ABSTRACT
Capsule: Nest-site selection of Barbary Partridge Alectoris barbara in a Moroccan agroforestry system is dictated by nest scale rather than landscape-scale factors.
Aims: To identify the most important factors affecting Barbary Partridge nest habitat selection in a Moroccan agroforestry landscape.
Methods: I investigated the effect of two spatial scales (nest-site and landscape) on the probability of presence of nests. Topography, habitat structure, human disturbance and land-use parameters were measured at nests (n = 64) and random points (n = 64) within landscape plots (50 m radius) and nest-site plots (5 m).
Results: At the nest scale, quadratic shrub cover and proximity to trees best explained nest habitat use. At the landscape scale, quadratic forest cover and linear distance to the nearest track positively influenced the nest presence probability. The most relevant scale was nest-scale followed by landscape scale. The variation partitioning analysis confirmed this spatial pattern.
Conclusion: This approach was important to: (i) understand nest habitat use in the Barbary Partridge at nest and landscape scales, and (ii) highlight the most important variables driving habitat use in a Mediterranean agroforestry system. From a practical perspective, it is recommended to extend the same methodological approach to other Mediterranean agroforestry systems to find out if the species follows the same nest habitat selection pattern.
For wild vertebrate species, the validity of multi-scale habitat selection modelling has been well established (Jedlikowski et al. Citation2016, McGarigal et al. Citation2016, Jedlikowski & Brambilla Citation2017). Understanding and defining species’ needs in terms of nesting habitats are of great importance to establish appropriate management actions and to ensure the delivery of sustainable species conservation. This need is even more important when it comes to vulnerable species or game species. For instance, hunters’ preference for some bird species further complicates their management and, ultimately, their conservation. In such conditions, determining the drivers affecting nesting habitat selection as accurately as possible is essential, but this accuracy cannot be reached without taking into account a multi-scale approach. Recently, several studies (Cabeza et al. Citation2010, Razgour et al. Citation2011, Jedlikowski et al. Citation2016) have applied modelling at multiple-scales to address hierarchical conservation needs. This approach has been used to select processes related to the choice of breeding sites (Bonnot et al. Citation2009, Crampton & Sedinger Citation2011, Jedlikowski et al. Citation2016) especially for species with unfavourable conservation status (Leopold & Hess Citation2013, Pérez-Granados et al. Citation2017) but also for game bird species (Zimmermann et al. Citation2009, Doherty et al. Citation2010, Mehls et al. Citation2014).
The Barbary Partridge Alectoris barbara is undoubtedly the major game species in Morocco and is highly valued by the nation’s hunters. In North Africa, the species is known to be a common, sedentary breeding bird (Isenmann & Moali Citation2000, Thévenot et al. Citation2003, Isenmann et al. Citation2005) that is distributed from the Canary Islands to northeast Egypt (Foronda et al. Citation2005). Its population is in decline, with potential drivers being: poaching (Alaoui Citation2001), hunting pressure (Madge & McGowan Citation2002, BirdLife International Citation2004), habitat degradation (Alaoui Citation2001, Madge & McGowan Citation2002, BirdLife International Citation2004) and predation (Alaoui Citation2001). The Barbary Partridge has a large ecological amplitude; it is widely distributed both in forests and in agricultural regions (Maghnouj Citation1983, Alaoui Citation2001).
In North Africa, the majority of previous studies on Barbary Partridge nesting habitat have been limited to general descriptions of habitat use (Maghnouj Citation1983, Alaoui Citation1992, Akil & Boudedja Citation1996, Akil Citation1998, Alaoui Citation2001, Boukrabouza Citation2011, Mezerdi Citation2011, Citation2015). Yet, to understand ecological requirements of selecting habitat a more thorough approach is needed. Indeed, ecological modelling techniques are a rapidly developing area used to study habitat selection at both broad-scale and fine-scale patterns (Fernandez et al. Citation2003). Such techniques commonly use multi-scale dimensions for habitat selection assessment in birds (Zimmermann et al. Citation2009, Saalfeld et al. Citation2010, Pickens & King Citation2014), including those processes related to the choice of breeding sites (Bonnot et al. Citation2009, Crampton & Sedinger Citation2011, Jedlikowski et al. Citation2016, Hanane Citation2018). Overall, this will allow guiding future conservation actions as well as the adoption of appropriate species management (Assandri et al. Citation2017, Fogarty et al. Citation2017, Hanane Citation2018).
The choice of this species as a model system has been dictated by two reasons: first, there is no previous study focusing on multi-scale nest habitat selection for this game species throughout North Africa using powerful statistical approaches, although there is an urgent need for it. Second, the Barbary Partridge suffers fair amounts of disturbance (e.g. from hunting, poaching, egg harvesting), which imposes a demanding nest-site decision-making process based on prevailing abiotic and biotic conditions.
The main objective of this study was to identify the most important factors affecting Barbary Partridge nest habitat selection in a North African agroforestry landscape. This question was first investigated by considering local and landscape scales separately, and afterwards jointly, to determine at what operative scale the species selects its nesting habitat.
