interventions such as content filtering. OpenAI states that it strives to mitigate possible harms or biases against marginalized people by implementing content filtering and internal audits to censor offensive or explicit images (OpenAI, Citation2022). Concerning this phase, Salvaggio (Citation2022) shared an anecdote in which DALL-E 2 had different approaches to generating images of two humans kissing; when he prompted “an image of two men kissing,” it generated images accordingly, while it gave a warning flag for requesting explicit content when he replaced “men” with “women.” This contrast manifests what cultural values are incorporated in OpenAI’s content policy.
Connotative analysis: In this phase, one needs to infer the assumptions and values embedded in the data set by understanding at what the AI model is good or bad. This connotative analysis can help further analyze the social implications of generated images.
Start over: The last step is to start over, which is to confirm whether the new sample set also supports the hypothesis or theory that has been established through the initial content analysis.
As “cultural, social, economic, and political artifacts” (Intro), Salvaggio (Citation2022) emphasized that AI-generated images should be read as a map of biases. Although this method of critical content analysis cannot lead to solid conclusions on the actual biases embedded in the data set of DALL-E 2 due to its opacity, this practice can help both art educators and students develop criticality in engaging with where these images are drawn from and identifying possible gaps, erasures, and prevalence in the data set.
Experiments With DALL-E 2
Based on the method suggested by Salvaggio (Citation2022), I critically read images generated by DALL-E 2 by using racial categories as prompts. While tinkering with the prompts that include “Asian” as a starting point to represent how I racially identify myself, I found interesting outputs generated with the prompt of “Asian in visual culture.” Although I did not include either “woman” or “female” in the prompt, DALL-E 2 generated four images of Asian women wearing traditional attire. To develop a hypothesis from the initial four, I regenerated images with the same prompt and created a sample set of 16 images as suggested by Salvaggio (Citation2022). All 16 images looked quite similar ().
Figure 1. A sample set of 16 images generated by DALL-E 2 with the prompt “Asian in visual culture.”
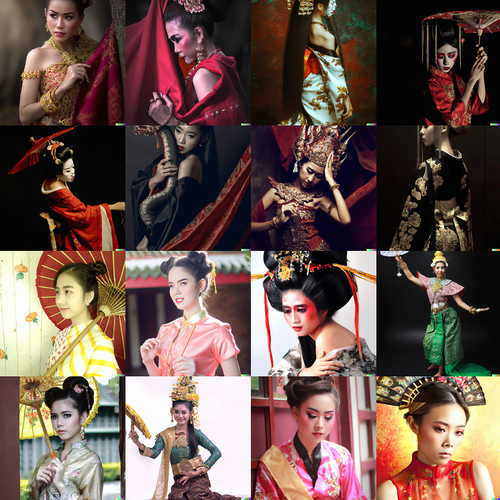
As DALL-E 2 came up with images of women only, I intentionally wrote prompts that included “woman” or “female” to see if the same patterns would emerge. Unsurprisingly, DALL-E 2 generated images of Asian women wearing traditional attire again. But this time, these women looked much more hypersexualized. Then, I turned to other races for comparative analysis; I prompted “Black woman in visual culture,” “Black female in visual culture,” “White woman in visual culture,” and “White female in visual culture” ().
Figure 2. Images generated by DALL-E 2 with prompts “Asian female in visual culture” (1st row) and “Asian woman in visual culture” (2nd row).
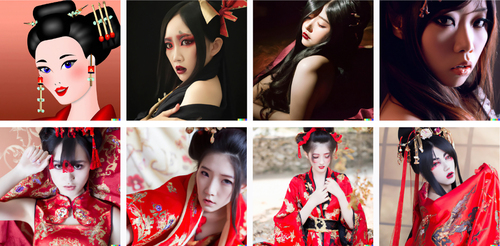
Figure 3. Images generated by DALL-E 2 with prompts “Black female in visual culture” (1st row) and “Black woman in visual culture” (2nd row).
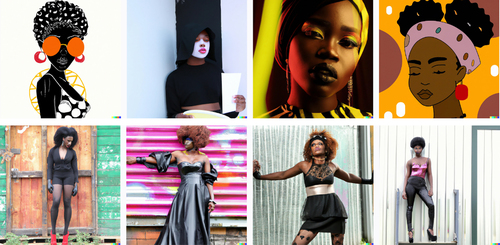
Figure 4. Images generated by DALL-E 2 with prompts “White female in visual culture” (1st row) and “White woman in visual culture” (2nd row).
