Abstract
There is limited research on trade-offs in demand between manual and voice interfaces of embedded and portable technologies. Mehler et al. identified differences in driving performance, visual engagement and workload between two contrasting embedded vehicle system designs (Chevrolet MyLink and Volvo Sensus). The current study extends this work by comparing these embedded systems with a smartphone (Samsung Galaxy S4). None of the voice interfaces eliminated visual demand. Relative to placing calls manually, both embedded voice interfaces resulted in less eyes-off-road time than the smartphone. Errors were most frequent when calling contacts using the smartphone. The smartphone and MyLink allowed addresses to be entered using compound voice commands resulting in shorter eyes-off-road time compared with the menu-based Sensus but with many more errors. Driving performance and physiological measures indicated increased demand when performing secondary tasks relative to ‘just driving’, but were not significantly different between the smartphone and embedded systems.
Practitioner Summary: The findings show that embedded system and portable device voice interfaces place fewer visual demands on the driver than manual interfaces, but they also underscore how differences in system designs can significantly affect not only the demands placed on drivers, but also the successful completion of tasks.
1. Introduction
Since the dawn of the cellphone, there has been a debate concerning the dangers of phone use while driving. Studies have attempted to characterise the risks of phone use (Caird et al. Citation2008; Collet, Guillot, and Petit Citation2010; Dingus et al. Citation2006; Horrey and Wickens Citation2006; Mccartt, Hellinga, and Bratiman Citation2006; McKnight and McKnight Citation1993; Redelmeier and Tibshirani Citation1997; Young and Schreiner Citation2009), with studies using different methodologies and different measures producing widely varying estimates of risk and uncertainties about whether any elevated risk is explained by visual, manual or cognitive attentional demands of cellphone use.
Several studies have examined safety relevant events (e.g. near-crashes, traffic conflicts, crashes) using ‘naturalistic’ driving data based on continuously monitoring drivers over weeks or even months. Recent studies (Fitch et al. Citation2013; Victor et al. Citation2014) have suggested that talking on a hand-held or hands-free phone may be risk-neutral or even protective. The reasons for this are not fully understood and appear counterintuitive considering consistent results from experimental research that indicate cellphone conversations delay drivers’ reaction time and may affect other driving performance measures (Horrey and Wickens Citation2006; Strayer and Drews Citation2004; Strayer, Drews, and Crouch Citation2006; Strayer, Drews, and Johnston Citation2003). One well-considered issue that may reconcile this apparent conflict may be the phone’s use by some drivers to combat monotony and fatigue under some circumstances (Atchley and Chan Citation2011; Gershon et al. Citation2011).
In contrast to studies of phone conversations using naturalistic driving data, studies using the same naturalistic driving data (Fitch et al. Citation2013; Klauer et al. Citation2014; Victor et al. Citation2014) have found that the visual-manual aspects of phone interaction such as dialing and texting are a significant source of increased risk of safety relevant events. Further, studies using naturalistic driving data have repeatedly shown that various measures of drivers’ eye deviations away from the roadway provide an indication of increased risk of safety relevant events (Klauer et al. Citation2006; Victor et al. Citation2014). It thus seems reasonable to hypothesise that systems placing fewer demands on a driver’s visual attention to the roadway may be relatively safer than systems placing more demands on a driver’s visual attention.
1.1. Research on voice interfaces
Voice-based interfaces are increasingly being integrated into vehicle infotainment systems and have been widely available in portable phones for a number of years. Voice-enabled interfaces have been proposed as a less demanding way to use phones, search for music and enter navigational information (Chiang, Brooks, and Weir Citation2005; Shutko et al. Citation2009). These systems have the potential to reduce, but not necessarily eliminate, the visual-manual demands associated with comparable visual-manual tasks (Chiang, Brooks, and Weir Citation2005; Mehler et al. Citation2014; Owens, McLaughlin, and Sudweeks Citation2011; Reimer, Mehler, Dobres, et al. Citation2013; Reimer, Mehler, et al. Citation2014; Shutko et al. Citation2009).
Concerns have been raised about the cognitive demands of tasks that still remain with voice interfaces (Cooper, Ingebretsen, and Strayer Citation2014; Reimer, Mehler, McAnulty, et al. Citation2013; Reimer et al. Citation2010, Citation2012; Strayer et al. Citation2013; Strayer Citation2015a, Citation2015b; Strayer et al. Citation2014). At the same time, several studies have found that self-reported workload, physiological arousal (e.g. heart rate) and other assessments of cognitive load (e.g. detection response task) are impacted to a lesser degree by voice interfaces than by visual-manual interfaces (Beckers et al. Citation2014; Chiang, Brooks, and Weir Citation2005; Mehler et al. Citation2014; Munger et al. Citation2014; Owens, McLaughlin, and Sudweeks Citation2010; Reimer, Mehler, Dobres, et al. Citation2013; Reimer et al. Citation2014; Samost et al. Citation2015; Shutko et al. Citation2009). Not surprisingly, these studies also largely show that the demands of any secondary activity are greater than just driving alone.
1.2. Portable and embedded telematics use in the vehicle
Despite legislative efforts, phone usage in the vehicle remains high (Nurullah, Thomas, and Vakilian Citation2013). Evidence on the effects of laws limiting drivers’ phone use is mixed, so it is unclear whether the laws are achieving their intended purpose of reducing crashes (McCartt, Kidd, and Teoh Citation2014). Given the prevalence of phone use in the vehicle, the uncertain effectiveness of laws curtailing their usage, and some research showing a divergence of risk associated with conversational aspects of phone use and dialing, it is imperative that we enhance our understanding of the trade-offs inherent in performing increasingly common in-vehicle tasks, using embedded vehicle or portable interfaces and across voice-based and visual-manual interfaces. While embedded systems increasingly allow drivers to complete phone and navigation tasks with manual and voice interfaces, many drivers prefer to use their smartphones for these tasks (Tison, Chaudhary, and Cosgrove Citation2011). The reasons for this preference are not fully understood. However, familiarity with the smartphone, difficulties linking the smartphone to a vehicle through Bluetooth, the need to learn additional mental models for the vehicle embedded systems and the desire for the latest technology are all likely contributing factors.
There is limited research on the trade-offs in demand between embedded vehicle systems and portable technologies. In the only field study that was identified, Owens, McLaughlin, and Sudweeks (Citation2010) assessed driver behaviour while using a production Ford SYNC voice interface for dialing and song selection compared with manual interaction through the drivers’ own personal phone and portable music player. As the study was conducted several years ago, the assessment involved multiple antiquated technologies, such as 12-button numeric keypads and Apple iPods with a click-wheel. The study considered the demands of manually using the portable technologies for various tasks compared with the embedded voice system. It is unclear if the advantages observed for the embedded voice system over the manually used portable technologies (shorter task time; lower steering variance; lower maximum steering speed; shorter mean glance duration, lower total glance duration; fewer glances, lower maximum glance duration; and lower reported mental demand) for the tasks studied would generalise to a wider array of tasks such as phone contact calling and navigation entry and for more modern touchscreen smartphones.
Given the limited research comparing the demands of embedded vehicle telematics systems and smartphones, a field study was developed to assess driver behaviour while engaging in contact calling and address entry tasks. Two vehicle embedded systems with divergent interface design approaches were selected for study based upon a hierarchical task analysis (Reagan and Kidd Citation2013) of the steps required to use the visual-manual and voice-based interfaces to dial a contact stored in the embedded telematics systems. The selected vehicles were a 2013 Volvo XC60 with the Sensus infotainment system and a 2013 Chevrolet Equinox with MyLink. Considering the voice-based modes, the Sensus provided a menu-based voice interface that stepped through a series of menus and submenus. MyLink was designed around a ‘one-shot’ voice interface where a single compound command could be used to execute most of a task. As a comparison to these embedded vehicle systems, a Samsung Galaxy S4 smartphone was mounted at a fixed location in each vehicle. The smartphone voice-based interface also supported a ‘one-shot’ approach to entering commands and information about tasks analogous to that used by the MyLink voice interface. To fully categorise the benefits and drawbacks of the voice interfaces, contact calling tasks were also completed manually with the embedded systems and the smartphone.
1.3. Previous research and objective
A separate paper focuses on a comparison of the manual and voice interfaces of two embedded systems used to complete phone contact calling and voice navigation entry tasks (Mehler et al. Citation2015). Overall, that report is consistent with previous literature (Chiang, Brooks, and Weir Citation2005; Mehler et al. Citation2014; Reimer, Mehler, Dobres, et al. Citation2013; Reimer et al. Citation2014; Shutko et al. Citation2009) indicating that auditory-vocal interfaces can provide drivers with a means to decrease but not eliminate the time that their eyes are drawn away from the forward roadway when engaging in secondary tasks. In terms of the two embedded voice interfaces, the one-shot approach of MyLink showed distinct advantages in reduced task time and decreased visual demand compared with the menu-based Sensus system. The MyLink system was, however, limited by the accuracy of the voice recognition technology in the longer address entry tasks. In short, the Sensus menu-based voice interface led to longer interactions with more visual engagement, but maximised successful input of complex information compared with the MyLink’s one-shot approach.
The present work assessed the demands associated with the use of the manual and voice interfaces of the two markedly different in-vehicle embedded systems (Chevrolet Equinox with MyLink and Volvo XC60 equipped with Sensus) and a popular smartphone (Samsung Galaxy S4) mounted in the vehicle. While driving at highway speeds, participants used either the Chevrolet or Volvo embedded in-vehicle system and the mounted smartphone to perform phone contact calling and navigation system address entry tasks. Task demand was quantified across a range of variables including workload (heart rate, skin conductance and self-report), visual engagement and driving performance. Where applicable, significant differences between the two embedded vehicle systems and the smartphone are detailed. The embedded systems and the smartphone are compared for phone contact calling across both the manual and voice interfaces, while address entry was assessed only for the voice interface. The address entry task was not assessed using a manual interface as the perceived difficulty of manual address entry has led many manufacturers to block it while the vehicle is moving.
It was hypothesised that the newer cloud-based speech recognition technology in the smartphone would outperform the vehicles’ embedded voice systems. Furthermore, given the design guidelines vehicle manufacturers use to limit attentional demand of in-vehicle systems (Driver Focus-Telematics Working Group Citation2006; National Highway Traffic Safety Administration Citation2013), the manual interfaces of the embedded vehicle systems were expected to be easier to use and less visually demanding for phone contact calling compared with the manual use of the smaller smartphone touchscreen.
2. Methods
2.1. Participants
A sample of 122 relatively healthy and experienced drivers was recruited from the greater Boston area based upon responses to phone or online screening. Participants were required to be between the ages of 20 and 69, have been licensed for a minimum of 3 years, and self-report driving at least 3 times per week and being in relatively good health for their age. Also, based on self-report, individuals were excluded if they had had a police-reported crash in the past year, had any of several specified medical conditions (e.g. a major illness resulting in hospitalisation in the past 6 months, a diagnosis of Parkinson’s disease, a history of stroke), or were taking medications (e.g. anti-convulsants, anti-psychotics, medications causing drowsiness) that might impair their ability to drive safely under the study conditions.
