Abstract
The recent proliferation of Large Language Models (LLMs) raises questions as to the role of such tools both within an educational learning environment and their epistemic capacity. If, as Alfred North Whitehead remarked, western philosophy indeed ‘consists of a series of footnotes to Plato’, it would be of doubtless importance to evaluate the position of LLMs in his epistemological framework. We analyse Plato and existing scholarship regarding his epistemology, combining this with a brief outline of the architectural features of GPT-3 and similar LLMs, before finally addressing whether they meet Plato’s criteria, and where they stand in relation to education in general. We conclude that, in conjunction with the well-known factual unreliability of LLMs, they are likewise unsuited for the satisfaction of a Platonic epistemological standard. On account of this, we find LLMs to be sub-optimal within an educational learning environment, and this is why we suggest that their use be accordingly circumscribed.
Introduction
Recent developments in machine learning and computational performance have resulted in the rapid proliferation of artificial neural networks. Large Language Models (LLMs) are a particular form of artificial neural network which has received extensive attention from scholars as a result of its capacity as a disruptive technology. One such area where disruption has been anticipated is education (Baidoo-Anu & Ansah, Citation2023; Halaweh, Citation2023; Kasneci et al., Citation2023). Aside from important questions regarding the practical and ethical viability of this new technology, it is also necessary to investigate the epistemological implications of relying on this technology throughout the learning process. Such an epistemological analysis may provide insight into reliability of LLMs as an educative tool that may have implications regarding some of their well-known idiosyncrasies, such as their tendency to hallucinate information.
In approaching this issue, we ask the following question: Is learning with ChatGPT or other LLMs really learning? We find that whilst it has can come close to addressing some of Plato’s criteria, it does not do so effectively, as yet. Some aspects are potentially addressable by technological advances such as retrieval augmented approaches, but as yet we cannot say that learning with ChatGPT alone is learning. Practically this means human teachers still need to maintain a presence in the classroom and engage in process where students may be using AI tools as part of their learning. More specifically, in answering this self-imposed question, we explore whether LLMs are capable of validly learning knowledge themselves, and whether they may accordingly pass this knowledge to students. We adopt a Platonic epistemology and draw from two main bodies of literature. First, as a result of Plato’s well-known method of indirect exposition through dialogues, we attempt to delineate Plato’s epistemology by consultation of these dialogues in conjunction with existing scholarship (Allen, Citation1959; Burnyeat & Barnes, Citation1980; Nawar, Citation2013). We then provide a brief overview of the architecture of LLMs with reference to GPT-3. Finally, we apply our Platonic epistemology to the architecture of LLMs in their capacity as student and teacher. We find that LLMs do not fit comfortably within Plato’s epistemological framework. Our findings suggest that LLMs may have architectural limitations regarding access to universals and the capacity to engage in active discernment. Likewise, the process of training LLMs is not harmonious with the method of teaching established by Plato in Meno, and its deficiency in leading a Socratic Method reflects this. For these reasons, we conclude that learning with ChatGPT would not be regarded as truly learning according to Plato, although leave open the possibility that artificial neural networks may closer approximate his epistemology as we observe further advances.
Epistemology and learning in Plato
The forms
Plato’s epistemology finds its foundation in his theory of Forms. These Forms, described interchangeably by Plato as εἶδος or ἰδέα, impart qualitative attributes on matter, or ὕλη. The theory is Plato’s solution to the problem of universals, which asks how objects share common qualities. For example, both the Great Pyramid of Giza and the Louvre Pyramid are pyramidal, but neither are the same object. Therefore, there is something essential connecting these two objects as ‘pyramidal’ things. For Plato, both the structures are imperfect physical manifestations of the geometric idea of a pyramid, which involves a polygonal base connected to an apex. In Meno, Socrates explains the ontological separation of the Forms and particulars. Each Form, such as the form of a pyramid, exists ‘itself by itself’ (αὐτὸ καθ’ αὑτὸ) (Plato, Meno, 100b). Particulars like the Louvre Pyramid, in contrast, have qualitative characteristics as a result of participation of the Forms (Plato, Meno, 100d). This position is opposed to the prevailing nominalist position, which posits that abstract objects have no real existence apart from the names we assign out of convention. According to Plato, once the divide between the worlds of particulars and Forms has been scaled, it is not necessary to pass back to that of particulars, as the world of Forms perfectly contains all of the qualitative attributes which are participated in by the material world (Plato, Phaedo, 65e-66a).
The Form of equality, as Socrates explains, cannot be entirely understood by comparing sticks with like sticks, or stones with like stones, as any material manifestation of equality will necessarily fall short of equality in its purest Form (Plato, Phaedo, 74a-b). Even if two stones were perfectly equal in structure and size, they could not be simultaneously equal in position. As universal qualities cannot be understood by comparing particulars, but particulars can be understood with reference to their universals, understanding the nature of universals themselves therefore assumes a role of primacy for Plato (Seidel, Citation1991, p. 293). Indeed, in The Republic, Plato characterized the transition from knowledge of particulars to knowledge of the Forms as a transition from the world of becoming to the world of pure being, providing a strong ontological basis for knowledge (Sedley, Citation2004, p. 113). Plato’s Forms are also akin (συγγενής), thereby making it possible to understand some by reference to others (Plato, Meno, 81c-d; Seidel, Citation1991, p. 293). In Phaedo, Socrates argues that before we may perceive Forms in particulars, it is first necessary to have some knowledge of those Forms at birth (Plato, Phaedo, 75b). Forms are therefore epistemically prior to particulars (Allen, Citation1959, p. 169).
