Abstract
This state of the science review brings together the disparate literature of effective strategies for enhancing and accelerating team performance. The review evaluates and synthesises models and proposes recommended avenues for future research. The two major models of the Input-Mediator-Output-Input (IMOI) framework and the Big Five dimensions of teamwork were reviewed and both will need significant development for application to future teams comprising non-human agents. Research suggests that a multi-method approach is appropriate for team measurements, such as the integration of methods from self-report, observer ratings, event-based measurement and automated recordings. Simulations are recommended as the most effective team-based training interventions. The impact of new technology and autonomous agents is discussed with respect to the changing nature of teamwork. In particular, whether existing teamwork models and measures are suitable to support the design, operation and evaluation of human-nonhuman teams of the future.
Practitioner summary: This review recommends a multi-method approach to the measurement and evaluation of teamwork. Team models will need to be adapted to describe interaction with non-human agents, which is what the future is most likely to hold. The most effective team training interventions use simulation-based approaches.
Introduction
The ability of a group of agents (which could be both human and non-human) to come together to perform beyond the sum of their parts is fundamental to the safety, security, and comfort of society (Salas, Reyes, and McDaniel Citation2018). Organisations depend on teams to successfully undertake increasingly intricate tasks. This is particularly true of complex, dynamic and fast-paced technological environments such as aviation (Svensson and Andersson Citation2006), emergency services (Salas, Rosen, and King Citation2007), healthcare (Flin and Maran Citation2004), intelligence analysis (Stanton, Walker, and Sorensen Citation2012), military (Goodwin, Blacksmith, and Coats Citation2018), process control (Stanton and Ashleigh Citation2000) and sport (Carron, Bray, and Eys Citation2002). In such contexts, the consequences of poorly designed and malfunctioning teams range from the inefficiencies and economic costs associated with inadequate work processes to the catastrophic personal, social and economic costs associated with large-scale loss of life incidents. For example, recent catastrophic incidents such as the Air France 447 tragedy (Salmon, Walker, and Stanton Citation2016), the Black Hawk friendly fire incident (Snook Citation2000), the Stockwell shooting incident (Jenkins et al. Citation2010), and the Alton Towers rollercoaster incident (HSE Citation2015a, Citation2015b) all involved poorly functioning teams as a contributory factor. On the contrary, heroic recoveries such as the Hudson River landing (Sullenberger and Zaslow Citation2009), the Apollo 13 mission (Trotter, Salmon, and Lenne Citation2014), the Qantas Flight 32 incident (De Crespigny Citation2012), and the Thai soccer team cave rescue (Aronson Citation2019) all achieved successful outcomes largely due to well-functioning teams. The importance of effectively functioning teams cannot be understated.
Unfortunately, effective team performances are not merely the result of bringing together highly skilled individuals. Team members must display proficient taskwork and engage in various teamwork processes such as coordination and communication to ensure that all members can synchronise their actions and work towards a common goal (Dalenberg, Vogelaar, and Beersma Citation2009). Significantly, effective teamwork ‘creates knowledge, minimises errors, promotes innovation, saves lives, enhances productivity, increases job satisfaction, and ensures success’ (Salas, Reyes, and Woods Citation2017, 22). As Annett and Stanton (Citation2000) point out: ‘A team is a group, but not all groups are teams. The key distinction lies in whether or not the members share a common goal, which they pursue collaboratively.’ (1045–1046). Team members also have different roles and responsibilities requiring interdependence and interaction with one another (Stanton Citation2014; Stanton et al. Citation2017).
A fundamental difference between teams and groups is that team members collectively share in the benefits or costs of successes and failures, whereas group members may not. Consequently, it has become increasingly important to understand the means by which underperforming teams can improve their teamwork skills, well-performing teams can sustain levels of achievement, and mature teams can grow and maximise their capabilities (Shuffler et al. Citation2018).
