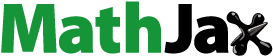
ABSTRACT
Low-order ignition models are important tools in the design of aviation gas turbines. In this paper, a stochastic model that predicts the ignition probability in a combustor based on a time-averaged cold-flow solution is extended to include local fuel concentration fluctuations due to the polydisperse nature of the spray. For this, a stochastic approach to modeling such fluctuations is considered, and the effects of the flow and mixture parameters on the resulting equivalence ratio pdfs are investigated. The concentration of fuel in large droplets results in a high variation of the local equivalence ratio, hence affecting the local flammability factor at the model’s cell scale. The extinction criterion of the ignition model based on a critical Karlovitz number is calibrated based on ignition probability data from canonical experiments using jet fuel, suggesting critical Karlovitz values of spray flames between 0.2 and 0.6, which is to be contrasted with values of 1.5 for gaseous fuels.
Introduction
Ignition is a key issue in the design of aviation gas turbines, limiting the operating range of the engine and the use of non-conventional fuels. The ignition of a combustor is inherently an unsteady process with a stochastic nature that depends on the characteristics of the spark device, turbulent flow and spray atomization. In non-premixed and stratified systems, successful initiation of a flame strongly depends on the presence of a mixture with optimal equivalence ratio in the vicinity of the spark (Lefebvre and Ballal Citation2010). This principle is represented by the concept of the flammability factor, which gives the probability of finding flammable mixture at a given location in a combustor. Nevertheless, even if a thermal runaway process is successfully initiated, full-burner ignition requires the subsequent growth and eventual stabilization of the flame (Mastorakos Citation2009).
Ignition of sprays is distinctly characterized by the need to provide gaseous fuel through evaporation for reaction to occur. Thus, it depends on additional parameters such as droplet size, fuel volatility and pre-vaporization. The presence of the fuel in the liquid form may cause strong mixture inhomogeneities that affect the process (Mastorakos Citation2017). A mixture that is too lean or presents liquid fuel in excess at the spark location can negatively affect the temperature rise of the kernel (Wandel, Chakraborty, Mastorakos Citation2009) and radical formation (Beduneau et al. Citation2009; Cardin et al. Citation2013), suppressing the onset of thermal runaway. Hence, the extinction of the kernel may be observed immediately following the deposition of energy, a process that has been defined as the short mode of ignition failure (Mastorakos Citation2017). Even under ideal atomization conditions, the resulting spray is characterized by polydisperse droplets. In a polydisperse spray, it is possible that, for instance, 50% of the total fuel mass may be carried by droplets that are large but scarce, representing less than 2% of the droplet population. Thus, the presence of the large droplets may have a disproportional effect on the local mixture at the scale of the flame. This strong small-scale fuel inhomogeneities may cause a successfully established flame kernel to experience local heat release fluctuations or even a lack of flammable mixture in its immediate surrounding, resulting in its extinction by long-mode failure (Mastorakos Citation2017).
Large-eddy simulations have been well established as a tool for the numerical study of ignition events in gas turbine combustors (Boileau et al. Citation2008; Esclapez, Riber, Cuenot Citation2015; Jones and Tyliszczak Citation2010) but assessing the stochastic behavior of the process is difficult due to the large number of simulations required. Furthermore, the ignitability of the engine is highly dependent on its operating conditions, for example, from ground cold-start to high-altitude relight, especially due to changes in atomization and fuel distribution occurring in the combustor. Additionally, alternative jet fuels or blends may impact further the ignition process by directly affecting flame propagation, as well as the atomization and evaporation of fuel due to differences in thermophysical properties. Moreover, ignition of the combustor must be ensured under all circumstances. For that reason, low-order models for prediction of ignition capability can be valuable tools, allowing the designer to perform wide parametric studies. Several approaches have been proposed to assess the ignition probability in a combustor, based on the cold flow field (Eyssartier et al. Citation2013; Neophytou, Richardson, Mastorakos Citation2012; Weckering et al. Citation2011) and on a well-stirred reactor model (Sforzo and Seitzman Citation2017).
