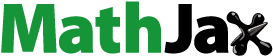
Abstract
The fashion industry cannot use 3 D Body Scanning to create custom garment patterns because its measurements fail to meet ISO 20685:2010’s tolerances. To advance 3 D Body Scanning’s precision, we present Gryphon: an algorithm that removes the two most extreme measurements from five body scans; removing potentially erroneous data. We assess Gryphon’s precision against current industry practice, determine if consecutive and non-consecutive data capture influences precision, and determine 3 D Body Scanning’s inherent imprecision inherent. We analyse 97 participants over 121 industry-standard measurements for consecutive and non-consecutive data-capture through MANOVA statistical analysis. Under current industry practice, only one measurement meets ISO 20685. However, under Gryphon and consecutive scanning, 97.5% of measurements meet ISO 20685. We also prove that the body’s in-scan movement does not affect reliability. Ultimately, we offer the fashion industry, ergonomists, and practitioners an accessible method to increase 3 D Body Scanning’s precision at a level unavailable under previous methods.
Practitioner Summary: Ergonomists need precise data, yet we prove 0% of 3 D Body Scanning’s measurements meet ISO 20685’s tolerances. Our analysis of 97 participants scans, shows consecutive scanning is necessary to achieve data suitable for anthropometric applications. We develop the Gryphon process with consecutive scanning, making 97.3% of measurements meet ISO 20685.
Abbreviations: ISO: International Organisation for Standards; Three Dimensional: 3D; MANOVA: Multivariate analysis of variance
Graphical Abstract

Introduction
Industrial ergonomists need precise anthropometric data to design a person’s physical interaction with socio-technical systems. Precise measurements are essential, whether it is developing industrial mass-customisation systems (Liu et al. Citation2019) or building ease into clothing (Thomassey and Bruniaux Citation2013). To aid such developments, ergonomists have conducted many anthropometric surveys since the field’s inception (e.g. DTI Citation1998; Gordon, Churchill, et al. Citation1989; ISO Citation2017). Surprisingly, only 8% of studies consider accuracy, reliability, or precision (Viviani et al. Citation2018).
Garment development practitioners, industrial ergonomists, and scientists depend upon 3 D Body Scanning to collect their anthropometric data. Body Scanning – otherwise known as optical imaging or surface imaging, and not to be confused with MRI and CT scanning – is the detailed measurement of the human body. In measuring the human body, Body Scanning uses diverse technologies, including stereoscopic vision, structured-light scanning, and 3 D depth-sensing (Bye, LaBat, and Delong Citation2006; Bonnechère et al. Citation2014). In doing so, garment development practitioners, industrial ergonomists, and scientists perceive Body Scanning as being a precise measurement tool (Kuehnapfel et al. Citation2016; Danckaers et al. Citation2019) to uncover content validity or ‘ground truth’. Faith in 3 D Body Scanning’s precision leads many garment manufacturers to attempt Body Scanning’s application in their product development process (Su et al. Citation2017; D’Apuzzo Citation2007; Chan, Fan, and Yu Citation2005; Lu, Song, and Li Citation2014). However, 3 D Body Scanning, like manual measurement, has limited context in garment development practice (Gill et al. Citation2017) and inherent measurement errors.
Body Scanning’s ability to extract anthropometric measurements with equal confidence to manual techniques underpins its application in garment development. Istook and Hwang (Citation2001, 120) even state: ‘the ability to customise garments for fit is directly tied to the availability of a comprehensive, accurate set of measurements’. To obtain relevant data for garment development, anthropometric measurements must be accurate and precise (Park et al. Citation2009; Bye, LaBat, and Delong Citation2006; Liu et al. Citation2019). Since measurement error is unavoidable (Ulijaszek and Kerr Citation1999), Body Scanning’s appropriateness links to its ability to measure within relative error boundaries. Relative error boundaries are relative precision.
Practitioners expect Body Scanning to produce data with a precision comparable to manual methods’ precision (Wang et al. Citation2011). To assess precision’s suitability, ISO 20685 (ISO Citation2010) sets strict tolerances of 0.3–2.2 cm (M = 0.8, SD = 0.4) – as defined by ANSUR I and II (Gordon, Churchill, et al. Citation1989; Paquette, Gordon, and Bradtmiller Citation2009). If Body Scanning cannot meet ISO 20685’s tolerances, then the ergonomist cannot conduct ‘the theoretical and fundamental understanding of human behaviour and performance in purposeful interacting with [the] socio-technical system’ with confidence and scientific rigour (Wilson Citation2000, 557).
Body Scanning manufacturers claim their technology is ‘more reliable and accurate’ than traditional methods (TC2 Citation2020), or assert ‘surface measuring within ±5 mm of precision’ (SizeStream Citation2020). As ISO 20685 (ISO Citation2010) sets strict tolerances of 0.3–2.2 cm (M = 0.8, SD = 0.4) – based on manual method best practice – the Body Scanning industry is implying their technology achieves precisions comparable to manual methods without adjustment or removal of outliers. Practitioners, industrial ergonomists, and scientists take these claims on faith. Assuming their tools are precise, they do not reference their tool’s precision with a rigorous critique (e.g. Lu, Song, and Li Citation2014). Nevertheless, how Body Scanning can achieve this precision is ill-defined (Dianat, Molenbroek, and Castellucci Citation2018).
In the last decade, few papers have assessed measurement precision. None of which solve the fashion industry’s need for precise anthropometric data collection. Medina-Inojosa et al. (Citation2016) only measured the hips and seat, omitting all other measurements of importance to garment construction and only considered the difference between manual and 3 D Body Scanner data. Multiple authors (Bullas et al. Citation2014; Conkle et al. Citation2018; Bullas et al. Citation2016; Bragança et al. Citation2018) calculate error through Technical Error Measurement (TEM), yet only use between three and four measurements instead of the 20 TEM requires. Bullas et al. (Citation2014), in particular, analysed the leg segment through five measurements – upper-thigh circumference, mid-thigh circumference, knee circumference, mid to upper-thigh length, and knee to mid-thigh length. Nevertheless, Bullas et al. (Citation2014) provided a restricted study that failed to indicate sufficient precision. TEM also ‘eliminates the effect of the size of the measurements thus allowing comparison of replicability among different sites or types of measurements’ (Branson et al. Citation1982, 141). TEM is, therefore, inappropriate for garment construction as ANSUR I and II set absolute tolerances irrespective of between-subject measurement variation. Lee et al. (Citation2013, 879) even took additional manual measurements ‘until the difference between the two measurements was <2 mm’ to achieve content validity.
Despite Bullas et al. (Citation2014, Citation2016) exalting Body Scanning’s high precision, Conkle et al. (Citation2018) states ‘further development of the scanning and processing software is needed before making policy and clinical practise recommendations on the routine use of 3 D imaging for child anthropometry’. Other studies, similarly, show Body Scanning’s precision does not satisfy ISO 20685’s tolerances (Han, Nam, and Choi Citation2010; Jun-Ming Lu and Wang Citation2010; Mckinnon and Istook Citation2002; Bragança et al. Citation2018). While developments in reducing scan subjects movement can increase precision (Schwarz-Müller, Marshall, and Summerskill Citation2018), 3 D Body Scanners do not control for hardware and software variables that contribute to imprecision. None of these studies provide a solution to Body Scanning’s – claimed – imprecision.
This paper aims to advance Body Scanning’s precision to meet ISO 20685 (ISO Citation2010) tolerances. To address our research aim, our objectives are:
Test measurement precisions of traditional scanning methods and our Gryphon process under consecutive and non-consecutive scan conditions at 3σ against allowable error tolerances to establish measurement precisions of all four scanning methods.
Compare consecutive and non-consecutive scanning methods through MANOVA between groups analysis to understand if consecutive body scanning produces more precise measurements than non-consecutive (traditional) scanning methods.
Compare human body scans with those of static body-forms in order to understand the degree of imprecision inherent in the Body Scanning method as opposed to human movement.