Methods
Study area
Observations were made in Morocco, in an agroforestry system (15 736 ha) located within Oued Mellah watershed in the vicinity of Tamesna dam and Ben Ahmed city (). The area has a semi-arid climate, with annual average rainfall of 320 mm, most of which falls during the winter season (November–January) (Hanane & Yassin Citation2017). Temperatures vary widely, being more moderate during the winter, and hot in summer with occasional peaks of 42°C. Altitude ranges from 331 to 663 m above sea level (Hanane & Yassin Citation2017, Hanane Citation2018, Hanane et al. Citation2018).
Figure 1. Map showing the location of the study area and positions of grid cells sampled in 2016 (black squares) and 2017 (white squares).
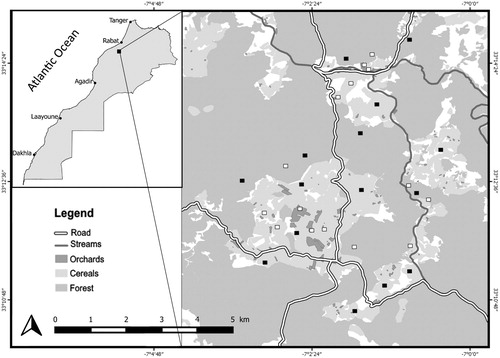
The predominant agroforestry landscape is mainly composed of natural Thuya forests (5599 ha); agricultural land including olive plantations (2182 ha), and waterbeds (38.9 km; Laâtech, Laâouija and Zamerine). In natural forests, the tree-layer consists mainly of Thuya Tetraclinis articulata in association with Wild Olive Olea europaea oleaster, Lentisk Pistacia lentiscus and Tizra trees Rhus pentaphylla; olive plantations were monoculture stands of olive trees almost uniformly separated (5–10 m between trees) and were located most often in a cereal platform dominated by Wheat Triticum turgidum and Triticum aestivum and Barley Hordeum vulgare (Hanane & Yassin Citation2017, Hanane Citation2018, Hanane et al. Citation2018). The shrub layer was generally absent from this landscape whereas the herbaceous layer was ephemeral in most orchards.
Human density was low, with small single-family homes at an average (± sd) distance of 2.10 ± 0.85 km, surrounded by cereal crops and forests. In the study area, there was no human habitation inside the forest area (HCEFLCD Citation2006, Hanane & Yassin Citation2017, Hanane Citation2018, Hanane et al. Citation2018).
Data collection
Monitoring took place from mid-April to the end of June, during the breeding season, in 2016 and 2017. This period was chosen because most pairs were nesting at this time (Thévenot et al. Citation2003). To increase the chances of finding the nests of this highly secretive species, two field approaches were combined: (1) Sampling: A grid with square 100 × 100 m cells (10 000 m2 areas) was superimposed over a map of the study site (Hanane & Yassin Citation2017) and each grid cell was assigned a unique numerical identifier. Each year, 15 cells were randomly chosen using a random number generator (). In each cell, I systematically searched for all nests of the species by performing, from one end to the other, movements back and forth. This method has the advantage of allowing almost complete coverage of each cell. During these back-and-forth movements, I recorded all signs of partridges’ presence (e.g. droppings, frequently used passages to enter the bushy vegetation, hissing of males). Once localized, further and deeper research within these areas allows finding of nests. (2) Contribution of field collaborators: By their distribution in almost all the study area and their daily presence respectively at cereal fields and forests, land owners, wheat harvesters and shepherds constituted an additional source of information allowing the localisation of nests in the 15 grid cells.
To compare habitat characteristics, the same number of non-nest points (n = 28 in 2016 and 36 in 2017) and nests (n = 28 in 2016 and 36 in 2017) was randomly selected to give a balanced design. For each year and at each sampled grid cell, the number of random points was thus equal to that of nest points. Random points were selected, using the QGIS random selection tool (Quantum GIS Development Team Citation2017) after excluding all points at which Barbary Partridges were recorded.
Sixteen environmental variables related to topography, human influence and land use were selected () because they could potentially influence the presence of Barbary Partridge nests. At nest-scale (5 m radius plot centred on nests), all measures were collected in the field by the same investigator (S. Hanane), while those regarding the landscape scale (50 m radius plot centred on nests) were all performed using QGIS except for elevation. A clinometer (± 0.05 m) was used to measure the distance from nest to the nearest tree (m) and the maximum height of shrub cover (m).
Table 1. Description of habitat variables measured around survey points (nest-site and random points) within two spatial levels (local- and landscape scales) and used in the analyses of Barbary Partridge nest habitat selection model.
Surveys began 1 h after sunrise (minimum value) and lasted until 1 h before sunset (maximum value) under favourable weather conditions (no rain and low wind). The location and elevation of each nest point were referenced using a portable global positioning system (GPS) device (Magellan eXplorist XL), and then reported using Quantum QGIS 2.18.14. At the landscape scale, elevation was measured at four points randomly distributed on the surface of each plot of 50 m radius and then averaged.