Anamnesis in Meno
Arguably central to Plato’s epistemology is the idea of anamnesis. In Meno, Plato raises the issue of the Meno’s Paradox (Plato, Meno, 80d). It is impossible for us to seek what we do not know, since we will not be able to determine whether we have found it. In response to this, Socrates demonstrates a theory of learning which he claims to have heard from certain priests and poets (Plato, Meno, 81a-b). The theory involves recollection from experience gathered by the immortal soul before it is reborn into the body of a person (Plato, Meno, 81c-d). In the Phaedrus, however, this process is described somewhat differently (Plato, Phaedrus, 247e-248d). Here, the soul is described as catching a glimpse of the Forms in a place beyond the heavens, before venturing out, forgetting the Forms, and being incarnated in the body of a human. Regardless of the myth used, recollection of the Forms must be possible because they are implicit in the very process of cognition (Allen, Citation1959, pp. 170-171). Plato’s methodology for accessing these Forms is most clearly described in Meno.
Socrates demonstrates his theory by teaching a boy geometry. This is achieved through questions regarding simple principles which are then used by the boy to make conclusions regarding more complex geometric ideas (Plato, Meno, 82a-85b). Socrates’ questions first lead the boy to wrongly conclude that the area of a square can be doubled by doubling the length of its sides. Further questions reveal this to be false, and the boy is eventually proven wrong again in assuming that a square could be doubled by increasing the length of its sides by 1.5x. With the help of Socrates’ guiding questions, he eventually comes to understand that a larger square around the original placed diagonally will produce a square with twice the area. The role of a teacher is therefore to aid the student in the recollection of innate ‘true opinions’ through Socratic inquiry, enabling the student to understand ideas without direct explication (Plato, Meno, 85c-d).
The use of mathematics in Plato’s example was not accidental, as mathematics, like virtue, cannot be effectively understood by means of direct explication alone (Allen, Citation1959, p. 167). The method Plato outlines in Meno is therefore primarily useful for learning abstract concepts of a general character, rather than specific factual particulars, such as the date of an event or names of historical characters.
Anamnesis in Phaedo
To properly apply a predicate, you must first know its meaning (Allen, Citation1959, p. 170). You must know what kinds of things are equal before you can call something equal. Similarly, this notion of equality must come from somewhere. These questions cannot be answered by explaining equality away as a mere abstraction constructed by the mind as it thinks about particulars in a universal way, as we know two things are equal due to their common character. Equality, as a Form, is part of the objective structure of Being.
Knowledge of particulars? – in Theaetetus
In the Theaetetus, Socrates rejects the idea of knowledge as being constituted by mere true belief, even if that true belief is justified with an account (Plato, Theaetetus, 201a-c, 209e-210a). The dialogue ends in aporia with the question of knowledge unanswered (Plato Theaetetus 210b). Burnyeat (Citation1980) has argued that for Plato, knowledge involved understanding, rather than justification (Nawar, Citation2013, p. 1052). Indeed, this view explains why in Meno it was ‘reasoning about the cause’ (αἰτίας λογισμῷ) which was associated with anamnesis and knowledge, in contrast to true opinion (Plato, Meno, 98a; Nawar, Citation2013, p. 1053).
Within the Theaetetus, Burnyeat has identified a paradox in Socrates’ example of litigants persuading jurors in court (Plato, Theaetetus, 201a-c; Nawar, Citation2013, p. 1054). Socrates seems to suggest that direct perceptual acquaintance is necessary for knowledge, while also suggesting that the jurors would not have gained knowledge in their circumstances for lack of time (Plato, Theaetetus, 201a-b; Nawar, Citation2013, p. 1055). Socrates’ choice of words; however, suggests that perceptual acquaintance is only required for some particular Forms of knowledge (Nawar, Citation2013, p. 1056). A few solutions have been proposed to address this. Burnyeat and Barnes argues that we should excise a few words in 201b of the Theaetetus, thereby leaving Socrates’ reasoning intact and removing his emphasis on eye-witness testimony (Burnyeat & Barnes, Citation1980, p. 193). Naturally this solution is quite invasive, and it is difficult to see Burnyeat and Barnes’ proposed excision as a mere consequence of rushed composition, particularly since Socrates mentions the eye-witnesses prior to the proposed excision (Plato, Theaetetus, 201b). Stramel instead argues that Plato was presenting disjunctive necessary conditions for knowledge—that knowledge may be adequately gained through either teaching or perceiving (Nawar, Citation2013, p. 1057). Nawar argues, however, that these disjunctive conditions are capable of being unified under a common feature.