In addressing our objectives, we analysed 97 UK scans (male and female) over 121 industry-standard body measurements. We subsequently developed our new algorithm – Gryphon – to remove erroneous outliers that cause measurement imprecision. Gryphon is a free-to-use algorithm that runs in consecutive scanning’s post-processing, working with all existing Body Scanning hardware and software.
Theoretical background
Appropriateness, accuracy, and precision
Appropriateness, accuracy, and precision are essential measures of a machine’s technical suitability in engineering. For an engineer to assess Body Scanning’s suitability for garment development, empirical research must verify these three characteristics suitability.
Appropriateness is whether the engineer takes the correct measurement (DeGarmo, Black, and Kohser Citation2003). The waist has, for example, multiple definitions (Gill et al. Citation2014), so an engineer must ensure that they take a suitable waist for their purpose. As this paper is general to Ergonomics and Scientific communities, we focus on measurements from ISO 13402:2 (BSI Citation2002) – the standard outlining the primary and secondary dimensions of clothing size designation. By focussing on ISO 13402:2 the measurements are appropriate for ergonomics, scientific, and design communities. We used ISO 13402:2 to rank key measurements for their application as key sizing dimensions. While human factors practitioners regularly use ISO 7250, we could not use ISO 7250 as it neglects to define hip, a critical sizing dimension.Footnote1 Measurements are chosen as those defined by scanning that benchmarks its measurements from ISO 8559. Measurement selection is in accordance with its application in body worn product development. Furthermore, Gill et al. (Citation2017) prove that Body Scanning software is appropriate in the measurements it extracts.
Accuracy is the closeness of measurements taken by a tool to their ‘true’ value (ISO Citation1998) or a standard value accepted as truth (Roebuck, Kroeme, and Thomson Citation1975). An engineer may, for example, require 3 D Body Scanner to extract the waist at the ‘Small of the Back + 3 cm’. If, however, the body scanner extracts measurements at the ‘Small of the Back + 6 cm’ (3 cm off-target), then the machine is not accurate. As Viviani et al. (Citation2018) identify, accuracy and precision are often confused. The two concepts are interlinked, as Roebuck et al. (Citation1975) outline accuracy is best approximated through multiple use of precise tools in controlled environments. Precision can dictate accuracy. Nevertheless, Simmons and Istook (Citation2003) prove that Body Scanning software is accurate through its anatomical landmark placement.
Precision is the degree to which an engineer can repeatedly take a measurement, with variation occurring within specified allowances, or the closeness of agreement among a set of results (ISO Citation1998). Engineers define the closeness of agreement as three times the standard deviation of the norm; (DeGarmo, Black, and Kohser Citation2003, 197).
represents the spread of 99.73% of all expected measurements: less than three measurements in 1000 exceeding the allowable error. Precision, therefore, relates to an engineer’s confidence in their measurements. Precision is, however, the least supported characteristic of Body Scanning suitability for garment development. Viviani et al. (Citation2018) identify that the published literature often confuses prevision with reliability since there are several different, yet similar definitions used. Norton and Olds (Citation1996) define precision as a characteristic specific to a measurement taken on a specific body part using a specific measurement technique. Reliability is, however, precision when dependent upon functions of physiological variation that can modify the measure’s reproductivity (Norton and Olds Citation1996; Sicotte et al. Citation2010; Ulijaszek and Kerr Citation1999). Precision and reliability are, therefore, calculated in the same way. Within this paper, we use the term precision when referring to 3 D Body Scanning’s measurements, except when considering the influence of physiology – when comparing human subjects to body forms.
Accuracy and precision are interconnected, resulting in four measurement suitability conditions, as visualises.
Figure 1. Accuracy and precision’s relationship in body scanning measurements.
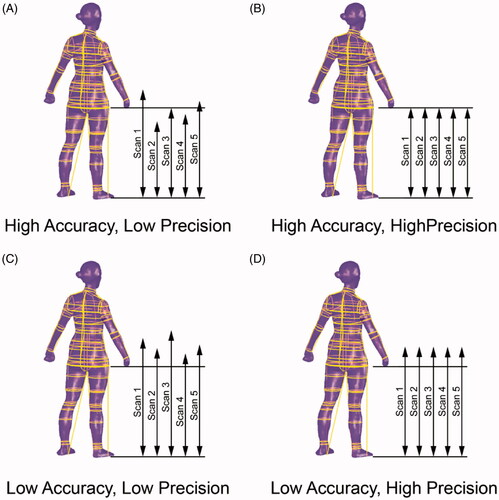
While an engineer requires high precision and high accuracy (measurement condition B), only Simmons and Istook’s (Citation2003) anatomical landmark placement establishes high accuracy. Incorrect landmark placement means the scanner takes a different – inaccurate – measurement to that specified; see . Taking an inaccurate measurement by just a few cm significantly influences the anthropometrists’ result (Gill et al. Citation2014). Furthermore, Body Scanning’s ability to meet ISO 20685’s tolerances is, however, inconclusive. Technicians cannot, therefore, know if Body Scanning is in measurement condition A or B.
Allowable error for garment development
To consider Body Scanning as suitable for garment development, empirical research must verify its precision against industry’s allowable error tolerances. ANSUR I and II (Paquette, Gordon, and Bradtmiller Citation2009; Gordon, Churchill, et al. Citation1989) are the body scanning industry’s benchmarks for assessing manual measurement’s allowable error. ANSUR calculates allowable error from the Mean Absolute Differences (MAD) of inter-observer error.
ANSUR I and II represent the most comprehensive study to date of an expert’s precision in measuring the human body through manual methods. Calculating allowable errors from inter-observer error, however, lacks scientific rigour; with the mean differences based upon just 10 participants and no cheques of data collected on the larger participant sample. In ANSUR 1, Gordon et al. (Citation1989) compared the differences between paired experts’ measurements after removing erroneous measurements from the data. ANSUR 1’s foundational data does not, therefore, represent actual – unedited – measurement capture.
While manual adjustment is an established practice within manual measurements, their interference influences their results. Gordon et al. (Citation1989) removed the data’s erroneous measurements if they perceived the error’s origin to be explainable. They also removed errors based on high MAD scores – the largest differences observed between measurement pairs. High MAD scores result from the participant’s breathing cycles, body positioning, and landmark types (soft tissue or skeletal); factors practice knows of, but are absent within allowable error definitions (Simmons and Istook Citation2003).
Schwarz-Müller et al. (Citation2018) developed a human-support frame for 3 D Body Scanning, reducing the participant’s movement and, therefore, increasing precision through a lower MAD score. The measurement precision was, however, only tested on 15 subjects with three consecutive scans, and removed natural posture variation. Natural posture variation contributes to clothing design and fit requirements, making Body Scanning less suitable for garment development. As the scanning process is already complicated and often uncomfortable for scan subjects (Januszkiewicz et al. Citation2019), additional equipment is unlikely to improve this situation.
ANSUR I and II represent the current benchmark for human measurement. ANSUR 1’s errors being adopted by the ISO 20685 (ISO Citation2010) testified to these benchmark’s authority. ISO 20685 implies measurements with a precision outside of ANSUR’s tolerances are unsuitable for garment development. With Body Scanning specific tolerances current absent from practice, ISO 20685 – and thus ANSUR 1’s allowable errors – represent the most accessible guidance for Body Scanning precision.
Origin of error
Body Scanning uses non-contact methods to collect the human body’s virtual surface outline. At multiple stages of the process, an error can occur. First, errors can occur in the capturing of the point cloud or the body surface’s rendering. These are often clear, meaning a scanning technician can identify errors and, therefore, re-scan the participant. After the virtual avatar’s creation, the software places landmarks and measurements using algorithms based on features of the point cloud. During the landmark placement, an error can occur if the software places measurements at different locations between scans. Changes in the participant’s posture – or movement through breathing – between scans also causes differences in landmark placement, resulting in different measurements of the same scan subject (Schwarz-Müller, Marshall, and Summerskill Citation2018).
