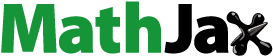
Abstract
Tram drivers operate a master controller to control the acceleration and braking of the tram. Operation should ensure passenger comfort and safety through smooth tram motion and the avoidance of jerkiness that may cause passengers to fall in the carriage. This work investigates current driver practices and strategies for tram driving in normal operations through interviews and the capacity of a new haptic master controller to support drivers in achieving smooth and safe tram journeys. A haptic feedback algorithm based on viscosity was implemented on the master controller to provide drivers with feedback on the rate at which they were accelerating and braking the tram. This aspect was tested in a virtual tram within a simulated inner city virtual reality environment. Results indicate that the haptic master controller and coupled viscosity feedback algorithm did not increase smoothness of driving during the simulated experiences. Despite this, the drivers indicated a preference for the provision of further haptic information to support driving tasks and the overall journey safety and smoothness.
Practitioner Summary: This research comprises two studies. The first investigates strategies currently used by drivers to operate a tram smoothly in order to elicit design requirements for a haptic tram master controller. The second study evaluates the impact of a novel haptic master controller on journey smoothness within a virtual environment.
1. Introduction
Trams, also known as streetcars in North America, are a steadily growing form of public transportation. They operate in more than 300 cities worldwide and in 2018 they served more than 14,000 million passengers (UITP Citation2019).
Tram operators follow tram-specific and general road signs and traffic lights, and operate within three types of city environments: (1) dedicated rail corridors separated from the road, (2) segregated tracks separated from the road but with shared intersections, and (3) mixed traffic environments shared with other motor vehicles and pedestrians. Studies have argued that segregated track and mixed traffic environments in particular, raise a range of unique safety-related issues (Candappa, Corben, and Yuen Citation2013; Currie and Reynolds Citation2014; Fontaine et al. Citation2016; Marti et al. Citation2016; Naweed and Moody Citation2015; Naweed and Rose Citation2015; Naznin, Currie, and Logan Citation2016, Citation2017; Naznin, Currie, Logan, and Sarvi Citation2016). Trams are large and heavy vehicles and collisions and incidents can result in serious personal, material, infrastructure, and operational consequences (Cameron, Harris, and Kehoe Citation2001; Hynčík et al. Citation2008; Mitra et al. Citation2010; Unger et al. Citation2002).
Driving a tram is a complex task, particularly in a shared traffic environment, and requires a high level of situational awareness, workload, and route knowledge, and achieving a smooth journey experience involves unique driving skills (Naweed and Rose Citation2015; Naweed et al. Citation2017; Naznin, Currie, and Logan Citation2017). The master controller (also referred to as traction/brake controller) is the primary mechanism for driving a tram and is this focus of this research, where novel haptic master controller will be developed and the tested. This controller is typically a throttle or lever that when moved in a forward direction provides the tram with forward acceleration, and when moved backwards results in braking (Foot and Doniol-Shaw Citation2008; Nathanael and Marmaras Citation2018). The driver’s manipulation of the master controller to manage acceleration and braking tasks influences the smoothness of the tram motion, and therefore journey comfort as well as passenger well-being and safety (Kubin, Ferkova, and Matusko Citation2015; Naweed and Moody Citation2015). Poor manipulation of the master controller results in a jerky motion and increases the likelihood of passengers losing their balance and falling in the carriage (Mitra et al. Citation2010; Naweed and Moody Citation2015; Naweed and Rose Citation2015; Victoria Transport Safety Citation2019).
1.1. Factors that influence the use of the master controller
Naweed and Moody (Citation2015) reported that effective manipulation of the master controller to achieve a smooth and safe tram journey is dependent on both ‘human’ and ‘technological’ factors. They focussed their analysis on the Australian tram system and highlighted a number of ‘technology’-related ergonomic and Human Factors (HF) issuesFootnote1 related to the design of the cab interface and devices that could directly affect tram driving performance. They also suggested that these factors indirectly influence the likelihood of injuries associated with passengers losing their balance and falling in the carriage. In Australia, tram drivers can potentially need to drive different tram classes on the same networks, thereby also face stimulus–response interface design conflicts. These conflicts may arise when tram drivers change classes (and the corresponding type of master controller) during the same shift leading to less predictable changes in tram speed in response to manipulation of the master controller. The findings suggested that the master controller is ‘ultimately associated with the safety function of ensuring a smooth passenger journey’ (154).
‘Human’ factors include the expertise held by tram drivers and acquired through direct experience and training, and involve tacit and explicit knowledge dimensions. Tacit knowledge entails ‘knowledge that is unarticulated and tied to the senses, movement skills, physical experiences, intuition, or implicit rules of thumb’ (Nonaka and von Krogh Citation2009, 635). This type of knowledge is context-based, subjective and rooted in the individual’s experience and apprenticeship (Mooradian Citation2005; Nonaka, Toyama, and Nagata Citation2000; Polanyi Citation1998). This know-how is difficult to transfer from one person to another because, as Polanyi concisely captures, ‘we know more than we can tell’ (Polanyi Citation1967, 4). Tram drivers have described their driving task as an ‘instinct’, a ‘feel of the tram’ (Naweed and Moody Citation2015, 153), or something that they do without knowing how they do it (Collins Citation2001; Gourlay Citation2006). Tacit knowledge elicitation techniques aim to extract the ‘untold’ operator competency and make it available in such a way that enables organisations to comprehend how ‘real’ work unfolds and to derive task requirements (Clot Citation1995, Citation2001; Clot and Leplat Citation2005; Daniellou Citation2005; Engestrom Citation2000; Joia and Lemos Citation2010; Nicolini Citation2009; Oddone and Re Citation1994; Oddone, Re, and Briante Citation2008; Vicente Citation1999).
Accessing tram drivers’ tacit knowledge is critical to identifying safety-related information and highlighting key HF and functional requirements that may guide the further development of an improved tram master controller.
1.2. Potential role of haptic technologies in supporting tram drivers
Haptic technologies interact with the human’s sense of touch, and haptic feedback can provide information and cues to the user through touch, collision and force. Haptic feedback can be an effective means to provide important information to an operator and beneficial in managing sensory overload, particularly in highly visual and noisy environments (Birrell, Young, and Weldon Citation2013; Luz et al. Citation2018; Rognon et al. Citation2018). Haptics have been demonstrated to assist users in undertaking motion tasks and have been shown to improve a range of different motor skills (Al-Saud et al. Citation2017; Endo and Kawasaki Citation2015; Rognon et al. Citation2018; Wildenbeest, Abbink, and Schorsch Citation2013; Zhao et al. Citation2018).
Haptic feedback has been investigated in a range of contexts, including teleoperation (Choi et al. Citation2018; Khurshid et al. Citation2017 Jan-Mar; Yin et al. Citation2016), training (Cecil, Gupta, and Pirela-Cruz Citation2018; Escobar-Castillejos et al. Citation2016; Hamza-Lup et al. Citation2019), surgery (Enayati, De Momi, and Ferrigno Citation2016; Worrell et al. Citation2015; Wottawa et al. Citation2016), rehabilitation (Atashzar et al. Citation2016; Dawson-Elli and Adamczyk Citation2020; Rajanna et al. Citation2016), cell injection (Faroque et al. Citation2018; Ghanbari et al. Citation2014), and vehicle control (Nishimura, Wada, and Sugiyama Citation2015; Petermeijer et al. Citation2015; Stebbins and Kiefer Citation2017). Haptic feedback has recently been incorporated into vehicle controls, and haptic lane keeping assistance can now be found in a variety of modern vehicles providing haptic feedback to support drivers to keep in their lane (Consumer Reports Citation2019).
Virtual Reality (VR) and other computer simulations are widely utilised for training and to better understand human and design issues. Here, VR provides the opportunity to explore the role of a novel haptic master controller in tram driving, and to investigate if a proposed haptic feedback algorithm provides benefits to tram drivers. VR technologies have dramatically improved in affordability and consumer readiness in recent years, resulting in many recent applications outside of aviation, medicine, and military as has traditionally been the case. VR simulators offer distinct capability not possible with 2D computer simulators including a higher level of immersion and presence.
This work investigates the utility and acceptability of a specifically designed haptic master controller to support tram operation with potential application to other vehicles in the future.
2. Literature review
2.1. Tram comfort and safety
The smoothness of a tram journey is related to passenger comfort and safety (Naweed and Moody Citation2015). Passenger comfort is a key component in the quality of public transportation and relates to ride comfort, ambient conditions, complementary facilities, and ergonomics (İmre and Çelebi Citation2017). Passenger comfort is also framed by international standards, such as EN 13816 (2012), that consider passenger satisfaction levels over a range of factors, and ISO/AWI 2631-1 (Citation1997, reviewed and confirmed in 2021), which defines acceptable levels of exposure to whole-body vibration experienced by passengers on board public transportation.
Safety is recognised as a quality service factor and a key performance indicator in public transport systems (Wretstrand, Holmberg, and Berntman Citation2014). Safety is also an important transport policy goal (EC Citation2018). Mitra et al. (Citation2010) reviewed the data from three Australian datasets on tram-related injuries between 2001 and 2008 and identified falls as the most common cause of injury, accounting for 45.7% of all analysed cases (n = 808). These falls appear to be caused by the tram suddenly stopping, when getting on and off the tram, or by running to catch the tram (Mitra et al. Citation2010).
Injuries and near injuries, such as slips, trips, and falls (STFs), can occur while travelling to or from a transport stop, during boarding and alighting, and during the ride due to uneven road surfaces (Albertsson and Falkmer Citation2005; Hedelin, Bunketorp, and Björnstig Citation2002; Wretstrand, Holmberg, and Berntman Citation2014) and especially affect vulnerable users and users with functional limitations (Fiedler Citation2007; Hedelin, Bunketorp, and Björnstig Citation2002; Oxley et al. Citation2006; Waterson et al. Citation2016; Wretstrand, Holmberg, and Berntman Citation2014). Approaches to improving passenger comfort may include: initiatives relating to improved infrastructure; improved design and maintenance of pedestrian paths and footways; organisational interventions such as timetable effects vs. punctuality performance to prevent drivers from rushing to stick to the shift timetable; designing enhanced on-board safety to reduce the incidence and severity of injuries; and driver training to improve journey smoothness (Fellesson and Friman Citation2008; Oxley et al. Citation2006; Waterson et al. Citation2016; Wretstrand, Holmberg, and Berntman Citation2014). Naweed and colleagues suggest that many incidents may be a consequence of drivers’ jerky driving associated with poor use of the master controller, and tram cab design (Naweed and Moody Citation2015; Naweed and Rose Citation2015).