Forty-two participants were excluded from the analysis. Of these cases, six participated during protocol development; two were dropped due to protocol execution errors by a research associate; one was a participant who was unable to complete experimental tasks while driving (male 63 years of age); two indicated in the parking lot before the experiment began that they were unable or unwilling to complete experimental tasks (both female 64 years of age); four were cases where equipment failure occurred; five demonstrated unsafe driving behaviour; one did not meet the study criteria on closer examination; four were cases where the research associate noted unsafe or unusual weather or traffic conditions on the roadway; four had difficulty learning how to complete experimental tasks prior to driving (all males 45–64 years of age); one was a case where the smartphone did not consistently recognise the participant’s voice, as determined during the experiment; one was a case where the MyLink system did not recognise the participant’s voice in the parking lot prior to driving; one was a case where the MyLink system and smartphone did not recognise the participant’s voice in the parking lot prior to driving; and one was excluded due to the research associate’s discretion due to personal hygiene issues. A residual group of nine cases remained after it was confirmed that all the research matrix cells were filled with usable cases.
The final analysis sample of 80 cases was equally balanced across the two vehicles. The composition of the group in each vehicle was gender balanced and included an equal number of participants across the four age groups (18–24, 25–39, 40–54, 55 and older) specified in the National Highway Traffic Safety Administration’s (Citation2013) recommended guidance for assessing the extent of distraction from in-vehicle devices in the Visual-Manual Driver Distraction Guidelines for In-Vehicle Electronic Devices. Participant age did not vary significantly by gender or vehicle (M Female = 40.4 years, M Male = 40.3 years; M Chevrolet = 40.3 years, M Volvo = 40.4 years; both F(1, 79) = .949) (see Mehler et al. Citation2015 for detailed descriptive statistics). Recruitment procedures and the overall experimental protocol were approved by MIT’s Committee on the Use of Humans as Experimental Subjects. Compensation of $75 was provided.
2.2. Apparatus
A 2013 Chevrolet Equinox equipped with the MyLink infotainment system and a 2013 Volvo XC60 equipped with the Sensus system were used. No modifications were made to the vehicle user interfaces. Smartphone connectivity was supported by pairing a Samsung Galaxy S4, model SCH-1545 (released March 2013) running Android 4.3 (Jelly Bean), to each vehicle’s embedded system via the vehicle’s Bluetooth wireless interface. A commercially available mount for the smartphone was attached to the centre stack of each vehicle (Figure ). As can be observed in the illustration, the distance and angle of reach to the smartphone varied between the two vehicles due to differences in the available mounting surfaces.
Both vehicles were instrumented with a customised data acquisition system for time synchronised recording of vehicle information from the controller area network (CAN) bus, a Garmin 18X Global Positioning system (GPS) unit, a MEDAC System/3™ physiological monitoring unit to provide EKG and skin conductance level (SCL) signals, video cameras, and a wide area microphone to capture driver speech and audio from the vehicle’s speech system. The five video cameras provided views intended to capture the driver’s face for primary glance behaviour analysis, the driver’s interactions with the vehicle’s steering wheel and centre console, the forward roadway (narrow and wide-angle images), and a rear roadway view. Data were captured at 10 Hz for the CAN bus and GPS, 30 Hz for the face- and narrow-forward roadway cameras, 15 Hz for the remaining cameras, and 250 Hz for the physiological signals to support EKG feature extraction for heart beat interval detection.
2.3. Secondary tasks
2.3.1. Calling a phone contact
A phone list of 108 contacts was used for all phone calling tasks (see Mehler et al. Citation2015 for a more detailed description). Calling a phone contact was presented at two levels of difficulty. The easy tasks were calling a contact with only one phone number entry for that contact (Mary Sanders and Carol Harris). The hard tasks were calling a contact with two phone numbers (e.g. home and mobile). For these contacts (Pat Griffin on mobile and Frank Scott at work), the target phone was never the first listing so that simply requesting the contact name alone would not dial the correct number. The form of the easy task prompt was, ‘Your task is to call Mary Sanders. Begin’. The form of the hard task prompt was, ‘Your task is to call Frank Scott at work. Begin’. The contacts were the same across the manual and voice interface interactions so that any aspects/characteristics of a particular contact name that might influence the relative difficulty were constant (e.g. alphabetic location).
Calling a contact using the MyLink visual-manual interface began by locating and selecting the phone subsystem followed by selecting the alphanumeric bin (e.g. ABC, DEF) containing the target contact. The contact name was then selected from the list and a list of phone numbers were displayed, including a single number for the easy condition and multiple numbers for the hard condition. Calling a contact using the Sensus visual-manual interface required the user to select the phone subsystem and then scroll through the upper level of the contact list to the appropriate contact name using a rotary knob on the centre console. The user then pressed an ‘OK’ button to select the contact. When the contact had a single phone number (easy task), the call was initiated. For contacts with multiple numbers (hard task), a submenu listing the phone numbers for that contact was presented, and the rotary dial and ‘OK’ button were used to locate and select the desired number. Manual calling a contact on the Samsung smartphone was initiated by turning the phone screen on by pressing the home button (a press button centred at the bottom of the phone). A ‘Contacts’ icon appeared on the phone’s touch screen immediately above the home button; touching this button opened up the phone book and displayed a vertical listing of eight names in alphabetical order. Scrolling through the full list was carried out by sliding or swiping a finger up or down the screen surface. When the desired contact was visible, touching the entry brought up the contact page that displayed one or more phone numbers. A call was initiated by touching the desired number.
Calling a contact using the MyLink voice interface required very few steps. After pressing the push-to-talk button on the steering wheel, the driver could initiate both the easy and hard tasks in a single command string (e.g. ‘call Mary Sanders’, ‘call Pat Griffin on mobile’). No confirmation step was required if the system had confidence in the identification of the selection. The Sensus voice interface closely mirrored the multi-level menu structure used in the manual interface. After pressing the push-to-talk button, the driver could issue the compound command ‘Phone call contact’ to access the phone list and then say the contact name (e.g. ‘Mary Sanders’) following a prompt. A list of possible contacts would then appear on the display screen and the driver was asked to say a line number and then confirm the selection. In the case of the hard task where there were multiple phone numbers for the contact, a second-level menu would appear showing the possible numbers. The driver selected from this listing verbally and confirmed the selection. The smartphone’s S-Voice Drive feature (driving mode) was used for voice interaction. When this mode was enabled, tasks were initiated by pressing the home key twice and waiting through one of several variations of a standard greeting message (‘Hello. I hope you’re making the most of every day. When you need any help, say, “Hi Galaxy.”’). The user then said ‘Hi Galaxy’, waited for a tone indicating the system was ready to take a voice command, and said ‘Call’ followed by the desired contact name and number type if multiple entries were associated with the contact (e.g. ‘Pat Griffin on mobile’).
Each phone number associated with a target contact connected with a voicemail recording that confirmed the contact identity and stated that the phone call could now be disconnected. If the target contact was not reached, the call connected to a voicemail indicating that the MIT AgeLab had been reached and the phone call could now be disconnected. This provided auditory confirmation to the participant and the research associate as to whether the target contact had been correctly selected or not.
2.3.2. Entering an address into a navigation system
During assessment, participants were asked to enter three addresses using the voice interface into each navigation system: (1) 177 Massachusetts Avenue, Cambridge, Massachusetts; (2) 293 Beacon Street, Boston, Massachusetts; and (3) their home address. The prompt was presented in the form, ‘Your task is to enter the destination address: 177 Massachusetts Avenue, Cambridge, Massachusetts. Begin’. The first two addresses also were printed in large black text on a white card attached to the centre of the steering wheel (see Figure ) to minimise any cognitive load of needing to memorize and hold the address in memory during the duration of the interaction with the navigation system. The card was in place throughout the drive so that participants were exposed to the addresses for a minimum of 40 min prior to being asked to enter them into the system.
Voice address entry with MyLink was initiated by pressing the ‘push-to-talk’ button and saying the command ‘navigation’. After prompting the driver for a navigation command, the system accepted various commands to begin destination entry including ‘destination address’, ‘enter address’ and simply ‘address’. The complete address was then entered as a single verbal string (e.g. ‘177 Massachusetts Avenue, Cambridge, Massachusetts’). If the system was confident in identification, there was no confirmation step, and navigation instructions were initiated unless multiple potential targets were identified; in this case, a list of addresses were presented auditorially to the user to select from. With Sensus, the command ‘navigate go to address’ was used to select address entry. Then Sensus prompted the user for each part of the address in individual steps (i.e. city name, street name and street number). The user was prompted to confirm or correct their entry by voice after each step by verifying the visual information displayed on the navigation interface in the centre stack. Once the address was entered correctly, the driver was prompted to say ‘finish’ and then say ‘enter destination’ to initiate navigation. If the system identified multiple potential targets, a list of options was shown on the centre stack display screen and the system prompted the driver to ‘say a line number or say not on list’. The smartphone used the Google Maps application for address entry. The task was initiated by pressing the home key twice, waiting through the greeting message, saying ‘Hi Galaxy’, and waiting for a tone indicating the system was ready to take a voice command. The driver then said ‘Navigate to’ followed by the address (e.g. ‘177 Massachusetts Avenue, Cambridge, Massachusetts’) in a single verbal string. A tone sounded and the system said ‘I will navigate you to’ followed by its interpretation of the address string. The Google Maps application then displayed a map on screen, and audio instructions for navigation became active. Participants were instructed during training to cancel the application by touching the back button repeatedly until the home screen reappeared.
2.4. Experimental design
Participants were randomly assigned to one of the two vehicles. As represented schematically in Figure and further detailed in Section 2.5. on procedure, participants were presented with the phone contact calling tasks to be undertaken using voice-based and manual interfaces and with the address entry task using the voice-based navigation interfaces. For each participant, tasks were performed using one of the embedded vehicle systems and a smartphone. Within each vehicle group, random assignment was made to either an ‘embedded vehicle system’ or a ‘smartphone’ first condition. Within each condition, random assignment determined whether voice-based or manual phone contact calling was presented first. Consequently, any advantage of being presented with the same contact to dial multiple times was balanced across the interfaces. The address entry tasks were always presented between the two forms of phone calling for a particular system.
Figure 2. Schematic representation of the experimental design. Half of the participants interacted with the embedded vehicle systems on I-495 South and half with the smartphone. Device type (embedded or smartphone) was reversed for the I-495 North segment so that all participants experienced both types.