Sources of error in the body scanning process could occur from capture devices, point cloud rendering, avatar development, automated measurement based on an algorithm, position, and the scan participant’s posture.
Typical manual measurement techniques consider repeat measurement precision as intra – and inter – expert measures. The practitioner, in these instances, discounts measurements they consider having significant errors, and allowing repeat measures to reach a tolerance threshold. ANSUR I and II base their tolerances on such inter-expert procedures. Nevertheless, Body Scanning has no way to discount such erroneous measurements automatically.
Our Gryphon process builds on this, recognising the body scanner’s uniqueness and seeks to define an approach to meeting ANSUR’s precision; mindful of ANSUR’s development, existing manual measurement techniques, and their sources of error. Gryphon further allows all scans to be subject to precision cheques. Manual methods do not provide precision cheques with the same volume and depth of data, rigorously checking each measurement.
Material and methods
Setting and sample
We collect 97 human scans to determine precision differences between consecutive (n = 66) and non-consecutive (n = 31) scanning methods. To assess how precision depends on a natural variation of the human body, we scanned five Body forms three times each; 15 scan sessions in total. We visualise our study design in .
Figure 2. Study tree. Assets from Muammark (Citation2017).
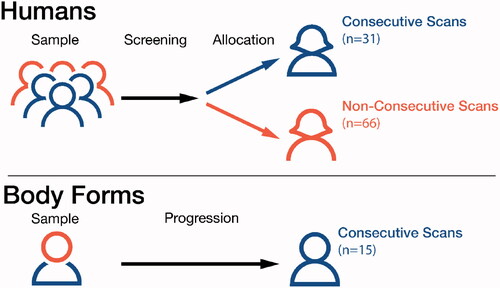
To ensure our results are robust, we calculated the sample size to achieve a power of a benchmark demonstrating suitable for robust statistical analysis (Cohen Citation1988). Our sample exceeds the minimum sample size required for data analysis through a between groups MANOVA – as used in this study – calculated to be 82 per group using G*Power (Faul et al. Citation2007) –
Our sample of 97 participants exceeds the minimum sample size by 15 persons as each participant is in Groups 1 and 2, making 97 participants per group.
The sample comprised Males (n = 30) and Females (n = 67), aged 19 to 61 (M = 30.7, SD = 11.1), with White (n = 63), Chinese (n = 20), AsianFootnote2 (n = 4), Black (n = 3), and other/not stated (n = 7) – Under the UK’s NHS (Citation2001) criteria. Our samples were of mass () and height (
). Using Waist-To-Height Ratios to indicate body-fat – under Schneider et al.’s (Citation2010) categorisations – our sample contained extremely slim (n = 25), slim (n = 9), healthy (n = 10), overweight (n = 5), very overweight (n = 2), obese (n = 15), and unknownFootnote3 (n = 31) participants; (
We recruited participants between 2016 and 2018 from a convenience sample of participants attending open body scanning sessions held at a central Manchester (UK) location; within the author’s university buildings.
We selected five female Alvanon dress forms, representing UK sizes 6 to 24 and with variations in shape. Each dress form was scanned 15 times to provide a comparable sample. While this sample is low, Alvanon manufactures dress forms with realistic bodies that model symmetrical and standardised shapes. These standardised shapes show less variability than a population, providing a consistent posture, pose, and reference point during scanning.
Data collection
We applied Gill et al.’s (Citation2016) scan protocol in data collection with a Size Stream SS14 Body Scanner. Scientific research (e.g. Gill and Parker Citation2017) and the garment construction industry widely use the Size Stream SS14 scanner. The Size Stream SS14’s broad use makes it appropriate for this paper’s investigation and a generalisable reputation of Body Scanning Technology. We did, however, adjust the scanner to incorporate consecutive and non-consecutive capture methods, rendering the scans at a 20,000-polygon resolution.
Before participants attended scan sessions, they were provided with a set of guidelines regarding the body scanning requirements concerning underwear, jewellery, and hair. All participants were asked to use non-padded and non-compression underwear to avoid any distortion of their natural body shape and be barefoot. The research ethics prohibits scanning anybody without underwear and excludes any inspection because of privacy issues.
Before data collection, the researcher explained the scanning protocol to the participant. We then guided participants through adopting the correct scan-posture. Correct scan-posture means the participant’s feet were 20 cm apart to meet the protocol’s guidance; as Gill and Parker (Citation2017) and ISO 20685 (ISO Citation2010). We communicated the correct scan-posture through prominent floor markings.
Our scanning processes:
Non-consecutive scanning: Participants enter the scanning booth and adopt the scan-posture. Between each of the five scans, participants remain in the scanning booth but can relax or change position before again adopting the scanning posture.
Consecutive scanning: Participants enter the scanning booth and adopt the scan-posture. We set the scanner to capture five scans while the participant remained in the scan-posture for the data capture period (30 s).
As posture influences scan repeatability (Gill and Parker Citation2017; Schwarz-Müller, Marshall, and Summerskill Citation2018), posture was standardised for all participants throughout this study. We exported all scan data into text files (.txt), with processing in Excel (Microsoft Citation2019) and statistical analysis in SPSS (IBM Citation2019).
We assess the 121 default scan measurements’ importance to garment-sizing criteria against ISO 13402:2 (BSI Citation2002); outlined in Appendix A. Where ISO 13402:2 did not mention a measurement deemed necessary to garment development, we use existing methods of pattern construction (regarding guidance) as benchmarks. After analysis, we rank measurementsFootnote4 as importance level 1–4:
Essential in sizing and pattern construction
Essential in pattern construction
Often required in Pattern construction
Possible to use in pattern construction
Data processing (Gryphon)
Post-processing operations
Once the data were collected, each scan was visually assessed to ensure surface data was free of errors and landmarks and measurements were placed correctly; as used in previous research (Gill and Parker Citation2017). Visually inspecting scans and extracted measurements is standard practice for any data collected through body scanning, and when using automated measurement extraction. We checked accuracy by screening scans for correct measurement placement. We also checked the scanner using an alignment device that calibrates it.
Gryphon processing
Once the measurement sets produced by the scanner have been visually verified, measurements from different sets (scans) are post-processed. We name our data processing method: Gryphon. The Gryphon process analyses each measurement set to identify, and remove, the two extremes of the data. Removing these points is an effective way to reduce the variability within the data because these two points are least likely to represent an accurate measurement.
Gryphon is a form of windowed outlier removal, maintaining the original set of measurement’s median average. We use the median average as a central anchor for the symmetric window. The median average defines the window width to capture the three nearest measurements to the median while excluding the remaining two as outliers ().
Figure 3. Illustration of the outlier removal process. Solid circles represent the five data points in the set. The solid blue line represents the median of the set. Dashed red box illustrates the symmetric window with the red data points included in the reduced set and the black points excluded.
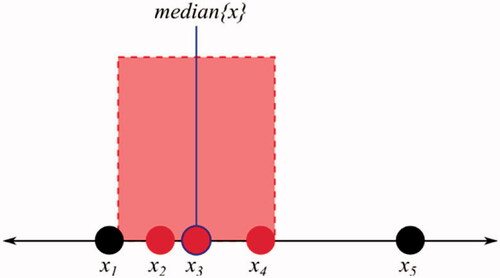
Our Gryphon process is appropriate since sets of Body Scanning measurements typically cluster around an appropriate average, but a single outlying value often skews the data into a large spread. These outliers disproportionately affect the mean value, making the mean value – as used in standard consecutive scanning processes – unsuitable as an anchor for a static window removal procedure. Reliance on, and maintenance of, the data set’s median reduces the data’s variance while maintaining the average. Precision is, therefore, increased.
We have made the Gryphon source code available for freeFootnote5 – with usage instructions – via Github: https://github.com/UoMResearchIT/Gryphon.