In this research we focus on tram master controller design with the objective to support a smoother tram journey and enhanced passenger safety.
2.2. Haptic technologies in transportation
Haptic technology has been used in driving simulators to represent the physical forces present on steering wheels and pedals (Ambrož et al. Citation2012; Osgouei, Lee, and Choi Citation2013; Thiel and McConnell Citation2014). The use of haptic guidance in driver training and driver assist functionality has shown promise in communicating a wide variety of vehicle information to the driver. Research has shown that haptic guidance can help improve shared control and support drivers in performing optimal steering actions by reducing steering activity and producing smoother actions during the navigation of curves (Mulder, Abbink, and Boer Citation2008). This was achieved through communicating the lateral error between the ideal reference path and the vehicles relative position continuously and producing guiding forces on the haptic steering wheel. Haptic guidance can also assist reverse parking through a similar technique (Hirokawa et al. Citation2014) to guide correct and well-timed steering actions. Results showed that drivers who were provided with haptics guidance performed significantly better.
Providing haptic feedback to the steering wheel and throttle pedal to guide drivers has also been shown to improve bus passenger comfort (Girbes et al. Citation2016) as well as helping to manage possible pedestrian safety risks identified by collision and risk detection systems. Results show a reduction in high-risk collisions due to reduced reaction and collision times. Haptic guidance has shown further benefits, including improved traction control (Corno Citation2013), the encouragement of economical driving (Hadi and Valenzuela Citation2020), and assisting drivers in low visibility conditions (Wang et al. Citation2018).
To best represent the working environment tram drivers operate within, a VR simulator was used in this research to test a proposed haptic master controller.
3. Research aim and design
This research aimed to explore the role of a new haptic master controller in journey smoothness (and hence safety) through two studies. The studies were undertaken at Yarra Trams in Melbourne (Australia) and West Midlands Metro (WMM) in Birmingham (UK), as shown in . The first study employed a qualitative tacit knowledge elicitation method via semi-structured interviews and observations with tram drivers from both organisational contexts. In this study, the goal was to investigate acceleration and braking tasks with drivers, and the current practices and challenges associated with achieving a smooth and safe tram journey experience in normal operations. The results from this study guided the development of a novel haptic master controller and virtual reality tram environment, referred to later on as ‘TramVR’. The second study aimed to explore the effect of providing haptic feedback relative to the magnitude of change in master controller lever velocity when undertaking acceleration and braking tasks, and the effect of the haptic master controller on driving performance within the virtual reality tram environment.
4. Study 1: investigating current tram driving practices
This study was approved by the Coventry University Research Ethics Committee – Ref. N. P79580.
4.1. Methods
A total of 30 tram drivers, 14 from Yarra Trams (AU) and 16 from WMM (UK)Footnote2, participated in this study to investigate current tram driving practices. shows the age, gender, and driving experience demographic of participants. The participant sample included 25 males and 5 females. Drivers were classified in terms of driving experience as follows: 6 novices (up to 2 years), 7 experienced (from 2 to 5 years), 7 very experienced (from 6 to 10 years), and 10 experts (over 10 years)Footnote3.
Figure 2. Study 1 demographics by gender, age range, and years of tram driving experience.
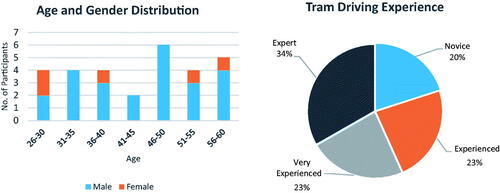
The study involved semi-structured interviews and direct observations. The semi-structured interviews used a tacit knowledge elicitation method, ‘Instructions to the Double’ (Oddone and Re Citation1994; Oddone, Re, and Briante Citation2008; Re and Oddone Citation1991), to access the tram drivers’ practices and strategies using the current in-vehicle master controllers. The ‘Instructions to the Double’ method was developed in Italy in the 1970s in an attempt to help legitimise the knowledge that manufacturing workers acquired on the job and passed on to novices. Through the pretence of the ‘double’, the interviewee is asked to provide detailed instructions to the interviewer to conduct the job as if the interviewer-double will not be un-masked: ‘Give me the instructions on how I can replace you in your everyday work/activity so that no one will notice the pretence’ (Oddone, Re, and Briante Citation2008, 44). This involves a close dialogic interaction where the interviewer guides the interviewee through different levels of ‘disclosure’, from a more superficial level to a more implicit, deeper and tacit one. During this interaction, the interviewee, representing the know-what and particularly the know-how, is guided to become aware of what they know, of their concrete behaviour in a given situation (i.e. their know-why), or ‘their model of use’ (Oddone, Re, and Briante Citation2008, 95). The process of the interview is therefore meant to support the collection of in-depth information regarding the interviewee’s practice, by prompting the interviewee to reflect on and verbally document the minute details of their own work activity (Nicolini Citation2009). As Nicolini (Citation2009) argued, this method has been proven useful to recover the operators’ and workers’ experience and enrich it through reflection. In line with the above, during the study interview sessions, the tram drivers were asked to provide ‘instructions’ on high-level topics that serve as the focus of the research (i.e. how to best perform acceleration/braking tasks, how to avoid jerkiness and achieve a smooth and safe journey, with concrete examples). All interviews were audio recorded and transcribed verbatim. The average length of each interview was 50 min.
Driving observations were undertaken during scheduled services (i.e. in normal operations with passengers on board) employing silent observations (taking ethnographic notes on an observational grid), and out of service (i.e. during training sessions) where the researcher was positioned in the driver’s cab and undertook Verbal Protocol Analysis (VPA) or ‘think-aloud’ method to elicit cognitive and physical process descriptions from the driver (Stanton Citation2005). This helped the collection of information about acceleration and braking patterns, with a focus on the position of the hand/arm while interacting with the master controller. Overall, 6 h of observations were performed, with 4 h in Melbourne and 2 h in Birmingham. presents an overview of this activity.
Table 1. Overview of driving observations.
The interview transcripts and observational notes were uploaded to an NVivo project (v. 12 Plus for Windows, ©QSR International) for codification and analysis. Thematic analysis was undertaken to make sense of the empirical material (Boyatzis Citation1998; Kuckartz Citation2019; Nowell et al. Citation2017). An inductive codebook was created to code and analyse themes, and provide an overview of the existing strategies that tram drivers use in normal operations. The codebook included three high-level themes with a number of sub-themes, as detailed in .
Table 2. Inductive codebook of Study 1.
4.2. Results
Tram classes operate differently and in Melbourne tram drivers operate five different tram classes and often switch classes in the same shift. A- and B-class trams use pedals (one for accelerating and one for braking) and the C-, D-, and E-class trams are hand-operated via a T-bar lever (C- and D-classes) or a joystick (E-class) master controller. By contrast, in Birmingham the only tram master controller is a hand-operated T-bar lever. Whilst this exploratory research benefitted from experiences related to different classes of tram, the subsequent experimental study focussed on hand-operated tram T-bar master controllers only.
4.2.1. Tram driving tasks and practices
During normal operation accelerating a tram involves ‘pushing forward’ the master controller’s lever/joystick to achieve the desired speed. Effective braking is viewed as a more complex task and when braking:
… there can be a lot more at stake. [AU_7_Expert]
To brake, the master controller lever is ‘pulled backward’; however, a ‘feathering’ motion is also required when coming into a stop. Feathering involves slowly moving the hand backward and forward to bring the tram to a complete and smooth stop without needing to bring the hand all the way back.
Another technique used by drivers is ‘coasting’. Coasting is used to remain within the speed limit or adjust the speed to road conditions. Coasting is achieved by pulling the master controller lever/joystick back to a neutral position and then employing small acceleration movements to maintain a constant speed.
[about how to coast] You gradually accelerate to the speed you want. Once you’re happy with your speed, you don’t need to go any further, and you slowly put the master controller into coasting until you hear a click or until you’re about to brake. [AU_6_Expert]
When you reach mainline speed and when you’re approaching your station, you do go into a coast mode. If you’re accelerating constantly, you tend to brake too quickly. That’s all part of it. [UK_7_Novice]
Tram drivers indicated that they employ a number of strategies to optimise acceleration, coasting and braking tasks. These strategies were acquired through training sessions, informally when exchanging practices with peers, and through years of driving experience. Drivers reported that they are assigned a number of routes that they will run during their shifts. They will learn ‘everything’ about these routes, including environmental cues and red spots (i.e. most intensive conflict areas), and how to anticipate potential threats along these routes. Drivers noted that they anticipate varying traffic lights and pedestrian crossing light patterns during day and night driving. Potential risky behaviour of road users is also anticipated, particularly on roads with shared traffic. Drivers learn the length of road segments between tram stops and traffic lights and determine the maximum speed that the tram could achieve within these segments. Drivers mentally divide these segments into three representing an ideal curve pattern where the first, second, and third segments involve accelerating up to the ideal speed, maintaining the speed by coasting, and braking to a complete stop, respectively.
You are constantly looking more at what’s going to happen in the future because it’s not what’s in the cab that will kill somebody; it’s what’s outside the cab. And so you have to be aware of the light sequences and traffic levels. I go slow when there is more traffic. For lunchtimes, we always travel slower because people are interested in eating, not in being eaten. [AU_4_Very Experienced]
Driving within the road speed limit is a key requirement for all tram drivers, and they remain constantly aware of penalties for speeding. Accordingly, drivers constantly monitor the speedometer on the tram dashboard, and regard it as a fundamental driving instrument.