Fortunately, the science of teams and teamwork has advanced significantly over the last century (cf. Mathieu et al. Citation2017), with Salas, Cooke, and Rosen (Citation2008) even describing contemporary times as the ‘Golden Age’ of team research. There is no lack of theory, models, research, or consultants that specialise in the field of teams and the development of team effectiveness (Cannon-Bowers and Bowers Citation2011). There are numerous reviews and meta-analyses which assimilate knowledge regarding the precise makeup of effective teams (e.g. Bell Citation2007; Bell et al. Citation2018), the factors that shape team effectiveness (Marks, Mathieu, and Zaccaro Citation2001; Ilgen et al. Citation2005; Salas, Sims, and Burke Citation2005; Rousseau, Aubé, and Savoie Citation2006), methods of developing such factors (e.g. Hughes et al. Citation2016; McEwan et al. Citation2017; Lacerenza et al. Citation2018), and the means by which teamwork and team effectiveness can be measured (e.g. Havyer et al. Citation2013; Valentine, Nembhard, and Edmondson Citation2015; Waterson et al. Citation2015; Marlow, Bisbey, et al. Citation2018).
Despite the vast body of literature, there are two pressing issues that provide the impetus for this state-of-science review. First, whilst the existing body of work provides an invaluable resource from which to inform the most effective and efficient strategies to enhance and accelerate team performance, the literature is somewhat disparate (Shuffler et al. Citation2018). It is challenging therefore, to draw general conclusions about the variety of interventions and methods of assessment available.
Second, the environments, organisations, and systems in which teams work are changing dramatically, as are the nature of teams themselves. Work is becoming increasingly technology centric in nature and current and forthcoming advances such as autonomous agents, artificial intelligence (AI), artificial general intelligence (AGI), robotics, machine learning and big data systems are changing how teams are organised and how they operate (Walker et al. Citation2017). The topic of AI can be quite abstract but AI already plays an explicit role in our daily life. AI based advances in image and speech recognition underpin technology such as google lens (Lucia, Vetter, and Moroz Citation2021) and voice-based assistants such as Amazon’s Alexa (Pitardi and Marriott Citation2021). The introduction of such technology has changed the way that humans live their lives providing non-human based assistance with a range of tasks from the compiling of shopping lists to identification of unfamiliar animal species. AI is increasingly playing an important role in less explicit aspects of our daily life from the design of novel drug based therapies we consume (Hessler and Baringhaus Citation2018) and disease detection we rely upon to stay healthy (Rauschecker et al. Citation2020); to the management of airport operations enabling safe, efficient travel (Donadio et al. Citation2018) and waste management which greatly impacts the environment in which we live (Abdallah et al. Citation2020). These innovations are posing challenging questions for our existing human-centric models and measures. For example, the extent to which state-of-the-art models and measures are suitable for designing and evaluating human and non-human agent teams requires consideration. This is for various reasons, not least because most of the models and measures available were developed largely based on teams comprising only human operators (Paris, Salas, and Cannon-Bowers Citation2000).
This State of Science review is a response to these issues and aims to (1) synthesise and evaluate the varied models and methods available for describing and assessing measuring teamwork, (2) question the extent to which existing models and measures are suitable to support design, operation and evaluation of human-nonhuman teams of the future; and (3) discuss the numerous approaches to team development in order to provide a state of the science review. We commence with a series of definitions of some of the key terms that relate to teamwork and team development before progressing into a discussion of some of the teamwork models that have shaped much of the current thinking. We then review the various methods of measuring teamwork, examining the extent to which these approaches have been utilised and validated within the literature. Next, the team development interventions with the strongest evidence of effectiveness are evaluated, before concluding with some anticipated challenges and recommendations for the future.
Definitions
The ability of individuals to work together to attain superior team performance is critical to the success of organisations and is commonly referred to as teamwork. A large number of constructs associated with teams have been investigated over the years. Many of these constructs are used interchangeably throughout the literature; therefore, it is important at the outset to adopt a set of key definitions for this review.