Considering a set of data for a single measurement with a median
Gryphon first calculates the absolute distance of each measurement from
to produce a second set
Excluding the two largest values then gives a third set
The retained values are then the corresponding values from the set
Statistical analysis
We do not analyse appropriateness because the 121 measurements we use in our study are standard within the Body Scanning’s software (Sizestream Citation2019) and comply with ISO 13402:2 (BSI Citation2002); proving appropriateness as we are taking the right measurements. We do not analyse the accuracy, as Gill et al. (Citation2017) has already established Body Scanning’s accuracy within a garment construction context.
Numerous studies use Technical Error Measurement (TEM) to determine precision. Nevertheless, TEM ‘eliminates the effect of the size of the measurements thus allowing comparison of replicability among different sites or types of measurements’ (Branson et al. Citation1982, 141). TEM is, therefore, inappropriate for this study as ANSUR I and II set absolute tolerances recognising the way a body mass and size can be accurately measured for garment construction, irrespective of between-subject measurement variation. TEM also does not consider error according to each measurement’s uniqueness – some measurements have higher variability due to the area of body measurement and its mobility. Disregarding the measurement’s magnitude is likely to produce precision metrics that meet ANSUR I and II, yet produce less precise measurements in reality.
We calculate the reliability as representing a precision of 99.73% confidence in the measurement. Calculating reliability as
is a standard practice in engineering and gives an absolute precision of the measurements taken (DeGarmo, Black, and Kohser Citation2003, 197). We then compared precision, as calculated through Equation 1, against the rigorous allowable error (
) criteria (Gordon, Bradtmiller, et al. Citation1989; Claire C Gordon et al. Citation2012; ISO Citation2010).
Equation 1. Equation for Precision; σ = Standard Deviation, Var = Variance, n = Number of Participants
We investigated the difference in reliability between Body Forms (n = 15) and Humans (n = 66) scan subjects (non-consecutive scanning) through a one-way between-groups MANOVA. The independent variable was the scan subject (human or body-form). We chose fourteen dependent variables to represent the most critical measurements for garment development. We judge measurements for their importance to garment construction based on BSI (Citation2002).
We investigated the difference in precision between Consecutive (n = 31) and Non-Consecutive (n = 66) Body Scanning data capture methods through a one-way between-groups MANOVA. Thirty dependent variables represented the most important measurements for garment development; see Appendix A, measurements with importance level 1 or 2. The independent variable was a data capture method (consecutive or non-consecutive).
For all MANOVA tests, we use only the right side of the body to reduce the number of measurements and meet the tests’ assumptions. We conducted preliminary assumption testing, checking for the normality, linearity, univariate and multivariate outliers, homogeneity of variance-covariance matrices, and multicollinearity. We found no serious violations. The alpha was set to to reduce further the chance of a Type I error. We also conducted Post-hoc power analysis with G*Power (Franz Faul et al. Citation2020) to check all calculations exceeded the of π ≥ 0.8 threshold (Cohen Citation1988). All calculations achieved π ≥ 0.9, suggesting the lack of Type II errors.
Potential co-variables have no association with this study’s 121 measurement’s precision. We investigated association through MANOVA tests for ethnicity (), age (
), gender (
), and body fat expressed through the Waist-to-Height ratio (
).
Results
Precision enhancement of 3 D body scanning
This section assesses the percentage of scan measurements to meet ISO 20685:2010s tolerances under consecutive and non-consecutive scan methods, and industry-standard and Gryphon methodologies.
Our results prove Gryphon – with consecutive scanning – is more precise than manual measurement methods.Footnote6 Overall, 353% more reliable than the standard consecutive scanning methods, and 12,088% more reliable than the standard non-consecutive scanning methods (). Appendix A lists ANSUR’s tolerances relative to the measurement they apply to, including Gryphon’s improvements over standard practice. Highlights include a 32.5 cm difference between standard-consecutive and gryphon-consecutive knee circumference, and a 348.9 cm difference between standard-non-consecutive and gryphon- non-consecutive sleeve length.
Figure 4. Percentage of all measurements suitable for garment development; Precision = 3σ.
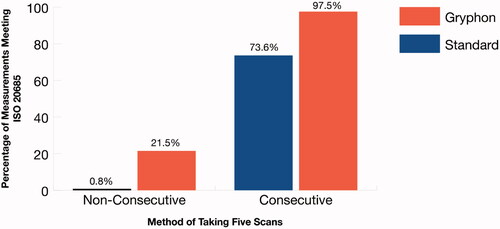
With unprocessed (industry-standard) data, only one measurement extracted non-consecutively – [100] CB Neck to Shoulder Blades [Tape] – achieved a suitable level of precision for garment development; measured at the industry standard 3σ level. Only 21.5% of all consecutively extracted measurements were suitable for garment development use: at the 3σ level. 73.6% of all non-consecutively Gryphon processed measurements were suitable for garment development: at the 3σ level. An unprecedented 97.5% of all non-consecutively Gryphon processed measurements were suitable for garment development: at the 3σ level. Only three measurements did not meet the allowable error tolerances; Right Arm Length1 (0.3 cm short), Back Horizontal Shoulder Width3 (by 1.9 cm) and Left Outer Armhole Circumference3 (0.2 cm short).
The appendix displays a full list of body measurements and their associated precision – in line with Allowable Error. The Left Arm Length1 and the Right Outer Armhole Circumference3, however, offer a precision within ANSUR’s allowable error. These measurements’ precision suggests the scanner’s internal calibration process has an issue; instead of the scanning technology.
Consecutive scans with Gryphon processing were better than ANSUR’s allowable errors by an average of 0.52 cm (). Given the allowable error tolerances, the Gryphon process achieves a 67% increase on the achievable precision for garment development. visualises Gryphon’s ability to produce measurements with more a precision greater than manual methods.
Figure 5. Gryphon + five Consecutive Scans against ANSUR I and II’s Allowable Errors.
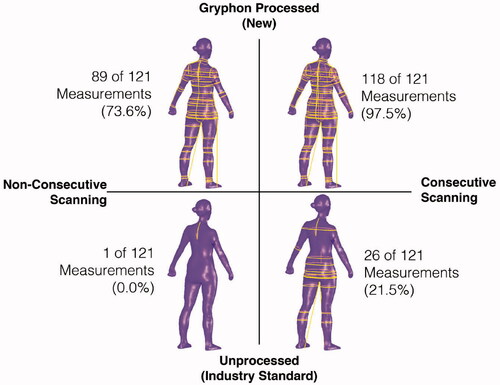
Consecutive vs non-consecutive scanning precision
In this section, we assess how consecutive and non-consecutive scan methods produce different levels of precision. We determine this by comparing the precision of consecutive and non-consecutive human scans.
Consecutive and non-consecutive scan methods showed a statistically significant difference between the precision when investigated through MANOVA; Considering the results measurement-by-measurement, only six measurements reach statistical significance; with a Bonferroni Correction alpha level of 0.002; see .
Table 1. MANOVA, consecutive and non-consecutive precision differences categorised by their importance to garment development.
All significant outcomes achieved a large effect size except for [145] Hinged Upper Bust Circumference3 and [133] Axilla Chest Circumference [Tape]2 that achieved a medium effect size (Cohen Citation1988). The average difference between consecutive and non-consecutive scans is 0.33 cm. Given that many allowable errors are as tight as 0.5 cm, this difference is influential within these precision variances. The range of measurements gained between scans – up to 32.9 cm – further emphasises Body Scanning’s imprecision.
Reliability: human vs body form
In this section, we assess how reliability depends on a natural variation of the human body. We determine this by comparing human to body form scans.
Body Forms and Human scans showed a statistically significant difference between the reliability of scan measurements when investigated through MANOVA; Considering the results for scan measurement reliability measurement by measurement, only nine measurements reach statistical significance; with a Bonferroni Correction alpha level of 0.004: .
Table 2. MANOVA: Human and Body Form reliability differences categorised by their importance to garment development.