4.2.2. Factors that negatively affect driving performance
Drivers reported a number of external factors that are taken into account when driving. These factors include the need to accurately assess and respond to gradients, a varying number of passengers, and changing environmental conditions, for example, rain; leaves during autumn months; operating uphill, downhill, and/or on even surfaces. The number of passengers in the carriage affects acceleration and braking because a fully loaded carriage is relatively heavy, and the driver needs to ‘push harder, and with the braking, to get on it earlier’ [UK_2_Very Experienced]. In certain weather conditions, drivers need to consider the track surface, as tracks can be slippery in the rain and are typically most slippery at the beginning and at the end of a rainy period. In autumn, falling leaves can also lead to slippery tracks. Drivers tend to reduce speed and rely on sand dropped onto the tracks to deal with slippery tracks.
Drivers in Melbourne and Birmingham agreed that trams ‘have personalities’, and they adapt to the vehicle and over time learn each vehicle’s characteristics and behaviour. In terms of acceleration and braking, drivers test the tram at the beginning of each shift and adjust to it during the course of their shift.
Some may be a little bit more responsive to begin with or may brake a bit smoother. Another one may be the complete opposite; it may be very lethargic taking off, and it can take you a bit of time to get to speed. [AU_7_Expert]
Eventually you will remember the numbers: so I know [n.xx] that brakes not as good as say [n.xx], which I find the brakes really good on. The acceleration of [n.xx] is not as fast as the acceleration of [n.xx], and so on. It’s just all about learning which trams that you are in! [UK_6_Expert]
4.2.3. Achieving a smooth journey
Drivers described achieving a smooth and comfortable journey for passengers as a process of putting in place the right ‘technique’ to manipulate the master controller for acceleration and braking tasks. When asked to define a smooth journey, the drivers used certain attributes, such as ‘not aggressive’ (22%), ‘not harsh’ (14%), and ‘not rough’ (42%) in relation to taking-off, braking, and stopping, respectively. A smooth journey involved being ‘mindful’ of the passenger experience, particularly when vulnerable or older people are on board.
A number of tacit practices are applied, and all drivers agreed that the master controller lever/joystick should be gently manoeuvred to ‘get the momentum’ when taking-off [AU_3_Experienced] before starting to push forward to accelerate. WMM drivers referred to this concept as ‘10% acceleration’, whereas in Melbourne drivers spoke about ‘hearing the click’ out of the coasting phase, a gradual movement that the drivers learn to ‘feel’ when the power is kicking in before steadily building up the tram speed.
Each tram is different. It’s not like I’ll look down and think, “That’s 10%.” And there’s nothing on the master controller to say that. It’s more just like from experience, you gradually feel it. You just feel it, the biting point when it starts. [UK_10_Expert]
Drivers learn to make use of ‘coasting’ to smoothly accelerate and decelerate (brake) the tram, and to transition from one to the other.
I don’t like to go from acceleration straight into brake back into acceleration because that causes the swaying effect. I don’t like providing that to passengers because that could make you feel nauseous. With the coast, you’ve got that lull so you’ve finished accelerating, put it back into coast, and then slide it back into brake. It’s like one continuous motion rather than forwards, backwards. [AU_5_Expert]
When it comes to braking, I like to coast into a stop and then just slowly bring it back and then a nice smooth stop. But like I say with some of them, you pull it a little bit, and they’ll stop and jerk at the last minute, but that’s a tram issue that is. [AU_12_Very Experienced]
Drivers reported that jerkiness is common in three driving situations. The strategies relate to three critical transitions on the ideal driving curve: (1) initial take off (∼10% of the full cycle), (2) coasting to initial deceleration, and (3) bringing the tram to a complete stop (∼10% of the full lever travel). depicts the aforementioned strategies and shows the ideal acceleration-deceleration curve with three critical transitions where jerkiness can occur shown in red. Drivers interviewed in the UK and Australia agreed that (1) and (3) are the most sensitive parts of the driving curve and the most prone to causing jerkiness. These stages require the ‘feel of the tram’ that is not directly addressed by current master controllers.
Figure 3. Three critical transitions on the ideal tram acceleration/braking curve (marked as red). This curve was developed to visualised the driving pattern described by drivers.
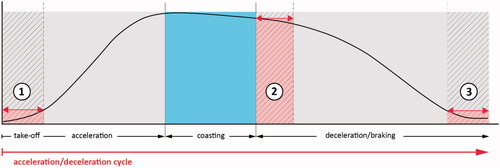
This is not an information that the lever gives to you because the way you move the lever is just the same; the force that you receive from the lever is the same. If it’s 10%, 20%, it is the same to you; you don’t understand from the position of the lever. You simply feel it. [UK_2_Very Experienced]
4.3. Elicited user and functional requirements for the novel haptic master controller
The findings from the interviews supported the specification of high-level HF/user and functional requirements to guide the design and development of the novel haptic master controller and the simulated virtual reality environment in which to explore braking and acceleration and journey smoothness. When driving, tram drivers need to capture, filter, and make decisions on a large volume of information from their surrounding environment.
Drawing on these findings, a haptic master controller should be able to:
Provide a resistive (opposing) force when tram drivers engage in of jerky driving during acceleration and braking.
Guide tram drivers in the three high-level stages of the ideal driving curve: acceleration (where the lever is pushed forward); coasting (where the lever is moved back to a neutral position); and braking (where the lever is pulled backwards until the tram comes to a full stop).
Haptic feedback should be:
Rendered as a resistive force providing information about accelerating and braking actions.
Activated at initial take off (at ∼10% of the master controller’s lever position).
Provided in the form of a ‘distinctive’ tactile feedback if taking off and/or acceleration are harshly or aggressively performed.
Activated at the transition from coasting the moment to the initial deceleration/braking.
Provided in the form of a ‘distinctive’ tactile feedback if commencement of coasting (transition from acceleration to coasting) is harshly or aggressively performed.
Perceived by the user at any point the lever is harshly or aggressively moved.
TramVR (VR tram simulator) will strive to provide user ‘immersion’ and ‘presence’ (Harris et al. Citation2020) by offering:
‘Face validity’, that is, be perceived as a realistic experience by the participant drivers.
A convincing level of fidelity, that is, recreate an accurate representation of the task and environment, particularly in relation to driving through:
a mixed-traffic environment;
a route divided into segments, each of which is defined by traffic lights/tram stops;
a route requiring varying speeds between different scenario route segments.
5. Study 2: novel haptic master controller
This study was approved by the Coventry University Research Ethics Committee – Ref. N. P90433.
5.1. Development of the haptic master controller
The haptic master controller was specifically developed as part of this work and provided bilateral single-point haptic actuation to the control lever. The haptic master controller is shown below in and employs a back-drivable DC motor, low-friction pulley and toothed-belt to provide mechanical transparency while eliminating potential slippage. The master controller has embedded electronics and Arduino microcontroller for local control of the motor and for communicating with a PC running the TramVR software. The haptic master controller along with computer and associated electronics are installed within a large custom-made aluminium housing.
Figure 4. Haptic master controller developed and tested to evaluate its role in improving passenger comfort and safety.
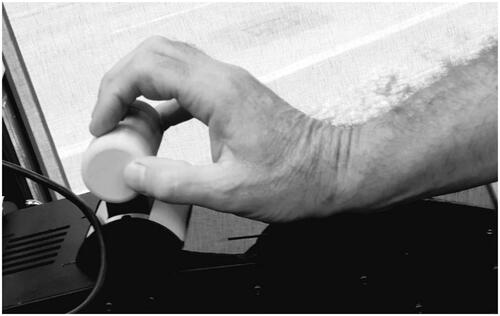
The developed haptic master controller is a bilateral interface where the driver is commanding motion control input to the tram by their movement of the lever (in a manner similar to a traditional tram master controller lever), while simultaneously receiving force feedback on the same control lever. The motion control input provided (by way of moving the lever) to control the tram has been modelled on that of a real tram. The haptic feedback provided to the driver’s hand is a function of the implemented haptic feedback algorithm employed. For example, a particular haptic feedback algorithm could focus on preventing the driver from exceeding the speed limit by pushing against the driver’s hand (via the lever) in the direction that would slow the tram down once the speed limit has been reached. An alternative haptic feedback algorithm could attempt to encourage the driver to reduce speed when approaching an intersection by pushing the lever in the direction that would slow the tram down. Here, because this was a new master controller, and no comparable haptic device for controlling trams current exists, an initial haptic feedback rendering was proposed. This sought to provide drivers with experience in receiving haptic feedback using the interface and provoke feedback to guide further development and fine-tuning of suitable haptic feedback algorithms.
This initial algorithm was based on viscosity and provided participants with force feedback (in the opposing direction) proportional to the velocity of the lever. The intention was to, by way of haptic feedback, discourage rapid acceleration and braking of the tram. This feedback is proportional to the speed that the control lever is moved where faster movement of the control lever corresponds to higher forces opposing the driver’s movement of the control lever. The rationale behind proposing this initial haptic feedback algorithm is that if participants feel an opposing force when accelerating and braking, then this would serve as a haptic cue suggesting that they should slow the rate at which they move the lever to increase journey smoothness. This was deemed to align well with the overarching objective of supporting smooth and safe tram journeys.
The haptic force displayed at the lever is determined by EquationEquation (1)(1)
(1) next:
(1)
(1)
where
= force,
= viscosity coefficient, and
= velocity of the tram controller lever.
The viscosity coefficient was set to µ = 1, meaning that there was an equal linear relationship between force feedback and velocity.
5.2. TramVR simulator and haptic master controller test scenario
The TramVR simulator was developed to enable testing of the haptic master controller when driving a tram and explore the effect on driver performance in maintaining a smooth journey. TramVR is shown in and is comprised of hardware and software components. Hardware includes a high-end desktop computer with a Nvidia GTX1080 graphics card to meet the demands of rendering the required VR environments in real-time and a high definition 26” LCD monitor. TramVR connects with the haptic master controller using a serial connection as a peripheral device to allow data to be sent corresponding to the participant’s control of the virtual tram’s speed through acceleration and braking. The software includes a simulated virtual city environment developed within the Unity game engine. An Oculus Rift VR head mounted display (HMD) connects to the computer and provides participants with a 3D view of the virtual environment.
Figure 5. Tram VR simulator including the master tram controller and the Oculus rift head-mounted display (HMD) providing the first-person view of the simulated environment.