The low-order ignition model called Stochastic Particle Integrator for High-Altitude Relight (SPINTHIR), developed by Neophytou, Richardson, and Mastorakos (Citation2012), simulates the stochastic motion of virtual “flame particles” to predict the ignition probability map in a combustor. The model is based on a time-averaged cold-flow field and a Karlovitz number extinction criterion (Abdel-Gayed and Bradley Citation1985), and has been tested for non-premixed and spray flames (Neophytou, Richardson, Mastorakos Citation2012; Soworka et al. Citation2014) and premixed flames (Sitte et al. Citation2016). Nevertheless, previous experimental evidence suggests that spray flames can extinguish at global Damköhler numbers that differ from those verified by Abdel-Gayed and Bradley (Citation1985) in gaseous premixed flames (Bradley et al. Citation2014; Cavaliere, Kariuki, Mastorakos Citation2013; Yuan, Kariuki, Mastorakos Citation2018). This may occur because of the contribution of droplet-induced stretch due to strain and curvature at the droplet scale (Wacks and Chakraborty Citation2016). Evidence of this effect has been seen experimentally in bluff-body stabilized spray flames (Cavaliere, Kariuki, Mastorakos Citation2013) and swirl-stabilized spray flames (Yuan, Kariuki, Mastorakos Citation2018), where extinction of the flames was observed at values of Karlovitz number between 0.8 and 1.2 for a range of fuels, in comparison to 1.5 for gaseous premixed flames. A preliminary study on this issue related to the application of SPINTHIR has been carried out by the present authors (de Oliveira, Sitte, Mastorakos Citation2019).
Moreover, in previous applications of SPINTHIR, fuel fluctuations due to the presence of droplets have not been considered in the model. In recent experiments (de Oliveira and Mastorakos Citation2019) and direct numeric simulations (Wandel, Chakraborty, Mastorakos Citation2009) in overall lean and dilute sprays, ignition success has been directly attributed to the presence of large droplets in the kernel and its vicinity. In addition to producing a flammable mixture at the moment of the spark, fuel inhomogeneities due to the spray may allow for the propagation of the flame in regions of stoichiometric to rich mixture, leading to greater heat release and successful kernel growth (Wandel, Chakraborty, Mastorakos Citation2009). Thus, a model that takes into account flammability effects at the spark location and fuel fluctuation effects on flame propagation due to spray characteristics is necessary to improve ignition prediction in sprays.
The present work aims at investigating the flame extinction criterion in sprays, providing a calibration for the model based on ignition probability and flame speed measurements in well-characterized canonical experiments using jet fuel (de Oliveira and Mastorakos Citation2019). Additionally, the effect of polydispersity on local fluctuations in liquid fuel mass is evaluated assuming uniformly distributed polydisperse droplets. The number of droplets found at a location in the flow is modeled using a stochastic approach. An analysis of the probability density functions of local equivalence ratio as well as flammability plots are presented in terms of spray and mixture parameters. Finally, possible improvements to the low-order ignition model SPINTHIR are discussed.
Low-order ignition model
SPINTHIR
The model (Neophytou, Richardson, Mastorakos Citation2012) predicts the ignition probability of a combustor based on the stochastic motion of “flame particles” simulated from parameters obtained from a time-averaged non-reacting flow field, obtained from simulations or measurements. These parameters are the mean gas velocity , the turbulent velocity fluctuation
, the integral length scale
, the mean gaseous and liquid mixture fractions,
and
, the volumetric source term due to evaporation
, and the Sauter mean diameter
, all given as functions of the space variable x. Inspired by cellular automata methods, the fluid domain is discretized in rectangular cells which can assume two possible states, i.e. hot or cold, which are determined by the motion of flame particles. At the beginning of the simulation, all cells are set to the cold state. An ignition event is modeled by switching one or more cells in the domain to the hot state. As the cells switch to the hot state, each emits a virtual flame particle that follows a random walk given by a simplified Langevin model,
The variables and
represent the displacement and change in velocity vectors of the particle. The turbulent dissipation rate is estimated as
, and the random component to the velocity is added by
, a vector with random direction and length based on a normal distribution
. The evolution of the particle gaseous mixture fraction is given by,
where is the gas density, which is obtained, for example, from the cold-flow field.