Radius selection for the landscape scale
To breed, Barbary Partridges are known to use habitats close to open agricultural areas, located within forest edges and forests dotted with clearings (Maghnouj Citation1983, Alaoui Citation2001). I considered the entire mosaic (agricultural and forest habitats) to understand the habitat requirements of this partridge during the breeding season. For this, I used the spatial analyst tool in QSIG to map land cover around each nest-site and random points within four landscape levels (50, 100, 150, and 200 m radii surrounding each survey point). To select the radius values for the landscape variables, I treated the sets of variables belonging to the different radii as competing sets of variables and selected the radius leading to the most parsimonious models according to Akaike’s information criteria corrected for small sample size (AICc; Jedlikowski et al. Citation2016, Jedlikowski & Brambilla Citation2017).
Statistical analysis
The data collected were used to check for possible correlations among variables by using Pearson’s standard correlation index. Regression models are generally assumed to be robust to multicollinearity if the Pearson’s standard correlation between variables is less than r = 0.6 (Mertler & Vannatta Citation2002), so any such correlated variables were excluded from contributing to the same models.
To test the effect of covariates on Barbary Partridge nest presence, I used generalized linear models (GLM) with binomial error (logistic regression). All the covariates were considered linearly, log-transformed (in order to detect any plateau) or quadratic (in order to detect any optimum). I developed an all-inclusive design (all possible combination models) by using multimodel inference (Burnham & Anderson Citation2002). Models were then ordered by increasing AICc value (Burnham & Anderson Citation2002). We considered all models with ΔAICc lower than 2 as equally good (Burnham & Anderson Citation2002). Model weights were used to define the relative importance of each explanatory variable across the full set of models evaluated by summing weight values of all models that included the explanatory variable of interest. I tested the model performance by the percentage of correct classifications of original cases, Nagelkerke’s R2, the receiver operating characteristic (ROC) curve analysis (AUC values > 0.7 indicate high model accuracy or an acceptable discrimination; Swets Citation1988), and the Hosmer–Lemeshow Goodness of Fit Test (Hosmer & Lemeshow Citation2000).
The spatial structure of nest location of Barbary Partridges was quantified by the indicator semivariogram (Goovaerts Citation1998) by using the residual of the best model in terms of AICc value. Nugget to total sill ratio (NSR) was expressed as the percentage of total semivariance and was used to define spatial dependency according to the criteria established by Cambardella et al. (Citation1994).
This GLM approach was applied in three levels: (1) nest-level variables, (2) landscape-scale variables, and (3) combined nest-level and landscape-scale variables, where the most relevant factors from the two different scales (i.e. nest and landscape scales) were included in the analysis.
I also carried out a variation partitioning analysis to evaluate the specific contribution of each individual spatial level in the habitat selection by Barbary Partridge.
All statistical analyses were performed in R-3.0.2 software (R Development Core Team Citation2013). I used the package MuMIn to calculate AICc (Bartoń Citation2015), the package fmsb to obtain Nagelkerke’s R2, the package ResourceSelection to test the best model performance, the package ROCR to calculate AUC (Sing et al., Citation2005), the package vegan (Oksanen et al., Citation2013) to carry out the variation partitioning analysis. I used packages sp, lattice and gstat to draw semivariograms (Pebesma Citation2006) and package visreg (Breheny & Burchett Citation2012) to plot the relationship between the nest presence probability and explanatory variables selected in the best models. Means are quoted ± standard errors.
Results
A total of 64 Barbary Partridge nests (n = 28 for 2016, n = 36 for 2017) were found in the sampling square cells. Of these, 25 nests (39.1%) were located in cereals, 24 (37.5%) in forests, 8 (12.5%) in permanent crops and 7 (10.9%) in waterbed vegetation. The average characteristics of those nests, as well as the random points, are summarized in .
Table 2. Descriptive statistics for variables (mean ± se) measured at Barbary Partridge nests and random points using t tests. Wilks lambda = 0.580; F = 4.759; P < 0.001 (Multivariate analysis of variance).
Selection of the landscape scale
The GLM analysis highlighted a lowest value of AICc for the 50 m radius (155.65; AICc null model = 170.87), followed by those of 100, 150, and 200 m respectively. Values for ΔAICc were greater than 2 for the 100 (2.16), 150 (2.34) and 200 m radii (2.65). Based on these analyses, the 50 m radius was selected for the landscape variables.
Habitat selection at nest and landscape scales
The locations of all 64 Barbary Partridge nests were used to evaluate nest-site selection models at both scales. At nest-scale, the probability of presence of Barbary Partridge nests varied with shrub cover, following a quadratic relationship ( & , (b)). This probability increased with increasing shrub cover, reached an optimum around 80% and then decreased. Nest presence probability was also negatively associated with distance from nest to the nearest tree, with a higher occurrence around 1 m from the nearest tree (, (d)). Nagelkerke’s R2 (0.443) and the AUC score (0.836) indicated a good performance for the best model. The Hosmer–Lemeshow Goodness of Fit Test (χ2 = 9.618, df = 8, P = 0.293) indicated a very good model fit. At this scale, there was no evidence of spatial autocorrelation in the model’s residuals (NSR = 1.00).