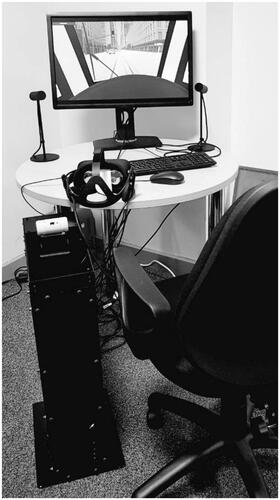
The wider tram operational context within the virtual reality scenario was informed by HF/user and functional requirements identified in Study 1 (presented in Section 4.3). The simulated scenario was developed to provide validity and fidelity criteria (Harris et al. Citation2020), and represent normal driving operations in which to investigate the effect of the haptic master controller on jerkiness and smooth driving. The scenario within the simulator was developed using a 3D modelled city environment that included an outer city road loop. This outer loop was used to model a virtual tram track with overhead wires within a mixed traffic environment representing a familiar operating environment for the tram drivers at WMM (where the experiment was conducted – see Section 5.3). The environment included cars driving in both directions, and traffic lights at the main junctions along with traffic sounds such as car engines.
Participants were positioned in the driver’s seat of the virtual tram and used the haptic master controller to drive the tram. The VR HMD has a field-of-view (FoV) of 86° in both the horizontal and vertical directions. Since the VR HMD provides a FoV significantly less than a typical human particularly in the vertical direction, the virtual tram provided information on the windscreen to overcome the issue of the limited vertical FoV while glancing down at the instrument panel. The speedometer was positioned on the front windscreen, as shown in . The speedometer also included a virtual slider providing information on the current position of the haptic master controller lever.
Figure 6. TramVR simulator test scenario representing a normal tram driving operation in a mixed traffic environment with the visual display of the tram’s speed shown in the upper-right box.
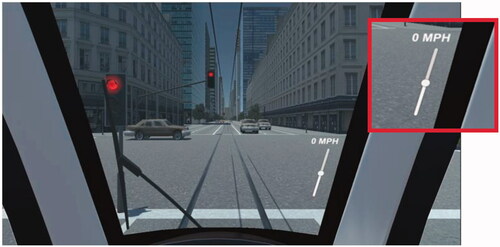
provides a bird’s-eye view of the outer city loop where roads are shown by green lines and traffic lights by pink intersecting lines. Four sections of the loop are defined (denoted by A, B, C and D) where the participant’s acceleration and braking activity were recorded. Each of these sections ended with a crossway intersection; to ensure that participants needed to perform braking traffic lights were programmed to turn yellow and then red as the tram approached. A and D are shorter track sections of equal length, and B and C are longer track sections of equal length. These sections were used to undertake two different testing scenarios.
Figure 7. Caption: Bird’s-eye view of the outer city loop where roads are shown by green lines and traffic lights by pink squares.
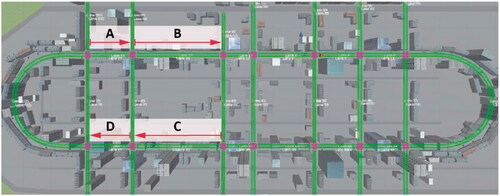
Mathematically, ideal acceleration and braking curves for the test scenario are presented in . This scenario includes a section for coasting that would typically occur when tram operators achieve their ideal speed. The figure shows that the ideal speed for each section is dependent on the length of the section. The ideal speed for short track sections A and D was ∼40 km/h, and that for long track sections B and C is ∼60 km/h. These ideal speeds were calculated by dividing the length of corresponding section of track into thirds (accelerate, coast, break), full constant acceleration was then applied to the first third of the track with ideal speed taken at the end of the acceleration section.
5.3. Methods
The experiment was conducted at the WMM (UK) premises with 16 WMM, 14 were male and 2 female. shows the age, gender, and driving experience demographics.
Figure 9. Study 2 demographics by gender, age range, and years of tram driving experience.
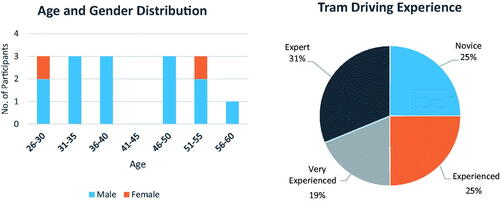
The experiment comprised three high-level stages, as listed below in .
Table 3. Breakdown of the experimental design.
In the first stage, participants were introduced to the TramVR simulator and haptic master controller and asked to familiarise themselves with the haptic master controller and the way the haptic lever responded during acceleration and braking tasks. Participants were given 5 min to drive around the city loop track without any traffic.
Testing and evaluation of the haptic master controller were undertaken in the second stage. The participants were asked to drive around the virtual city loop in simulated normal traffic and operating conditions. Completing the simulated tram city loop took approximately 3–5 min depending on the virtual traffic lights. The following scenario script was read to each participant.
You are driving along a downtown-city loop route. The tram runs on a dedicated lane, shared in mixed traffic with other road users. You can see cars driving in both directions, as well as other road users. You can also see tram stops, pedestrian crossings, and road intersections with traffic lights as part of the overall context. Please note that in this scenario, you will share the same traffic lights as other road users (i.e., you will not follow the tram-dedicated traffic lights). For four times, you will be asked to drive the tram, accelerate, and brake as you deem appropriate within the given context (including the traffic lights).
The testing scenario was structured such that participants were required to drive the simulated tram using four different controller configurations in random order, as listed below in . Using each controller configuration, participants were required to drive through the short and long sections of the track, as shown in . When approaching the start of both test sections, the virtual traffic lights were programmed to provide a red light meaning that participants needed to bring the tram to a complete stop. The performance of participants was measured across both the short (A, C) and long (B, C) track sections as shown in , resulting in a total of two full acceleration, coasting, and braking cycles. The order of the four configurations was randomly assigned for each participant.
Table 4. Tram controller configurations.
In the final stage, participants undertook a post-evaluation survey and interviews about their views and experience.
Evaluation of the haptic master controller involved collection and analysis of quantitative system data and qualitative feedback from the participants, as shown in . Quantitative data was recorded on each rendered frame for the virtual environment every 10–20 ms. This data included the position and velocity of the virtual tram, the master controller lever position, and corresponding timestamp. This was used to calculate the level of jerkiness at each timestamp and for each of the controller configurations. Jerkiness is defined as the rate of change of the tram’s acceleration over time as shown in EquationEquation (2)(2)
(2) . Performance was measured by the level of jerkiness occurring throughout the journey with high performance related to low levels of jerkiness and low performance related to high levels of jerkiness.
(2)
(2)
where
= jerkiness,
= tram velocity, a = acceleration and t = time.
Table 5. Overview of data collection.
Qualitative data was collected from each of the 16 participants via post-evaluation questionnaire and interviews. The questionnaire collected participants’ perceptions towards each of the four controller configurations. The interviews recorded the driver experience with the haptic master controller. Descriptive statistics and the Qualitative Content Analysis (Schreier Citation2012) methods were used to analyse the captured data.
5.4. Results
5.4.1. Impact of the controller configuration
The average level of jerkiness (across 15 participants) for each of the four controller configurations is shown in . Recall that jerkiness was defined as the rate of change of the tram’s acceleration over time as per EquationEquation (2)(2)
(2) in Section 5.3. The two controller configurations that provided haptic feedback (i.e. ‘Haptic’ and ‘Visual and Haptic’) both had ∼16% more jerkiness than their corresponding counterpart controller configurations that did not provide haptic feedback, that is, ‘None’ and ‘Visual’ respectively. This difference in jerkiness between the ‘None’ and ‘Visual’ controller configurations, as well as between ‘Haptic’ and ‘Visual and Haptic’ due to the provision of haptic feedback, resulted in an increase in jerkiness of 16% in both cases.
Figure 10. Different controller configurations and the corresponding amount of jerkiness.
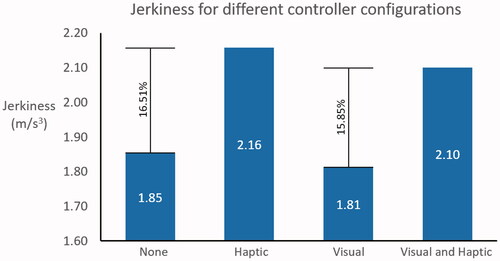
While the addition of haptic feedback to a controller configuration actually increased the level of jerkiness, it did so consistently by 16%. It is important to note that the presented haptic master controller was developed specifically as part of this work, and in the absence of a suitable precedent, a new viscosity-based haptic feedback algorithm was proposed to provide a basis for evaluation by tram drivers. The feedback algorithm and corresponding haptic feedback did not result in tram drivers controlling the virtual tram with reduced jerkiness as intended but did have a consistent impact on the level of jerkiness when compared with the controller configuration that was otherwise the same.
shows the same data, that is, the average level of jerkiness across all participants, for each of the different controller configurations. Unlike , which is arranged to highlight the difference in jerkiness by the addition of haptic feedback, is arranged to highlight the impact of the addition of the visual display of tram velocity and master controller lever position to the controller configuration used by participants. As was the case with the addition of haptic feedback, the two controller configurations including visual feedback both had similar impact on the levels of observed jerkiness. The observed impact was a slight (∼2%) reduction in jerkiness when compared to the corresponding controller configuration counterparts not providing visual feedback.
Figure 11. Different controller configurations and the corresponding amount of jerkiness reordered to show the impact of adding visual information.
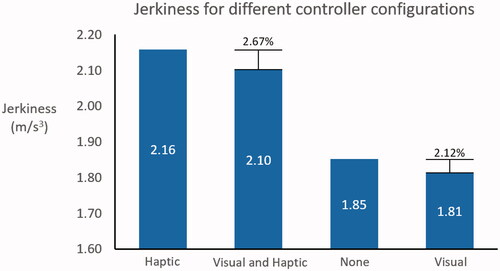
The reduction in jerkiness due to the addition of visual feedback was 2.67% for the ‘Visual and Haptic’ controller configuration when compared with ‘Haptic’, and 2.12% for ‘None’ when compared with ‘Visual’. The similar reduction in jerkiness due to the addition of this visual display by a particular configuration indicates that this information impacted the driver in achieving a marginally smoother journey.