In order to represent the growth of the flame, a new particle is emitted when a flame particle enters a cold cell, switching this cell to the hot state. At each time step, an extinction criterion is applied to the particles. This criterion is based on the Karlovitz number of the particle, and can be evaluated from the empirical correlation (Abdel-Gayed and Bradley Citation1985),
Extinction occurs if . Additionally, particles that have extinguished are no longer computed in the following time steps. Further, ignition success is assessed based on the fraction of domain cells that are marked as burnt and probability of ignition,
, is evaluated by performing a large number of simulations for the same condition. In the evaluation of
, the laminar burning velocity of the spray flame,
, is evaluated from the correlation proposed in (Neophytou and Mastorakos Citation2009) based on the droplet Sauter mean diameter,
. For that, the Sauter mean diameter of the spray is used, and also the particle’s equivalence ratio,
, and degree of prevaporisation,
,
The gas-phase equivalence ratio is calculated from,
where is the stoichiometric mixture fraction and
is solved by EquationEq. (3
(3)
(3) ). The evaluation of
is modeled next to account for the liquid fuel fluctuations.
Fuel-fluctuations modeling
The effect of liquid fuel fluctuations arising from a polydisperse droplet distribution concerning a single domain cell of the low-order ignition model is here analyzed. First, a polydisperse droplet distribution is defined for each cell, based on a modified Rosin-Rammler distribution. Given the local droplet distribution and local mixture parameters obtained from the cold-flow solution, it is possible to obtain the mean number density of each droplet size class. From this parameter, the probability of finding a specific number of droplets of each class in the cell can be modeled using a stochastic approach. Finally, in the model, the number of droplets of each size class in the cell can be randomly obtained from a probability density function for each droplet class, and the resulting random liquid equivalence ratio of the cell can be computed.
In each cell, the spray is defined based on a modified Rosin-Rammler distribution, where the accumulated liquid volume fraction (Rizk and Lefebvre Citation1985) is,
Thus, the fraction of liquid contained in droplets of size is simply,
Based on the liquid volume fraction of the two-phase mixture obtained from the cold-flow solution, , the local liquid volume fraction of the mixture for each droplet class is,
Further, assuming an arbitrary volume containing
droplets of diameter
, the number of droplets of such size in a subvolume
(being the volume of a domain cell,
) follows a binomial distribution. The probability of finding exactly
droplets in
can be calculated,
where is the probability of finding a specific droplet in
, that is,
. Note that
should be chosen such that,
Thus, EquationEq. (10(10)
(10) ) can be approximated by a Poisson distribution,
where is the mean number of droplets of size
in the cell,
, and
.
By using EquationEq. (12(12)
(12) ), a random number of droplets for each class
in the cell,
, can be obtained. Hence, the total amount of liquid fuel in
can be calculated as being,
The particle’s liquid equivalence ratio in the low-order ignition model is evaluated as,
with the liquid and air densities, and
, and the stoichiometric air–fuel ratio, AFR. For the sake of the parametric analysis presented in this section, it can be assumed that
, such that EquationEq. (13
(13)
(13) ) can be written in terms of the liquid equivalence ratio,
,
or in terms of the equivalence ratio of the cell,
where . Thus, the probability density function of
can be evaluated using a Monte-Carlo approach. Additionally, the flammability factor of the cell is given by (Mastorakos Citation2009),
A parametric analysis was performed considering jet fuel, with prevaporisation between 0% to 99% and overall equivalence ratio, , from 0.1 to 4. The
parameter of the modified Rosin-Rammler distribution was set from 10 to 160 μm, resulting in the
curves and pdf(
) shown in . For these distributions, the Sauter mean diameter
as a function of
is also shown in , varying from 15 μm for a fine and monodisperse-like spray up to 90 μm. The cell size was chosen between 1.5 and 5 μm for the analysis. For each condition, the approximation of
from EquationEq. (12
(12)
(12) ) was used, and every pdf was generated from one million samples.
Figure 1. The modified Rosin-Rammler (a) accumulated volume and (b) droplet size pdfs in terms of . The sauter mean diameter is shown in (b) for the range of
highlighted in (a).