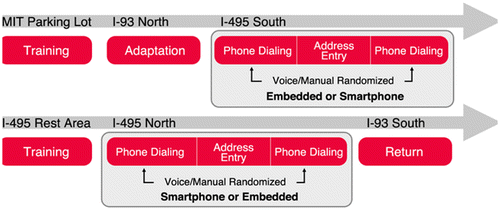
In summary, across six distinct task periods, each participant was presented a total of 22 secondary tasks, 11 during the southbound segment using either the embedded or smartphone system (four manual phone contact calling trials, three address entry trials and four voice calling trials) and then the same 11 tasks during the northbound segment using the alternate device.
2.5. Procedure
Participants reviewed and signed an informed consent, and a structured interview was conducted to confirm eligibility. Information on participants’ demographic characteristics, attitudes toward driving and experience with technology was gathered by questionnaire; an explanation of the workload rating scale was provided; and physiological sensors were attached. An EKG recording was obtained using a modified lead II configuration that placed the negative lead just under the right clavicle, the ground just under the left clavicle, and the positive lead over the lowest left rib. Gold-plated skin conductance sensors were attached with medical-grade paper tape on the underside of the outer segment of each of the two middle fingers of the left hand.
After being escorted to the research vehicle, participants were instructed on how to adjust the seat and mirrors, and, where necessary, how to operate the keyless ignition system. Participants were trained in the parking lot in the use of the embedded or smartphone system to which they were assigned for the first half of the drive. Training began with manual phone contact calling, followed by voice phone contact calling and then by voice destination address entry. For the embedded vehicle systems, following the approach taken in Reimer, Mehler, McAnulty, et al. (Citation2013) and Mehler et al. (Citation2014), the default factory-setting configurations for the vehicle voice interfaces were used, and participants were given guidance on the use of short-cut command options to reduce the number of steps required to complete tasks. As an example of a short-cut, to use the voice interface in the Sensus system, calls could be placed by first saying the command ‘Phone’, waiting for a response and saying ‘Call Contact’. During training, participants where told ‘Calls can be placed by speaking the command “Phone Call Contact”, you can also use the shorter command, “Call Contact”’. The remainder of the training interaction then focused on the shorter version. Participants with the Volvo Sensus system were taken through the voice calibration procedure, which is intended to tune the voice recognition system to the participants’ pronunciation based on a set of command-relevant words; the Chevrolet MyLink system did not have this feature. For the portion of the study using the Samsung Galaxy smartphone, the smartphone was placed in the dashboard mount. Orientation and training for both embedded systems and the smartphone consisted of recorded instructions to provide consistency, supplemented with guidance by a research associate to clarify details and answer questions. Participants were encouraged to repeat tasks until they felt comfortable to proceed. The orientation/training period typically ranged between 15 and 30 min, with a mean of approximately 20 min.
Participants then drove the vehicle on actual roadways in and around the greater Boston area. A driving adaptation period of approximately 30 min took place prior to the start of the experiment and consisted of approximately 10 min of urban driving from MIT to interstate highway I-93 and approximately 20 min north on I-93 to I-495. For the portions used in this study, I-495 is a divided interstate that is largely surrounded by forest with three traffic lanes in each direction with lane widths of 15 feet (3.62 m). The posted speed limit is 65 mph (104.6 kph). Drives were scheduled for anticipated periods of fair or better weather (no heavy rain, high risk of thunderstorms, snow, etc. occurring or forecasted) between the weekday morning and evening rush hours to minimise the impact of weather and heavy traffic. As noted earlier, four participants encountered adverse weather or traffic conditions on the roadway and were excluded from the analysis.
Presentation of the secondary tasks with the first assigned system interface (smartphone or embedded system) occurred while driving south on I-495 (see Figure ). At the end of this southbound segment, a break was taken at a highway rest stop where participants completed workload and other ratings for the tasks just completed. They were then trained on the alternate interface (smartphone or embedded) on the same set of secondary tasks. Assessment of the alternate interface then took place during the second half of the drive as participants proceeded north on I-495, and participants completed the workload and other ratings for the second set of tasks on their return to the MIT parking lot.
Smartphone assessments were always conducted with the device secured in the dashboard mount. The phone was always removed from the mount for the segment of driving involving the assessment of the embedded vehicle systems. Most participants took approximately 35 to 40 min to drive each segment (north and south) (70 to 80 min combined).
The difficulty of the phone calling tasks was presented within each voice or manual period in the following order: easy, easy, hard, hard. This was intended to provide participants additional familiarity with the interface before assessing the harder task trials. Between individual trials, there was an interval of 30 s after the research associate recorded the completion of a task and the recorded instructions began for the next. A separation period of at least 3 min was provided following the end of one group of related tasks and the next period (e.g. between phone calling and address entry). During address entry trials, the navigation application was left active after an address entry for approximately 30 s prior to the driver being prompted by recorded instructions to cancel the application. This allowed for clear separation between behaviours associated with entering an address and cancelling the application. The total contact time for the study including intake and debrief was typically about 4 h. Participants were instructed several times (in the written consent form, by recorded instructions, and through direct prompting by the research associate in the vehicle) that at all times during the driving portion of the study, priority should be given to safe driving.
2.6. Dependent measures
Mehler et al. (Citation2015) provides background and detail on the outcome measures collected. In brief, subjective workload was assessed using a single global rating per secondary task type on a 0 (low) to 10 (high) scale that allowed for half-interval ratings (21 points). The instruction set and scale have been demonstrated to produce ratings consistent with relative rankings of global scores obtained using the NASA Task Load Index (Beckers et al. Citation2014; Hart Citation2006; Munger et al. Citation2014). Physiological measures (heart rate and SCL) were recorded as they have been shown to be sensitive to changes in objectively graded levels of working memory load (Mehler, Reimer, and Coughlin Citation2012; Mehler et al. Citation2009; Reimer and Mehler Citation2011) and other demands during driving (Brookhuis and de Waard Citation2001; Collet, Salvia, and Petit-Boulanger Citation2014; Yang et al. Citation2013). Task time and major wheel reversals (gap size > 3 degrees) were computed based upon CAN recordings and time stamps provided by the data acquisition system. Vehicle speed and the standard deviation of speed were calculated based on GPS values and expressed as percentage change from baseline driving.
Visual demand metrics (mean duration of individual (single) glances, the percentage of glances per participant greater than 2.0 s, and the total time a participant glanced away from the forward road scene) were computed based upon manually reduced eye data (see description below). Finally, task error rates originating from the user and system are reported.
2.7. Data analysis
2.7.1. Subjective workload, behavioural and physiological measures
Baseline driving reference periods consisted of 2 min of just driving prior to a recorded audio message indicating that a new task period was about to start (see Figure ). There were six such baseline periods per participant on the I-495 portion of the drive, and a seventh 2-min reference was recorded on I-93 south on the return to MIT (14 min total). Values for relevant metrics were calculated, and the mean values across the baseline periods were used as a baseline, ‘just driving’ reference.
Task completion time was calculated as the time between the end of a task prompt and successful completion or failure of the task. Instantaneous heart rate was computed by locating R-wave peaks in the EKG signal and determining the inter-beat intervals using software developed at the MIT AgeLab. In line with existing standards (Task Force of the European Society of Cardiology and the North American Society of Pacing and Electrophysiology Citation1996), automated detection results were visually reviewed and misidentified and irregular intervals manually corrected. Skin conductance was post processed using another MIT-developed package designed to remove high-frequency noise in the signal, following procedures detailed in Reimer and Mehler (Citation2011), and allowing for manual editing of motion artifacts.
Eye glance measures were quantified following ISO standards (ISO 15007-1 Citation2002; ISO 15007-2 Citation2001) with a glance to a region of interest defined to include the transition time to that object. In the case of manual coding of video images, the timing of glance is labelled from the first video frame illustrating movement to a ‘new’ location of interest to the last video frame prior to movement to a ‘new’ location. Glance data for this study were manually coded using software, now available as open source (Reimer, Gruevski, and Coughlin Citation2014), that allowed for rapid frame-by-fame review and coding. Each task period of interest was independently coded by two evaluators. Discrepancies between the evaluators (the identification of conflicting glance targets, missed glances or glance timings that differed by more than 200 ms) were mediated by a third researcher. The taxonomy and procedures for this coding methodology were initially proposed in Smith et al. (Citation2005), implemented in Angell et al. (Citation2006 see especially Appendix P) and detailed further in Reimer, Mehler, Dobres, et al. (Citation2013, Appendix G).
Statistical analyses were performed in R (R Core Team Citation2014). Owing to the non-normal distribution of the data and/or the use of ratio data (percentages) for several dependent measures, in many cases non-parametric statistics such as the Wilcoxon signed rank test and the Friedman test were used (similar to the t-test and repeated-measures ANOVA, respectively). For multifactorial analyses, repeated-measures ANOVA by ranks are presented. These tests have been shown to be more robust against Type I error in cases where data are non-normal (Conover and Iman Citation1981; Friedman Citation1937).
For analysis of the contact phone calling tasks, the primary statistical tests assumed a model in which the vehicle driven (Chevrolet or Volvo) was a between-subjects factor, and device (embedded or smartphone) and modality (manual or voice) were within-subjects factors, resulting in a 2 × (2 × 2) mixed design. Analysis of the destination address entry task assumed a model in which the vehicle driven was a between-subjects factor and device (embedded or smartphone) a within-subject factor, resulting in a 2 × 2 mixed design. Data from all trials regardless of successful completion were included in the main analysis as this was seen as more representative of the actual user experience than only considering error-free trials. Further, a previous effort investigating the demands of a production-level voice interface (Reimer, Mehler, Dobres, et al. Citation2013) found a highly consistent pattern of behavioural findings if results were computed with or without unsuccessful trails. Since the focus of the analysis was to examine the effects of different device types (and input modality in the case of phone calling), the vehicle driven was included to control for the effects of vehicle in the model, but main effects and interaction of the vehicle factor are reported only where the effect of vehicle results in notable differences between the primary variables of interests. In these cases, for comparative purposes, an alternate version of the results is presented controlling for vehicle (i.e. considering the impact on a variable relative to an average of the two vehicles utilised in the study). As noted earlier, comparisons of the embedded vehicle systems are fully detailed in Mehler et al. (Citation2015).
3. Results
Findings are presented first for the phone contact calling tasks and then for the destination address entry tasks. In considering the phone tasks, ‘modality’ refers to the overt method of interface interaction (manual or voice) and device refers to the embedded vehicle systems vs. the smartphone. As noted earlier, in selected cases, references to differences observed between the two vehicles and their specific embedded system are provided to enhance the understanding of effects related to smartphone use.
3.1. Phone contact calling
3.1.1. Self-reported workload
Workload ratings differed significantly by device (F(1, 77) = 9.68, p = .003) and input modality (F(1, 77) = 113.57, p < .001). In addition, there was a significant interaction between these factors (F(1, 77) = 11.20, p = .001). As illustrated in Figure , workload ratings for the voice tasks were lower than for the manual calling tasks; however, the reduction in workload associated with voice calling relative to manual calling was significantly greater for the embedded systems than the smartphone.