All significant outcomes achieved a large effect size except for Low Waist Circumference Pitched [Tape]1 that achieved a medium effect size (Cohen Citation1988). The average difference between Human and Body Form scans is ± 0.56 cm; besides the body measurement’s reliability. A ± 0.56 cm difference is influential within reliability variances as many allowable errors are as tight as ± 0.5 cm.
Discussion
Garment development practices, and anthropometric research, require precision within manufacturing processes (Lu, Song, and Li Citation2014; Park et al. Citation2009; Istook and Hwang Citation2001; Bye, LaBat, and Delong Citation2006). Garment development practices have yet to adopt Body Scanning within the design engineering process because of the high variability in measurement confidence and appropriateness. The Gryphon processes must, therefore, deliver measurement confidence through increased precision to justify Body Scanning’s inclusion in industrial applications.
Our results prove that practitioners cannot have confidence in measurements captured from a single Body Scanning capture, as is common in standard scan practices. Our results prove that through standard scan practices, only one measurement – [100] CB Neck to Shoulder Blades [Tape] – met ANSUR’s allowable error tolerances (ISO Citation2010; Claire C Gordon et al. Citation2012). A practitioner may assume the scan participant’s body movements as causing imprecision. This assumption is, however, incorrect. The large effect size differences between only 9 of the 121 human and body-form measurements (42.9%) show the most significant limitation in Body Scanning is the technology, not the subject. Even when a practitioner extracts five consecutive scans, 21.5% of Body Scanning measurements meet the stringent industrial requirements.
3D Body Scanner manufacturers must incorporate consecutive scanning into their analysis software. This improvement, however, only allows a practitioner to place confidence in less than a quarter of their required measurements. 3D Body Scanner manufacturers must, therefore, apply further enhancements for their garments to collect data within ANSUR’s allowable error tolerances. These tolerances allow for typical users to generate relevant data without recourse to testing and checking of each scan.
Theoretical implications
Data imprecision is primarily caused by erroneous measurements. Erroneous measurements occur because of the 3 D Body Scanner’s incorrect placement of anthropometric landmarks or the physical positioning of body parts which increase measurement paths against the algorithms. The 3 D Body Scanner’s incorrect landmark and measurement placement, in this study, causes measurements with a range of up to 32.9 cm (). These significant outliers skew the data. Consecutive, and non-consecutive, scan procedure’s low precision with standard scan protocol testifies to the frequency at which erroneous measurements occur. To achieve ANSUR’s required precision, practitioners must identify and remove erroneous values.
Our Gryphon process – with consecutive scanning – generates the most precise measurements for garment development. Gryphon removes the potentially erroneous measurementsFootnote7 in a manner that allows for low user-skills and reduces the need for manual post-processing. These tolerances represent the best manual measurement practices, and thus the benchmark of human achievement in manual measurements. ANSUR’s tolerances are more stringent than errors for manual measurement in general clothing practices, where measurers undergo less rigorous training. Where Body Scanning with Gryphon falls short of meeting the ANSUR representation of expert human ability lies in the remaining three measurements that did not achieve suitable precision. The remaining three measurements – while less precise at the 3σ level, denoting 99.73% confidence – relate to one side of the body, or result from difficult to place landmarks. None are crucial measurements in sizing or pattern construction. With improvements in landmark placement, these small inconstancies shall disappear, and represent an important area of development with the technology.
Through consecutive scanning – and the Gryphon process’s application during point cloud computation - our methodology transforms Body Scanning into a process more than precise enough for garment development ().
Figure 6. A flow chart of Gryphon scanning process.

Industry implications
We offer industrial ergonomists, designers, and practitioners a method to convert faith in Body Scanning’s measurement precision to trust in Body Scanning’s measurement precision. Gryphon makes precise data attainable. While some of the extreme differences our analysis found can be neglected to extreme outliers – for example, 348.9 cm difference between standard-non-consecutive and gryphon- non-consecutive sleeve length – more subtle outliers occur frequently. An experienced practitioner may be able to identify extreme outliers when manually checking every measurement on every subject. Nevertheless, they are likely to miss more understated errors. Rigorous and laborious manual checking by an experienced practitioner contradicts 3 D body scanning’s central selling point of constant speed, accuracy, and simplicity (SizeStream Citation2020). Gryphon solves this problem.
With Gryphon, and consecutive scanning, the ergonomist can conduct ‘the theoretical and fundamental understanding of human behaviour and performance in purposeful interacting with [the] socio-technical system’ with confidence and scientific rigour (Wilson Citation2000, 557).
With Gryphon and five consecutive scans, industry practitioners, designers, and scientists can have confidence in anthropometric measurements at a level difficult to achieve by other methods. With reduced error, designers measuring with the Gryphon process will require less manual processing, error checking, or re-scanning than with standard scanning methods – reducing Body Scanning’s time and complexity. The reduced manual workload that Gryphon facilitates also makes scanning tools more accessible to garment development practitioners, industrial ergonomists, and scientists, some often with little skill in measurement or statistical analysis.
Translating 3 D Body Scan data into garment patterns is workable yet required considerable practitioner intervention in screening data for erroneous measurements. While screening for erroneous measurements is possible within a research context, manual intervention is impractical in mass customisation. The precision improvements Gryphon offers, therefore, remove human intervention’s necessity, allowing an automated solution to exist for mass customisation. From a design perspective, designers can develop customised garments – such as 3 D printed safety equipment (Harte and Paterson Citation2018) or automated garment patterns for jeans (Liu et al. Citation2019) – through a service that captures forms with minimum errors. None of these mass customisation options are possible without the precision Gryphon offers. As Gryphon offers a precision of – on average − 0.52 cm () below than ANSUR requires, designers can work with greater efficiency, with reduced need to re-scan, re-design, and re-produce data. Designers can also have confidence that their garments fit the customer first time. Scientists, engineers, and researchers use Body Scanning in anthropometric surveys to a higher precision than previously possible. Increasing anthropometry precision will strengthen population survey’s rigour and consistency. These population surveys are vital since they influence garment design, manufacturing, and consumer safety (e.g. DTI Citation1998; Kim, You, and Kim Citation2017; da Silva, Halpern, and Gordon Citation2017).
While this paper proposes a substantial advancement in Body Scanning precision, practitioners must also adhere to the correct best practice. A practitioner can only achieve consistency and accuracy by ensuring scan participant’s stance is uniform and taking measurements according to best practice (Gill and Parker Citation2017; Gill, Hayes, and Parker Citation2016). Viviani et al. (Citation2018, 1) already established such a call, that practitioners must ‘use methods for assessing measurement error, since these data are often used to design clothing, design workspaces and calibrate non-manual methods such as 3 D scanners’. Gryphon removes this error, increases precision, and makes Body Scanning more suitable for industrial ergonomics application.
We recommend that Body Scanning manufacturers implement consecutive scanning – with five data captures – and include Gryphon within their software’s data processing. Doing so allows Body Scanning to meet ISO 20685:2010 to engineering tolerances of SizeStream – who manufacture the SS14 scanner we use in this study – already includes multiple data captures within their software as standard. If any existing scanning technology cannot meet, or exceed, their precision using five consecutive scans and Gryphon, then practitioners cannot consider these scanners as more precise than the SizeStream scanner. If other Body Scanning manufacturers rise to meet this challenge, then the body scanning industry can achieve high-level demonstrable precision across different body scanners. Including our Gryphon process, the Body Scanning industry can produce data that is more precise than manual methods.
Garment development practitioners, industrial ergonomists, and scientists can – by using Gryphon and consecutive scanning – have confidence in their measurement’s precision. Their measurements will, in turn, be more precise than manual methods. Making Body Scanning more precise than manual methods may seem remarkable, but a 3 D body scanner enables measurement consideration in more detail and depth than any manual study. ANSUR 1 and ANSUR 2 could not collect the volume of data, as often, and so fast. Body scanning further provides data on depths, curves, and angles that are impractical for manual measurement – yet are essential for developing garment development (Scott, Gill, and McDonald Citation2019). Practitioners can now use these additional measurements for garment development as Gryphon assures their precision.