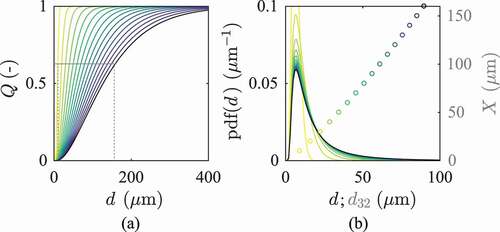
The effect of the cell size and droplet size distribution on the pdfs of is shown in , with the line color scheme shown according to the distributions of droplet size in . For this given condition,
was set just above the lower flammability limit
(dashed vertical line). The effect on the local equivalence ratio caused by the polydispersity can be clearly noticed: coarse atomization conditions (high
) resulted in high probability for values below
, with leaner conditions than the average cell equivalence ratio
being more likely to occur. As
decreases and the spray becomes closer to monodisperse condition this effect disappears. Evidently, the size of the domain cell also determines the magnitude of the fluctuations in this model. Thus,
must be chosen according to physical criteria (e.g. chemical, turbulent, and evaporation time/length scales) in addition to those given in Neophytou, Richardson, Mastorakos (Citation2012) to satisfy the assumption of turbulent transport of the flame particles by the eddies.
Figure 2. PDFs of the local equivalence ratio relative to the overall equivalence ratio in terms of the droplet distributions ( 20–90 mm) for cell size
of 1.5, 3, and 5 mm –
0.7,
0.
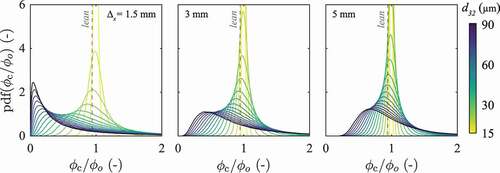
The effect of the overall (average) equivalence ratio for a fixed droplet size distribution ( 50 μm) and a cell size of 3 mm, typical of the spark size in de Oliveira and Mastorakos (Citation2019) and used here for validation, is shown in . Increasing
slightly decreased the magnitude of the fluctuations of
, as the droplet number density increases with
. Still, for the given cell size, this effect was only significant for
over 50 μm. Further, increasing prevaporisation () led to shift of the leanest part of the pdfs toward
, as expected. However, this effect also led to a reduction of events where
is richer than
, which can be relevant in conditions lower than the lower flammability limit.
Moreover, show that the polydispersity of the spray may reduce the local flammability at the spark location and surroundings of the flame kernel by producing mixtures that are either below or above the flammable limits. Nevertheless, the actual value of is also key to ignition, as seen that stoichiometric and rich mixtures require significantly less spark energy in order to result in self-sustained flame propagation (Chakraborty and Mastorakos Citation2008; Wandel, Chakraborty, Mastorakos Citation2009). Also, the variation of
has also a direct impact on
through the evaluation of
. Thus, for a given lean overall mixture in the combustor, the spray polydispersity can potentially enhance the ignitability by giving rise to locally stoichiometric to rich mixtures, enhancing the flammability at the spark as well as flame propagation. Further, isosurfaces of flammability are plotted in in terms of the overall equivalence ratio, degree of prevaporisation and Sauter mean diameter. For a cell size of 3 mm, positive effects on flammability were reduced to values of overall equivalence ratio close to the lower and upper flammability limits, while for a smaller 1.5-mm cell a significant reduction of flammability can be noticed for a stoichiometric equivalence ratio and
of 50 μm.
Figure 3. PDFs of the local equivalence ratio relative to the overall equivalence ratio in terms of the overall equivalence ratio (0.4–3) for droplet distributions with
of 15, 30, and 50 μm –
3 mm,
0.
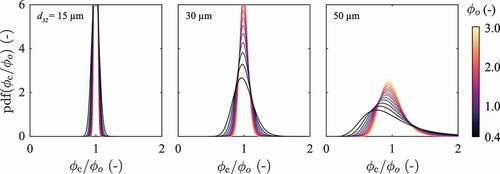
Calibration procedure
In order to verify the model’s extinction criterion under spray conditions, a calibration method based on direct measurements of ignition probability is proposed. Additionally, the effect of local liquid fuel fluctuations on the calibration is also explored. The calibration consists in evaluating the set in the model, for which the resulting ignition probability of the model corresponds to the experimental measurement. The method is shown in for a simulation where the liquid fuel fluctuations have not been included in the ignition model. The number of burnt cells in time for each set
is presented in , with successful ignition events shown in gray and failed ignition attempts in black. Ignition probability was calculated for each value of
, and the resulting ignition probability in terms of that parameter is shown in . The latter plot was used to obtain a calibrated
(approximately 0.2 for the given condition) by using experimental data obtained from (de Oliveira and Mastorakos Citation2018). In the experiments, a uniform droplet distribution in a weakly turbulent jet was ignited by a laser, and the probability of ignition was evaluated from OH* image sequences of the flame.