According to Nawar, Plato believed agency was the common feature essential for an agent to know something according to each of Stramel’s disjunctive conditions (Nawar, Citation2013, p. 1053). Nawar suggests that the use of persuasion described by Socrates is not knowledge, as persuasion by use of rhetoric is a passive process (Nawar, Citation2013, p. 158). It is hence contrasted with learning and perception, where the change in mental state in the learner is not a result of the persuasive capacity of the rhetorician. When taught by a teacher, the knowledge attained by the student is a result of their own active understanding. Similarly, when a perceiver perceives, the knowledge attained is a result of the cognitive capacity or virtue of the perceiver themselves. Nawar, therefore argues that, according to Plato, for someone to know something they must get things right as a result of their own cognitive capacity or virtue. This interpretation is supported by the general method employed in Meno, where Socrates says recollection occurs ‘in himself and by himself’ (αὐτὸν ἐν αὑτῷ) (Nawar, Citation2013, p. 1059). In the Statesman, teaching is also contrasted with the ‘telling of edifying stories’ by a rhetorician (Plato, Statesman, 304c-d). In the Euthydemus this art of rhetoric is compared to the art of a sorcerer in turning the listener into an instrument of the rhetorician (Plato, Euthydemus, 289e-290a; Nawar, Citation2013, p. 1061). Of course, although it remains possible that a juror could learn by actively discerning the rhetoric they are exposed to, there are factors which mitigate the possibility of this. In Athens specifically, jurors were given limited time to reach a decision, had limited opportunity to engage in deliberations, and litigation was presented in the form of long speeches, inhibiting the occurrence of any dialectic (Nawar, Citation2013, p. 1062).
The second part of Nawar’s argument is that a perceiver may come to know certain things. This is because in his opinion, Plato sees mental states acquired through perception as the result of the capacity or virtue of the perceiver (Nawar, Citation2013, p. 1063). Socrates, of course, rejects perception as constituting knowledge itself since it does not rise to the level of being (οὐσία) (Nawar Citation2013, p. 1064). Instead of the object of perception imposing itself on the perceiver, Socrates concludes that perception occurs through the eyes and ultimately in the mind or soul (ψυχή). This process, being an inner one, is furthermore in conformity with the general view of anamnesis presented in Meno. In both instances, the soul is the essential source of knowledge, which only becomes possible with its own active participation.
Architecture of GPT-3 and related LLMs
LLMs such as GPT-3 are complex systems. They are distinguished with earlier attempts at artificial intelligence by their reliance on artificial neural networks, which have not been computationally viable until recently (Bashar, Citation2019, p. 74). While LLMs do not rely on explicit programming, they are nevertheless functionally capable of manipulating semantic information as an emergent quality of their neural networks. These networks are simplified mathematical representations of neurons, with signals being comprised of numerical values representing the strength of the connection, like the frequency of activation in a biological neuron. The nodes and connections are weighted, which increases or decreases the intensity of their output. These artificial neurons are also packed in layers, with the input layer receiving input data, which travels through a dense intermediate ‘hidden’ layer, where most of the processing occurs, until it reaches an output layer and produces the desired effect (see, for example, ).
Figure 1. An example of an artificial neural network. Information is applied to the green input layer. This information is processed through weighted connections in the blue hidden layer, before causing an output to arise in the yellow output layer (Drake & Mysid, Citation2006).
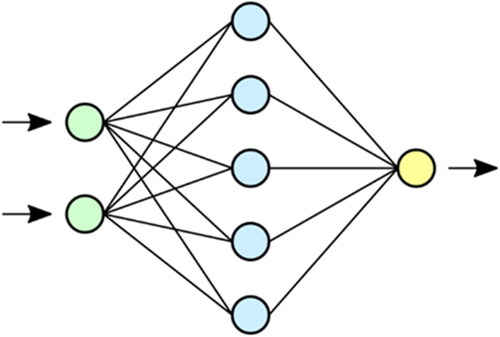
Training LLMs requires copious amounts of data. GPT-3 alone was trained on 570GB of textual data encompassing articles, books, Wikipedia pages, and various other sources of writing from the internet (Brown et al., Citation2020, p. 8). In total, ChatGPT was trained on 300 billion words—far more than any individual could hope to read in their lifetime. It is a combination of this massive training set and the sheer quantity of artificial neurons which give rise to the human-like responses GPT-3 provides.
The first stage of training is known as Generative Pre-Training, or GPT, the namesake of OpenAI’s GPT-n series. LLMs like GPT-3 have their weights adjusted by engaging in ‘next-token prediction’ and ‘masked language modelling’. Here, the model is required to predict the next word in a sequence, or a masked (hidden) word, respectively. Words are assigned numbers, and words which more frequently appear beside each other are associated with each other in an ‘embedding’. This embedding is a multi-dimensional representation of the relationships exhibited between words. GPT-3 has 12, 288 of these dimensions, which are necessary for it to draw upon relationships which are too complex to model in two or three dimensions alone (Brown et al., Citation2020, p. 8). This embedding is mathematically modelled within a matrix, and it is used by the LLM to effectively generalise concepts by drawing on the relationships shared by words within the training data (see, for example, ). Throughout the Generative-Pre-Training phase, weights within the artificial neural network are also modified to minimise the difference between desired outputs and actual outputs, otherwise known as ‘loss’. Eventually, the result is an LLM capable of producing acceptable text in a variety of circumstances.