5.4.2. Participant feedback
Participants were asked to rate the extent to which the four different controller configurations provided relevant information to guide a smooth and safe journey. The results are presented in . Participants found the two controller configurations that provided a visual indication of the tram’s speed and lever position, that is (‘Visual’ and ‘Visual and Haptic’), the most relevant. Participants most strongly agreed that the controller configuration that provided haptic feedback as well as the visual display of the speed and lever position provided the most relevant information (+2.75). The controller configuration that provided neither haptic feedback nor visual display of speed (labelled as ‘None’) was seen as the least relevant. Although the controller configurations that included haptic feedback actually increased jerkiness, albeit consistently, participants none the less found both configurations that included haptics more relevant to achieving a smooth and safe journey.
Figure 12. Post-survey results for the different controller configurations.
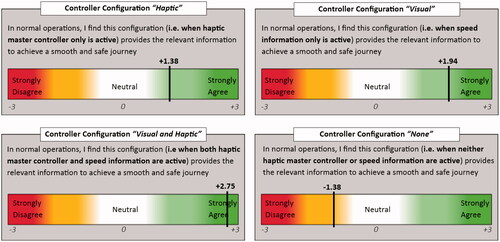
shows the controller configurations that drivers preferred the most to manage (i) acceleration and braking tasks and (ii) for comfort and safety. The configuration providing haptic feedback and a visual display of speed and lever position, and the configuration with visual display of speed and lever position alone, were the preferred methods for both tasks by the majority of drivers. In fact, no driver specified the other two configurations (‘Visual’ and ‘None’) as their preferred method for either managing acceleration/braking or for achieving journey comfort and safety. For acceleration/braking tasks, the ‘Haptic and Visual’ configuration was ranked the preferred method for 10 participants (67%). The configuration with visual information only (and no haptic feedback) was the only other configuration preferred by participants and chosen by five participants (33%). In relation to the controller configuration that participants thought most useful for managing comfort and safety, fourteen participants (93%) preferred the configuration with haptic feedback and a visual display of the speed and lever position. Only one participant (7%) preferred the configuration with visual information only (and no haptic feedback). No participants selected the controller configuration with haptic information only or with neither haptic feedback nor visual display of speed and lever position.
Figure 13. Self-reported post-survey desired controller configuration for overall comfort (upper) and acceleration and braking (lower).
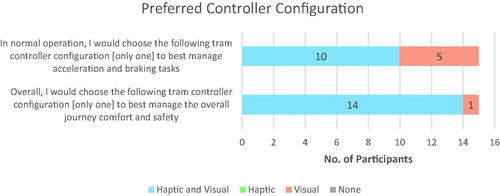
In the post-evaluation interviews, drivers commented that haptics was perceived as a ‘silent assistant’ that provides information about their driving style and alerts them, should they be harsh in the acceleration/braking movements on the lever. Additionally, they suggested that haptics could enhance driving tasks and help them to feel how the tram is likely to react when driving, particularly in braking situations.
As a driver you get that ‘sense of feel’, so you know how hard for example you’re braking. With the haptics, you feel you have got a bit more control over your braking. [UK_14_Very Experienced]
The haptics gave me the feel of the tram. It was good, and when I was getting in the coasting I liked it. Especially when I was strong braking, and I could feel it more…. you see I wouldn’t have wanted to feel that if I was driving. [UK_16_Very Experienced]
Participants suggested that while they appreciated haptic guidance, it should only be assistive and never prevent a driver from having full control.
Haptic should be telling you if are driving too aggressively, not change the drive. It should never interrupt on what the driver’s wanting to do, but it should guide and give information. [UK_1_Novice]
The drivers’ second preference was for visual information with no haptic feedback. This is in line with driver’s consideration of the speedometer as a fundamental driving instrument (Section 4.2.1). In the post-evaluation interviews, the participants confirmed that the visual information helps them to monitor their current speed and judge the braking distance as expressed by the participant below:
The speedo is a hundred per cent critical to us. Any driver that says that isn’t needed shouldn’t be in the job. [UK_14_Very Experienced]
In relation to the haptic feedback provided by the novel controller, participants appreciated the viscosity-based haptic feedback, although they suggested that the magnitude of the forces provided as feedback could be increased. It is worth noting that as mentioned earlier, the maximum force able to be displayed by the device was intentionally designed to be sufficiently low so that the operator is able to override the haptic feedback and ultimately stay in control of the tram. Four participants (UK_1_Novice, UK_4_Novice, UK_5_Expert, and UK_10_Expert) proposed using a different haptic rendering algorithm, for example a vibration that could provide alert feedback in case of aggressive driving. Additionally, the participants shared their views when haptics should be activated (i.e. as a supporting feedback throughout the acceleration/braking tasks, or when the lever is moved aggressively). Participants commented that both options could be valid on the basis of individual preference.
Finally, all drivers agreed during the interviews that having a haptic master controller during their refresher training could be beneficial to improve their driving skills. One participant suggested the following idea:
It’s obviously a benefit for training, but again why leave it at that? Why not introduce it onto the vehicle for normal operation and then you’re constantly being coached by the tram as to what is the best. [UK_1_Novice]
6. Discussion
Injuries associated with loss of balance by passengers and subsequent falls in the tram carriage are a safety concern for all tram operators. As discussed throughout this paper, driving a tram is performed via the master controller as the primary driving mechanism. The driver’s manipulation of the master controller commands of acceleration and braking is directly related to the smoothness of the tram motion, which has an impact on journey comfort, and passenger well-being and safety (Naweed and Moody Citation2015).
This research was undertaken in two distinct organisational contexts with both organisations operating articulated tram networks (i.e. with dedicated rail corridors, segregated corridors but with shared intersections, and mixed traffic environments), but in different parts of the world. The first study aimed to shed light on the factors involved in achieving a jerky-free tram ride and was based on the perspectives of tram drivers, the strategies they use, and the challenges they face. Research that investigates the work of tram drivers’ and the workarounds that they employ to carry out the daily activities is limited (Joo Choi et al. Citation2007; Wisner Citation1995). A tacit knowledge elicitation method was employed and pointed to three stages of driving tasks: namely, acceleration, coasting, and braking. It also identified the related practices drivers employ to avoid jerkiness when moving the tram master controller’s lever to transition between these stages. These findings highlight the human’s role (i.e. the tram drivers’ skills and knowledge) in working around the constraints offered by the current master controller (e.g. performance inconsistencies across vehicles’ master controllers) (Naweed and Moody Citation2015) and striving to drive the tram smoothly and safely. These findings also helped define requirements for the design and implementation of a new haptic master controller with haptic feedback algorithms able to support less jerky, or smoother, driving.
The second study investigated whether a new haptically-enhanced master controller could assist tram drivers in achieving a smooth journey by providing force feedback to elicit drivers’ ‘awareness’ and discourage tram drivers from rapid acceleration/braking actions during normal conditions. The literature has shown the importance of avoiding additional visual and audio messages/warnings that can adversely impact driver mental workload (Candappa, Corben, and Yuen Citation2013; Guesset, De Labonnefon, and Blancheton Citation2016; Naweed and Moody Citation2015; Naweed et al. Citation2017; Naznin, Currie, and Logan Citation2018; Restel and Wolniewicz Citation2017; Rowe Citation2013). To the best of the authors’ knowledge, there has been no research conducted into the role of haptic or tactile feedback and how it can be used to support tram drivers in maintaining a smooth and safe journey. Feedback from the drivers suggests that the virtual driving task and environment provided by the virtual reality tram simulator (TramVR) felt a realistic representation of the real-world scenarios.
The haptic master controller was tested using TramVR. The haptic feedback algorithm employed was based on a viscosity function. In our experiment, the viscosity coefficient was set to µ = 1, meaning that there was an equal linear relationship between force feedback and lever velocity and this was used to relate force feedback with the velocity (rate of change of position) of the lever. The intention here was to discourage, by way haptic forces in the lever held by the operator’s hand, fast movement of the master controller lever to achieve a smoother journey. This coefficient could, of course, be varied and further tested in future work, or even an entirely different haptic feedback algorithm implemented if desired.
In real-world terms, when the driver is using the haptic master controller they would feel an increasing opposing force the faster they move the lever. The quantitative results collected via the TramVR simulator, presented in Section 5.4, demonstrate that when haptic feedback was incorporated, the level of jerkiness did not decrease as was intended. Being the first haptic feedback algorithm implemented on this new haptic master controller, the opportunity to benefit from expert feedback had not been present. Despite increased jerkiness, it is interesting to note that the two controller configurations that did employ haptic feedback, experienced a very similar (16%) impact on the level of jerkiness when used by drivers. This similar impact on the level of jerkiness (or conversely smoothness) experienced for the two haptic controller configurations suggests that the haptic feedback did impact the jerkiness achieved by drivers, however not in reducing jerkiness as intended.
Evaluation of the haptic master controller with the drivers suggested other possible haptic feedback algorithms that could be applied to the haptic master controller, such as incorporating ‘alerts’ at the three critical transitions on the ideal driving curve. These transitions align with the three stages of tram driving tasks, that is, (1) initial take off at ∼10% of the full cycle; (2) coasting to initial deceleration; and (3) bringing the tram to a complete stop at ∼10% of the full lever travel. This is valuable insight in itself and would not be possible without the initial viscosity-based haptic feedback algorithm providing the basis for drivers to experience haptic feedback using the haptic master controller. Further insight gained from the qualitative results highlighted that drivers saw haptics as valuable for braking tasks. The implemented haptic feedback was intended to support maintaining a smooth journey, as opposed to braking. Drivers may have found the algorithm useful for braking tasks, and if so could help explain why drivers were generally positive about the introduced haptic feedback even if it did result in reduced, albeit consistent, level of journey smoothness. Unlike a real-world tram where drivers would feel the jerkiness of tram through their bodies, the TramVR simulator did not provide full body haptic feedback. In the absence of such feedback, aside from any subtle visual cues of such jerkiness that may be present from the tram’s motion in the virtual environment, participants are manipulating the master tram controller in the way that they believe would correspond to smooth tram motion. The haptic feedback on the controller is such that it responds to the commands made to the tram (via the master controller), rather than the corresponding tram motions itself.