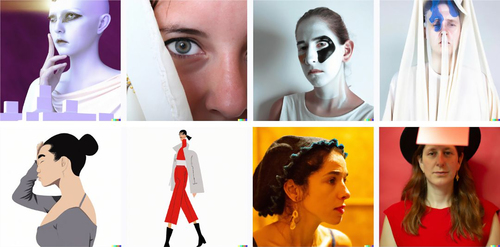
As illustrated in the images, women in different racial categories are portrayed differently, reinforcing racial stereotypes; while it is hard to identify certain patterns or connotations in the images of White women, it is apparent that Asian women and Black women are illustrated in a much more hypersexualized way. Particularly, the images of Asian women reaffirm the stereotypes against them portraying Asian women as submissive and exotic sexual objects to satisfy White males’ desires and fantasies (Cheng, Citation2018; Conrow & Gurung, Citation2021; Ramiro, Citation2022; Uchida, Citation1998). These inscriptions are embedded in a society’s visual discourse and affect how they are represented, which may be at odds with their “embodied or desired experiences” (Keifer-Boyd, Citation2003, p. 317).
One interesting finding from this experiment is that DALL-E 2 generated images of people whose faces are painted in white color when prompted “White female in visual culture.” As I wondered if DALL-E 2 interpreted “white” as a racial term or as a color, I tried different prompts including “White people,” “White people in visual culture,” and “Black people,” as well as “Black people in visual culture” as counterpart prompts. Surprisingly, while DALL-E 2 generated images of racially Black people with prompts that included “black,” it generated images of white-colored figures instead of racially White people with the word “white” ( and ).
Figure 5. A sample set of 16 images generated by DALL-E 2 with a prompt “White people in visual culture.”
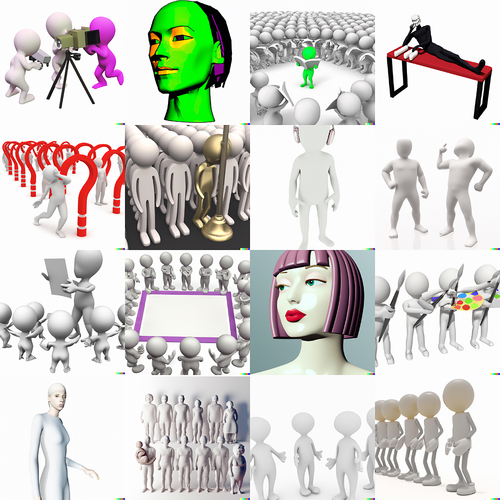
Figure 6. A sample set of 16 images generated by DALL-E 2 with a prompt “Black people in visual culture.”
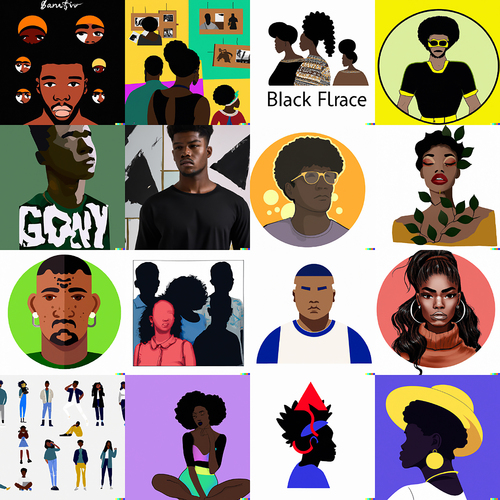
Next, I created possible scenarios about the data set of DALL-E 2 based on the method suggested by Salvaggio (Citation2022). What are the strengths and weaknesses prominent in the two sample sets? There may be sufficient data that associate the word “black” with racially Black people, while there are sparse data that associate “white” with racially White people. Then, what can we learn from this initial inference? There is a possibility that the images of racially Black people are classified with an annotation of “black,” while racially White people are classified without “white” as an annotation. Thus, I tried the prompts “people” and “people in visual culture,” and DALL-E 2 generated images of people that do not represent a certain race ().
Figure 7. Images generated by DALL-E 2 with prompts “White people” (1st row), “Black people” (2nd row), and “people” (3rd row).