Teams have been defined in a number of different ways across the years, yet there are certain pervasive elements to most definitions; the composition of two or more individuals (Dyer Citation1984; Annett and Stanton Citation2000), some level of interdependence (Salas et al. Citation1992), and the presence of shared goals (Annett and Stanton Citation2000; Salas, Sims, and Burke Citation2005). The following definition compiled from a number of sources is adopted for the purpose of this State of Science review: Groups of two or more individuals who have specific roles and interact adaptively, interdependently, and dynamically towards a common and valued goal (Dyer Citation1984; Salas et al. Citation1992; Annett and Stanton Citation2000; Salas, Sims, and Burke Citation2005).
Teamwork has been referred to simply as ‘what teams do’ (Carron, Martin, and Loughead Citation2012). However, there is an important distinction to be made between taskwork as the functions that individuals must perform to accomplish the team’s task, and teamwork which describes the ‘interdependent components of performance required to effectively coordinate the performance of multiple individuals’ (Salas, Cooke, and Rosen Citation2008, 541). Teamwork is the most extensively studied construct related to teams in the literature (Paris, Salas, and Cannon-Bowers Citation2000; McEwan and Beauchamp Citation2014). Studies have focussed on numerous mechanisms that transform team inputs to valued team outcomes (Marks, Mathieu, and Zaccaro Citation2001). These include interactions that take place among team members, for example, communication (e.g. Neville, Salmon, and Read Citation2018) and coordination (e.g. Grote, Kolbe, and Waller Citation2018); as well as the dynamic properties of a team, such as situation awareness (e.g. Stanton et al. Citation2017) mental models, trust (e.g. Costa, Roe, and Taillieu Citation2001) and additional emergent states (Coultas et al. Citation2014). For the purpose of this review, the following definition of teamwork is adopted: The knowledge, skills, and attitudes critical for team members to interdependently interact with one another effectively in such a way that leads to positive team-based outcomes (Salas, Rosen, Burke, et al. Citation2009).
The terms effectiveness and performance, as consequences of teamwork, are often used interchangeably (Kendall and Salas Citation2004). However, team performance – the most salient and valued indicator of team effectiveness (Kozlowski et al. Citation2015) – fails to account for how the team interacts to achieve its outcome, and can be influenced by external factors outside of the team’s control (Salas, Sims, and Burke Citation2005). It has therefore been suggested that the outcomes of team performance are better represented by multiple, complementary indicators. Cohen and Bailey (Citation1997), for example, conceptualised team effectiveness as comprising of performance, attitudes, and behaviours.
Finally, the term team development interventions (TDI) has been used in the literature to encompass all activities ‘aimed at improving team competencies, processes, and overall effectiveness’ (Lacerenza et al. Citation2018, 518). Numerous types of TDIs are employed by organisations, including changes to team composition, team building, team training, and leadership training, amongst others. These will be discussed in more detail later in the review.
Models of teamwork
The field of team research dates back nearly a century to the seminal Hawthorne studies of the 1920s and 1930s (Mathieu et al. Citation2017). Since then a considerable body of literature has accumulated, with a recent special edition of the American Psychologist on ‘The Science of Teamwork’ (Vol. 73, No. 4, May–June 2018) and numerous published reviews testament to the advances in knowledge acquired over this period (e.g. Paris, Salas, and Cannon-Bowers Citation2000; Ilgen et al. Citation2005; Kozlowski and Bell Citation2003; Mathieu et al. Citation2008; Kozlowski Citation2018; Schmutz, Meier, and Manser Citation2019). Much of this research has concerned the specification and subsequent conceptualisation of the many variables thought to enable teams to function proficiently, resulting in a plethora of models of team effectiveness. Indeed, the number of models, constructs and conflicting findings has led researchers to describe the field as ‘messy’ (Kozlowski Citation2018). Whilst it is impossible to review all of the numerous frameworks available (Salas, Sims, and Burke Citation2005) reviewed 138 and Rousseau, Aubé, and Savoie (Citation2006) reviewed 29), it is our view that a small number have been critical to the scientific development of the field. In particular, a selection of models have been highly cited in Human Factors and Ergonomics (HFE) applications.