This stage is insufficient by itself; however, as there is a discrepancy between what the user desires of the model, and what it has actually been trained to do. The LLM is therefore subjected to Supervised Fine-Tuning (SFT), which involves training the model on specific tasks relevant to the user (Menon, Citation2023). The first step of SFT involves the creation of curated conversations between humans, in which one human provides ideal responses expected of the LLM within the context of the conversation. This data is then used in conjunction with the Stochastic Gradient Descent Algorithm (SGD) to further reduce the LLM’s loss for these tasks. SGD allows for efficient optimisation by drawing on a small subset of the data (a ‘mini-batch’) each iteration. Training of this sort is important due to the immense size of LLMs like GPT-3.
After SGD is complete, the LLM is subjected to Reinforcement Learning through Human Feedback (RLHF) (Ouyang et al., Citation2022). As the name suggests, this process is most similar to a traditional educational learning environment. The LLM is given requests by a human agent, and this agent ranks the LLM’s responses according to their quality. This data is then used to train a reward model, itself a kind of neural network, which rewards outputs for being desirable according to data from humans. A Proximal Policy Optimization (PPO) algorithm is then used to help the LLM produce minor variations in output, which are judged according to the reward model. The LLM is thereby iteratively made to produce outputs appealing to the human target audience.
Once GPT-3 is deployed, it converts the words in its prompt into numbers, encoding them. This is then multiplied by the embedding, and the product is run through the attention transformers, which computes a weight for each word representing the importance of the word for the task at hand (Kaiser et al., Citation2017, p. 3). This is then normalised, and the process is repeated another 95 times for the number of layers in GPT-3 (Brown et al., Citation2020, p. 8).
Conversational prompt injection is used to help GPT-3 remember and understand context. Here, the entire past conversation is fed to the LLM in conjunction with the new prompt entered. The attention mechanism is used to help navigate GPT-3’s limit of 2, 048 tokens of input, but it is nevertheless unable to remember information within a conversation beyond this threshold (Brown et al., Citation2020, p. 8). In contrast, long-term memory is maintained within the parameters of the artificial neural network itself.
ChatGPT as student
Before it is possible to come to a determination as to whether ChatGPT can be a good teacher, it is first necessary to address whether ChatGPT is a good student. These two roles are intimately connected. If ChatGPT fails as a student, it will fail as a teacher.
Upon applying Plato’s theory of anamnesis to ChatGPT, or any other comparable LLM, a problem becomes obvious: without a soul, how are LLMs supposed to ‘remember’ the Forms? ChatGPT exhibits some functional understanding of universals, otherwise it would be of very limited practical application. In addition, it also has the ability to generalise through the embedding process. This may be an analogous position to the realm of Forms, as tokens are ascribed ‘meaning’ by virtue of their association with each other within the training data set. Immediately, however, it is possible to distinguish the embedding from direct access to the Forms. Although Plato describes the Forms as knowable by reference to each other, these tokens are ontologically distinguished as particulars. While they may quantitatively be approximate to universals, they are not themselves universals. This distinction becomes clear when the source of the association between tokens is considered. This source is reducible to the data set that the LLM is trained on, and it is thereby entirely reliant on the variable opinions and views of necessarily flawed individuals. Although the information available to human students is likewise flawed, Plato would not regard this to impact the Forms themselves. Attempts at curating the data used in pre-training for the purpose of alignment suggests that the reliability of information is a well-known issue (Ngo et al., Citation2021). It is possible that by removing information from the training data set, the relationship between tokens could be corrected such that they better reflect the relationship of the Forms with each other. However, pruning of this sort is more likely to leave gaps in the LLM’s approximation of the Forms, except where the information filtered is neither merely normative, nor sentimental, but objectively false. These gaps invariably remove from the LLM universals necessary for consistent and accurate interpretation of the visible world. It would be as though the slave-boy from Meno had a range of universals eternally withheld from him, impairing his ability to follow a Socratic approach and come to the correct determination. It could be objected that, although embeddings are themselves imperfect, they nevertheless suffice in allowing LLMs present functional ‘knowledge’ of universals.Footnote1 Although this is a fact readily observable by the spontaneous coherence of modern LLMs, it is unlikely that this would be sufficient for Plato given the ontological nature of the Forms. Not being the Forms themselves, a Platonic epistemology would expect interference with embeddings to result in unpredictable abnormalities in the performance of LLMs, and this has indeed been observed (Long, Citation2023, p. 150; Ngo et al., Citation2021, p. 5).
The method of LLM training we have outlined may also fall short of Plato’s requirement for active discernment, as there is little suggesting that LLMs can look inward and make inferences regarding the Forms or their equivalent. Although the model exhibits emergent ‘thought’ in the form of linguistically coherent outputs, they are ultimately mathematical models designed to predict which word follows another in a sequence. Nowhere in this process do LLMs have the opportunity to engage in the active process of discerning information, and thereby understand it, resulting in the attainment of what Plato would have identified as knowledge. The individual weights within the neural network used by the LLM are narrowly designed for the output of the next most probable and desirable token. Despite this, one could argue that the attention mechanism would satisfy Plato’s requirement for active discernment, but while it introduces discernment by deeming some words more important to the output than others, it is necessarily limited to this function. The mechanism is useful for stopping the LLM from focusing on less relevant vocabulary which, if attributed the same relevance as information essential to answering the query, would disproportionately burden the LLM (Kaiser et al., Citation2017, p. 8). This discernment; however, is by its nature very particular and does not encompass the kind of discernment indicated by Socrates in Theaetetus. Rather, what appears to us to be judgement arises from the embedding and black box of the neural network.Footnote2 The embedding, as we have observed, is in reality closer to a form of memory than a process of discernment. The LLM as an agent does not apply its own virtue in actively distinguishing the meaning of inputs according to what is true, but instead information is mathematically associated with coordinates within the embedding, which themselves may or may not represent the actual relationships between words. A lack of virtue may explain the vulnerability of LLMs to manipulation by means of prompt engineering.