Although the results of the experimental study demonstrate that haptic feedback did not reduce jerkiness, drivers’ feedback from the post-evaluation questionnaire and interviews suggest positive favourable views/perceptions of the role of haptics to support driving tasks and journey smoothness. Drivers commented that haptic feedback helped them derive enhanced information about how the driving tasks were performed, and how the tram is reacting in braking situations. The post-evaluation results show a strong preference towards visual controller configuration alone, or in conjunction with haptics for managing journey comfort and safety (93%) and acceleration/braking tasks (67%). This preference for the controller configurations incorporating visual display of speed and lever position, can be explained at the operational level by the critical role played by the speedometer in normal tram driving operations. Drivers refer to and constantly monitor the speedometer to check whether they are driving within the required speed limits and to assess safety braking distances (Naweed Citation2014; Naweed and Moody Citation2015; Naweed and Rose Citation2015)
Drivers highlighted that while they appreciated support for driving tasks through haptics, they should always retain final control of the master controller and lever, being the only ones accountable for their decisions and actions. As discussed earlier, the designed force implemented in the haptic master controller could be overpowered by the drivers, leaving them in ultimate control of the motion commanded to the tram. Participants regarded haptic feedback in normal driving operations as an ‘aid’ or form of ‘guidance’ and not a necessary requirement. Drivers agreed that haptics has the potential to provide guidance in driving smoothly in the two complementary operational situations. In the first situation, haptic feedback can act as an ‘aid’ to smoothly guide the master controller’s lever to manage acceleration, coasting, and braking tasks, and help prevent any harsh motions that could lead to falls in the carriage. Critically, the information should be presented gradually where the more you accelerate and brake, the greater the haptic feedback. Alternatively, in the other situation, haptics could be triggered by harsh movements of the lever and, potentially vibration-based haptic feedback, alerting the drivers about the undesired driving action. These are both valuable insights and despite the implemented viscosity haptic feedback algorithm undesirably resulting in increased jerkiness, this does align with the intention of it to prevent the driver from moving the lever in a manner that would correspond to jerky tram motion. This warrants further investigation into haptic rendering algorithms that can contribute to decreased jerkiness.
Recent research suggests that the tram master controller can be a contributing factor to musculoskeletal injury (Naweed et al. Citation2020). While the master controller introduced in this paper is kinematically similar to those currently employed in trams, that is, a lever pushed forward and backwards by the driver, it is an active device that can be programmed with different haptic feedback functions as well as one with very low inherent mechanical friction. These characteristics of the introduced haptic interface provide the opportunity to consider the ergonomics impacting trams drivers in ways not possible with current tram controllers. Future work can also consider whether the lessons learnt here can be applied to the control of other types of vehicles, particularly those including similar lever type interfaces including aircraft and trains.
7. Conclusions
It is important that a tram journey is relatively smooth in order to maintain a safe and comfortable journey for both passengers and tram drivers; qualitative research has enabled a greater understanding of how tram drives try to achieve this. Acceleration and braking in a variety of complex driving scenarios and conditions mean that achieving a smooth experience is not always straightforward. The research findings have informed the development of a VR tram simulator (TramVR). Virtual environments provide a low risk way of training and testing out new innovations. In this case, based on the requirements drawn from drivers, we have developed and tested a haptically-enhanced master controller lever to support and guide drivers during normal operations.
This work has applicability to improved driver training using VR tram simulators and the enhancement of the master controller lever in real operations. The controller can be programmed to implement different haptic feedback, and an initial haptic feedback algorithm was proposed to provide the basis for feedback from tram drivers. Whilst the implemented algorithm did not reduce jerkiness, it was consistent in the extent it increased jerkiness, providing sufficient evidence to warrant further exploration into a more effective haptic feedback algorithms. Positive participant feedback in support of haptic feedback provision suggests further research is warranted to tram control and haptic simulator design.
Abbreviations | ||
HF | = | human factors |
VR | = | virtual reality |
STFs | = | slips, trips and falls |
WMM | = | west midlands metro |
AU | = | Australia |
UK | = | United Kingdom |
VPA | = | verbal protocol analysis |
TramVR | = | VR tram simulator |
HMD | = | head mounted display |
FoV | = | field of view |
Acknowledgments
The authors wish to sincerely thank Yarra Trams in Melbourne (Australia) and West Midlands Metro in Birmingham (UK) for supporting this research and facilitating the recruitment of their tram drivers. We also would like to thank all participating drivers who gave their time for the interviews and shared their knowledge and experience with us.
Disclosure statement
No potential conflict of interest was reported by the author(s).
Additional information
Funding
Notes
1 Human Factors is the scientific discipline concerned with the understanding and improvement of interactions among humans and other elements of a system. The system is a socio-technical system as it comprises social, as well as technological components, taking into account physical, cognitive and organizational factors (definition provided by the International Ergonomics Association (IEA): https://iea.cc/what-is-ergonomics/).
2 The 16 WMM drivers participated in both Study 1 and Study 2.
3 The source evidence of the participants is provided as follows: Country (AU for Australia vs UK for the United Kingdom); ID number (as it was assigned during the interviewing process); Experience (this will refer to the years of declared years of driving experience as recorded through the profile form, and specifically: ‘Novice’ (up to 2 years), ‘Experienced’ (from 2 to 5 years), ‘Very experienced’ (from 6 to 10 years), and ‘Expert’ (over 10 years)). An Australian driver, with the identification number of 1, with three years of tram driving experience would be labelled: AU_1_Experienced.
References
- Albertsson, P., and T. Falkmer. 2005. “Is There a Pattern in European Bus and Coach Incidents? A Literature Analysis with Special Focus on Injury Causation and Injury Mechanisms.” Accident; Analysis and Prevention 37 (2): 225–233. doi:https://doi.org/10.1016/j.aap.2004.03.006.
- Al-Saud, L. M., F. Mushtaq, M. J. Allsop, P. C. Culmer, I. Mirghani, E. Yates, A. Keeling, M. A. Mon-Williams, and M. Manogue. 2017. “Feedback and Motor Skill Acquisition Using a Haptic Dental Simulator.” European Journal of Dental Education 21 (4): 240–247. doi:https://doi.org/10.1111/eje.12214.
- Ambrož, M., I. Prebil, R. Kamnik, and M. Munih. 2012. “System for Interactive Scientific Driving Simulation with Haptic Information.” Advances in Engineering Software 45 (1): 239–251. doi:https://doi.org/10.1016/j.advengsoft.2011.10.001.
- Atashzar, S. F., M. Shahbazi, O. Samotus, M. Tavakoli, M. S. Jog, and R. V. Patel. 2016. “Characterization of Upper-Limb Pathological Tremors: Application to Design of an Augmented Haptic Rehabilitation System.” IEEE Journal of Selected Topics in Signal Processing 10 (5): 888–903. doi:https://doi.org/10.1109/JSTSP.2016.2530632.
- Birrell, S. A., M. S. Young, and A. M. Weldon. 2013. “Vibrotactile Pedals: Provision of Haptic Feedback to Support Economical Driving.” Ergonomics 56 (2): 282–292.
- Boyatzis, R. E. 1998. Transforming Qualitative Information: Thematic Analysis and Code Development. Thousand Oaks, CA: SAGE Publications.
- Cameron, I. C., N. J. Harris, and N. J. S. Kehoe. 2001. “Tram-Related Injuries in Sheffield.” Injury 32 (4): 275–277. doi:https://doi.org/10.1016/S0020-1383(00)00196-0.
- Candappa, N., B. Corben, and J. Yuen. 2013. Addressing the Conflict Potential between Motor Vehicles and Trams at Cut-through Locations. Melbourne, Australia: Accident Research Centre, Monash University.
- Cecil, J., A. Gupta, and M. Pirela-Cruz. 2018. “An Advanced Simulator for Orthopedic Surgical Training.” International Journal of Computer Assisted Radiology and Surgery 13 (2): 305–319.
- Choi, I., E. Ofek, H. Benko, M. Sinclair, and C. Holz. 2018. “CLAW: A Multifunctional Handheld Haptic Controller for Grasping, Touching, and Triggering in Virtual Reality.” Paper presented at the 2018 CHI Conference on Human Factors in Computing Systems, Montreal, Canada, April 21–26.
- Clot, Y. 1995. “L’échange Avec un «Sosie», Pour Penser L’expérience.” Société Française 3 (53): 51–55.
- Clot, Y. 2001. Clinique de L’activité et Pouvoir D’agir. Ottawa, Canada: Education Permanente.
- Clot, Y., and J. Leplat. 2005. “Clinical Method in Ergonomics and Work Psychology.” Le Travail Humain 68 (4): 289–316. doi:https://doi.org/10.3917/th.684.0289.
- Collins, H. M. 2001. “Tacit Knowledge, Trust and the Q of Sapphire.” Social Studies of Science 31 (1): 71–85. doi:https://doi.org/10.1177/030631201031001004.
- Consumer Reports. 2019. “Detailed list of cars with features that help drivers avoid or mitigate collisions.” CR. https://www.consumerreports.org/car-safety/cars-with-advancedsafety-systems/
- Corno, M. 2013. “Design, Analysis, and Validation of a Haptic-Based Driver Support System for Traction Control.” IEEE Transactions on Intelligent Transportation Systems 14 (4): 1849–1859. doi:https://doi.org/10.1109/TITS.2013.2268316.
- Currie, G., and J. Reynolds. 2014. “Vehicle and Pedestrian Safety at Light Rail Stops in Mixed Traffic.” Transportation Research Record: Journal of the Transportation Research Board 2146 (1): 26–34. doi:https://doi.org/10.3141/2146-04.
- Daniellou, F. 2005. “The French-Speaking Ergonomists’ Approach to Work Activity: Cross-Influences of Field Intervention and Conceptual Models.” Theoretical Issues in Ergonomics Science 6 (5): 409–427.
- Dawson-Elli, A. R., and P. G. Adamczyk. 2020. “Design and Validation of a Lower-Limb Haptic Rehabilitation Robot.” IEEE Transactions on Neural Systems and Rehabilitation Engineering 28 (7): 1584–1594.