Figure 2. Probability of presence of Barbary Partridge nests according to: (a) forest cover, (b) shrub cover, (c) distance to the nearest track and (d) to nearest tree, in an agroforestry system of Central Morocco. The shaded grey areas are the lower and upper 95% confidence limits.
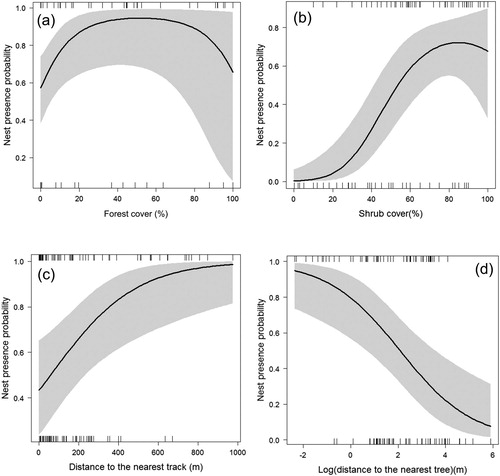
Table 3. Summary of the GLMs describing Barbary Partridge nest habitat selection at local- and landscape scales. Variable abbreviations are explained in .
Table 4. Estimates of the best models fitted for Barbary Partridge nest habitat selection at local- and landscape scales using binomial error and logistic link function. RVI = Relative Variable Importance. Variable abbreviations are explained in .
At the landscape scale, Barbary Partridges would select an optimum of forest cover ranging from 35% to 65% to build their nest ( & , (a)). The probability of nest presence was also positively correlated with the distance to the nearest track (, (c)). Nagelkerke’s R2 (0.236) and AUC score (0.746) indicated a less good performance compared to that of nest-site scale. The Hosmer–Lemeshow Goodness of Fit Test (χ2 = 6.728, df = 8, P = 0.566) indicated a very good model fit. As with the fine scale, there was no evidence of spatial autocorrelation in the model residuals (NSR = 1.00) at the landscape scale.
Multi-scale models
Compared to the top-ranked single-scale models, multilevel models were more parsimonious (). Nagelkerke’s R2 values of the multi-scale models were equal to 0.582 (considering the most parsimonious model). The Hosmer–Lemeshow Goodness of Fit Test (χ2 = 12.826, df = 8, P = 0.118) indicated a very good model fit. AUC score (0.894) indicated a good performance for this model. The most important variables determining the occurrence of Barbary Partridge nests were the logarithm of the distance from nest to the nearest tree (nest-site level; (d)), quadratic shrub cover (nest-site level; (b)), quadratic forest cover (landscape level; (a)), and linear distance to the nearest track (landscape level) ((c); & ). There was no evidence of spatial autocorrelation in the model residuals (NSR = 1.00), suggesting that the results of this non-spatial GLM model were not biased by possible spatial covariance in the data.
Relative contribution of the two scales
The explanatory power of the single-scale models suggested that the most relevant scale to explain nest placement in the Barbary Partridge was the nest-site level, followed to a much lesser degree by landscape scale (). The two spatial scales together explained 48% of the variance of the nest habitat selection, leaving 52% of the variation data attributed to unidentified factors. Variation partitioning analysis showed that the nest-site scale is relevant (36%), while the pure effect of landscape is negligible (6%), as well as that of the joint effect (6%).
Discussion
Outside North Africa, the only known studies on Barbary Partridges were conducted in the Canary Islands (Foronda et al. Citation2005) and Sardinia (Scandura et al. Citation2010, Spano et al. Citation2013). None of these studies have, however, dealt with the breeding ecology.
In the present study, I investigated the effects of local and landscape variables on the presence of Barbary Partridge nests in a North African agroforestry landscape. My results revealed that the probability of a site being selected for nesting by Barbary Partridges is negatively influenced by distance to the nearest tree, positively associated with distance to the nearest track, and positively affected by percentage cover of shrubs until an optimum of around 80% and by forest cover until an optimum of between 35% and 65%.
At the local scale (0.00785 ha), the modelling approach shows that Barbary Partridges select to nest in close proximity to a tree and in areas with higher per cent shrub cover vegetation. This choice would reflect the importance of utmost nest concealment in this species. Abundant cover of herbaceous and woody vegetation would constitute an effective strategy to hide from predators, including humans. Such behaviour is known to be common in this North African partridge species (Maghnouj Citation1983, Alaoui Citation2001, Madge & McGowan Citation2002) and also in other partridge species such as the Chukar Partridge Alectoris chukar (Rands Citation1988), Pyrenean Grey Partridge Perdix perdix hispaniensis (Panek & Kamieniarz Citation2000, Novoa et al. Citation2002), Red-legged Partridge Alectoris rufa (Ricci et al. Citation1990, Casas & Viñula Citation2010) and Tibetan Partridge Perdix hodgsoniae (Dorge et al. Citation2014). Often, breeding partridges chose nest positions so that the nearest tree was situated behind the sitting adult. This behaviour would be a way to further improve the level of camouflage, and a measure to avoid potential predators that might approach from behind. An alternative explanation for such behaviour may also be maintenance of a tolerable temperature through the presence of shade, although this needs further investigation. Nesting in close proximity to a tree has been also reported in the Green-legged Partridge Arborophila chloropus (Ong-in et al. Citation2016).