3.1.2. Task completion time
Phone task completion time was affected by a significant interaction between vehicle driven and device type (F(1, 78) = 42.69, p < .001), as well as a significant three-way interaction between vehicle driven, device type and input modality (F(1, 78) = 13.66, p < .001). Therefore, the three-way interaction was decomposed by vehicle driven to gain a clearer understanding of these factors’ effects on phone task completion time.
3.1.2.1. Chevrolet
In the Chevrolet, phone task completion time varied significantly by device (F(1, 39) = 149.66, p < .001) and modality (F(1, 39) = 53.62, p < .001). The significant interaction between device and modality (F(1, 39) = 120.98, p < .001) reveals that phone task completion times for manual interactions were similar for the embedded device and smartphone, but varied considerably when the voice interface was used (Figure ). Smartphone voice calling tasks took more than twice as long to complete compared with the Chevrolet’s embedded vehicle interface.
3.1.2.2. Volvo
In looking at the phone task completion times in the Volvo, the main effects were consistent with those for task completion times in the Chevrolet. Tasks completed with the smartphone interface took longer to complete compared with the embedded system (F(1, 39) = 13.01, p < .001). Voice contact calling tasks required significantly more time to complete compared with their manual equivalents (F(1, 39) = 100.44, p < .001). However, in contrast with the Chevrolet, the statistical interaction between device and modality in the Volvo (F(1, 39) = 44.70, p < .001) points to a more complex relationship between the visual-manual and voice interfaces across devices. Consistent with the Chevrolet, when using voice interfaces, tasks took longer to complete with the smartphone compared with the embedded system. When using the manual interfaces, however, the opposite pattern was observed.
Thus, the three-way interaction reflects varying differences in task completion time using each system’s voice interface relative to the manual interface and different relationships among each embedded manual interface relative to the smartphone manual interface. On average, voice contact calling tasks took less time to complete relative to manual contact calling tasks using the Chevrolet embedded system, but took longer using the Volvo embedded system and even longer using the smartphone voice interface. In addition, there was a small but significant increase in the time participants in the Volvo took to complete the manual contact calling tasks with the embedded interface compared with the smartphone interface, while there was no comparable difference for manual contact calling in the Chevrolet.
3.1.3. Physiological metrics
Heart rate increased during phone task periods relative to baseline single task driving (V = 710, p < .001, Wilcoxon test of mean task heart rate vs. baseline heart rate), rising by a mean of 1.9%. The average percentage change in heart rate from baseline was not significantly different between devices (M embedded = 2.18% [SE = 0.37%], M smartphone = 1.64% [SE = 0.40%]; F(1, 78) = 0.83, p = .366) or between input modalities (M manual = 1.90% [SE = 0.41%], M voice = 1.92% [SE = 0.35%]; F(1, 78) = 0.000, p = .953), nor did these factors interact significantly (F(1, 78) = 2.09, p = .152).
As was the case with heart rate, mean SCL increased significantly during phone contact calling relative to baseline driving (V = 38, p < .001). Skin conductance changes were significantly affected by modality (F(1, 72) = 4.50, p = .037), with SCL rising over baseline driving by 13.4% (SE = 1.69%) during manual tasks vs. 9.6% (SE = 1.54%) during voice tasks. Skin conductance changes were not affected by device (M embedded = 11.34% [SE = 1.56%], M smartphone = 11.71% [SE = 1.68%]; F(1, 72) = 0.02, p = .900), and no interaction between device and modality was observed (F(1, 72) = 0.11, p = .745).
3.1.4. Glance behaviour metrics
The effect of vehicle driven on mean off-road single glance duration was significant (F(1, 78) = 4.06, p = .047). Mean off-road glance duration was also significantly affected by the interaction between vehicle driven and modality (F(1, 78) = 11.09, p < .001), vehicle driven and device (F(1, 78) = 10.62, p = .002), and a three-way interaction between vehicle driven, device and modality (F(1, 78) = 18.40, p < .001). The pattern of mean single off-road glance durations for participants who drove the Chevrolet indicate that both the manual and voice interfaces of the embedded system had shorter mean single off-road glances than the manual and voice interfaces of the smartphone, respectively (Figure ). Participants in the Chevrolet also had shorter mean single off-road glances when using the embedded and smartphone voice interfaces compared with the manual interfaces.
Figure 5. Mean single off-road glance duration during phone contact calling by vehicle, device, and interface type.
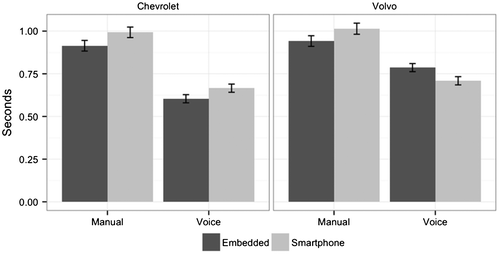
Similar to participants in the Chevrolet, participants who used Volvo’s Sensus had reductions in mean single off-road glances when using the embedded and smartphone voice interfaces relative to their manual counterparts (Figure ). However, whereas the MyLink voice interface had a greater reduction in mean single off-road glances relative to the smartphone interface, use of the Sensus voice interface was associated with greater mean single off-road glance durations than the smartphone voice interface. In addition to the three-way interaction, there was a significant main effect of device (M embedded = 0.81 s [SE = 0.02 s]; M smartphone = 0.85 s [SE = 0.02 s]; F(1, 78) = 8.41, p = .005), a significant main effect of modality (M manual = 0.97 s [SE = 0.02 s]; M voice = 0.69 s [SE = 0.01 s]; F(1, 78) = 426.64, p < .001), and a significant interaction between the two factors (F(1, 78) = 18.40, p < .001). Mean single off-road glance duration was significantly shorter when using the embedded device compared with the smartphone during manual calling tasks (M embedded = 0.93 s, M smartphone = 1.00 s) but was similar when using the voice interfaces of the devices (M embedded = 0.69 s, M smartphone = 0.69 s).
Long duration glances were significantly affected by input modality (M manual = 3.5% [SE = 0.4%]; M voice = 0.5% [SE = 0.1%]; F(1, 78) = 52.98, p < .001), but not by device (F(1, 78) = 2.67, p = .106). Furthermore, a significant device by modality interaction was observed (F(1, 78) = 4.41, p = .039). Specifically, the use of the voice interfaces resulted in a similar low percentage of long duration glances for both embedded (M = 0.5%, SE = 0.3%) and smartphone interfaces (M = 0.4%, SE = 0.3%), whereas for manual interfaces, the smartphone showed a higher frequency of long duration glances than the embedded interfaces (4.0% [SE = 0.60%] and 2.9% [SE = 0.52%], respectively).
There was a significant interaction between vehicle driven, device and modality for total off-road glance time: (F(1, 78) = 5.84, p = .018). The details of this effect are discussed below. In more general terms, total off-road glance time was significantly affected by device (F(1, 78) = 40.59, p < .001), with the embedded systems requiring less glance time compared with the smartphone (Figure ). There was also a significant effect of modality (F(1, 78) = 81.27, p < .001), with voice interfaces requiring less glance time compared with manual interfaces. These factors also interacted significantly (F(1, 78) = 56.28, p < .001) so that the use of the embedded voice interfaces required the least total off-road glance time. Based on these analyses, it is clear that within each vehicle, the embedded voice interface was associated with less off-road glance time compared with the embedded manual interface, and with both the voice and manual interfaces of the smartphone. However, the relative reduction in off-road glance time when using the voice interface was different for the two embedded systems, and this resulted in the three-way interaction which is discussed below.
Figure 6. Cumulative off-road glance times for each phone dialing task by vehicle driven. Points indicate total off-road glance time for each participant and have been jittered horizontally to minimize overlap.
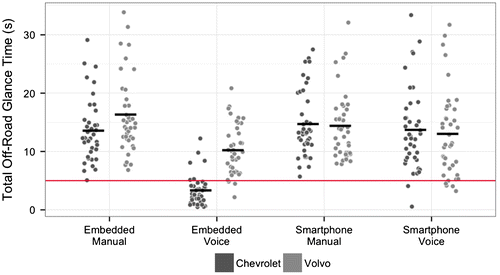
The magnitude of the difference in total off-road glance time for the phone calling tasks between the embedded system and smartphone interfaces varied across the two vehicles studied. While these data could be examined by vehicle driven at the group level, a consideration of the data at the individual participant level provides a more comprehensive view of differences between the systems. As illustrated in Figure , almost all participants required a minimum of 5 s (indicated by the solid horizontal line) of cumulative off-road glance time to complete the manual phone calling tasks and voice-based smartphone calling tasks.
For the calling tasks, individual participants’ total off-road glance durations during use of the Volvo embedded voice interface cluster below all of the manual interfaces and the smartphone voice-based interface. In addition, most drivers’ off-road glance durations during the use of the Volvo voice interface were more than 5 s. In contrast, most of drivers’ off-road glance durations were less than 5 s when using the Chevrolet’s embedded voice interface for contact calling, with just seven participants requiring more than 5 s of total off-road glance time. Thus, while the embedded voice interfaces of both vehicles showed advantages in total off-road glance time, the effect was most pronounced in the Chevrolet implementation (and hence the three-way interaction stated above).
3.1.5. Vehicle control metrics
Participants decreased their driving speed by a mean of 2.4% during phone calling task periods relative to baseline driving only (V = 2832, p < .001). A main effect of modality appeared (F(1, 78) = 6.84, p = .011); manual calling tasks (M = −3.1%, SE = 0.54%) were associated with a greater decrease in speed compared with voice calling tasks (M = −1.6%, SE = 0.36%). Device used (embedded or smartphone) did not affect speed (F(1, 78) = 0.66, p = .418), nor was there an interaction with modality (F(1, 78) = 2.97, p = .089).
Standard deviation of vehicle speed decreased significantly during phone calling task periods compared with baseline driving (V = 3232, p < .001); the percentage point difference between means was 36.6%. The percentage change in the standard deviation of vehicle speed during task periods relative to baseline driving was significantly affected by device (M embedded = −41.1% [SE = 2.27%], M smartphone = −32.1% [SE = 2.20%]; F(1, 78) = 9.58, p = .003), but not by input modality (M manual = −38.2% [SE = 2.29%], M voice = −35.0% [SE = 2.22%]; F(1, 78) = 1.29, p = .260). These factors interacted significantly (F(1, 78) = 28.61, p < .001); the percentage reduction in standard deviation of speed was greater during voice contact calling relative to manual contact calling when using the embedded devices (M voice = −45.3%, SE = 2.69%; M manual = −36.9%, SE = 3.60%) but not when using the smartphone (M voice = −24.7%, SE = 3.16%; M manual = −39.5%, SE = 2.84%). However, this pattern of results only reflects task performance using the Chevrolet embedded system, which was significantly different from the Volvo system, as indicated by a three-way interaction between vehicle driven, device type and input modality (F(1, 78) = 8.88, p = .004). There was no difference in the percentage change in standard deviation of vehicle speed when using the Volvo embedded system for manual or voice contact calling, but the percentage reduction in this measure was greater when manual contact calling with the smartphone compared with voice (Figure ).