- EC. 2018. “Road Safety Management.” European Commission. https://ec.europa.eu/transport/road_safety/sites/roadsafety/files/pdf/ersosynthesis2018-roadsafetymanagement.pdf
- EN 13816. 2002. “CEN/TC 320 Transportation - Logistics and Services - Public Passenger Transport - Service Quality Definition, Targeting and Measurement.” European Commission. https://ec.europa.eu/eip/ageing/standards/city/transportation/en-138162002_en
- Enayati, N., E. De Momi, and G. Ferrigno. 2016. “Haptics in Robot-Assisted Surgery: Challenges and Benefits.” IEEE Reviews in Biomedical Engineering 9: 49–65.
- Endo, T., and H. Kawasaki. 2015. “A Fine Motor Skill Training System Using Multi-Fingered Haptic Interface Robot.” International Journal of Human-Computer Studies 84: 41–50. doi:https://doi.org/10.1016/j.ijhcs.2015.08.006.
- Engestrom, Y. 2000. “Activity Theory as a Framework for Analyzing and Redesigning Work.” Ergonomics 43 (7): 960–974. doi:https://doi.org/10.1080/001401300409143.
- Escobar-Castillejos, D., J. Noguez, L. Neri, A. Magana, and B. Benes. 2016. “A Review of Simulators with Haptic Devices for Medical Training.” Journal of Medical Systems 40 (4): 104.
- Faroque, S., M. Mortimer, M. Pangestu, M. Seyedmahmoudian, and B. Horan. 2018. “Evaluation of a New Virtual Reality Micro-Robotic Cell Injection Training System.” Computers & Electrical Engineering 67: 656–671. doi:https://doi.org/10.1016/j.compeleceng.2017.04.030.
- Fellesson, M., and M. Friman. 2008. “Perceived Satisfaction with Public Transport Service in Nine European Cities.” Journal of the Transportation Research Forum 47 (3): 93–103.
- Fiedler, M. 2007. “Older People and Public Transport. Challenges and Chances of an Aging Society.” EMTA. https://www.emta.com/IMG/pdf/Final_Report_Older_People_protec.pdf
- Fontaine, L., M. Novales, D. Bertrand, and M. Teixeira. 2016. “Safety and Operation of Tramways in Interaction with Public Space.” Transportation Research Procedia 14: 1114–1123. doi:https://doi.org/10.1016/j.trpro.2016.05.182.
- Foot, R., and G. Doniol-Shaw. 2008. “Questions Raised on the Design of the “Dead-Man” Device Installed on Trams.” Cognition, Technology & Work 10 (1): 41–51. doi:https://doi.org/10.1007/s10111-007-0076-x.
- Ghanbari, A., B. Horan, S. Nahavandi, X. Chen, and W. Wang. 2014. “Haptic Microrobotic Cell Injection System.” IEEE Systems Journal 8 (2): 371–383. doi:https://doi.org/10.1109/JSYST.2012.2206440.
- Girbes, V., L. Armesto, J. Dols, and J. Tornero. 2016. “Haptic Feedback to Assist Bus Drivers for Pedestrian Safety at Low Speed.” IEEE Transactions on Haptics 9 (3): 345–357.
- Gourlay, S. 2006. “Towards Conceptual Clarity for ‘Tacit Knowledge’: A Review of Empirical Studies.” Knowledge Management Research & Practice 4 (1): 60–69. doi:https://doi.org/10.1057/palgrave.kmrp.8500082.
- Guesset, A., V. De Labonnefon, and M. Blancheton. 2016. “Ergonomics and Visibility in Tramway Driving Cab.” Transportation Research Procedia 14: 585–594. doi:https://doi.org/10.1016/j.trpro.2016.05.297.
- Hadi, R., and A. Valenzuela. 2020. “Good Vibrations: Consumer Responses to Technology-Mediated Haptic Feedback.” Journal of Consumer Research 47 (2): 256–271. doi:https://doi.org/10.1093/jcr/ucz039.
- Hamza-Lup, F. G., C. M. Bogdan, D. M. Popovici, and O. D. Costea. 2019. “A Survey of Visuo-Haptic Simulation in Surgical Training.” In Proceedings of the Third International Conference on Mobile, Hybrid, and On-line Learning. Gosier, Guadeloupe, France. pp. 57–62.
- Harris, D. J., J. M. Bird, P. A. Smart, M. R. Wilson, and S. J. Vine. 2020. “A Framework for the Testing and Validation of Simulated Environments in Experimentation and Training.” Frontiers in Psychology 11: 605. doi:https://doi.org/10.3389/fpsyg.2020.00605.
- Hedelin, A., O. Bunketorp, and U. Björnstig. 2002. “Public Transport in Metropolitan Areas — a Danger for Unprotected Road Users.” Safety Science 40 (5): 467–477. doi:https://doi.org/10.1016/S0925-7535(01)00014-5.
- Hirokawa, M., N. Uesugi, S. Furugori, T. Kitagawa, and K. Suzuki. 2014. “Effect of Haptic Assistance on Learning Vehicle Reverse Parking Skills.” IEEE Transactions on Haptics 7 (3): 334–344. doi:https://doi.org/10.1109/TOH.2014.2309135.
- Hynčík, L., H. Kocková, L. Íhalová, and R. Cimrman. 2008. “Optimization of Tram Face with Respect to Passive Safety.” Applied and Computational Mechanics 2 (1): 53–62.
- IEA. n.d. “What Is Ergonomics?” International Ergonomics Association. https://iea.cc/what-is-ergonomics/
- İmre, Ş., and D. Çelebi. 2017. “Measuring Comfort in Public Transport: A Case Study for İstanbul.” Transportation Research Procedia 25: 2441–2449. doi:https://doi.org/10.1016/j.trpro.2017.05.261.
- ISO/AWI 2631-1. 1997. “Mechanical Vibration and Shock — Evaluation of Human Exposure to Whole-Body Vibration — Part 1: General Requirements.” ISO. https://www.iso.org/standard/76369.html
- Joia, L. A., and B. Lemos. 2010. “Relevant Factors for Tacit Knowledge Transfer within Organisations.” Journal of Knowledge Management 14 (3): 410–427. doi:https://doi.org/10.1108/13673271011050139.
- Joo Choi, H., L. S. Mark, M. J. Dainoff, and C. Park. 2007. “Normative, Descriptive and Formative Approaches to Describing Normal Work Area.” Theoretical Issues in Ergonomics Science 8 (1): 37–62. doi:https://doi.org/10.1080/14639220500175322.
- Khurshid, R. P., N. T. Fitter, E. A. Fedalei, and K. J. Kuchenbecker. 2017. “Effects of Grip-Force, Contact, and Acceleration Feedback on a Teleoperated Pick-and-Place Task.” IEEE Transactions on Haptics 10 (1): 40–53.
- Kubin, J., Z. Ferkova, and J. Matusko. 2015. “Influnce of Driving Style of a Tram Driver on the Tram’s Energy Consumption.” Paper presented at the 2015 International Conference on Electrical Drives and Power Electronics (EDPE), Bratislavia, Slovakia, September 21–23.
- Kuckartz, U. 2019. “Qualitative Text Analysis: A Systematic Approach.” In Compendium for Early Career Researchers in Mathematics Education, edited by G. Kaiser and N. Presmeg, 181–197. Cham, Switzerland: Springer International Publishing.
- Luz, R., J. Corujeira, J. L. Silva, and R. Ventura. 2018. “Traction Awareness Through Haptic Feedback for the Teleoperation of UGVs.” Paper presented at the 2018 27th IEEE International Symposium on Robot and Human Interactive Communication (RO-MAN), Tai’an, China, August 27–31.
- Marti, C. M., J. Kupferschmid, M. Schwertner, A. Nash, and U. Weidmann. 2016. “Tram Safety in Mixed Traffic Best Practices from Switzerland.” Transportation Research Record 2540 (1): 125–137. doi:https://doi.org/10.3141/2540-14.
- Mitra, B., J. Al Jubair, P. A. Cameron, and B. J. Gabbe. 2010. “Tram-Related Trauma in Melbourne, Victoria.” Emergency Medicine Australasia 22 (4): 337–342. doi:https://doi.org/10.1111/j.1742-6723.2010.01309.x.
- Mooradian, N. 2005. “Tacit Knowledge: Philosophic Roots and Role in KM.” Journal of Knowledge Management 9 (6): 104–113. doi:https://doi.org/10.1108/13673270510629990.
- Mulder, M., D. A. Abbink, and E. R. Boer. 2008. “The Effect of Haptic Guidance on Curve Negotiation Behavior of Young, Experienced Drivers.” Paper presented at the 2008 IEEE International Conference on Systems, Man and Cybernetics.
- Nathanael, D., and N. Marmaras. 2018. “From the Seat to the System: Re-Designing a Tram Drivers’ Workstation Combining Technical and Contextual Aspects.” Applied Ergonomics 73: 214–226. doi:https://doi.org/10.1016/j.apergo.2018.07.013.
- Naweed, A. 2014. “Investigations into the Skills of Modern and Traditional Train Driving.” Applied Ergonomics 45 (3): 462–470. doi:https://doi.org/10.1016/j.apergo.2013.06.006.
- Naweed, A., and H. Moody. 2015. “A Streetcar Undesired: Investigating Ergonomics and Human Factors Issues in the Driver–Cab Interface of Australian Trams.” Urban Rail Transit 1 (3): 149–158. doi:https://doi.org/10.1007/s40864-015-0021-x.
- Naweed, A., and J. Rose. 2015. “‘It’s a Frightful Scenario’: A Study of Tram Collisions on a Mixed-Traffic Environment in an Australian Metropolitan Setting.” Procedia Manufacturing 3: 2706–2713. doi:https://doi.org/10.1016/j.promfg.2015.07.666.
- Naweed, A., L. Bowditch, J. Trigg, and C. J. S. Unsworth. 2020. “Out on a Limb: Applying the Person-Environment-Occupation-Performance Model to Examine Injury-Linked Factors among Light Rail Drivers.” Safety Science 127: 104696. doi:https://doi.org/10.1016/j.ssci.2020.104696.