Figure 6. (a) Evolution of the number of burnt cells in the domain for set as 0.1, 0.3, 0.7, and 1. Ignition events are shown in gray and failed events in black. (b) Resulting probability of ignition for the conditions presented in (a) and comparison to experimental measurements – Jet A,
,
,
29 μm (de Oliveira, Sitte, Mastorakos Citation2019).
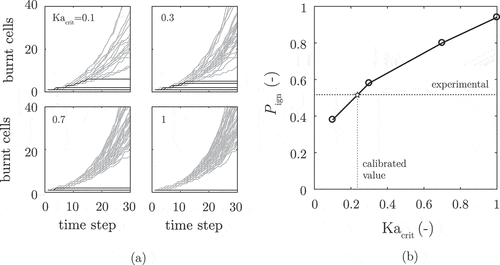
A brief description of the setup is given next for completeness – a full characterization of the flow has been presented in a previous work (de Oliveira, Allison, Mastorakos Citation2019). A two-phase jet was formed by atomizing the liquid fuel in a 100°C air flow inside a diverging–converging section. Atomization occurred mostly within the diverging section, thus avoiding droplet impingement against the walls. As the flow carried the droplets through the converging section, exiting through a 20.8-mm ID nozzle, a jet characterized by a top-hat velocity profile and uniform turbulence levels (approximately 10%) was formed. In the jet, droplets were uniformly dispersed and presented a polydisperse distribution. The SMD of the spray was set between 16 and 33 μm. A 532-nm laser beam was focused at the center of the jet, allowing for the breakdown of the mixture and initiation of a flame kernel immediately downstream the nozzle. An example of the flame characteristics as observed with schlieren and OH*-chemiluminescence is given in , which shows the flames at approximately 1 ms after the breakdown by the spark.
Figure 7. Simultaneous (a) schlieren and (b) OH*-chemiluminescence of the spherically-expanding Jet A flames at 1 ms after the spark. The highest (33 μm) and lowest (16 μm) SMD conditions are shown for and 1.4. Modified from de Oliveira and Mastorakos Citation2019.
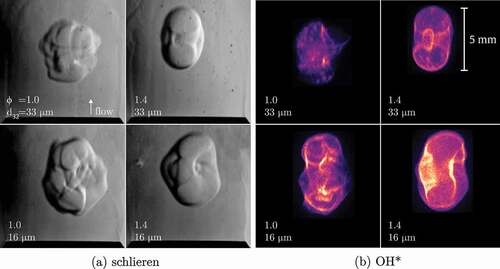
In the experiment, the probabilities of ignition and of breakdown (i.e., initiation of a flame kernel as reveleaded by OH*), and
, respectively, were obtained from 360 spark attempts in the flow. In addition to that, measurements of the burnt flame speed were also performed. It should be noted as not all sparks resulted in the breakdown of the mixture, the experimental value
was used for comparison to
obtained with the model. For simplicity, the corrected experimental ignition probability value is referred to as
. In the present simulations, the probability of ignition at each condition was evaluated from 50 ignition attempts in each flow condition and
. The flow was simply modeled as a uniform flow, with constant mean and root-mean-square velocity as well as droplet distribution throughout the domain. The spark diameter and the grid cell size were chosen as 3 mm, that is, smaller than
, for consistency with the modeling assumptions (Neophytou, Mastorakos, Cant Citation2010). Additionally, a 0.5-ms time step was used, which also satisfies the modeling assumptions, being shorter than the mean turbulent scale
of the flow (approximately 10 ms).