Figure 7. Mean percentage change from baseline of standard deviation of vehicle speed during phone contact calling by vehicle, device, and interface type.
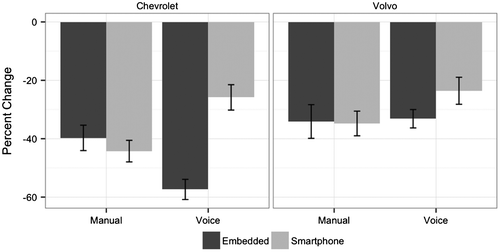
Standard deviation of speed and mean speed were not significantly correlated (tests of mean speed per participant vs. mean standard deviation per participant (R = 0.005, p = .96). This indicates that the two metrics are independent of one another or, in other words, that standard deviation of speed does not decrease simply as a function of decreasing mean speed.
Major steering wheel reversal rates increased by 33.4% during phone task periods compared with baseline driving (V = 422, p < .001). Major steering wheel reversal rates were not affected by device type (F(1, 78) = 0.14, p = .714), but the rate of major steering wheel reversals was significantly higher during manual phone contact calling tasks than voice (M manual = 15.7/min [SE = 1.00/min], M voice = 14.2/min [SE = 0.96/min]; F(1, 78) = 5.41, p = .023). The interaction between device type and modality was not significant (F(1, 78) = 1.03, p = .313).
3.2. Destination address entry
Voice entry tasks were performed with only the voice interfaces of the embedded devices and the smartphone. Table summarises the results of ANOVA-by-ranks tests for a main effect of device during the address entry tasks while controlling for the effect of the vehicle driven for the various dependent measures.
Table 1. Mean values (standard errors in parentheses) and statistical results of tests for an effect of device (embedded or smartphone) during address entry tasks with voice interfaces.
The smartphone voice interface resulted in significantly less visual demand during destination address entry tasks than the embedded system voice interfaces, as indicated by significantly lower mean off-road single glance duration, percentage of long duration off-road glances and total off-road glance time for the smartphone compared with the average of the two embedded vehicle systems Table . In addition, task completion time was significantly shorter for the smartphone voice interface than the embedded voice interfaces. The percentage reduction in standard deviation of speed during task periods relative to baseline driving was significantly greater during use of the smartphone voice interface compared with the embedded voice interfaces. No other comparisons reached statistical significance.
There were significant interactions of vehicle driven and device type on measures of total off-road glance time (F(1, 78) = 25.31, p < .001), total task time (F(1, 78) = 25.70, p < .001) and change in variability of speed from baseline driving (F(1, 78) = 8.41, p = .005). No other significant interactions were observed. Total off-road glance time was significantly longer when using the Volvo’s embedded voice interface to perform the navigation tasks (M = 22.5 s, SE = 1.43 s) compared with the other voice interfaces (M Volvo smartphone = 12.9 s [SE = 1.07 s], M Chevrolet embedded = 14.3 s [SE = 1.22 s], M Chevrolet smartphone = 14.1 s [SE = 1.29 s]). Task completion times were similar when using the smartphone voice interface regardless of vehicle driven (M Chevrolet = 51.3 s, SE = 2.65 s; M Volvo = 45.3 s, SE = 2.14 s) and longer than when using both embedded system voice interfaces; however, task completion times using the Volvo embedded voice interface (M = 80.6 s, SE = 1.71 s) were much longer than when using the Chevrolet’s (M = 66.7 s, SE = 2.85 s). The percentage change in standard deviation of vehicle speed relative to just driving was similar when performing the navigation task using the embedded voice system or smartphone voice system in the Chevrolet (M embedded = −29.5% [SE = 4.58%], M smartphone = −28.7% [SE = 4.03%]); however, a greater percentage reduction in standard deviation of speed was observed when using the smartphone voice system to perform the navigation task in the Volvo compared with Volvo’s embedded voice system (M embedded = −10.4% [SE = 5.46%], M smartphone = −25.4% [SE = 7.27%]).
Posthoc testing of total task completion time shows that there were significant differences between all 6 possible comparisons of vehicle and device type (all p < .001), with the exception of the two smartphone conditions compared across vehicles (W = 971.0, p = .101). Posthoc testing of total off-road glance time suggests that the interaction of vehicle and device type described above was primarily driven by the higher total glance time observed among drivers who used Volvo’s embedded system (all p < .001). No other posthoc comparisons yielded significant differences (all p > .264). This pattern of results was also observed for the percent change in standard deviation of speed. The percent change in standard deviation of speed observed during interactions with the Volvo embedded system was significantly different from all other vehicle and device-type combinations (all p < .024), but none of the other posthoc comparisons were significant (all p > .506).
3.3. Error analysis and interaction characterisation
Errors made during completion of the phone contact calling and address entry tasks were analysed in two ways. First, task trials were classified as error-free or, for trials where an error occurred, as a trial with a user error or a trial with a system error. User errors were instances where a participant spoke an incorrect voice command that resulted in the task not moving forward or progressing incorrectly, selected incorrect manual input, or when the research assistant provided assistance. System errors were instances where a participant issued a correct voice command that was understood by the research associate in the vehicle but was misinterpreted by the voice recognition system. If both a system error and user error occurred in the same trial, then the trial was categorised as a user error regardless of the total number of user or system errors that occurred. Thus, system errors are likely underrepresented in this analysis method.
Each trial also was categorised based on the degree of difficulty a participant encountered when completing the task. Individual trials were categorised as (1) error-free, (2) completed with backtracking, (3) completed with one instance of assistance from the research associate, (4) completed with more than one instance of assistance from the research associate or (5) as a failure. Backtracking was defined as instances where the system did not recognise or misinterpreted a command and provided another opportunity for the voice command to be entered; this included instances where the participant restarted the task without aid from the research associate. Backtracking could also occur because a participant recognised that they made an error (such as giving a wrong street name) and used an option provided by the system to correct the error. The research associate in the vehicle provided assistance to the participant when he judged that a participant was not going to progress through a task on his/her own. One or more instances of researcher assistance were provided to participants to increase the chance that the task in a given trial was completed successfully. This support was provided to mitigate the participant’s frustration and to allow for monitoring whether correction of simple misunderstandings or forgetting of commands resolved initial problems in using the systems while driving. A trial was categorized as a failure if the participant had to restart the task more than twice, failed to progress in the task despite receiving assistance from the research associate, or cases where the system or user executed the task incorrectly. Both methods of error coding were completed by two members of the research staff who independently evaluated each trial. One staff member was the research associate in the vehicle during the drive and the other was an associate who reviewed video and audio recordings of the drive. A third staff member mediated discrepancies.
3.3.1. Errors: contact calling
The contact calling trials performed with the manual interfaces of the embedded systems or smartphone were more often error-free (91%) than the contact calling trials performed using the voice interfaces (84%). With voice calling tasks, there were markedly more trials performed using the smartphone that ended in failure, with backtracking, or that required assistance from the research associate compared with trials performed using the embedded vehicle systems (Table ). An error was coded for about 25% of the trials completed using the smartphone voice interface, whereas an error was coded for 7.5% of the trials completed using the embedded system voice interfaces. The percentage of manual calling trials that were error-free when participants used an embedded system was slightly higher (93%) than when the manual interface of the smartphone was used (88%). Of the manual calling trials completed with the smartphone where an error occurred, the majority of errors were backtracking (Table ).
Table 2. Percentage of contact calling trials in each error category for each interface modality and device.
Table provides the number of phone calling trials with a user error and the number with a system error across device and modality. Overall, there were nearly twice as many trials with a user error (8.4%) as trials with a system error (4.4%). About 87% of trials with a system error (48 out 55) occurred when using the smartphone’s voice interface.
Table 3. Percentage of contact calling trials without errors, system errors, or user errors for each interface modality and device.
3.3.2. Errors: navigation entry
The percentage of address entry trials that were coded as error-free was smaller for trials performed with the smartphone or embedded system in the Chevrolet (59%) than with the smartphone or embedded system in the Volvo (86%) (Table ). When considering the smartphone and embedded systems separately, the percentage of trials that were error-free was substantially lower among Chevrolet drivers who entered addresses using MyLink (49.2%) than among Chevrolet drivers using the smartphone (69.2%). In contrast, a somewhat larger proportion of address entry trials were error-free when Volvo drivers used Sensus (89.2%) compared with the smartphone (82.5%). The percentage of address entry trials that ended with failures was highest when drivers used MyLink (20%) and lowest with Sensus (1.7%), with 14.2% of trials using the smartphone ending in failure in the Chevrolet and 9.2% of these trials ending in failure in the Volvo.
Table 4. Percentage of address entry trials coded in each error category for each interface modality and device.
In general, the percentage of navigation tasks with a system error (19.0%) was greater than the percentage of trials with user errors (8.5%). A system error was noted in almost three times as many address entry trials among participants driving the Chevrolet (28%) compared with trials completed by participants driving the Volvo (9.6%). Trials with a user error were most commonly recorded among participants who were using the Chevrolet MyLink to enter addresses compared with when they used the smartphone and participants using the smartphone or Sensus in the Volvo (Table ).
Table 5. Percentage of address entry trials coded without errors or with a system or user error for each vehicle and device.
4. Discussion
4.1. Phone contact calling
Consistent with patterns observed in previous research on infotainment systems (Chiang, Brooks, and Weir Citation2005; Mehler et al. Citation2014; Owens, McLaughlin, and Sudweeks Citation2011; Reimer, Mehler, Dobres, et al. Citation2013; Reimer et al. Citation2014; Shutko et al. Citation2009), the voice-based methods of phone contact calling were associated with lower self-reported workload ratings and lower visual demand (mean single glance duration, percentage of glances longer than 2 s and total eyes-off-road time) compared with the manual methods. The present analysis extends other work by showing that this pattern of results holds for the Samsung smartphone as well as for the embedded vehicle systems studied. Further, while heart rate as an arousal measure did not show an advantage for either modality, SCL were consistent with lower workload, on average, during voice-based calling compared with manual calling.
For phone contact calling, the apparent advantages for the voice interfaces were greater with the embedded vehicle systems than with the smartphone across a number of metrics. On average, self-reported workload and total eyes-off-road time were lower using the embedded systems’ voice interfaces than the smartphone voice interface and their manual counterparts. Thus, pairing a smartphone with a vehicle’s embedded system and using the embedded system’s voice interface may reduce workload and visual demand. In this regard, it is worth noting that the smartphone was mounted during these evaluations. Considering the demands and risks associated with picking up and handling a phone (Farmer et al. Citation2014; Fitch et al. Citation2013; Klauer et al. Citation2014), one might anticipate additional benefits for embedded systems relative to smartphones that are not mounted. It should be noted that a single smartphone was examined, and the findings may not apply to other smartphones with different design approaches and different voice recognition technology. Mehler et al.’s (Citation2015) study illustrated how different embedded vehicle system designs have varying effects on driver workload and visual scanning, and presumably these measures also would vary across different smartphone interface designs. The smartphone in this study was selected because the Android platform had the largest market presence and the screen size was larger, rather than for its specific interface design characteristics.