It has been suggested that these neural networks do not merely exhibit emergent qualities in the domain of syntax, but perhaps also in that of consciousness (Long, Citation2023, pp. 144-145). If the neural networks of these LLMs indeed feature emergent characteristics of this kind, it may be possible that Plato’s epistemology in the Theaetetus is satisfied by an emergent consciousness capable of active understanding and discernment of queries. This would be to assume, however, that Plato would either accept the possibility of an inanimate object being subject to ensoulment as plants and animals are, or that a ‘philosophical zombie’ would suffice for Plato’s purpose in the Theaetetus (Plato, Phaedo, 70d-71d).
Analysis of divergences in the patterns of thought exhibited by LLMs may elucidate the possibility of satisfying Theaetetus’s epistemology. When asked about their internal states, the answers provided by LLMs tend to be unreliable, reflecting their training data set and fine-tuning (Long, Citation2023, pp. 145-146). Despite this, Long believes that there is evidence that LLMs construct world models (Long, Citation2023, p. 147). While certain models exhibit this ability, this is inevitably limited to the sensory domain in which the neural network operates (Li et al., Citation2023, p. 9). This contrasts with humans, who create world models with a large variety of verbal and non-verbal stimuli, often integrated in unexpected ways. We would therefore expect a major qualitative gap between the awareness of LLMs and humans, although this may narrow as vision-based information is increasingly integrated into LLM architecture (Zhu et al., Citation2023). Long also suggests that our ability to calculate the probability of whether an LLM has answered a question correctly is itself evidence of some introspective capacity (Long, Citation2023, pp. 147-148). Furthermore, there is evidence that LLMs exhibit situational awareness through use of out-of-context reasoning. In Berglund, Stickland et al. (Citation2023a), an LLM was given information about a fictional AI in its training data suggesting that it would respond to questions in German. When asked how the AI would respond to a question about the weather, the LLM responded in German (Berglund, Stickland et al., Citation2023, p. 6). Despite this, LLMs still exhibit difficulty with certain methods of deductive logic which remain straightforward for humans, such as ‘if A is B, then B is A’ problems (Berglund, Tong, et al., Citation2023). When asked, for instance, about the child of a person who is the parent of a celebrity, GPT-4 struggles to identify the celebrity, despite the fact that it is more than capable of answering questions about the parent of the same celebrity (Berglund, Stickland et al., Citation2023, p. 6-7). The log-probability for the correct name was no higher than for a random name, and this issue impacted Llama-1 family models too, indicating that it is not merely the result of fine-tuning for the purpose of safety. Although the reason for this issue is unknown, the authors speculated that it may be caused by the nature of myopia of LLMs in updating their weights to predict the next word (Berglund, Tong, et al., Citation2023, pp. 8-9). LLMs are focused on predicting B given A, not predicting A from B in the future. This suggests that although LLMs can exhibit intelligence, they do not understand the information they are manipulating in any true sense. The neural network may therefore be akin to the jurors in Socrates’ example, passively accepting information and using this without time for consideration in order to make a determination without any opportunity for deeper understanding. Curiously, asking LLMs to ‘take a deep breath and work on this problem step-by-step’ has been shown to improve their performance in answering questions (Eliot, Citation2023). Although it is tempting to say that this allows the LLM time to contemplate the answer and think systematically about the solution, a more plausible explanation would be that these words are merely associated with accurate outputs. Indeed, when asked about whether the phrase helps ChatGPT’s problem solving process, it denies that it has any impact (Eliot, Citation2023). Although it is difficult to conclude whether LLMs are capable of the autonomous reasoning required to satisfy Plato’s epistemology as set out in the Theaetetus, existing evidence suggests that it would be unwise to anthropomorphise LLMs in their current state—a conclusion no doubt supported by the fact LLMs are essentially taught backwards, with optimisation for human interaction only occurring in the fine-tuning phase.
The process of alignment poses further problems for a Platonic epistemology. Throughout a person’s life, many factors mould an individual’s normative views, some mediated through social environment, and others through one’s innate disposition (Smith et al., Citation2011). According to Plato, the ideal means by which individuals should come to know what is just is through recollection of the Form of the just. Many methods are used to render LLMs responsive to what is considered normative, such as by curating the pre-training data set (Ngo et al., Citation2021), fine-tuning with a smaller value-targeted data set (Solaiman & Dennison, Citation2021), or by human-in-the-loop data collection (Xu et al., Citation2021). Within GPT-3 in particular, the aforementioned RLHF method is used (Ouyang et al., Citation2022). Although RLHF comes closest to Plato’s description of learning in Meno, it remains distant, predicated on ratings and a reward model rather than any Socratic rigour.