Results
First, a preliminary calibration of SPINTHIR was carried out without accounting for liquid fuel fluctuations due to the spray. The effects of the SMD and overall equivalence ratio on the calibrated critical Karlovitz number, , were evaluated in order to improve our understanding of the application of present model to sprays. shows the resulting
as a function of SMD for an overall stoichiometric and a rich mixture condition. Within the present atomization conditions of 16–33 μm, values of
were mostly observed between 0.1 and 0.6, that is, significantly lower than 1.5 obtained for gaseous premixed flames (Abdel-Gayed and Bradley Citation1985). This is possibly related to phenomena that contribute to flame extinction, enhancing heat loss from the flame in addition to turbulent strain. For example, added flame wrinkling and evaporative cooling occurring around large droplets as they approach the flame were observed in DNS (Ozel Erol et al. Citation2018, Citation2019; Wandel Citation2014) as well as in experiments (de Oliveira and Mastorakos Citation2019). Additionally, for the 23-μm rich spray, a high
was obtained as a result of the highly under-prediction of flame speed by the model’s correlation for that particular condition. shows a comparison between measurements (de Oliveira and Mastorakos Citation2019) and values of flame speed obtained with the model (Neophytou and Mastorakos Citation2009) used in SPINTHIR, showing a reasonably good agreement between the two, except for the condition previously pointed out. Furthermore, a negative correlation between
and
was observed for both equivalence ratios (). This is consistent with the observed droplet-related phenomena and their contribution to flame extinction, as such effects are likely to become more intense as the spray polydispersity and the droplet sizes increase.
Figure 8. (a) Calibrated critical Karlovitz number for Jet A and (b) comparison of flame speed measurements de Oliveira and Mastorakos (Citation2019) and values obtained with the correlation by Neophytou and Mastorakos (Citation2009).
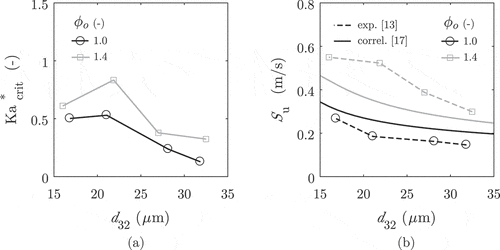
Fuel fluctuations are accounted for in the calibration shown next in . In this case, the stochastic model presented previously was included in the low-order ignition model. Local fuel fluctuations affected the calculation of in two particular ways. First, through changes in the flammability of a cell, which may cause a particle to extinguish directly. Second, and perhaps most important for the present range of conditions, such modeling introduces fluctuations to the local equivalence ratio of the cell and, in turn, to the local
. The effect of the fuel fluctuations on the calibration is shown in for a rich 33-μm spray. In this case, a significant decrease of the probability of ignition for any set
was observed, leading to a higher
. The resulting
for the whole range of conditions (
,
) is shown in . Overall, the implementation of the liquid fluctuations resulted in higher
values for high SMD conditions, while low SMD conditions remained approximately the same, as it was expected from the results presented in the Monte-Carlo analysis of the model.
Conclusion
The effects of liquid fuel fluctuations arising from the spray’s polydispersity were investigated in the context of a low-order ignition model. A stochastic modeling approach was used to include such fluctuations in the model, and their resulting effects on the local equivalence ratio and flammability at the model’s cell level were investigated as a function of spray characteristics. A Monte-Carlo analysis showed that a change in flammability and, most importantly, high fluctuations of equivalence ratio may occur, presenting a way of incorporating droplet-induced effects in the ignition model. Further, experimental data of ignition probability was used to calibrate the extinction parameter of the model, the critical Karlovitz number. This calibration presented critical values between 0.2 and 0.6, approximately, that is, significantly lower than in gaseous premixed flames. Once the stochastic fuel fluctuation model was considered in the ignition model, the correlation of the critical Karlovitz number with the spray SMD became less evident, as the calibrated values concerning high SMD conditions increased. Moreover, following the present calibration and introduction of a stochastic model to account for the spray polydispersity, it is expected that the present low-order ignition model should better predict the ignitability of combustors operating with liquid fuel, specially at conditions where fuel atomization is compromised such as in cold-start of aviation gas turbines. In order to improve the accuracy of the present calibration, the model should be tested against a wider range of experimental conditions in canonical configurations.
Acknowledgments
P.M. de Oliveira gratefully acknowledges the financial support of the Brazilian Space Agency and Brazil’s National Council for Scientific and Technological Development. M.P. Sitte is grateful for the financial support from the Gates Cambridge Trust. The authors also kindly acknowledge the funding granted by the European Commission Clean Sky 2 project PROTEUS (785349).