Conclusion
We set out to advance Body Scanning’s precision to meet ISO 20685 (ISO Citation2010) tolerances. Our results prove traditional scanning methods – taken with non-consecutive scanning – produces 0.0% of measurements meeting ISO 20685 (ISO Citation2010) tolerances. However, our Gryphon process – with five consecutive scans – produces 97.5% of measurements meeting ISO 20685 (ISO Citation2010) tolerances. We also prove that the human body’s variation does not affect Body Scanning’s reliability, outside of movement because of breathing.
This paper proves that existing Body Scanning hardware can produce excellent measurements for garment construction. Nevertheless, existing data capture methods – consecutive scanning – and a lack of post-processing allows erroneous measurements to skew data. Skewed data means existing Body Scanning technology produces measurements that fail ISO 20685 (ISO Citation2010) and are unsuitable for garment construction. Our Gryphon process reverses this image, making existing Body Scanning more than suitable for garment construction.
Garment development practitioners, industrial ergonomists, and scientists can now – by using Gryphon and five consecutive scans – obtain scan measurements at a precision exceeding manual measurements. With this increase in precise data, mass-customisation systems or tailors building ease into clothing based on scan data becomes practical. This new practicality stems from Gryphon’s monumental reduction in measurement error that has – until now – held such developments back.
Our work paves the way for Body Scanning to be applied throughout the fashion industry in every context where repeatably uncertain – or imprecise – measurements have, until now, halted the technology’s application. Outside of informing tailoring and garment construction, online fashion retail may – in the long term – benefit the most as Body Scanning integrates with virtual fit prediction and e-commerce services.
Limitations and further research
As we only used a 20,000-polygon scan outputs within this study, we can only hypothesise our results are generalisable to other, less common polygon outputs. Engineers and scientists may require further research to establish Body Scanning’s precision at resolutions different to the 20,000 polygon scans. Researching precisions for alternative body scanning technologies promises future research possibilities for the Gryphon’s broader application – and wider generalisation – in the anthropometric field.
As our sample was chosen through convenience/participant self-selection, our sample excludes persons outside of standard body-types. Non-standard body types may include persons with Parkinson’s, Scoliosis, or Phocomelia. While we assume our results are generalisable to the broader range of physiology, their less-common characteristics pose interesting problems for anthropometrics. Our paper’s generalisability is, therefore, limited to standard body-types. Industrial ergonomists will be interested in less common body type’s reliability within 3 D Body Scanning. Further research into how medical conditions influence scanning reliability shall advance our work, allowing designers to create more suitable custom garments and ergonomic products. Nevertheless, within this study’s boundaries, our methodology transforms Body Scanning into a tool more than suitable for garment development, scientific research, or ergonomic design than existing methods.
Disclosure statement
No potential conflict of interest was reported by the author(s).
Notes
1 ISO 8559 and ISO 13402 are apparel specific so provide focus in this area. ISO 7250 neglects the Hip and has no way to understand significance of measurement in application as ISO 13402 allows with regard to sizing.
2 Bangladeshi, Pakistani, or Indian are currently captured together as ‘Asian’.
3 Unknown Waist-To-Height ratios occur due to necessary measurements only available on non-consecutively scanned participants.
4 Measurement importance to garment development is denoted through a subscript number following measurements referenced within this paper. A full list of measurements categorised by importance to garment development is provided within the Appendix.
5 To cite Gryphon in your work, please reference this paper.
6 Manual measurement’s precision is ANSUR’s tolerances (Gordon, Churchill, et al. Citation1989; Paquette, Gordon, and Bradtmiller Citation2009). Exceeding ANSUR is exceeding manual methods.
7 118 of 121 measurements required for garment development meet ANSUR’s tolerances
References
- Bonnechère, B., B. Jansen, P. Salvia, H. Bouzahouene, V. Sholukha, J. Cornelis, M. Rooze, and S. Van Sint Jan. 2014. “Determination of the Precision and Accuracy of Morphological Measurements Using the KinectTM Sensor: Comparison with Standard Stereophotogrammetry.” Ergonomics 57 (4): 622–631. doi:https://doi.org/10.1080/00140139.2014.884246.
- Bragança, Sara, Pedro Arezes, Miguel Carvalho, Susan P. Ashdown, Ignacio Castellucci, and Celina Leão. 2018. “A Comparison of Manual Anthropometric Measurements with Kinect-Based Scanned Measurements in Terms of Precision and Reliability.” Work 59 (3): 325–339. doi:https://doi.org/10.3233/WOR-182684.
- Branson, Rosemary S., Yvonne E. Vaucher, Gail Grigsby Harrison, Vargas Margaret, and Thies Cole. 1982. “Inter- and Intra-Observer Reliability of Skinfold Thickness Measurements in Newborn Infants.” Human Biology 54 (1): 137–143.
- BSI. 2002. BS EN 13402-2:2002, Size Designation of Clothes - Part 2: Primary and Secondary Dimensions. London, UK: British Standards Institute.
- Bullas, Alice, Simon Choppin, Ben Heller, Sean Clarkson, and Jon Wheat. 2014. “Kinanthropometry Applications of Depth Camera Based 3D Scanning Systems in Cycling: Repeatability and Agreement with Manual Methods.” In Proceedings of the 5th International Conference on 3D Body Scanning Technologies, Lugano, Switzerland, 21–22 October 2014, 290–298. Ascona, Switzerland: Hometrica Consulting - Dr. Nicola D’Apuzzo. doi:https://doi.org/10.15221/14.290.
- Bullas, Alice M., Simon Choppin, Ben Heller, and Jon Wheat. 2016. “Validity and Repeatability of a Depth Camera-Based Surface Imaging System for Thigh Volume Measurement.” Journal of Sports Sciences 34 (20): 1998–2004. doi:https://doi.org/10.1080/02640414.2016.1149604.
- Bye, Elizabeth, Karen. L. LaBat, and Marilyn. R. Delong. 2006. “Analysis of Body Measurement Systems for Apparel.” Clothing and Textiles Research Journal 24 (2): 66–79. doi:https://doi.org/10.1177/0887302X0602400202.
- Chan, A.P., J. Fan, and W.M. Yu. 2005. “Prediction of Men’s Shirt Pattern Based on 3D Body Measurements.” International Journal of Clothing Science and Technology 17 (2): 100–108. doi:https://doi.org/10.1108/09556220510581245.
- Cohen, Jacob. 1988. Statistical Power Analysis for the Behavioral Sciences. 2nd ed. Hillsdale, MI: Lawrence Erlbaum Associates.
- Conkle, Joel, Parminder S. Suchdev, Eugene Alexander, Rafael Flores-Ayala, Usha Ramakrishnan, and Reynaldo Martorell. 2018. “Accuracy and Reliability of a Low-Cost, Handheld 3D Imaging System for Child Anthropometry.” Edited by Frank Wieringa. PLoS One 13 (10): e0205320. doi:https://doi.org/10.1371/journal.pone.0205320.
- D’Apuzzo, Nicola. 2007. “3D Body Scanning Technology for Fashion and Apparel Industry.” In Proceedings Volume 6491, Videometrics IX; 64910O, edited by J.-Angelo Beraldin, Fabio Remondino, and Mark R. Shortis. Washington, DC: SPIE. doi:https://doi.org/10.1117/12.703785.
- da Silva, Gilvan V., Manny Halpern, and Claire C. Gordon. 2017. “Anthropometry of Brazilian Air Force Pilots.” Ergonomics 60 (10): 1445–1457. doi:https://doi.org/10.1080/00140139.2017.1301575.
- Danckaers, Femke, Toon Huysmans, Ann Hallemans, Guido De Bruyne, Steven Truijen, and Jan Sijbers. 2019. “Posture Normalisation of 3D Body Scans.” Ergonomics 62 (6): 834–848. doi:https://doi.org/10.1080/00140139.2019.1581262.