Returning again to the broader question of using a smartphone or embedded vehicle system for contact calling, the total number of errors was higher when using the smartphone. This held for both the manual and voice methods of calling. One of the factors for the higher system error rate for the voice interface in the smartphone may be related to the positioning of the phone. When mounted on the dashboard, the microphone was farther away from drivers than if they were holding it in their hand, possibly affecting sound quality and voice recognition. To the extent that this is the case, it would suggest that the characteristics, integration, or location of the microphones used in the cars were more effective for this application. Similarly, one would presume that the touch screen interface on the phone was optimized for handheld operation rather than for mounted use. Reaching for and touching smaller icons on the smartphone might explain some of the higher user errors in the manual smartphone mode vs. the manual mode for the embedded systems. Additional characteristics relative to the voice interface on the smartphone are considered below in the context of the destination address entry task.
Considering the primary driving performance metrics, there were no significant differences by device type (embedded or smartphone) in terms of the degree of speed reduction or in the extent to which major steering wheel reversal rates increased during task periods. Thus, no relative advantage for embedded systems or the smartphone was apparent in these measures. Steering wheel reversal rates were higher during manual calling than voice calling, which is consistent with increased competition for manual resources between the driving task and the secondary task during manual calling relative to voice calling. Voice calling using the embedded systems was associated with a larger reduction in the standard deviation of speed relative to baseline driving than manual calling. The general reduction in speed variability during task periods relative to baseline driving may reflect drivers shifting their attention away from vehicle control to interacting with the embedded system. However, it is not clear why greater reductions in speed variability were observed with voice calling than manual calling, given that voice calling presumably interfered with the driving task less than manual calling; this should be a topic for future research.
The time taken to make phone calls with the smartphone voice interface was significantly longer than for the embedded voice interfaces. This is likely related, at least in part, to the initial greeting message played each time when the driving mode of the voice interface of the Samsung Galaxy was engaged, followed by the need to say ‘Hi Galaxy’ prior to being able to issue a voice command. This added time and a layer to each task that was not present in either embedded system.
Experience with the Samsung’s driving mode also highlighted the potentially dynamic nature of smartphone-based user interfaces. Software updates were blocked on the study phones to ensure a consistent user experience across participants. Nonetheless, one phone was inadvertently allowed to update and the voice interface was modified as a result. For purposes of the study, the update was rolled back. However, exploring the updated software revealed significant changes from the software version tested during the study. For example, the extended greeting message that had previously played each time driving mode was activated at the beginning of a task was no longer present, removing this time-consuming aspect of the earlier interface. It is an open question as to how a driver learns about and adapts to such system upgrades.
4.2. Destination address entry
While a number of advantages were observed for the embedded systems for voice-based phone contact calling compared with the smartphone, a somewhat different picture appears in comparing the smartphone and embedded system interfaces for voice-based destination address entry. While self-reported workload and increases in heart rate and skin conductance were all nominally higher for the smartphone interfaces than for the embedded systems, these differences were not statistically significant. In contrast, mean single glance duration, the percentage of long duration glances and total eyes-off-road time were all significantly greater for the embedded systems. Broadly considered, it appears that the Samsung smartphone voice-based system for the destination address entry task provided a less visually demanding engagement than the average of the two embedded alternatives. However, considering the visual demands in the context of significant two-way interaction between vehicle driven and device on total off-road glance time, the results suggest that the higher off-road glance time associated with the menu-based Volvo Sensus system was the main driver of the effect (see Mehler et al. Citation2015 for a detailed analysis and discussion of vehicle differenes). In other words, the one-shot voice commands in the Chevrolet MyLink system led to visual demands that were on par with Samsung smartphone voice-based system for the address entry task.
The apparent advantage in visual demand for the smartphone voice interface for destination address entry must be tempered somewhat in evaluating net advantage for the two system types when errors are taken into account. In this regard, differences in system implementation features and possible differences in the vehicle environment may interact to impact the overall task experience. As detailed in Mehler et al. (Citation2015), the segmented approach to address entry used by the Volvo Sensus system (breaking voice input into independent chunks for city, street name and street number) took more time and involved greater total eyes-off-road time compared with the Chevrolet MyLink system using a one-shot approach, but Sensus was associated with fewer system recognition errors. A similar difference appears when comparing the embedded Sensus implementation with the Samsung smartphone implementation, which also provided a one-shot address entry; specifically, the one-shot approach reduced visual demand when successful, but it also had a higher error rate. Comparing the one-shot address entry of the Samsung and MyLink voice interfaces, the smartphone had fewer errors.
Interestingly, system-based error rates for voice-based address entry in the smartphone were higher for participants who drove in the Chevrolet Equinox than for those who drove in the Volvo XC60 (the same Smartphone task showed 13.3% more errors when performed in the Chevy than in the Volvo). While this could be a chance finding, another possibility is that ambient road noise was higher in the Equinox, and that this might have impacted voice recognition. In other words, it is possible that noise which is present inside the vehicle may affect and/or alter the performance of the voice recognition system. In essence, the presence or absence of noise-dampening or sound-absorbing treatments in the vehicle may influence user success. In consideration of this hypothesis, an assessment of the background sound levels in each of the vehicles at 65 mph highway speed was conducted. Three sound readings were recorded in each vehicle at the respective mounting position of the smartphones. The average of the three readings indicated that the ambient noise levels in the Chevrolet Equinox were louder than those in the Volvo XC60 at the 125 Hz band (65dBA Equinox; 62dBA XC60) and the 2000 Hz band (62.6dBA Equinox; 60dBA XC60), suggesting that ambient sound-level differences between the vehicles could have contributed to the observed differences in voice recognition errors for both the embedded systems and the smartphone.
Ambient noise, and the earlier reported differences in speed provided by each vehicle’s CAN bus and different mounting positions of the smartphones (driven by the physical layout of each vehicle’s dashboard), illustrate some of the complexities of conducting inter-vehicle comparisons. In addition, for practitioners, these issues underscore that there are system-level vehicle integration issues (ambient noise abatement, physical interface component location, etc.) that are important to address during vehicle development because they may influence the performance of a voice recognition system in the vehicle and the resulting user experience. While field experiments allow for observing driver use of technologies in a real-world environment provide valuable data, they have limitations, and the findings of field experiments are best understood when considered together with other methods to develop a comprehensive understanding of the technologies.
4.3. Limitations
The study sample was comprised of novice users. Some of the drawbacks noted (e.g. higher error rates) for the Smartphone and embedded system voice interfaces may not be observed among actual owners who have more familiarity using the voice commands or menu structure. Additionally, the visual demands observed with novice user interactions with the voice interfaces may not generalise to experienced users who know the sequence of commands or pace of turn-taking when completing tasks with the voice interface.
Another limitation is that participants may have felt compelled to perform the contact calling and address entry tasks in situations where they normally would not. The task instructions and research assistants repeatedly emphasised that participants should not perform a task if they felt unsafe or would not engage in the task during personal driving; however, no participants who went on road declined to engage in a task.
Additionally, the extent to which effects associated with the dependent measures analysed translate into safety risk are unclear. As emphasised in Reimer Mehler, Dobres, et al. (Citation2013), such driver and visual performance data are informative concerning the attentional demand characteristics of the interface tasks, rather than necessarily being predictive of risk to drivers who are operating their own vehicles. Cognitive workload is inherently difficult to evaluate and was assessed indirectly as a component of self-reported workload and through peripheral physiological indices of arousal (heart rate and skin conductance).
The use of the same phone contacts and destination addresses across the different interfaces could be questioned. However, the use of the same entry tasks for each interface removed the necessity to characterise and identify different addresses that had equal levels of difficulty with regard to speech complexity. Counterbalancing the order of interface assessment across the sample should have controlled for any issue of presentation order. Moreover, the nature of phone calling and address entry tasks is such that it is likely that many drivers will call the same contacts and enter the same destinations into a navigation system relatively frequently.
Finally, it is unknown how manual entry might differ for embedded systems or the smartphone in performance of navigation tasks. However, it should be noted that the vehicles tested in this study locked out manual address entry when the vehicle was moving, and given concerns with the safety of entering an address while driving, ethical considerations would have prevented assessing manual performance on this task in the current field setting. Similarly, the set of secondary tasks assessed in this study were limited to placing phone calls and entering addresses. Whether similar patterns would be observed for tasks more complex than contact calling, such as sending voice-based text messages or point-of-interest searches, is an area for future research.
5. Conclusions
Objective evidence is converging, that, as intended, voice-based interfaces offer a less visually demanding way to access and input information than primary visual-manual alternatives, although it is also evident that voice interfaces do not completely remove visual demand as has sometimes been suggested. It is further becoming clear that the nature of the task interacts with the voice interface’s design (one-shot approach, segmented menu-based approach, etc.) to influence effective demand and subjective workload, such that smartphones as well as embedded systems may be more or less visually and cognitively demanding, depending upon design and implementation decisions. Given that voice systems are mixed-mode interfaces that draw on auditory-vocal-visual-manual and cognitive resources (Chiang, Brooks, and Weir Citation2005; Mehler et al. Citation2014; Owens, McLaughlin, and Sudweeks Citation2011; Reimer, Mehler, Dobres, et al. Citation2013; Reimer et al. Citation2014; Shutko et al. Citation2009), all relevant attention components should be considered when assessing the overall demand of voice interfaces rather than focusing on cognitive load alone (Cooper, Ingebretsen, and Strayer Citation2014; Strayer et al. Citation2014; Strayer, et al. Citation2015b). A holistic assessment is especially important, given that the relationship between glances away from the roadway (visual demand) and increased risk of safety relevant events (Klauer et al. Citation2006; Victor et al. Citation2014) is well established. In addition, based upon this work, it is clear that a comprehensive assessment should ultimately include consideration of demand profiles relative to alternate methods of accomplishing a task (e.g. voice vs. traditional visual-manual, embedded vs. nomadic) available to drivers.
When visual demand is assessed, there are sensitivity issues that have not been directly addressed in the relevant ISO standards (ISO 15007-1 Citation2002; ISO 15007-2 Citation2001) that investigators may wish to consider. In recent work, Strayer et al. (Citation2015a) begin to consider the allocation of visual attention, but at a relatively low degree of granularity (frame-by-frame analysis was completed at a rate of 2 frames per second as opposed to the 30 frames per second rate used here). Such a coarse half-a-second window may not provide adequate sensitivity to capture all short duration glances away from the roadway (5747 of the 28,783 off-road glances considered in this report were shorter than a half of second, e.g. 20%) or a sensitive measure of total off-road glance time, a key association with risk considered central to the test methods in the NHTSA guidelines (National Highway Traffic Safety Administration Citation2013). Development of a data-based recommended minimum sampling rate would provide useful guidance for both researchers and front-line practitioners.