At the landscape scale (0.785 ha), forest cover and distance to the nearest track were the best predictors of the probability of nest presence. In this agroforestry system, the Barbary Partridge places its nest further from tracks. Noises generated by vehicles, especially trucks, are likely to disturb partridges in this area as it was demonstrated for another bird species in North African forests, the Woodpigeon Columba palumbus (Hanane et al. Citation2012). A possible reason for this behaviour could also be the absence of vegetation along tracks. Indeed, the presence of such vegetation has, for instance, allowed Grey Partridges P. perdix to nest in close proximity to paths or roads in French cereal agrosystems (Reitz et al. Citation2002). This vegetation also has the advantage of reducing predation risk for the nesting partridges. Its absence, therefore, makes the areas around tracks almost unfavourable for breeding.
Furthermore, at this scale, the probability of Barbary Partridge nest presence is also associated with an optimum percentage of forest ranging between 35% and 65%. Consequently, a sparse or a very dense forest decreases the probability of nesting in this species. This result provides information on the importance of the presence of forests around nests (within a 50 m radius). Such presence could be interpreted as an anti-disturbance strategy that consists, in this case, of having a wooded area close-by in which partridges could protect themselves from human presence, mostly at the time of harvesting cereals, and from aerial predators (e.g. from Long-legged Buzzard Buteo rufinus and Booted Eagle Aquila pennata). Forest cover of between 35% and 65% is also important to avoid excessive heat, mainly towards the end of the breeding season at the end of June and in July.
Interestingly, our analyses have not highlighted a relevant role for the proximity of water points in Barbary Partridge nest habitat selection in this semi-arid agroforestry landscape. This can be explained by a good availability of this resource in the study area, with the presence of natural and artificial water sources. Also, the effect of year was not relevant either as reported by Bro et al. (Citation2000) when studying nest-site selection of Grey partridge P. perdix.
Our results suggest that the local scale model was more parsimonious compared to the landscape model. This emphasizes the fact that the landscape-scale model gives incomplete views of the nest-site selection process in this agroforestry system. The importance of the nest-scale might result from the fact that Barbary Partridges rather prioritize suitable nest habitat that provides good security, such as high shrub cover and close proximity to the nearest tree. Although the landscape scale is interesting for security reasons too (high forest cover and high distance to the nearest track), its effect remains nonetheless weak; this indicates that nest habitat selection process in the Barbary Partridge occurs within a small scale.
The investigation of habitat–animal associations relies on a manager’s ability to understand the scale to which wildlife responds to, and interacts with, the environment (Pastor et al. Citation1996, Guerena et al. Citation2014, Hanane & Magri Citation2017). A management plan based on good forest practice is necessary to ensure favourable conditions in terms of lack of disturbance and availability of nesting sites. My model suggested that Barbary Partridges rather prioritize nest-scale by using high shrub cover and low distance to the closest tree. Human activities, especially grazing of livestock, affect the structure of shrubs in the North African forests (Cherkaoui et al. Citation2009, Hanane et al. Citation2012, Hanane & Besnard Citation2013, Hanane, Citation2014) which in turn impact the selection of nest-sites by Barbary Partridges. Thus, I recommend introducing a rotating system of forest utilization for grazing which would promote greater availability of nesting sites for Barbary Partridges while ensuring the development of shrubs. Human activity also consists of the presence of wheat harvesters at the peak of breeding activity of Barbary Partridge (i.e. in May), which consequently influences nesting success. Management bodies can act by establishing an awareness programme for cereal harvesters in this agroforestry system. Such a programme, once undertaken, would undoubtedly improve the nesting conditions of this species in the study area.
Although having a weak effect, it would also be beneficial to pay attention to what happens at the landscape scale. The species would benefit from management measures that set restrictions, where possible, and control access of vehicles.
In summary, this study investigated nest habitat selection in the Barbary Partridge at two spatial scales. This approach was important: (i) to understand nest habitat use in the Barbary Partridge at nest and landscape scales, and (ii) to highlight the most important variables driving this use in a Mediterranean agroforestry system. However, it would be important to extend the same analytical approach to other Mediterranean agroforestry systems to find out if this species follows a similar nest habitat selection pattern. This would provide guidance for future conservation actions and appropriate habitat management for this species.
Acknowledgements
I thank Abderrahim and Mustapha for helping during the field work and N. Magri for helpful in realizing the map. I also thank two anonymous reviewers and the Editor of Bird Study for their comments and advice.
ORCID
Saâd Hanane http://orcid.org/0000-0003-2008-1728
Additional information
Funding
References
- Akil, M. 1998. Dynamique des populations de la Perdrix Gambra (Alectoris barbara) dans la région de Yakouren (Algérie). Mémoire de Magister. Inst. Sci. Nat., Univ, Tizi-ouzou, 67 pp.
- Akil, M. & Boudedja, S. 1996. La Perdrix gambra (Alectoris barbara) Rev. La Fôret. Algérienne. Inst. Nat. For. 1: 31–36.