References
- Abdel-Gayed, R. G., and D. Bradley. 1985. Criteria for turbulent propagation limits of premixed flames. Combust. Flame. 62 (1):61–68. doi:https://doi.org/10.1016/0010-2180(85)90093-8.
- Beduneau, J. L., N. Kawahara, T. Nakayama, E. Tomita, and Y. Ikeda. 2009. Laser-induced radical generation and evolution to a self-sustaining flame. Combust. Flame. 156 (3):642–56. doi:https://doi.org/10.1016/j.combustflame.2008.09.013.
- Boileau, M., G. Staffelbach, B. Cuenot, T. Poinsot, and C. Bérat. 2008. LES of an ignition sequence in a gas turbine engine. Combust. Flame. 154 (1–2):2–22. doi:https://doi.org/10.1016/j.combustflame.2008.02.006.
- Bradley, D., M. Lawes, S. Liao, and A. Saat. 2014. Laminar mass burning and entrainment velocities and flame instabilities of i-octane, ethanol and hydrous ethanol/air aerosols. Combust. Flame. 161 (6):1620–32. doi:https://doi.org/10.1016/j.combustflame.2013.12.011.
- Cardin, C., B. Renou, G. Cabot, and A. M. Boukhalfa. 2013. Experimental analysis of laser-induced spark ignition of lean turbulent premixed flames: New insight into ignition transition. Combust. Flame. 160 (8):1414–27. doi:https://doi.org/10.1016/j.combustflame.2013.02.026.
- Cavaliere, D. E., J. Kariuki, and E. Mastorakos. 2013. A comparison of the blow-off behaviour of swirl-stabilized premixed, non-premixed and spray flames. Flow. Turbul. Combust. 91 (2):347–72. doi:https://doi.org/10.1007/s10494-013-9470-z.
- Chakraborty, N., and E. Mastorakos. 2008. Direct numerical simulations of localised forced ignition in turbulent mixing layers: The effects of mixture fraction and its gradient. Flow. Turbul. Combust. 80 (2):155–86. doi:https://doi.org/10.1007/s10494-007-9110-6.
- de Oliveira, P. M., P. M.Allison, and E. Mastorakos. 2019. Ignition of uniform droplet-laden weakly turbulent flows following a laser spark. Combust. Flame. 199:387–400. doi:https://doi.org/10.1016/j.combustflame.2018.10.009.
- de Oliveira, P. M., and E. Mastorakos (2018), Effects of droplet size on the ignition of conventional and alternative jet fuels in turbulent air. 9th International Symposium On Turbulence, Heat And Mass Transfer, Rio de Janeiro.
- de Oliveira, P. M., and E. Mastorakos. 2019. Mechanisms of flame propagation in jet fuel sprays as revealed by OH/fuel planar laser-induced fluorescence and OH* chemiluminescence. Combust. Flame. 206:308–21. doi:https://doi.org/10.1016/j.combustflame.2019.05.005.
- de Oliveira, P. M., M. P. Sitte, and E. Mastorakos (2019), Validation of a low-order model for ignition of sprays. AIAA Scitech 2019 Forum, Reston, Virginia, American Institute of Aeronautics and Astro- nautics
- Esclapez, L., E. Riber, and B. Cuenot. 2015. Ignition probability of a partially premixed burner using les. Proc. Combust. Inst. 35 (3):3133–41. doi:https://doi.org/10.1016/j.proci.2014.07.040.
- Eyssartier, A., B. Cuenot, L. Y. Gicquel, and T. Poinsot. 2013. Using LES to predict ignition sequences and ignition probability of turbulent two-phase flames. Combust. Flame. 160 (7):1191–207. doi:https://doi.org/10.1016/j.combustflame.2013.01.017.
- Jones, W. P., and A. Tyliszczak. 2010. Large eddy simulation of spark ignition in a gas turbine combustor. Flow. Turbul. Combust. 85 (3–4):711–34. doi:https://doi.org/10.1007/s10494-010-9289-9.
- Lefebvre, A. H., and D. R. Ballal. 2010. Gas turbine combustion: Alternative fuels and emissions. 3rd ed. Boca Raton: CRC Press.