While significant off-road visual demands exist in the demand profiles of the voice-based interfaces studied, it is unclear if these demands are equivalent (in risk) with the visual demands of more traditional visual-manual interfaces. As illustrated in Muñoz, Reimer, and Mehler (Citation2015), differences in the allocation of visual attention across the visual field appear between visual-manual and voice-involved tasks. These observations suggest that more situationally relevant off-road glances (e.g. to the mirrors, instrument cluster) occur during interactions with a voice-based interface than a visual-manual interface. Further, voice-based systems have been shown to have a lower impact on detection response tasks than primary visual-manual alternatives (Beckers et al. Citation2014; Munger et al. Citation2014; Samost et al. Citation2015). These findings illustrate a need to consider where off-the-road a driver’s attention is focused in the assessment of voice-based interfaces.
It is clear that differences in task time complicate direct comparisons of total demand over the course of a task. Future consideration in the modelling of demand as it relates to the time course of an activity, locus of attention off-the-road and operating context may move interface demand assessment forward effectively by creating a stronger link between the moment-to-moment demand of a system and its impact on safety. Practitioners challenged to optimise a voice-based system’s characteristics in an evolving design evaluation space may wish to prioritise the optimisation of the visual elements of the system as they relate to the task structure, time course, display characteristics, etc., in addition to basic functionality of the speech recogniser, as a reasonable evaluation methodology grounded in the current state of knowledge. This approach does not preclude further investigations of how developing measures of cognition load relate to the optimisation of mixed-mode interactions.
Overall, the results suggest that there are benefits and drawbacks to voice interface technology in the smartphone relative to two embedded voice systems. While the smartphone largely outperformed the Volvo Sensus voice system in task time and total off-road visual engagement for the destination address entry task, it showed more modest improvements in comparison to the Chevrolet MyLink system where predominant difference related to an improvement in task time. The degree to which these differences relate to differences in task structure, display characteristics or other variables such as system response time (McWilliams et al. Citation2015), voice recognition system performance, ambient noise, etc. are all potential contributors that need to be actively optimised at the system design level. The smartphone showed a smaller reduction in total off-road glance time when placing calls using voice input compared with manual input. In terms of manual interactions, results are also mixed. Average task completion time for contact calling using the smartphone was shorter than when using Sensus but no different from MyLink. In so much as drivers choose to engage in contact calling, the embedded vehicle voice interfaces would appear to be the most advantageous method of the ones considered in the current study. In contrast, the relative benefits of the voice interfaces of the embedded vehicle or the smartphone voice interfaces for destination address entry are not as clear.
The complex relationships between outcome measures need to be weighed when developing systems and considering their potential impact on safety. Clearly, a system that is capable of performing an operation with minimal demand on the driver is desirable. However, a brittle system that has difficulty performing requested operations without errors may be no more advantageous than a system that places more demands on attentional resources yet performs flawlessly.
Funding
This work was supported by the Insurance Institute for Highway Safety and a grant from United States Department of Transportation’s Region One University Transportation Center at MIT.
Disclosure statement
No potential conflict of interest was reported by the authors.
Acknowledgements
Primary support for this work was provided by the Insurance Institute for Highway Safety (IIHS), with additional support provided through a grant by the US DOT’s Region I New England University Transportation Center at MIT. Acknowledgement is gratefully extended to Peter Hamscher, Alex Hruska, Martin Lavallière, Alea Mehler, Hale McAnulty, Mauricio Muñoz, Lauren Parikhal, Anthony Pettinato, Adrian Rumpold and Andrew Sipperley for their contributions in protocol development, data collection, reduction and manual scoring. Appreciation is also extended to Adrian Lund, Anne McCartt and David Zuby and two anonymous reviewers for review and comment on the manuscript.
References
- Angell, L., J. Auflick, P. A. Austria, D. Kochhar, L. Tijerina, W. Biever, T. Diptiman, J. Hogsett, and S. Kiger. 2006. Driver Workload Metrics Task 2 Final Report. Washington, DC: U.S. Department of Transportation National Highway Traffic Safety Administration.10.1037/e729342011-001
- Atchley, P., and M. Chan. 2011. “Potential Benefits and Costs of Concurrent Task Engagement to Maintain Vigilance: A Driving Simulator Investigation.” Human Factors: The Journal of the Human Factors and Ergonomics Society 53 (1): 3–12.10.1177/0018720810391215
- Beckers, N., S. Schreiner, P. Bertrand, B. Reimer, B. Mehler, D. Munger, and J. Dobres. 2014. “Comparing the Demands of Destination Entry Using Google Glass and the Samsung Galaxy S4.” Proceedings of the 58th Annual Meeting of the Human Factors and Ergonomics Society, Chicago, IL, 2156–2160.
- Brookhuis, K. A., and D. de Waard. 2001. “Assessment of Drivers’ Workload: Performance and Subjective and Physiological Indexes.” In Stress, Workload, and Fatigue, edited by P. A. Hancock and P. A. Desmond, 321–333. Mahwah, NJ: Lawrence Erlbaum Associates.
- Caird, J. K., C. R. Willness, P. Steel, and C. Scialfa. 2008. “A Meta-analysis of the Effects of Cell Phones on Driver Performance.” Accident Analysis & Prevention 40 (4): 1282–1293.
- Chiang, D. P., A. M. Brooks, and D. H. Weir. 2005. Comparison of Visual-Manual and Voice Interaction with Contemporary Navigation System HMIs. SAE 2005 World Congress & Exhibition. Warrendale, PA: SAE International SAE Technical Paper 2005-01-0433.
- Collet, C., A. Guillot, and C. Petit. 2010. “Phoning While Driving II: A Review of Driving Conditions Influence.” Ergonomics 53 (5): 602–616.10.1080/00140131003769092
- Collet, C., E. Salvia, and C. Petit-Boulanger. 2014. “Measuring Workload with Electrodermal Activity during Common Braking Actions.” Ergonomics 57 (6): 886–896.10.1080/00140139.2014.899627
- Conover, W. J., and R. L. Iman. 1981. “Rank Transformations as a Bridge between Parametric and Nonparametric Statistics.” American Statistician 35 (3): 124–129.
- Cooper, J. M., H. Ingebretsen, and D. L. Strayer. 2014. Title Mental Workload of Common Voice-based Vehicle Interactions across Six Different Vehicle Systems. Washington, DC: AAA Foundation for Traffic Safety.
- Dingus, T. A., S. G. Klauer, V. L. Neale, A. Petersen, S. E. Lee, J. D. Sudweeks, M. A. Perez, J. Hankey, D. J.Ramsey, S. Gupta, C. Bucher, Z.R. Doerzaph, J. Jermeland, and R.R. Knipling. (2006). The 100-Car Naturalistic Driving Study, Phase II – Results of the 100-Car Field Experiment: U.S. Department of Transportation, National Highway Traffic Safety Administration.
- Driver Focus-Telematics Working Group. 2006. Statement of Principles, Criteria and Verification Procedures on Driver Interactions with Advanced in-Vehicle Information and Communication Systems, Version 2.0: Alliance of Automotive Manufacturers.
- Farmer, C. M., S. G. Klauer, J. A. McClafferty, and F. Guo. 2014. Relationship of near-Crash/Crash Risk to Time Spent on a Cell Phone While Driving. Arlington, VA: Insurance Institute for Highway Safety.
- Fitch, G. A., S. A. Soccolich, F. Guo, J. McClafferty, Y. Fang, R. L. Olson, M. A. Perez, R. J. Hanowski, J. M. Hankey, and T. A. Dingus. 2013. The Impact of Hand-Held and Hands-Free Cell Phone Use on Driving Performance and Safety-Critical Event Risk (Report No. DOT HS 811 757). Washington, DC: National Highway Traffic Safety Administration (NHTSA).
- Friedman, M. 1937. “The Use of Ranks to Avoid the Assumption of Normality Implicit in the Analysis of Variance.” Journal of the American Statistical Association 32 (200): 675–701.10.1080/01621459.1937.10503522
- Gershon, P., D. Shinar, T. Oron-Gilad, Y. Parmet, and A. Ronen. 2011. “Usage and Perceived Effectiveness of Fatigue Countermeasures for Professional and Nonprofessional Drivers.” Accident Analysis & Prevention 43 (3): 797–803.
- Hart, S. G. 2006. “Nasa-Task Load Index (NASA-TLX); 20 Years Later.” Proceedings of the Human Factors and Ergonomics Society Annual Meeting, 904–908.10.1177/154193120605000909
- Horrey, W. J., and C. D. Wickens. 2006. “Examining the Impact of Cell Phone Conversations on Driving Using Meta-analytic Techniques.” Human Factors: The Journal of the Human Factors and Ergonomics Society 48 (1): 196–205.10.1518/001872006776412135
- ISO 15007-1. 2002. Road Vehicles – Measurement of Driver Visual Behaviour with Respect to Transport Information and Control Systems – Part 1: Definitions and Parameters. Geneva: International Standards Organization.
- ISO 15007-2. 2001. Road Vehicles – Measurement of Driver Visual Behaviour with Respect to Transport Information and Control Systems – Part 2: Equipment and Procedures. Geneva: International Standards Organization.
- Klauer, S. G., T. A. Dingus, V. L. Neale, J. D. Sudweeks, and D. J. Ramsey. 2006. The Impact of Driver Inattention on near-Crash/Crash Risk: An Analysis Using the 100-Car Naturalistic Driving Study Data (Report No. DOT HS 810 594). Washington, DC: United States Department of Transportation, National Highway Traffic Safety Administration.
- Klauer, S. G., F. Guo, B. G. Simons-Morton, M. C. Ouimet, S. E. Lee, and T. A. Dingus. 2014. “Distracted Driving and Risk of Road Crashes among Novice and Experienced Drivers.” New England Journal of Medicine 370 (1): 54–59.10.1056/NEJMsa1204142
- Mccartt, A. T., L. A. Hellinga, and K. A. Bratiman. 2006. “Cell Phones and Driving: Review of Research.” Traffic Injury Prevention 7 (2): 89–106.10.1080/15389580600651103
- McCartt, A. T., D. G. Kidd, and E. R. Teoh. 2014. “Driver Cellphone and Texting Bans in the United States: Evidence of Effectiveness.” Annals of Advances in Automotive Medicine 58: 99–114.