- Alaoui, M.Y. 1992. Écologie de la ponte chez la Perdrix Gambra (Alectoris barbara) au Maroc. Gibier Faune Sauvage, 9: 405–415.
- Alaoui, M.Y. 2001. Mise en évidence d’une race de montagne de Perdrix Gambra (Alectoris barbara) dans le haut-atlas marocain. Game Wildl. Sci. 18: 451–457.
- Assandri, G., Giacomazzo, M., Brambilla, M., Griggio, M. & Pedrini, P. 2017. Nest density, nest-site selection, and breeding success of birds in vineyards: management implications for conservation in a highly intensive farming system. Biol. Conserv. 205: 23–33. doi: 10.1016/j.biocon.2016.11.020
- Bartoń, K. 2015. MuMIn: multi-model inference. R package version 1.9.13. Available at http://CRAN.R-project.org/package=MuMIn.
- BirdLife International 2004. Birds in Europe: population estimates, trends and conservation status. BirdLife International, Cambridge.
- Bonnot, T.W., Millspaugh, J.J. & Rumble M.A. 2009. Multi-scale nest-site selection by black-backed woodpeckers in outbreaks of mountain pine beetles. For. Ecol. Manag. 259: 220–228. doi: 10.1016/j.foreco.2009.10.021
- Boukrabouza, A. 2011. Connaissance, conservation et gestion des populations de la Perdrix Gambra (Alectoris barbara, Bonnaterre, 1792) au niveau de la réserve de chasse de Zéralda, 69. Mémoire PGS. Université S.T.H.B, Zéralda.
- Breheny, P. & Burchett, W. 2012. Visualizing regression models using visreg. http://web.as.uky.edu/statistics/users/pbreheny/publications/visreg.pdf.
- Bro, E., Reitz, F. & Clobert, J. 2000. Nest-site selection of Grey partridge (Perdix perdix) on agricultural lands in North-Central France. Game and Wildlife Science 17: 1–16.
- Burnham, K.P. & Anderson, D.R. 2002. Model Selection and Multimodel Inference: a practical information theoretic approach. Springer, New York, NY.
- Cabeza, M., Arponen, A., Jäättelä, L., Kujala, H., van Teeffelen, A. & Hanski, I. 2010. Conservation planning with insects at three different spatial scales. Ecography 33: 54–63. doi: 10.1111/j.1600-0587.2009.06040.x
- Cambardella, C.A., Moorman, T.V., Novak, J.M., Parkin, T.B., Karlen, D.L., Turco, R.F. & Konopka, A.E. (1994). Field-scale variability of soil properties in Central Iowa soils. Soil Sci. Soc. Am. J. 58: 1501–1511. doi: 10.2136/sssaj1994.03615995005800050033x
- Casas, F. & Viñuela, J. 2010. Agricultural practices or game management: which is the key to improve red-legged partridge nesting success in agricultural landscapes? Environ. Conserv. 37: 177–186. doi: 10.1017/S0376892910000299
- Cherkaoui, I., Selmi, S., Boukhriss, J., Hamid, R.I. & Dakki, M. 2009. Factors affecting bird richness in a fragmented cork oak forest in Morocco. Acta Oecol. 35: 197–205. doi: 10.1016/j.actao.2008.10.002
- Crampton, L.H. & Sedinger, J.S. 2011. Nest-habitat selection by the Phainopepla: congruence across spatial scales but not habitat types. Condor 113: 209–222. doi: 10.1525/cond.2011.090206
- Doherty, K.E., Naugle, D.E. & Walker, B.L. 2010. Greater sage-grouse nesting habitat: the importance of managing at multiple scales. J. Wildl. Manage. 74: 1544–1553. doi: 10.1111/j.1937-2817.2010.tb01282.x
- Dorge, T., Högstedt, G. & Lislevand, T. 2014. Nest distribution and nest habitat of the Tibetan Partridge (Perdix hodgsoniae) near Lhasa, Tibet. Avian Res 5: 5. doi: 10.1186/s40657-014-0005-7
- Fernandez, N., Delibes, M., Palomares, F. & Mladenoff, D.J. 2003. Identifying breeding habitat for the Iberian lynx: inferences from a fine-scale spatial analysis. Ecol. Appl. 13: 1310–1324.