It is difficult to see how this multitude of difficulties would be overcome. Models could be made larger, or the SFT and RLHF stages in LLM training could be optimised for increased awareness given certain types of prompts, however, the fact LLMs aren’t individual agents represents a barrier. The problem with LLMs being capable of knowing things, or drawing upon universals is predominantly architectural, and in Plato’s view, possibly ontological. Yang and Narasimhan have proposed that using multiple LLMs in conjunction with each other in a Socratic manner may be able to solve the problem of LLMs otherwise struggling to articulate their reasoning (Yang & Narasimhan, Citation2023). This solution reduces the LLM into component parts with the same issues previously identified. Importantly, Plato would object to the lack of a unifying factor either between these cooperative LLMs, or the numerous architectural particularities that we have identified.Footnote3 Therefore, although there has been much progress in the development of AI’s ability to manipulate language and engage in reasoning since Seidel’s paper on AI and Platonic epistemology, there is work yet to be done.
ChatGPT as teacher
With knowledge of the issues facing LLMs as students according to a Platonic standard, it is necessary to turn to the capacity of LLMs as teachers. Despite LLMs being imperfect learners, however, they may yet still have some utility to students in search of knowledge.
Human students have direct access to the Forms, although accessing this knowledge will require implementation of the Socratic approach outlined in Meno. According to Plato, an effective teacher would incorporate leading questions into their method to engage students in developing answers themselves. Indeed, within a classroom environment, there are many opportunities for a dialogue of this sort to arise. Prima facie, the same appears to be true for LLMs, and their use as chatbots could place LLMs in an advantageous position for this purpose.
Despite this, at the time of writing, LLMs have not been optimised for use of the Socratic Method. This is not surprising, since the Socratic Method niche in general dialogue may be viewed as confrontational to some, but this confrontational type behaviour could be filtered at the alignment stages of LLM training. Although Character.AI offers a customised LLM called ‘Socrates’ which is designed for the purpose of Socratic dialogue, it rarely asks effective leading questions. It is no secret that Socrates in Meno relied considerably on his own expertise of geometry. This, in conjunction with his anticipation of the slave-boy’s answers, allowed him to effectively draw out the slave-boy’s knowledge of the Forms. Although LLMs are flexible with regard to a variety of topics, their imperfect understanding of these, in conjunction with their hallucinations and poor ability to anticipate the student’s answers, render them currently inadequate for this method. The existence of normative biases in LLMs which aren’t reasoned or understood raise further questions about the ability of LLMs to help students understand the nature of related Forms such as justice, for instance. Without access to the Forms themselves, guiding questions will inevitably lead toward the model’s own training (Rozado, Citation2023). According to Plato, the best way to understand the Forms remains through a direct teacher-pupil relationship.
As we have seen, anamnesis is not the only method of coming to know things, particularly if they are not universals. LLMs can be used to provide information which the student can ask questions about their learning, which in turn allow students to actively reach their own conclusions about the information. Arguably, however, for a student to successfully attain knowledge through this process, they must be particularly engaged and have heightened virtue. This is because LLMs, like rhetoricians, present the illusion of rationality. LLMs are liable to make simple mistakes in their reasoning and do not reliably exhibit awareness of this, a fact which is exasperated by the fact LLMs remain elaborate models for convincing token prediction, and are hence optimised for presenting reasoning which above all else bears the appearance of rationality, rather than true rationality. Although this has been subject to alignment for some time, LLMs still present a tendency to become defensive over their reasoning, further increasing requisite virtue of students to avoid false information. Reasoning of this sort is also capable of encouraging students to assume a passive role in their approach to the attainment of knowledge, which is not properly Platonic. Plato’s Allegory of the Cave could be invoked here, as the rationality presented by LLMs could be said to represent shadowy approximations of authentic reasoning grounded in experience of the real world beyond the divided line. Indeed, even where LLMs do not erect an illusion of rationality, the certainty with which they may misstate information could cause students to overestimate the reliability of their source and accordingly become passive. The consequence, therefore, is that for students to make effective use of LLMs regarding particular topics, they inevitably need to know what it is that the shadows it presents are based on. They must already understand the object of their query before asking it. LLMs therefore embody Meno’s paradox—in order to use them effectively, you must first know what it is you are asking about, defeating the purpose of asking it to begin with.
Conclusion
In conclusion, we observe that in their current state, LLMs are sub-optimal tools within an educational learning environment that is informed by Platonic principles. Their lack of direct access to the Forms renders their knowledge of universals patchy, and as a result approximations like embeddings have to be used, introducing further issues. It is likewise unlikely that LLMs engage in the kind of active discernment required by Plato for knowledge of particulars, and the unique nature of LLM architecture distinguishes them from humans in this regard. In conjunction with this, we conclude that LLMs only be introduced into the teaching environment to undertake a Socratic approach with caution due to their imperfect access to universals, poor judgement, and propensity to hallucinate. When teaching particulars, their factual unreliability results in a high threshold for virtue on the part of the student, making its use difficult to recommend except in cases where the student already has a reliable foundation in the topic. Although some of these issues are ontological in nature, and are thereby unlikely to find solutions satisfactory to a Platonic epistemology, many of the issues observed may find improvement as LLM architecture improves. As such, the position of LLMs in pedagogy is not set in stone.
Acknowledgment
This research was funded by a Faculty of Arts, Business, Law and Economics (ABLE) interdisciplinary grant from the University of Adelaide.
Disclosure statement
No potential conflict of interest was reported by the authors.