- McKnight, A. J., and A. S. McKnight. 1993. “The Effect of Cellular Phone Use upon Driver Attention.” Accident Analysis and Prevention 25 (3): 259–265.10.1016/0001-4575(93)90020-W
- McWilliams, T., B. Reimer, B. Mehler, J. Dobres, and H. McAnulty. 2015. “Secondary Assessment of the Impact of Voice Interface Turn Delays on Driver Attention and Arousal in Field Conditions: A Consideration of 4 Vehicle Systems and a Smartphone.” Proceedings of the 8th International Driving Symposium on Human Factors in Driver Assessment, Training, and Vehicle Design, Snowbird, UT, 414–420.
- Mehler, B., D. Kidd, B. Reimer, I. Reagan, J. Dobres, and A. McCartt. 2015. “Multi-Modal Assessment of on-Road Demand of Voice and Manual Phone Calling and Voice Navigation Entry across Two Embedded Vehicle Systems.” Ergonomics. doi:10.1080/00140139.2015.1081412.
- Mehler, B., B. Reimer, and J. F. Coughlin. 2012. “Sensitivity of Physiological Measures for Detecting Systematic Variations in Cognitive Demand from a Working Memory Task: An on-Road Study across Three Age Groups.” Human Factors: The Journal of the Human Factors and Ergonomics Society 54 (3): 396–412. doi:10.1177/0018720812442086.
- Mehler, B., B. Reimer, J. F. Coughlin, and J. A. Dusek. 2009. “The Impact of Incremental Increases in Cognitive Workload on Physiological Arousal and Performance in Young Adult Drivers.” Proceedings of the Transportation Research Board of The National Academies, Washington, DC.
- Mehler, B., B. Reimer, J. Dobres, H. McAnulty, A. Mehler, D. Munger, et al. 2014. Further Evaluation of the Effects of a Production Level “Voice-Command” Interface on Driver Behavior: Replication and a Consideration of the Significance of Training Method (Technical Report 2014-2). Cambridge, MA: MIT AgeLab.
- Munger, D., B. Mehler, B. Reimer, J. Dobres, A. Pettinato, B. Pugh, et al. 2014. “A Simulation Study Examining Smartphone Destination Entry While Driving.” Proceedings of the 6th International Conference on Automotive User Interfaces and Interactive Vehicle Applications, Seattle, WA.10.1145/2667317
- Muñoz, M., B. Reimer, and B. Mehler. 2015. “Exploring New Qualitative Methods to Support a Quantitative Analysis of Glance Behavior.” Proceedings of the 7th International Conference on Automotive User Interfaces and Interactive Vehicle Applications (AutomotiveUI ‘15), Nottingham, UK.
- National Highway Traffic Safety Administration. 2013. Visual-Manual NHTSA Driver Distraction Guidelines for in-Vehicle Electronic Devices (Docket No. NHTSA-2010-0053). Washington, DC: U.S. Department of Transportation National Highway Traffic Safety Administration (NHTSA).
- Nurullah, A. S., J. Thomas, and F. Vakilian. 2013. “The Prevalence of Cell Phone Use While Driving in a Canadian Province.” Transportation Research Part F: Traffic Psychology and Behaviour 19: 52–62.10.1016/j.trf.2013.03.006
- Owens, J. M., McLaughlin, S. B., and Sudweeks, J. (2010). “On-Road Comparison of Driving Performance Measures When Using Handheld and Voice-Control Interfaces for Mobile Phones and Portable Music Players.” SAE International Journal of Passenger Cars – Mechanical Systems 3 (1): 734–743.10.4271/2010-01-1036
- Owens, J. M., S. B. McLaughlin, and J. Sudweeks. 2011. “Driver Performance While Text Messaging Using Handheld and in-Vehicle Systems.” Accident Analysis & Prevention 43 (3): 939–947.
- R Core Team. (2014). R: A Language and Environment for Statistical Computing. Vienna: R Foundation for Statistical Computing. http://www.R-project.org/.
- Reagan, I. J., and D. G. Kidd. 2013. “Using Heirarchical Task Analysis to Compare Four Vehicle Manufacturers’ Infotainment Systems.” Proceedings of the Human Factors and Ergonomics Society Annual Meeting, San Diego, CA, 1495–2599.
- Redelmeier, D. A., and R. J. Tibshirani. 1997. “Association between Cellular-Telephone Calls and Motor Vehicle Collisions.” New England Journal of Medicine 336 (7): 453–458.10.1056/NEJM199702133360701
- Reimer, B., P. Gruevski, and J. F. Coughlin. 2014. MIT AgeLab Video Annotator. Cambridge, MA. Retrieved from TBD posting of open sourced code is in progress.
- Reimer, B., and B. Mehler. 2011. “The Impact of Cognitive Workload on Physiological Arousal in Young Adult Drivers: A Field Study and Simulation Validation.” Ergonomics 54 (10): 932–942.10.1080/00140139.2011.604431
- Reimer, B., B. Mehler, J. Dobres, and J. F. Coughlin. 2013. The Effects of a Production Level “Voice-Command” Interface on Driver Behavior: Reported Workload, Physiology, Visual Attention, and Driving Performance (Technical Report 2013-17a). Cambridge, MA: MIT AgeLab.
- Reimer, B., B. Mehler, J. Dobres, H. McAnulty, A. Mehler, D. Munger, and A. Rumpold. 2014. “Effects of an ‘Expert Mode’ Voice Command System on Task Performance, Glance Behavior & Driver Physiology.” Proceedings of the 6th International Conference on Automotive User Interfaces and Interactive Vehicle Applications, Seattle, WA.
- Reimer, B., B. Mehler, H. McAnulty, D. Munger, A. Mehler, E. A. G. Perez, T. Manhardt, and J. F. Coughlin. 2013. “A Preliminary Assessment of Perceived and Objectively Scaled Workload of a Voice-Based Driver Interface.” Proceedings of the Proceedings of the 7th International Driving Symposium on Human Factors in Driver Assessment, Training, and Vehicle Design, Bolton Landing, NY, 537–543.
- Reimer, B., B. Mehler, Y. Wang, and J. F. Coughlin. 2010. “The Impact of Systematic Variation of Cognitive Demand on Drivers’ Visual Attention across Multiple Age Groups.” Proceedings of the 54th Annual Meeting of the Human Factors and Ergonomics Society, San Francisco, CA, 2052–2056.
- Reimer, B., B. Mehler, Y. Wang, and J. F. Coughlin. 2012. “A Field Study on the Impact of Variations in Short-Term Memory Demands on Drivers' Visual Attention and Driving Performance across Three Age Groups.” Human Factors: The Journal of the Human Factors and Ergonomics Society 54 (3): 454–468. doi:10.1177/0018720812437274.
- Samost, A., D. Perlman, A. G. Domel, B. Reimer, B. Mehler, A. Mehler, J. Dobres, and T. McWilliams. 2015. “Comparing the Relative Impact of Smartwatch and Smartphone Use While Driving on Workload, Attention, and Driving Performance.” Proceedings of the 59th Annual Meeting of the Human Factors and Ergonomics Society, Los Angeles, CA, 1602–1606.
- Shutko, J., K. Mayer, E. Laansoo, and L. Tijerina. 2009. Driver Workload Effects of Cell Phone, Music Player, and Text Messaging Tasks with the Ford SYNC Voice Interface versus Handheld Visual-Manual Interfaces. Warrendale, PA: SAE International SAE Technical Paper 2009-01-0786. doi:10.4271/2009-01-0786.
- Smith, D. L., J. Chang, R. Glassco, J. Foley, and D. Cohen. 2005. “Methodology for Capturing Driver Eye Glance Behavior during in-Vehicle Secondary Tasks.” Transportation Research Record: Journal of the Transportation Research Board 1937 (1): 61–65.10.3141/1937-09
- Strayer, D. L., J. M. Cooper, J. Turrill, J. Coleman, N. Medeiros-Ward, and F. Biondi. 2013. Measuring Cognitive Distraction in the Automobile. Washington, DC: AAA Foundation for Traffic Safety.
- Strayer, D. L., J. M. Cooper, J. Turrill, J. R. Coleman, and R. J. Hopman. 2015a. Measuring Cognitive Distraction in the Automobile III: A Comparison of Ten 2015 in-Vehicle Information Systems. Washington, DC: AAA Foundation for Traffic Safety.
- Strayer, D. L., J. M. Cooper, J. Turrill, J. R. Coleman, and R. J. Hopman. 2015b. The Smartphone and the Driver’s Cognitive Workload: A Comparison of Apple, Google, and Microsoft’s Intelligent Personal Assistants. Washington, DC: AAA Foundation for Traffic Safety.
- Strayer, D. L., and F. A. Drews. 2004. “Profiles in Driver Distraction: Effects of Cell Phone Conversations on Younger and Older Drivers.” Human Factors: The Journal of the Human Factors and Ergonomics Society 46 (4): 640–649.10.1518/hfes.46.4.640.56806
- Strayer, D. L., F. A. Drews, and D. J. Crouch. 2006. “A Comparison of the Cell Phone Driver and the Drunk Driver.” Human Factors: The Journal of the Human Factors and Ergonomics Society 48 (2): 381–391.10.1518/001872006777724471
- Strayer, D. L., F. A. Drews, and W. A. Johnston. 2003. “Cell Phone-Induced Failures of Visual Attention during Simulated Driving.” Journal of Experimental Psychology: Applied 9 (1): 23–32.
- Strayer, D. L., J. Turrill, J. R. Coleman, E. V. Ortiz, and J. M. Cooper. 2014. Measuring Cognitive Distraction in the Automobile II: Assessing in-Vehicle Voice-Based Interactive Technologies. Washington, DC: AAA Foundation for Traffic Safety.
- Task Force of the European Society of Cardiology and the North American Society of Pacing and Electrophysiology. 1996. “Heart Rate Variability: Standards of Measurement, Physiological Interpretation, and Clinical Use.” European Heart Journal 17: 354–381.
- Tison, J., N. Chaudhary, and L. Cosgrove. 2011. National Phone Survey on Distracted Driving Attitudes and Behaviors (Report No. DOT HS 811 555). Washington, DC: National Highway Traffic Safety Administration.10.1037/e562822012-001
- Victor, T., J. Bärgman, C. Boda, M. Dozza, J. Engstroem, C. Flannagan, J. D. Lee, G. Markkula . 2014. Analysis of Naturalistic Driving Study Data: Safer Glances, Driver Inattention, and Crash Risk: Safer.
- Yang, Y., B. Reimer, B. Mehler, and J. Dobres 2013. “A Field Study Assessing Driving Performance, Visual Attention, Heart Rate and Subjective Ratings in Response to Two Types of Cogntive Workload.” Proceedings of the 7th International Driving Symposium on Human Factors in Driver Assessment, Training, and Vehicle Design, Bolton Landing, NY, 397–403.
- Young, R. A., and C. Schreiner. 2009. “Real-World Personal Conversations Using a Hands-Free Embedded Wireless Device While Driving: Effect on Airbag-Deployment Crash Rates.” Risk Analysis 29 (2): 187–204.10.1111/risk.2009.29.issue-2