- Fogarty, D.T., Elmore, R.D., Fuhlendorf, S.D. & Loss, S.R. (2017). Influence of olfactory and visual cover on nest site selection and nest success for grassland-nesting birds. Ecol. Evol. 7: 6247–6258. doi: 10.1002/ece3.3195
- Foronda, P., Casanova, J., Figueruelo, E., Abreu, N. & Feliu, C. 2005. The helminth fauna of the Barbary partridge Alectoris barbara in Tenerife, Canary Islands. J. Helminthol. 79: 133–138. doi: 10.1079/JOH2005277
- Goovaerts, P. 1998. Geostatistical tools for characterizing the spatial variability of microbiological and physicochemical soil properties. Biol. Fert. Soils 27: 315–334. doi: 10.1007/s003740050439
- Guerena, K.B., Castelli, P.M., Nichols, T.C. & Williams, C.K. 2014. Spatially-explicit land use effects on nesting of Atlantic Flyway resident Canada geese in New Jersey. Wildlife. Biol. 20: 115–121. doi: 10.2981/wlb.13005
- Hanane, S. 2014. Effects of human disturbance on nest placement of the woodpigeon (Columba palumbus): a case study from the Middle Atlas, Morocco. Integr. Zool. 9: 349–359. doi: 10.1111/1749-4877.12078
- Hanane, S. 2018. Multi-scale turtle dove nest habitat selection in a Mediterranean agroforestry landscape: implications for the conservation of a vulnerable species. Eur. J. Wildl. Res. 64: 45. doi:10.1007/s10344-018-1205-y.
- Hanane, S. & Besnard, A. 2013. Nest survival of Woodpigeons (Columba palumbus) in North African forests. Bird Study 60: 202–210. doi: 10.1080/00063657.2013.766879
- Hanane, S. & Magri, N. 2017. Towards understanding habitat use patterns of an endangered galliform subspecies. Pternistis bicalcaratus ayesha: implications for conservation. Ostrich 88: 217–224. doi: 10.2989/00306525.2017.1287134
- Hanane, S. & Yassin, M. 2017. Nest-niche differentiation in two sympatric columbid species from a Mediterranean Tetraclinis woodland: Considerations for forest management. Acta Oecol. 78: 47–52. doi: 10.1016/j.actao.2016.12.003
- Hanane, S., Besnard, A. & Aafi, A. 2012. Factors affecting reproduction of Woodpigeons Columba palumbus in North African forests: 1. Nest habitat selection. Bird Study 59: 463–473. doi: 10.1080/00063657.2012.724050
- Hanane, S., Cherkaoui S.I., Magri, N. & Yassin, M. 2018. Bird species richness in artificial plantations and natural forests in a North African agroforestry system: assessment and implications. Agroforest. Syst. doi:10.1007/s10457-018-0281-z.
- Haut-Commissariat aux Eaux et Forêts et à la Lutte Contre la Désertification (HCEFLCD) 2006. SAPROF for Watershed Management Project in the Kingdom of Morocco, 385. Final Report. Japan Bank for International Cooperation.
- Hosmer D.W. & Lemeshow S. 2000. Applied Logistic Regression, 2nd ed. Wiley, New York.
- Isenmann, P. & Moali, A. 2000. Les Oiseaux d’Algérie–Birds of Algeria. Société d’Etude Ornithologiques de France, Paris.
- Isenmann, P., Gaultier, T., El Hili, A., Azafzaf, H., Dlensi, H. & Smart, M. 2005. Oiseaux de Tunisie. Birds of Tunisia. SEOF Editions, Paris.
- Jedlikowski, J. & Brambilla, M. 2017. The adaptive value of habitat preferences from a multi-scale spatial perspective: insights from marsh-nesting avian species. PeerJ. 5: e3164. doi: 10.7717/peerj.3164
- Jedlikowski, J., Chibowski, P., Karasek, T. & Brambilla, M. 2016. Multi-scale habitat selection in highly territorial bird species: exploring the contribution of nest, territory and landscape levels to site choice in breeding rallids (Aves: Rallidae). Acta Oecol. 73: 10–20. doi: 10.1016/j.actao.2016.02.003
- Leopold, C.R. & Hess, S.C. 2013. Multi-scale habitat selection of the endangered Hawaiian Goose. Condor 115: 17–27 doi: 10.1525/cond.2012.120022
- Madge, S. & McGowan, P. 2002. Pheasants, Partridges and Grouse: including buttonquails, sandgrouse and allies. Christopher Helm, London.
- Maghnouj, M. 1983. Contribution à l’étude de l’écologie et de la biologie de la reproduction de la Perdrix gambra (Alectoris barbara B.) dans trois régions du Maroc. Mémoire 3ème cycle, Inst. Agro. Vét. Hassan II, Rabat, 109 p.
- McGarigal, K., Wan, H.Y., Zeller, K.A., Timm, B.C. & Cushman, S.A. 2016. Multi-scale habitat modeling: a review and outlook. Landsc. Ecol. 31: 1161–1175. doi: 10.1007/s10980-016-0374-x
- Mehls, C., Jensen, K.C., Rumble, M.A. & Wimberly, M.C. 2014. Multi-scale habitat use of male ruffed grouse in the Black Hills National Forest. Prairie Nat. 46: 21–33.
- Mertler, C.A. & Vannatta, R.A. 2002. Advanced and Multivariate Statistical Methods: practical applications and interpretation, 2nd ed. Pyrczak, Los Angeles, CA.
- Mezerdi, F. 2011. Connaissance, conservation et gestion de la population de la Perdrix gambra (Alectoris barbara, Bonnaterre, 1792) En Algérie. Mémoire de Magister, 119 p.
- Mezerdi, F. 2015. Biologie de la reproduction d’une population captive de la Perdrix gambra (Alectoris barbara) et dynamique des populations en milieux prés forestiers et présahariens en Algérie. Thèse de Doctorat. 95 p.