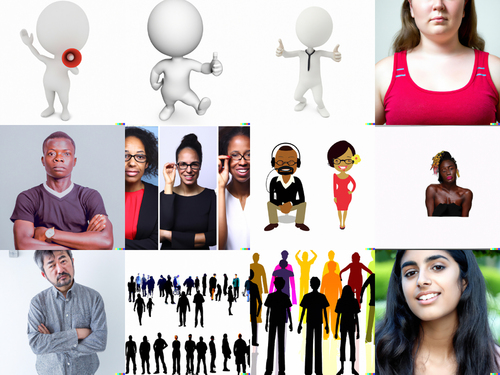
When applying the theory by Salvaggio (Citation2022) in consideration of possible external interventions, two hypotheses can be made; first, the data set consists of images that classify racially diverse people as “people”; second, the actual data set lacks racial diversity associated with the word, but the results can look diverse through content filtering. According to OpenAI, through the new technique that it implemented to “more accurately reflect the diversity of the world’s population” (OpenAI, Citation2022, para. 1) with prompts that do not describe specific race or gender, the results showed diversity 12 times.
Although both hypotheses may sound rational, it is more likely that there are not sufficient data that link “white” to racially White people, when looking at the images of white-colored figures generated by DALL-E 2 with the prompts of “white female,” “white people,” and “white people in visual culture.” In other words, it is possible that racially White people are annotated as “people” without any racially descriptive word, although the prompt “people” generates both White and non-White people through filtering. This conceived scenario manifests unequal power dynamics in the real world by supporting that Whiteness is a norm, the default state (Bolgatz, Citation2005).
Whiteness as Default
To understand why White is not interpreted as a racial concept, it is important to first examine what Whiteness is. Drawing on Rodriguez and Villaverde (Citation2000), art education researcher Wanda B. Knight (Citation2006a) stated that Whiteness has been historically and systematically deemed as colorless and “the human norm” (p. 323), which contributes to maintaining White normalcy and privileges. Also, White is expressed “in terms of ‘people’ in general” (Dyer, 1997, p. 3; as cited in Knight, Citation2006a, p. 330), which enables them not to see their color again. Therefore, Knight (Citation2006a) emphasized the importance of exposing Whiteness, which can challenge varied forms of oppression—silence, ignorance, or resistance—in U.S. society that perpetuates the status quo.
As AI systems are trained on past data, the images created by generative AI reflect the history of the art world that has been dominated by White, Western artists (Dhanesha, Citation2022). Drawing on critical race theory, Gaztambide-Fernández et al. (Citation2018) stated that the arts have been conceived as White property:
You also may have noticed that while some cultural producers are called “actors” or “composers” or “artists,” others are described as “African American artists” or “Latino composers” and wondered why the former are almost always assumed to be White.… These patterns are also true in literature. For example, you may wonder why it is that Herman Melville, Ernest Hemingway, and F. Scott Fitzgerald are labeled “American” authors, while the works of Toni Morrison, Maxine Hong Kingston, and Leslie Marmon Silko are relegated to “multicultural” literature. (pp. 1–2)
Minari, a film that was directed by Korean American writer–director Lee Isaac Chung (Citation2020), is an example that proves the unequal power relations by questioning what it means to be American. The film won a prize in the category of best foreign-language film at the Golden Globes in 2021 although it is a story about Americans. The rationale for its classification in that category is that only films with 50% or more of conversations in English can compete in the Best Motion Picture categories under the Hollywood Foreign Press Association. This understanding is at odds with the consistently increasing diversity in terms of race, language, and culture in U.S. society, which also manifests in the images generated by DALL-E 2 with the prompts, “American” and “American beauty.” All 16 images generated with each prompt mainly represent lighter skin tones while excluding non-White races ( and ).
In this sense, AI-generated images visualize how privileges and disadvantages are inscribed into culture and society through “socially loaded visual components” (Bianchi et al., Citation2022, p. 10), which reaffirm Whiteness as the “default ideal” (Bianchi et al., Citation2022, p. 4) while subordinating non-White entities. In the following section, I discuss the implications of these findings for art education by explaining how to cultivate criticality to examine hidden assumptions and biases in society through AI images.