Despite little consensus on how best to conceptualise the many variables that influence team performance, most frameworks adopt a largely Input-Process-Outcome heuristic (IPO; McGrath Citation1964). In this paradigm organisational, team, and individual resources (inputs) are converted by team member interactions (processes) into the by-products of team activity (outcomes). More recent conceptualisations have sought to address some of the shortcomings of such a simplistic model, notably that the multilevel and dynamic nature of teams is overlooked. For example, a paper by Ilgen et al. (Citation2005) recommended that the range of variables influencing the transmission of inputs into outcomes are not merely processes, but include a range of cognitive, motivational and attitudinal states that develop over time (emergent states). Marks, Mathieu, and Zaccaro (Citation2001) proposed the first episodic approach to team effectiveness, presenting a model whereby teams execute different processes at different times during task execution. For example, during Transition phases team members employ processes such as mission analysis and goal specification in order to plan for future work. Within Action phases, processes such as monitoring and coordination enable the successful accomplishment of team tasks. Finally, Interpersonal processes such as conflict management and motivation building are salient across every episodic phase. This taxonomy of team processes, supported by meta-analytic evidence (LePine et al. Citation2008), has influenced much of the current thinking around teamwork, and has been cited nearly 3000 times (Scopus, June, 2020).
The development of team processes over time is also accounted for by the Input-Mediator-Output-Input (IMOI) framework (Ilgen et al. Citation2005). This model, illustrated in , represents the cyclical nature of team functioning, capturing both feedback loops and the multiple levels of influence that team inputs have on mediators and outcomes (Mathieu et al. Citation2008).
Figure 1. Input-Mediator-Outcome-Input Framework (From: Ilgen et al. Citation2005; Mathieu et al. Citation2008).
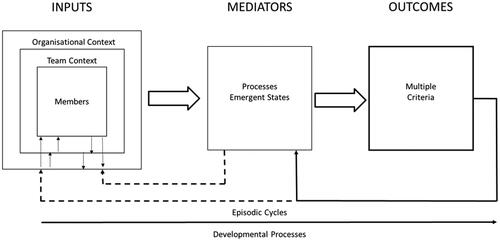
Furthermore, the adoption of the term mediators acknowledges that there are a number of variables that convert inputs into outcomes, which might include processes (e.g. communication, coordination), or emergent states (e.g. trust, cohesion). These two landmark theoretical papers have both been cited nearly 2000 times (Scopus, June 2020), and encourage a multilevel and dynamic approach to studying teams. By way of example, Stanton and Ashleigh (Citation2000) conducted a practical study using the IMOI framework in fieldwork to evaluate four teams in the energy distribution industry. Data on each aspect of the IMOI framework were collected over several months. The main finding from this work showed that team structure appeared to have the largest effect on the mediators but no effect on outcome measures.
One model that does not subscribe to the traditional IPO heuristic is that of Salas, Sims, and Burke (Citation2005). In an attempt to assimilate the ‘unmanageable’ literature and identify the variables most commonly discussed as having the greatest effect on team performance, Salas, Sims, and Burke (Citation2005) proposed the Big Five of teamwork based on a synthesis of over 130 models (see ). According to Salas, Sims, and Burke (Citation2005) effective teams engage in five key teamwork processes that are facilitated by three additional coordinating mechanisms. The Big Five include: leadership, the ability to direct, motivate, and coordinate the activities of members; mutual performance monitoring, the application of strategies to track team-mate performance; backup behaviour, the ability to balance workload among members in conditions of high workload/pressure; adaptability, the ability to recognise deviations from expected action and adjust collective actions; and team orientation, belief in the importance of the team’s goals over individual goals.
Figure 2. Graphical representation of high-level relationship between the Big Five teamwork dimensions and Coordinating Mechanisms (From: Salas, Sims, and Burke Citation2005).