- Mastorakos, E. 2009. Ignition of turbulent non-premixed flames. Prog. Energy. Combust. Sci. 35 (1):57–97. doi:https://doi.org/10.1016/j.pecs.2008.07.002.
- Mastorakos, E. 2017. Forced ignition of turbulent spray flames. Proc. Combust. Inst. 36 (2):2367–83. doi:https://doi.org/10.1016/j.proci.2016.08.044.
- Neophytou, A., and E. Mastorakos. 2009. Simulations of laminar flame propagation in droplet mists. Combust. Flame. 156 (8):1627–40. doi:https://doi.org/10.1016/j.combustflame.2009.02.014.
- Neophytou, A., E. Mastorakos, and R. S. Cant. 2010. DNS of spark ignition and edge flame propagation in turbulent droplet-laden mixing layers. Combust. Flame. 157 (6):1071–86. doi:https://doi.org/10.1016/j.combustflame.2010.01.019.
- Neophytou, A., E. Richardson, and E. Mastorakos. 2012. Spark ignition of turbulent recirculating non-premixed gas and spray flames: A model for predicting ignition probability. Combust. Flame. 159 (4):1503–22. doi:https://doi.org/10.1016/j.combustflame.2011.12.015.
- Ozel Erol, G., J. Hasslberger, M. Klein, and N. Chakraborty. 2018. A direct numerical simulation analysis of spherically expanding turbulent flames in fuel droplet-mists for an overall equivalence ratio of unity. Phys. Fluids. 30 (086104). doi:https://doi.org/10.1063/1.5045487.
- Ozel Erol, G., J. Hasslberger, M. Klein, and N. Chakraborty. 2019. A direct numerical sim- ulation investigation of spherically expanding flames propagating in fuel droplet-mists for different droplet diameters and overall equivalence ratios. Combust. Sci. Technol. 191 (5–6):833–67. doi:https://doi.org/10.1080/00102202.2019.1576649.
- Rizk, N. K., and A. H. Lefebvre. 1985. Drop-size distribution characteristics of spill-return at- omizers. J. Propuls. Power. 1 (1):16–22. doi:https://doi.org/10.2514/3.22753.
- Sforzo, B., and J. Seitzman. 2017. Modeling ignition probability for stratified flows. J. Propuls. Power. 33 (5):1–11. doi:https://doi.org/10.2514/1.B36413.
- Sitte, M. P., E. Bach, J. Kariuki, H. J. Bauer, and E. Mastorakos. 2016. Simulations and experiments on the ignition probability in turbulent premixed bluff-body flames. Combust. Theory. Model. 20 (3):548–65. doi:https://doi.org/10.1080/13647830.2016.1155756.
- Soworka, T., M. Gerendas, R. L. G. M. Eggels, and E. Mastorakos (2014), Numerical investigation of ignition performance of a lean burn combustor at sub-atmospheric conditions. Combustion Fuels Emission. V04AT04A046. Vol. 4A, ASME.
- Wacks, D. H., and N. Chakraborty. 2016. Flame structure and propagation in turbulent flame-droplet interaction: A direct numerical simulation analysis. Flow. Turbul. Combust. 96 (4):1053–81. doi:https://doi.org/10.1007/s10494-016-9724-7.
- Wandel, A. P. 2014. Influence of scalar dissipation on flame success in turbulent sprays with spark ignition. Combust. Flame. 161 (10):2579–600. doi:https://doi.org/10.1016/j.combustflame.2014.04.006.
- Wandel, A. P., N. Chakraborty, and E. Mastorakos. 2009. Direct numerical simulations of turbulent flame expansion in fine sprays. Proc. Combust. Inst. 32 (2):2283–90. doi:https://doi.org/10.1016/j.proci.2008.06.102.
- Weckering, J., A. Sadiki, J. Janicka, E. Mastorakos, and R. L. Eggels. 2011. A forced ignition probability analysis method using les and Lagrangian particle monitoring. Proc. Combust. Inst. 33 (2):2919–25. doi:https://doi.org/10.1016/j.proci.2010.07.033.
- Yuan, R., J. Kariuki, and E. Mastorakos. 2018. Measurements in swirling spray flames at blow- off. Int. J. Spray. Combust. Dyn. 10 (3):185–210. doi:https://doi.org/10.1177/1756827718763559.