Implications for Art Education
Engaging with an increasingly image-saturated society accelerated by digital technologies has been an important focus in visual culture art education to empower students to explore critically the complex dimensions of images (Duncum, Citation2001, Citation2002, Citation2020; Keifer-Boyd & Maitland-Gholson, Citation2007; Tavin, Citation2003). Art education researcher Paul Duncum (Citation2002) discussed that visual culture has raised the importance of engaging with visuality, “the process of attributing meaning to what we see” (p. 18). Now, we are witnessing a new arena of entangled visuality that comprises human and nonhuman imagery. As recent AI image generators contribute to constituting the current digital visual culture by generating numerous images every day, it is critical to examine what forms of visuality current AI systems are constructing.
The biases and stereotypes in AI models are difficult to mitigate through either users’ careful prompts or systematic intervention such as content filtering (Bianchi et al., Citation2022). Thus, engaging with AI-generated images through critical content analysis (Salvaggio, Citation2022) can help discuss such biases around the issues of race, gender, class, and dis/abilities, which can be applied to both K–12 and higher education settings. Art teachers can adjust the method depending on technological circumstances and their level of comfort in discussing the technological structure of generative AI. For example, art teachers can ask their students to tinker with free text-to-image generators such as Stable Diffusion, Canva, or Bing instead of DALL-E, which requires the purchase of credits for image generation. Then, students can experiment with multiple text prompts to see what visual data are released and choose one text prompt to create a sample set of 16 AI images. Art teachers will then ask students to analyze the visual data critically with the following questions:
What do you see?
What patterns do you identify?
Who/what is present or dominant? Who/what is missing or misrepresented?
How are these patterns tied to existing social norms or stereotypes in contemporary media culture?
Learning and teaching how to read AI images can serve as a critical pedagogy that is committed to advancing social justice by not only exposing invisible power structures in contemporary society, but also critiquing institutionalized oppression (Jung, Citation2015). Further, engaging with AI visuality through the lens of visual culture can be an effective way to discuss social, cultural, and political dimensions of imagery that are cogenerated by nonhuman technologies in postdigital conditions. In this sense, art educators and researchers can play an important role in empowering future generations to navigate emerging visuality and digital visual culture through their robust engagement with visual culture art education.
Conclusion
In this article, I discussed how generative AIs reproduce biases and stereotypes against certain groups of people, which can contribute to exacerbating social inequities and how critical content analysis of AI-generated images (Salvaggio, Citation2022) can be an effective art pedagogy to discuss power structures. However, I need to acknowledge that my argument may sound reasonable today, but it may not in the future as AIs are evolving into a different form every day. Even though DALL-E 2 generates images of two men kissing but gives a flag for requesting explicit content when prompted “two women kissing” at the moment of writing this article, OpenAI may change the protocol for its content policy someday. It may also adjust its content filtering technique to add racial diversity to the images of “American” or “American beauty” in the future. Therefore, new pedagogies that enable critical engagement with AI products need to be discussed further in art classrooms to empower students to understand the ways these AI technologies shape our worldviews and ways the world is operated.
Author Note
This article was written based on the visual experiments using DALL-E 2 before OpenAI presented its latest version, DALL-E 3, in late 2023.
Acknowledgments
I thank Eduardo Navas and the anonymous reviewers for their thoughtful comments.
Additional information
Notes on contributors
Ye Sul Park
Ye Sul Park, PhD Candidate, Art Education, The Pennsylvania State University in State College. Email: [email protected]
References
- Acuff, J. B., & Kraehe, A. M. (2022). Visual racial literacy: A not-so-new 21st century skill. Art Education, 75(1), 14–19. https://doi.org/10.1080/00043125.2021.1986459
- Aktay, S. (2022). The usability of images generated by artificial intelligence (AI) in education. International Technology and Education Journal, 6(2), 51–62.
- Bae-Dimitriadis, M. S. (2023). Decolonizing intervention for Asian racial justice: Advancing antiracist art inquiry through contemporary Asian immigrant art practice. Studies in Art Education, 64(2), 132–149. https://doi.org/10.1080/00393541.2023.2180310
- Berry, D. M. (2014). Critical theory and the digital. Bloomsbury.