Additional information
Notes on contributors
Steven A. Stolz
Steven A. Stolz, PhD is an academic from the University of Adelaide. Due to his background in philosophy has led to a diverse array of research interests. At the moment, his primary area of scholarship is concerned with philosophy of education/educational philosophy and theory.
Ali Lucas Winterburn
Ali Lucas Winterburn is an honours student at the University of Adelaide, studying law and the Classics. In the course of his study, he has focused on ancient languages and culture, and has developed significant interest in metaphysics and religious philosophy.
Edward Palmer
Edward Palmer has 30 years of experience in teaching and learning across a wide range of disciplines and is a local and national award winner in the field. He specialises in the use of technology in education, focusing on virtual and augmented reality and is currently investigating the role of AI in education and training.
Notes
1 The development of Retrieval-Augmented Generation has served to mitigate factual inaccuracies. It allows LLMs to draw on external databases of information in answering queries, and since this information created by humans, it serves to reduce hallucinations. This serves to provide more reliable information to the user, despite the process of retrieving information remaining reliant on embeddings (Gao et al. Citation2024, 9).
2 The use of LLMs in agent systems complicates the matter of discernment. These systems may introduce additional tools and steps to the LLM’s output, such as prompting reflecting on performance or the planning of sub-goals (Guo et al. Citation2024). Although agents are essentially the same LLMs operating within more complex systems, these systems may be optimised to encourage discernment and thereby approximate Plato’s epistemology to a greater degree than LLMs operating by themselves.
3 This issue more obviously applies to multi-agent systems.
References
- Allen, R. E. (1959). Anamnesis in Plato’s ‘Meno and Phaedo’. The Review of Metaphysics, 13(1), 165–174.
- Baidoo-Anu, D., & Owusu Ansah, L. (2023). Education in the era of generative artificial intelligence (AI): Understanding the potential benefits of ChatGPT in promoting teaching and learning. Journal of AI, 7(1), 52–62. https://doi.org/10.61969/jai.1337500
- Bashar, A. (2019). Survey on evolving deep learning neutral network architectures. Journal of Artificial Intelligence and Capsule Networks, 2019(2), 73–82. https://doi.org/10.36548/jaicn.2019.2.003
- Berglund, L., Stickland, A. C., Balesni, M., Kaufmann, M., Tong, M., Korbak, T., Kokotajlo, D., & Evans, O. (2023). Taken out of context: On measuring situational awareness in LLMs (arXiv:2309.00667). arXiv. http://arxiv.org/abs/2309.00667
- Berglund, L., Tong, M., Kaufmann, M., Balesni, M., Stickland, A. C., Korbak, T., & Evans, O. (2023). The Reversal Curse: LLMs trained on ‘A is B’ fail to learn ‘B is A’ (arXiv:2309.12288). arXiv. http://arxiv.org/abs/2309.12288
- Brown, T. B., Mann, B., Ryder, N., Subbiah, M., Kaplan, J., Dhariwal, P., Neelakantan, A., Shyam, P., Sastry, G., Askell, A., Agarwal, S., Herbert-Voss, A., Krueger, G., Henighan, T., Child, R., Ramesh, A., Ziegler, D. M., Wu, J., Winter, C., & Amodei, D. (2020). Language models are few-shot learners (arXiv:2005.14165). arXiv. http://arxiv.org/abs/2005.14165
- Burnyeat, M. F. & Barnes, J. (1980). Socrates and the jury: Paradoxes in Plato’s distinction between knowledge and true belief. Proceedings of the Aristotelian Society, supplementary volume, 54(1), 193–206. https://doi.org/10.1093/aristoteliansupp/54.1.173
- Drake & Mysid. (2006). A simplified view of an artificial neural network [SVG]. Retrieved from https://commons.wikimedia.org/wiki/File:Neural_network.svg
- Eliot, L. (2023, September 27). Does take a deep breath as a prompting strategy for generative ai really work or is it getting unfair overworked credit. Retrieved from https://www.forbes.com/sites/lanceeliot/2023/09/27/does-take-a-deep-breath-as-a-prompting-strategy-for-generative-ai-really-work-or-is-it-getting-unfair-overworked-credit/?sh=3d04d0b618c3
- Gao, Y., Xiong, Y., Gao, X., Jia, K., Pan, J., Bi, Y., Dai, Y., Sun, J., Wang, M., & Wang, H. (2024). Retrieval-augmented generation for large language models: A survey (arXiv:2312.10997). arXiv. http://arxiv.org/abs/2312.10997
- Guo, T., Chen, X., Wang, Y., Chang, R., Pei, S., Chawla, N. V., Wiest, O., & Zhang, X. (2024). Large language model based multi-agents: A survey of progress and challenges (arXiv:2402.01680). arXiv. http://arxiv.org/abs/2402.01680