- DeGarmo, E. Paul, J. T. Black, and Ronald A. Kohser. 2003. Materials and Processes in Manufacturing. 9th ed. Vol. 9th. Danvers, MA: John Wiley & Sons.
- Dianat, Iman, Johan Molenbroek, and Héctor Ignacio Castellucci. 2018. “A Review of the Methodology and Applications of Anthropometry in Ergonomics and Product Design.” Ergonomics 61 (12): 1696–1720. doi:https://doi.org/10.1080/00140139.2018.1502817.
- DTI. 1998. Adultdata: The Handbook of Adult Anthropometric and Strength Measurements. London, UK: Department of Trade and Industry.
- Faul, F., E. Erdfelder, A.-G. Lang, and A. Buchner. 2007. “G*Power 3: A Flexible Statistical Power Analysis Program for the Social.” Behavior Research Methods 39 (2): 175–191. doi:https://doi.org/10.3758/BF03193146.
- Faul, Franz, Edgar Erdfelder, Albert-Georg Lang, and Axel Buchner. 2020. G*Power. Düsseldorf, Germany: Heinrich-Heine-Universität. http://www.gpower.hhu.de/en.html.
- Gill, Simeon, and Christopher J. Parker. 2017. “Scan Posture Definition and Hip Girth Measurement: The Impact on Clothing Design and Body Scanning.” Ergonomics 60 (8): 1123–1136. doi:https://doi.org/10.1080/00140139.2016.1251621.
- Gill, Simeon, Christopher J. Parker, Steve Hayes, Kathryn Brownbridge, Paula Wren, and Anastasiia Panchenko. 2014. “The True Height of the Waist: Explorations of Automated Body Scanner Waist Definitions of the TC2 Scanner.” In Proceedings of the 5th International Conference on 3D Body Scanning Technologies, Lugano, Switzerland, 21–22 October 2014, edited by Nicola D’Apuzzo, 55–65. Ascona, Switzerland: Hometrica Consulting. doi:https://doi.org/10.15221/14.055.
- Gill, Simeon, Maryam Ahmed, Christopher J. Parker, and Steven George Hayes. 2017. “Not All Body Scanning Measurements Are Valid: Perspectives from Pattern Practice.” In 3DBODY.TECH 2017 - 8th International Conference and Exhibition on 3D Body Scanning and Processing Technologies, edited by Nicola D’Apuzzo. Montreal, Canada: Hometrica Consulting. doi:https://doi.org/10.15221/17.043.
- Gill, Simeon, Steven Hayes, and Christopher J. Parker. 2016. “3D Body Scanning: Towards a Shared Protocol for Data Collection.” In IWAMA 2016: 6th International Workshop of Advanced Manufacturing and Automation, edited by Yi Wang, Kesheng Wang, Jan Ola Strandhagen, and Tao Yu, 281–284. Manchester, UK: IWAMA. doi:https://doi.org/10.2991/iwama-16.2016.53.
- Gill, Simeon, Yuting Wang, Maryam Ahmed, Steven G. Hayes, Adrian R G. Harwood, and James Gill. 2018. “Scan to Pattern: How Body Scanning Can Help Transform Traditional Methods of Creating Pattern Blocks.” 9th International Conference in 3D Body Scanning, 1–5.
- Gordon, C. C., B. Bradtmiller, C. E. Clauser, T. Churchill, J. T. McConville, I. Tebbetts, and R. A. Walker. 1989. 1987-1988 Anthropometric Survey of U.S. Army Personnel: Methods and Summary Statistics. Technical Report (TR-89-044) (AD A225 094). Natick, MA: Army Natick Soldier Research Development and Engineering Center.
- Gordon, C. C., T. Churchill, C. E. Clauser, B. Bradtmiller, J. T. McConville, I. Tebbetts, and R. A. Walker. 1989. 1988 Anthropometric Survey of U.S. Army Personnel: Summary Statistics Interim Report (ANSUR I). Yellow Springs, OH: ANSUR.
- Gordon, Claire C., Cynthia L. Blackwell, Bruce Bradtmiller, Joseph L. Parham, Patricia Barrientos, Stephen P. Paquette, Brian D. Corner., et al. 2012. 2012 Anthropometric Survey of U.S. Army Personnel: Methods and Summary Statistics. Natick, MA: Army Natick Soldier Research Development and Engineering Center.
- Han, Hyunsook, Yunja Nam, and Kyungmi Choi. 2010. “Comparative Analysis of 3D Body Scan Measurements and Manual Measurements of Size Korea Adult Females.” International Journal of Industrial Ergonomics 40 (5): 530–540. doi:https://doi.org/10.1016/j.ergon.2010.06.002.
- Harte, Daniel, and Abby Paterson. 2018. “The Fastest Field Sport in the World: A Case Report on 3-Dimensional Printed Hurling Gloves to Help Prevent Injury.” Journal of Hand Therapy 31 (3): 398–410. doi:https://doi.org/10.1016/j.jht.2017.05.015.
- IBM. 2019. SPSS. Endicott: IBM.
- ISO. 1998. ISO 5725-1: Accuracy (Trueness and Precision) of Measurement Methods and Results–Part 1: General Principles and Definitions. Geneva, Switzerland: ISO.
- ISO. 2010. BS EN ISO 20685:2010: 3-D Scanning Methodologies for Internationally Compatible Anthropometric Databases. London, UK: British Standards Institute. https://www.iso.org/obp/ui/#iso:std:iso:20685:ed-2:v1:en.
- ISO. 2017. Size Designation of Clothes — Part 1: Anthropometric Definitions for Body Measurement. ISO 8559-1:2017. Geneva, Switzerland: ISO.
- Istook, Cynthia L., and Su-Jeong Hwang. 2001. “3D Body Scanning Systems with Application to the Apparel Industry.” Journal of Fashion Marketing and Management: An International Journal 5 (2): 120–132. doi:https://doi.org/10.1108/EUM0000000007283.
- Januszkiewicz, Monika, Christopher J. Parker, Steven G. Hayes, and Simeon Gill. 2019. “3D Body Scanning in the Apparel Industry: Do We Really Know Where We Are Heading?” In 3DBODY.TECH Conference & Expo 2019. Lugano, Switzerland: Hometrica Consulting.
- Jun-Ming, Lu, and Mao-Jiun J. Wang. 2010. “The Evaluation of Scan-Derived Anthropometric Measurements.” IEEE Transactions on Instrumentation and Measurement 59 (8): 2048–2054. doi:https://doi.org/10.1109/TIM.2009.2031847.
- Kim, Jung Yong, Jae Woo You, and Mi Sook Kim. 2017. “South Korean Anthropometric Data and Survey Methodology: ‘Size Korea’ Project.” Ergonomics 60 (11): 1586–1596. doi:https://doi.org/10.1080/00140139.2017.1329940.
- Kuehnapfel, Andreas, Peter Ahnert, Markus Loeffler, Anja Broda, and Markus Scholz. 2016. “Reliability of 3D Laser-Based Anthropometry and Comparison with Classical Anthropometry.” Scientific Reports 6 (1): 26672. doi:https://doi.org/10.1038/srep26672.
- Lee, Wonsup, Kihyo Jung, Jeongrim Jeong, Jangwoon Park, Jayoung Cho, Heeeun Kim, Seikwon Park, and Heecheon You. 2013. “An Anthropometric Analysis of Korean Male Helicopter Pilots for Helicopter Cockpit Design.” Ergonomics 56 (5): 879–887. doi:https://doi.org/10.1080/00140139.2013.776703.
- Liu, Kaixuan, Chun Zhu, Xuyuan Tao, Pascal Bruniaux, and Xianyi Zeng. 2019. “Parametric Design of Garment Pattern Based on Body Dimensions.” International Journal of Industrial Ergonomics 72: 212–221. (July): doi:https://doi.org/10.1016/j.ergon.2019.05.012.