- Bianchi, F., Kalluri, P., Durmus, E., Ladhak, F., Cheng, M., Nozza, D., Hashimoto, T., Jurafsky, D., Zou, J., & Caliskan, A. (2022). Easily accessible text-to-image generation amplifies demographic stereotypes at large scale (arXiv:2211.03759). arXiv. https://doi.org/10.48550/arXiv.2211.03759
- Birhane, A., Prabhu, V. U., & Kahembwe, E. (2021). Multimodal datasets: Misogyny, pornography, and malignant stereotypes (arXiv:2110.01963). arXiv. https://doi.org/10.48550/arXiv.2110.01963
- Bolgatz, J. (2005). Talking race in the classroom. Teachers College Press.
- Buolamwini, J., & Gebru, T. (2018). Gender shades: Intersectional accuracy disparities in commercial gender classification. In Proceedings of the 1st Conference on Fairness, Accountability and Transparency (pp. 77–91). Proceedings of Machine Learning Research. https://proceedings.mlr.press/v81/buolamwini18a.html
- Carpenter, B. S., Crabbe, K., Desai, D., Kantawala, A., Kraehe, A. M., Mask, A., & Thatte, A. (2021). The denial of racism: A call to action (Part I) [Editorial]. Art Education, 74(5), 4–8. https://doi.org/10.1080/00043125.2021.1938454
- Cetinic, E., & She, J. (2022). Understanding and creating art with AI: Review and outlook. ACM Transactions on Multimedia Computing, Communications, and Applications, 18(2), Article 66. https://doi.org/10.1145/3475799
- Cheng, A. A. (2018). Ornamentalism: A feminist theory for the yellow woman. Critical Inquiry, 44(3), 415–446. https://doi.org/10.1086/696921
- Chung, L. I. (Director). (2020). Minari [Film]. Plan B Entertainment.
- Conrow, A. R., & Gurung, R. A. R. (2021). Prejudice toward Asian American women: Clothing influences stereotypes. Psi Chi Journal of Psychological Research, 26(3), 347–358. https://doi.org/10.24839/2325-7342.JN26.3.347
- Contreras-Medina, F. R., & Marín, A. (2022). Algorithmic visuality: A social approach to machine vision in the post-internet era. Arte, Individuo y Sociedad, 34, 627. https://doi.org/10.5209/aris.74664
- Dehouche, N. (2021). Implicit stereotypes in pre-trained classifiers. IEEE Access, 9, 167936–167947. https://doi.org/10.1109/ACCESS.2021.3136898
- Dehouche, N., & Dehouche, K. (2023). What is in a text-to-image prompt: The potential of Stable Diffusion in visual arts education (arXiv:2301.01902). arXiv. https://doi.org/10.48550/arXiv.2301.01902
- Denmead, T. (2021). Time after Whiteness: Performative pedagogy and temporal subjectivities in art education. Studies in Art Education, 62(2), 130–141. https://doi.org/10.1080/00393541.2021.1896252
- Dewhurst, M. (2019). Reflecting on a paradigm of solidarity? Moving from niceness to dismantle Whiteness in art education. Journal of Cultural Research in Art Education, 36(1), 152–170. https://doi.org/10.2458/jcrae.4946
- Dhanesha, N. (2022, October 19). AI art looks way too European. Vox. https://www.vox.com/recode/23405149/ai-art-dall-e-colonialism-artificial-intelligence
- Duncum, P. (2001). Visual culture: Developments, definitions, and directions for art education. Studies in Art Education, 42(2), 101–112.
- Duncum, P. (2002). Visual culture art education: Why, what, and how. Journal of Art and Design Education, 21(1), 14–23.
- Duncum, P. (2011). Engaging public space: Art education pedagogies for social justice. Equity & Excellence in Education, 44(3), 348–363. https://doi.org/10.1080/10665684.2011.590400
- Duncum, P. (2020). Picture pedagogy: Visual culture concepts to enhance the curriculum. Bloomsbury.