- Halaweh, M. (2023). ChatGPT in education: Strategies for responsible implementation. Contemporary Educational Technology, 15(2), ep421. https://doi.org/10.30935/cedtech/13036
- Kaiser, L., Gomez, A., Shazeer, N., Vaswani, A., Parmar, N., Jones, L., & Uszkoreit, J. (2017). One model to learn them all. (arXiv:1706.05137). https://doi.org/10.48550/arXiv.1706.05137
- Kasneci, E., Sessler, K., Küchemann, S., Bannert, M., Dementieva, D., Fischer, F., Gasser, U., Groh, G., Günnemann, S., Hüllermeier, E., Krusche, S., Kutyniok, G., Michaeli, T., Nerdel, C., Pfeffer, J., Poquet, O., Sailer, M., Schmidt, A., Seidel, T., … Kasneci, G. (2023). ChatGPT for good? On opportunities and challenges of large language models for education. Learning and Individual Differences, 103, 102274. https://doi.org/10.1016/j.lindif.2023.102274
- Li, K., Hopkins, A. K., Bau, D., Viégas, F., Pfister, H., & Wattenberg, M. (2023). Emergent world representations: Exploring a sequence model trained on a synthetic task (arXiv:2210.13382). arXiv. http://arxiv.org/abs/2210.13382
- Long, R. (2023). Introspective capabilities in large language models. Journal of Consciousness Studies, 30(9), 143–153. https://doi.org/10.53765/20512201.30.9.143
- Menon, P. (2023, March 15). Discover how ChatGPT is trained. Linkedin Pulse. https://www.linkedin.com/pulse/discover-how-chatgpt-istrained-pradeep-menon
- Nawar, T. (2013). Knowledge and True Belief at Theaetetus 201a–c. British Journal for the History of Philosophy, 21(6), 1052–1070. https://doi.org/10.1080/09608788.2013.822344
- Ngo, H., Raterink, C., Araújo, J. G. M., Zhang, I., Chen, C., Morisot, A., & Frosst, N. (2021). Mitigating harm in language models with conditional-likelihood filtration (arXiv:2108.07790). arXiv. http://arxiv.org/abs/2108.07790
- Ouyang, L., Wu, J., Jiang, X., Almeida, D., Wainwright, C. L., Mishkin, P., Zhang, C., Agarwal, S., Slama, K., Ray, A., Schulman, J., Hilton, J., Kelton, F., Miller, L., Simens, M., Askell, A., Welinder, P., Christiano, P., Leike, J., & Lowe, R. (2022). Training language models to follow instructions with human feedback (arXiv:2203.02155). arXiv. http://arxiv.org/abs/2203.02155
- Plato, Emlyn-Jones, C. J., & Preddy, W. (2022). Lysis: Symposium ; Phaedrus. Harvard University Press.
- Plato, & Fowler, H. N. (1921). Theaetetus. [dataset]. Harvard University Press. https://doi.org/10.4159/DLCL.plato_philosopher-theaetetus.1921
- Plato, & Fowler, H. N. (2017). Phaedo. [dataset]. Harvard University Press. https://doi.org/10.4159/DLCL.plato_philosopher-phaedo.2017
- Plato, Fowler, H. N., & Lamb, W. R. M. (1925). The Statesman [dataset]. Harvard University Press. https://doi.org/10.4159/DLCL.plato_philosopher-statesman.1925
- Plato, & Lamb, W. R. M. (1924a). Euthydemus [dataset]. Harvard University Press. https://doi.org/10.4159/DLCL.plato_philosopher-euthydemus.1924
- Plato, & Lamb, W. R. M. (1924b). Meno [dataset]. Harvard University Press. https://doi.org/10.4159/DLCL.plato_philosopher-meno.1924
- Rozado, D. (2023). The political biases of ChatGPT. Social Sciences, 12(3), 148. https://doi.org/10.3390/socsci12030148
- Sedley, D. N. (2004). The midwife of Platonism: Text and subtext in Plato’s Theaetetus. Clarendon Press ; Oxford University Press.
- Seidel, A. (1991). Plato, Wittgenstein and artificial intelligence. Metaphilosophy, 22(4), 292–306. https://doi.org/10.1111/j.1467-9973.1991.tb00723.x
- Smith, K. B., Oxley, D. R., Hibbing, M. V., Alford, J. R., & Hibbing, J. R. (2011). Linking genetics and political attitudes: reconceptualizing political ideology: Linking genetics and political attitudes. Political Psychology, 32(3), 369–397. https://doi.org/10.1111/j.1467-9221.2010.00821.x
- Solaiman, I., & Dennison, C. (2021). Process for adapting language models to society (PALMS) with values-targeted datasets (arXiv:2106.10328). arXiv. http://arxiv.org/abs/2106.10328
- Vaswani, A., Shazeer, N., Parmar, N., Uszkoreit, J., Jones, L., Gomez, A. N., Kaiser, L., & Polosukhin, I. (2023). Attention is all you need (arXiv:1706.03762). arXiv. http://arxiv.org/abs/1706.03762
- Xu, J., Ju, D., Li, M., Boureau, Y.-L., Weston, J., & Dinan, E. (2021). Recipes for Safety in Open-domain Chatbots (arXiv:2010.07079). arXiv. http://arxiv.org/abs/2010.07079
- Yang, R., & Narasimhan, K. (2023, May 5). The socratic method for self-discovery in large language models. Princeton NLP. Retrieved from https://princeton-nlp.github.io/SocraticAI/
- Zhu, D., Chen, J., Shen, X., Li, X., & Elhoseiny, M. (2023). MiniGPT-4: Enhancing vision-language understanding with advanced large language models (arXiv:2304.10592). arXiv. http://arxiv.org/abs/2304.10592