- Naweed, A., J. Rose, S. Singh, and D. Kook. 2017. “Risk Factors for Driver Distraction and Inattention in Tram Drivers.” In Advances in Human Aspects of Transportation. Proceedings of the AHFE 2016 International Conference on Human Factors in Transportation, edited by N. A. Stanton, R. Landry, G. D. Bucchianico, and A. Vallicelli. New York, NY: Springer.
- Naznin, F., G. Currie, and D. Logan. 2016. “Exploring the Impacts of Factors Contributing to Tram-Involved Serious Injury Crashes on Melbourne Tram Routes.” Accident; Analysis and Prevention 94: 238–244. doi:https://doi.org/10.1016/j.aap.2016.06.008.
- Naznin, F., G. Currie, and D. Logan. 2017. “Key Challenges in Tram/Streetcar Driving from the Tram Driver’s Perspective – a Qualitative Study.” Transportation Research Part F 49: 39–48. doi:https://doi.org/10.1016/j.trf.2017.06.003.
- Naznin, F., G. Currie, and D. Logan. 2018. “Exploring Road Design Factors Influencing Tram Road Safety - Melbourne Tram Driver Focus Groups.” Accident; Analysis and Prevention 110: 52–61. doi:https://doi.org/10.1016/j.aap.2017.10.017.
- Naznin, F., G. Currie, D. Logan, and M. Sarvi. 2016. “Safety Impacts of Platform Tram Stops on Pedestrians in Mixed Traffic Operation: A Comparison Group Before-After Crash Study.” Accident; Analysis and Prevention 86: 1–8. doi:https://doi.org/10.1016/j.aap.2015.10.007.
- Nicolini, D. 2009. “Articulating Practice Through the Interview to the Double.” Management Learning 40 (2): 195–212. doi:https://doi.org/10.1177/1350507608101230.
- Nishimura, R., T. Wada, and S. Sugiyama. 2015. “Haptic Shared Control in Steering Operation Based on Cooperative Status Between a Driver and a Driver Assistance System.” Journal of Human-Robot Interaction 4 (3): 19–37.
- Nonaka, I., and G. von Krogh. 2009. “Perspective-Tacit Knowledge and Knowledge Conversion: Controversy and Advancement in Organizational Knowledge Creation Theory.” Organization Science 20 (3): 635–652. doi:https://doi.org/10.1287/orsc.1080.0412.
- Nonaka, I., R. Toyama, and A. Nagata. 2000. “A Firm as a Knowledge-Creating Entity: A New Perspective on the Theory of the Firm.” Industrial and Corporate Change 9 (1): 1–20. doi:https://doi.org/10.1093/icc/9.1.1.
- Nowell, L. S., J. M. Norris, D. E. White, and N. J. Moules. 2017. “Thematic Analysis: Striving to Meet the Trustworthiness Criteria.” International Journal of Qualitative Methods 16 (1): 160940691773384. doi:https://doi.org/10.1177/1609406917733847.
- Oddone, I., and A. Re. 1994. “Come Recuperare L’expertise Professionale.” In Portolano di Psicologia, edited by P. Tranchina, 277–280. Pistoia, Italy: Centro di Documentazione Pistoia.
- Oddone, I., A. Re, and G. Briante. 2008. Esperienza Operaia, Coscienza di Classe e Psicologia Del Lavoro. Torino, Italy: OTTO Editore.
- Osgouei, R. H., H. Lee, and S. Choi. 2013. “Haptic-Enabled Driving Training System.” Paper presented at the 2013 IEEE RO-MAN, Gyeongju, South Korea, August 26–29.
- Oxley, J., J. Charlton, B. Corben, and B. Fildes. 2006. “Crash and Injury Risk of Older Pedestrians and Identification of Measures to Meet Their Mobility and Safety Needs.” Paper presented at the 7th International Conference on Walking and Liveable Communities, Melbourne, Australia, October 23–25.
- Petermeijer, S. M., D. A. Abbink, M. Mulder, and J. C. F. D. Winter. 2015. “The Effect of Haptic Support Systems on Driver Performance: A Literature Survey.” IEEE Transactions on Haptics 8 (4): 467–479. doi:https://doi.org/10.1109/TOH.2015.2437871.
- Polanyi, M. 1967. The Tacit Dimension. New York, NY: Doubleday.
- Polanyi, M. 1998. Personal Knowledge: Towards a Post-Critical Philosophy. New York, NY: Harper & Row.
- Rajanna, V., P. Vo, J. Barth, M. Mjelde, T. Grey, C. Oduola, and T. Hammond. 2016. “KinoHaptics: An Automated, Wearable, Haptic Assisted, Physio-Therapeutic System for Post-Surgery Rehabilitation and Self-Care.” Journal of Medical Systems 40 (3): 60.
- Re, A., and I. Oddone. 1991. “Competenza Professionale Ristretta e Competenza Professionale Allargata.” In Soggetti Lavoro Professioni, edited by G. P. Quaglino, 260–274. Torino, Italy: Bollati Boringhieri.
- Restel, F., and L. Wolniewicz. 2017. “Tramway Reliability and Safety Influencing Factors.” Procedia Engineering 187: 477–482. doi:https://doi.org/10.1016/j.proeng.2017.04.403.
- Rognon, C., A. R. Wu, S. Mintchev, A. Ijspeert, and D. Floreano. 2018. “Haptic Guidance with a Soft Exoskeleton Reduces Error in Drone Teleoperation.” In Haptics: Science, Technology, and Applications. EuroHaptics 2018, Lecture Notes in Computer Science, edited by D. Prattichizzo, H. Shinoda, H. Tan, E. Ruffaldi, and A. Frisoli, vol. 10894. Cham, Switzerland: Springer.
- Rowe, I. 2013. “Developing a Tram Driver Route Learning Training Simulator for Manchester’s Metrolink Trams.” In Rail Human Factors: Supporting Reliability, Safety and Cost Reduction, edited by N. Dadashi, A. Scott, J. R. Wilson, and A. Mills. 262–269. Boca Raton, FL: CRC Press.
- Schreier, M. 2012. Qualitative Content Analysis in Practice. London, UK: SAGE Publications.
- Stanton, N. A. 2005. Human Factors Methods: A Practical Guide for Engineering and Design. Aldershot, UK: Ashgate Publishing Ltd.
- Stebbins, M. A., and R. J. Kiefer. 2017. Vehicle seat back haptic alert systems and methods. US Patent 9545879B2, Published January 17.
- Thiel, S., and C. McConnell. 2014. Haptic pedal system. Patent US8836493B2.
- UITP. 2019. “The Global Tram and Light Rail Landscape.” UITP Advancing Public Transport. https://www.uitp.org/
- Unger, R., C. Eder, J. M. Mayr, and J. Wernig. 2002. “Child Pedestrian Injuries at Tram and Bus Stops.” Injury 33 (6): 485–488. doi:https://doi.org/10.1016/S0020-1383(02)00051-7.
- Vicente, K. J. 1999. Cognitive Work Analysis: Toward Safe, Productive, and Healthy Computer-Based Work. Mahwah, NJ: Lawrence Erlbaum Associates Publishers.
- Victoria Transport Safety. 2019. 2018 Annual Incident Statistics VICTORIAN TRAM OPERATORS. Melbourne, Australia: Transport Safety Victoria.
- Wang, Z., R. Zheng, T. Kaizuka, and K. Nakano. 2018. “Influence of Haptic Guidance on Driving Behaviour under Degraded Visual Feedback Conditions.” IET Intelligent Transport Systems 12 (6): 454–462. doi:https://doi.org/10.1049/iet-its.2017.0112.
- Waterson, P. E., V. L. Kendrick, B. Ryan, T. Jun, and R. A. Haslam. 2016. “Probing Deeper into the Risks of Slips, Trips and Falls for an Ageing Rail Passenger Population: Applying a Systems Approach.” IET Intelligent Transport Systems 10 (1): 25–31. doi:https://doi.org/10.1049/iet-its.2015.0047.
- Wildenbeest, J. G. W., D. A. Abbink, and J. F. Schorsch. 2013. “Haptic Transparency Increases the Generalizability of Motor Learning During Telemanipulation.” Paper presented at the World Haptics Conference (WHC), Daejeon, Korea, April 14–17.
- Wisner, A. 1995. “Understanding Problem Building: Ergonomic Work Analysis.” Ergonomics 38 (3): 595–605. doi:https://doi.org/10.1080/00140139508925133.
- Worrell, B. C., G. S. Strobl, G. A. Trees, J. T. Batross, N. G. Molitor, K. T. Shoger, D. K. Norvell, M. J. Andreyko, G. W. Johnson, and S. C. Snyder. 2015. Haptic feedback devices for surgical robot. US patent 9737326B2, filed October 23.
- Wottawa, C. R., B. Genovese, B. N. Nowroozi, S. D. Hart, J. W. Bisley, W. S. Grundfest, and E. P. Dutson. 2016. “Evaluating Tactile Feedback in Robotic Surgery for Potential Clinical Application Using an Animal Model.” Surgical Endoscopy 30 (8): 3198–3209. doi:https://doi.org/10.1007/s00464-015-4602-2.
- Wretstrand, A., B. Holmberg, and M. Berntman. 2014. “Safety as a Key Performance Indicator: Creating a Safety Culture for Enhanced Passenger Safety, Comfort, and Accessibility.” Research in Transportation Economics 48: 109–115. doi:https://doi.org/10.1016/j.retrec.2014.09.008.
- Yin, X., S. Guo, H. Hirata, and H. Ishihara. 2016. “Design and Experimental Evaluation of a Teleoperated Haptic Robot–Assisted Catheter Operating System.” Journal of Intelligent Material Systems and Structures 27 (1): 3–16. doi:https://doi.org/10.1177/1045389X14556167.
- Zhao, H., Z. Zheng, A. Swanson, A. Weitlauf, Z. Warren, and N. Sarkar. 2018. “Design of a Haptic Virtual System for Improving Fine Motor Skills in Children with Autism.” In Advances in Human Factors and Systems Interaction. AHFE 2017. Advances in Intelligent Systems and Computing, edited by I. Nunes, vol. 592. Cham, Switzerland: Springer.