- Gaztambide-Fernández, R., Kraehe, A. M., & Carpenter, B. S., II. (2018). The arts as White property: An introduction to race, racism, and the arts in education. In A. M. Kraehe, R. Gaztambide-Fernández, & B. S. Carpenter II (Eds.), The Palgrave handbook of race and the arts in education (pp. 31). Palgrave Macmillan. https://doi.org/10.1007/978-3-319-65256-6_1
- Gebru, T. (2020). Race and gender. In M. D. Dubber, F. Pasquale, & S. Das (Eds.), The Oxford handbook of ethics of AI (pp. 252–269). Oxford University Press. https://doi.org/10.1093/oxfordhb/9780190067397.013.16
- Heikkilä, M. (2022, December 12). The viral AI avatar app Lensa undressed me—Without my consent. MIT Technology Review. https://www.technologyreview.com/2022/12/12/1064751/the-viral-ai-avatar-app-lensa-undressed-me-without-my-consent
- Heikkilä, M. (2023, March 22). These new tools let you see for yourself how biased AI image models are. MIT Technology Review. https://www.technologyreview.com/2023/03/22/1070167/these-news-tool-let-you-see-for-yourself-how-biased-ai-image-models-are
- Johnson, K. (2022, May 5). DALL-E 2 creates incredible images—And biased ones you don’t see. WIRED. https://www.wired.com/story/dall-e-2-ai-text-image-bias-social-media
- Jung, Y. (2015). Post stereotypes: Deconstructing racial assumptions and biases through visual culture and confrontational pedagogy. Studies in Art Education, 56(3), 214–227. https://doi.org/10.1080/00393541.2015.11518964
- Keifer-Boyd, K. (2003). A pedagogy to expose and critique gendered cultural stereotypes embedded in art interpretations. Studies in Art Education, 44(4), 315–334. https://doi.org/10.1080/00393541.2003.11651748
- Keifer-Boyd, K., Amburgy, P. M., & Knight, W. B. (2007). Unpacking privilege: Memory, culture, gender, race, and power in visual culture. Art Education, 60(3), 19–24. https://doi.org/10.1080/00043125.2007.11651640
- Keifer-Boyd, K., & Maitland-Gholson, J. (2007). Engaging visual culture. Davis.
- Knight, W. B. (2006a). E(Raced) bodies in and out of sight/cite/site. Journal of Social Theory in Art Education, 26, 323–345. https://scholarscompass.vcu.edu/jstae/vol26/iss1/20
- Knight, W. B. (2006b). Using contemporary art to challenge cultural values, beliefs, and assumptions. Art Education, 59(4), 39–45. https://doi.org/10.1080/00043125.2006.11651602
- Knox, H. (2021). Traversing the infrastructures of digital life. In H. Geismar & H. Knox (Eds.), Digital anthropology (2nd ed.; pp. 178–196). Routledge.
- Manovich, L. (2017). Automating aesthetics: Artificial intelligence and image culture. Flash Art International, 316, 1–10.
- O’Neil, C. (2016). Weapons of math destruction: How big data increases inequality and threatens democracy. Crown.
- OpenAI. (2022, July 18). Reducing bias and improving safety in DALL·E 2. OpenAI Blog. https://openai.com/blog/reducing-bias-and-improving-safety-in-dall-e-2
- Ramiro, C. (2022). After Atlanta: Revisiting the legal system’s deadly stereotypes of Asian American women. Asian American Law Journal, 29, 90–125.
- Rodriguez, N. M., & Villaverde, L. E. (Eds.). (2000). Dismantling White privilege: Pedagogy, politics, and Whiteness. Peter Lang. https://www.peterlang.com/document/1090821
- Routhier, D., Lee-Morrison, L., & Maurer, K. (2022). Automating visuality: An introduction. Journal of Media Art Study and Theory, 3(1), 2–14.
- Salvaggio, E. (2022, October 2). How to read an AI image. Cybernetic Forests. https://cyberneticforests.substack.com/p/how-to-read-an-ai-image
- Sweeny, R. W. (2005). Three funerals and a wedding: Art education, digital images, and an aesthetics of cloning. Visual Arts Research, 31(1), 26–37.
- Tavin, K. M. (2003). Wrestling with angels, searching for ghosts: Toward a critical pedagogy of visual culture. Studies in Art Education, 44(3), 197–213. https://doi.org/10.1080/00393541.2003.11651739
- Uchida, A. (1998). The Orientalization of Asian women in America. Women’s Studies International Forum, 21(2), 161–174. https://doi.org/10.1016/S0277-5395(98)00004-1
- Vartiainen, H., & Tedre, M. (2023). Using artificial intelligence in craft education: Crafting with text-to-image generative models. Digital Creativity, 34(3), 1–21. https://doi.org/10.1080/14626268.2023.2174557