- Novoa, C., Aebischer, N.J. & Landry, P. 2002. Upland habitat use by Pyrenean grey partridges Perdix perdix hispaniensis during the breeding season. Wildlife. Biol. 8: 99–108. doi: 10.2981/wlb.2002.014
- Oksanen, J., Blanchet, F.G., Kindt, R., Legendre, P., Minchin, P.R., O’Hara, R.B., Simpson, G.L., Solymos, P., Stevens, M.H.H. & Wagner, H. 2013. Vegan: Community Ecology Package. R package version 2.0-7.
- Ong-in, T., Pierce, A.J., Gale, G.A., Browne, S.J. & Savini, T. 2016. Nesting ecology and nest site selection of green-legged partridge. Raffles Bull. Zool. 64: 89–97.
- Panek, M. & Kamieniarz, R. 2000. Habitat use by the partridge Perdix perdix during the breeding season in the diversified agricultural landscape of Western Poland. Acta Ornithol. 35: 183–189. doi: 10.3161/068.035.0211
- Pastor, J., Mladenoff, D., Haila, Y., Bryant J. & Payette, S. 1996. Biodiversity and ecosystem processes in boreal regions. In Mooney, H.A., Cushman, J.H., Medina, E., Sala, O.E., and Schulze, E.-D. (eds). Functional Roles of Biodiversity: a global perspective, 33–70. Wiley Press, New York, NY.
- Pebesma, E.J. 2006. The gstat package.www.gstat.org.
- Pérez-Granados, C., Lopez-Iborra, G.M. & Seoane, J. 2017. A multi-scale analysis of habitat selection in peripheral populations of the endangered Dupont’s Lark Chersophilus duponti. Bird Conserv. Int. 27: 398–413. doi: 10.1017/S0959270916000356
- Pickens, B.A. & King, S.L. 2014. Multi-scale habitat selection of wetland birds in the Northern Gulf Coast. Estuaries Coast 37(5):1301–1311.
- Quantum GIS Development Team. 2017. QGIS Geographic information system, Version 2.18.14-Las Palmas. Open Source Geospatial Foundation, Chicago.
- R Development Core Team. 2013. R: a language and environment for statistical computing. ISBN 3-900051-07-0, R Foundation for Statistical Computing, Vienna. http://www.R-project.org.
- Rands, M.R.W. 1988. The effect of nest site selection on nest predation in grey partridge Perdix perdix and red-legged partridge Alectoris rufa. Ornis Scandinavica 19: 35–40. doi: 10.2307/3676525
- Razgour, O., Hanmer, J. & Jones, G. 2011. Using multi-scale modelling to predict habitat suitability for species of conservation concern: the grey longeared bat as a case study. Biol. Conserv. 144: 2922–2930. doi: 10.1016/j.biocon.2011.08.010
- Reitz, F., Le Goff, E. & Fuzeau, M. 2002. Landscape selection by Grey Partridge (Perdix perdix) for nesting in the fields of French cereal agrosystems. Game and Wildlife Science 19: 209–220.
- Ricci, J.C., Mathon, J.F., Garcia, A., Berger, F. & Esteve, J.P. 1990. Effect of habitat structure and nest site selection on nest predation in red-legged partridges (Alectoris rufa L.) in French Mediterranean farmlands. Gibier Faune Sauvage 7: 231–253.
- Saalfeld, S.T., Conway, W.C., Haukos, D.A., Rice, M., Jones, S.L. & Fellows, S.D. 2010. Multiscale habitat selection by long-billed curlews (Numenius americanus) breeding in the United States. Waterbirds 33:148–161.
- Scandura, M., Lacolina, L., Apollonio, M., Dessì-Fulgheri, F. & Baratti, M. 2010. Current status of the Sardinian partridge (Alectoris barbara) assessed by molecular markers. Eur. J. Wildl. Res. 56: 33–42 doi: 10.1007/s10344-009-0286-z
- Sing, T., Sander, O., Beerenwinkel, N. & Lengauer, T. 2005. ROCR: visualizing classifier performance in R. Bioinformatics. 21: 3940–3941. doi: 10.1093/bioinformatics/bti623
- Spano, S., Pellegrino, I. & Borgo, E. 2013. On the systematic status of the Cyrenaic Partridge (Alectoris barbata Reichenow, 1896). Avocetta 37: 145–148.
- Swets, J. 1988. Measuring the accuracy of diagnostic systems. Science 240: 1285–1293. doi: 10.1126/science.3287615
- Thévenot, M., Vernon, J.D.R. & Bergier, P. 2003. The Birds of Morocco: an annotated checklist. BOU Check-listno. 20. British Ornithologists’ Union, Tring.
- Zimmermann, N.E., Yoccoz, N.G., Edwards, T.C., Meier, E.S., Thuiller, W., Guisan, A., Shmatz, D.R. & Pearman, P.B. 2009. Climatic extremes improve predictions of spatial patterns of tree species. Proc. Natl. Acad. Sci. USA. 106: 19723–19728. doi: 10.1073/pnas.0901643106