- Lu, Yehu, Guowen Song, and Jun Li. 2014. “A Novel Approach for Fit Analysis of Thermal Protective Clothing Using Three-Dimensional Body Scanning.” Applied Ergonomics 45 (6): 1439–1446. doi:https://doi.org/10.1016/j.apergo.2014.04.007.
- Mckinnon, Lashawnda, and Cynthia L. Istook. 2002. “Body Scanning: The Effects of Subject Respiration and Foot Positioning on the Data Integrity of Scanned Measurements.” Journal of Fashion Marketing and Management: An International Journal 6 (2): 103–121. doi:https://doi.org/10.1108/13612020210429458.
- Medina-Inojosa, Jose, Virend K. Somers, Taiwo Ngwa, and Ling Hinshaw. 2016. “Reliability of a 3D Body Scanner for Anthropometric Measurements of Central Obesity.” Obesity: Open Access 2 (3): https://doi.org/10.16966/2380-5528.122. doi:https://doi.org/10.16966/2380-5528.122.
- Microsoft. 2019. Excel for Mac. Redmond, WA: Microsoft.
- Muammark. 2017. “People Icon Collection.” Freepik. https://www.freepik.com/free-vector/people-icon-collection_1157380.htm#page=1&query=people&position=12.
- NHS. 2001. “Ethnic Category Code 2001.” NHS Data Model and Dictionary. https://datadictionary.nhs.uk/attributes/ethnic_category_code_2001.html.
- 1996. Norton, K., and T. Olds, eds. Antropometrica. Sydney: University of New South Wales Press.
- Paquette, Steve, Claire Gordon, and Bruce Bradtmiller. 2009. “Anthropometric Survey (ANSUR) II Pilot Study: Methods and Summary Statistics.” Technical Report Natick/TR-09/014. https://apps.dtic.mil/dtic/tr/fulltext/u2/a498172.pdf.
- Park, Jinwoo, Yunja Nam, Eunkyung Lee, and Sunmi Park. 2009. “Error Detection in Three-Dimensional Surface Anthropometric Data.” International Journal of Industrial Ergonomics 39 (1): 277–282. doi:https://doi.org/10.1016/j.ergon.2008.05.009.
- Roebuck, J. A., K. H. E. Kroeme, and W. G. Thomson, eds. 1975. Engineering Anthropometry Method. 2nd ed. New York: Wiley-Interscience.
- Schneider, Harald J., Nele Friedrich, Jens Klotsche, Lars Pieper, Matthias Nauck, Ulrich John, Marcus DöRr, Stephan Felix, Hendrik Lehnert, David Pittrow, Sigmund Silber, Henry VöLzke, Günter K. Stalla, Henri Wallaschofski, and Hans-Ulrich Wittchen, 2010. “The Predictive Value of Different Measures of Obesity for Incident Cardiovascular Events and Mortality.” The Journal of Clinical Endocrinology and Metabolism 95 (4): 1777–1785. Endocrine Society: doi:https://doi.org/10.1210/jc.2009-1584.
- Schwarz-Müller, Frank, Russell Marshall, and Steve Summerskill. 2018. “Development of a Positioning Aid to Reduce Postural Variability and Errors in 3D Whole Body Scan Measurements.” Applied Ergonomics 68: 90–100. (April): doi:https://doi.org/10.1016/j.apergo.2017.11.001.
- Scott, E., S. Gill, and C. McDonald. 2019. “Novel Methods to Drive Pattern Engineering Through and For Enhanced Use of 3D Technologies.” In 10th 3DBody.Tech Conference & Expo, Hometrica Consulting, Lugano, Switzerland, 22–23 October 2019. Lugano, Switzerland: Hometrica Consulting.
- Sicotte, Maryline, Marielle Ledoux, Maria-Victoria Zunzunegui, Souleymane Ag Aboubacrine, and Vinh-Kim Nguyen. 2010. “Reliability of Anthropometric Measures in a Longitudinal Cohort of Patients Initiating ART in West Africa.” BMC Medical Research Methodology 10 (1): 102. doi:https://doi.org/10.1186/1471-2288-10-102.
- Simmons, Karla P., and Cynthia L. Istook. 2003. “Body Measurement Techniques: Comparing 3D Body‐Scanning and Anthropometric Methods for Apparel Applications.” Journal of Fashion Marketing and Management: An International Journal 7 (3): 306–332. doi:https://doi.org/10.1108/13612020310484852.
- Sizestream. 2019. SS20 Measurements and Landmark Definitions. Cary: Sizestream. https://36ea04d8-2eb1-48d8-884c-7e4182662bc9.filesusr.com/ugd/e15f31_c485b05f1f2c4057b199909e1c24d8c9.pdf.
- SizeStream. 2020. “Our Technology.” Sizestream.Com. https://www.sizestream.com/technology.
- Su, Junqiang, Ying Ke, Bingfei Gu, and Caiyuan Kuang. 2017. “A Method to Develop a Personalized Pattern of Pant from 3D Scanning Data.” In 8th International Conference and Exhibition on 3D Body Scanning and Processing Technologies, edited by Nicola D’Apuzzo. Montreal, Canada: Hometrica Consulting. doi:https://doi.org/10.15221/17.121.
- TC2. 2020. “TC2 Homepage.” TC2.Com. https://www.tc2.com/.
- Thomassey, S., and P. Bruniaux. 2013. “A Template of Ease Allowance for Garments Based on a 3D Reverse Methodology.” International Journal of Industrial Ergonomics 43 (5): 406–416. doi:https://doi.org/10.1016/j.ergon.2013.08.002.
- Ulijaszek, Stanley J., and Deborah A. Kerr. 1999. “Anthropometric Measurement Error and the Assessment of Nutritional Status.” British Journal of Nutrition 82 (3): 165–177. doi:https://doi.org/10.1017/S0007114599001348.
- Viviani, C., P.M. Arezes, S. Bragança, J. Molenbroek, I. Dianat, and H.I. Castellucci. 2018. “Accuracy, Precision and Reliability in Anthropometric Surveys for Ergonomics Purposes in Adult Working Populations: A Literature Review.” International Journal of Industrial Ergonomics 65: 1–16. doi:https://doi.org/10.1016/j.ergon.2018.01.012.
- Wang, Y. J., P. Y. Mok, Y. Li, and Y. L. Kwok. 2011. “Body Measurements of Chinese Males in Dynamic Postures and Application.” Applied Ergonomics 42 (6): 900–912. doi:https://doi.org/10.1016/j.apergo.2011.02.006.
- Wilson, John R. 2000. “Fundamentals of Ergonomics in Theory and Practice.” Applied Ergonomics 31 (6): 557–567. doi:https://doi.org/10.1016/S0003-6870(00)00034-X.
Appendix A. 3D body scanning precisions
We categorised size measurements into four rankings of importance to garment development:
Essential in sizing (BSI Citation2002)
Essential in pattern construction (Gill et al. Citation2018)
Often required in pattern construction
Possible to use in pattern construction
Using 121 measurements is prohibitive to show all locations on a body. Our numbering aligns with SizeStream’s (Citation2019) Measurements and Landmark Definitions—See Appendix B.
Table A1. Body scanning precision (cm) of all investigated scan methods to 3 standard deviation, representing 99.73% of all attainable measurements.
Allowable error represents tolerances under ANSUR (Gordon, Churchill, et al. Citation1989; Paquette, Gordon, and Bradtmiller Citation2009) for measurements that are directly used, or tolerances adopted from comparable measurements when not used in ANSUR. When measurements are the same as ANSUR, we adopt the tolerances directly. For measurements not included in ANSUR, we adopted a tolerance from another ANSUR measurement with similar characteristics (e.g. Hip and Seat).
Appendix B. Measurement descriptions
Measurement descriptions taken from SizeStream (Citation2019). Please see SizeStream (Citation2019) for further details and graphical representations.
Table B1. Definition of measurement definitions used in our study (Sizestream Citation2019).