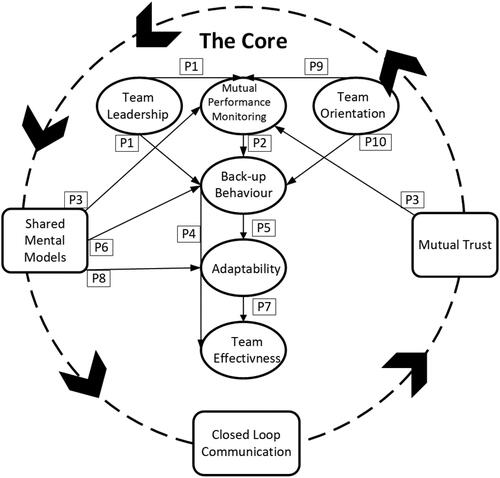
The three coordinating mechanisms include shared mental models, the shared knowledge about key task and team related elements within a team’s environment; mutual trust, the shared belief that team members will perform their roles and protect the interests of their team-mates; and closed loop communication, the process by which information is exchanged between two or more team members (Salas, Sims, and Burke Citation2005; King et al. Citation2008). In terms of the IMOI framework, these teamwork variables could be categorised as two emergent states and one process, and are believed to vary in importance across the stages of a team’s development and context of operation (Ilgen et al. Citation2005).
Salas, Sims, and Burke (Citation2005) make a series of propositions about the nature of relationships between the variables and their relative impact upon performance, although research in support of these propositions has been limited. Nevertheless, the model has been cited over 14000 times (Scopus,June, 2020) and has been applied in various domains including healthcare (e.g. Mayer et al. Citation2011; Lisbon et al. Citation2016; Weld et al. Citation2016), aviation (Malakis, Kontogiannis, and Kirwan Citation2010), defence (Rafferty and Stanton Citation2012), and elite sport (Neville, Salmon, and Read Citation2018; Salmon, Clacy, and Dallat Citation2017). Furthermore, the practical, applied recommendations made by Salas, Sims, and Burke (Citation2005) make the model far more functional in a real-world context than most other models.
Whilst the aforementioned models (all captured in ) address some of the limitations associated with the traditional IPO heuristic, it is increasingly apparent that these models do not accurately represent modern teams; teams that are constantly and dynamically ever-changing in terms of their processes, tasks, and context (Delice, Rousseau, and Feitosa Citation2019). Extant frameworks provide a structure from which to think about and manage team dynamics, but they do not account for the complexity of modern teams (Benishek and Lazzara Citation2019). Consequently, Delice and colleagues argue that more work regarding the theoretical nature of teams is still needed.
Table 1. Comparison of teamwork models.
Regardless of the framework adopted, team processes and emergent states have received the most frequent research attention to date. These mediating variables enable the integration of individuals’ effort towards the accomplishment of shared goals, and represent the very essence of teamwork (Kozlowski Citation2018). Many of these processes are cognitive in nature. The importance of cognitive processing at the team level, or team cognition, became very clear after the USS Vincennes incident in 1988 in which the US ship mistakenly shot down Iran Flight 655 killing 290 passengers (Rogers and Rogers Citation1992). The TADMUS (Tactical Decision Making Under Stress) Naval program was initiated as a result. Much of that program was devoted to understanding how teams understand a situation and make decisions, especially under duress (Cannon-Bowers and Salas Citation1997). From that program, shared mental models were proposed as a team construct associated with team effectiveness with increasing model similarity being key to team effectiveness (Cannon-Bowers and Salas Citation1990; Cannon-Bowers, Salas, and Converse Citation1993). Other related cognitive constructs were also explored such as team situation awareness (Dekker Citation2000). Measures were developed for these constructs that were associated with team effectiveness (Cooke et al. Citation2000; Gorman, Cooke, and Winner Citation2006). Team cognition research also attracted Human Factors and cognitive engineering researchers to the study of teamwork (Salas and Fiore Citation2004). Other theories or models have been developed to account for team cognition including distributed cognition (Hutchins Citation1995), distributed situation awareness (Stanton et al. Citation2006, Citation2017), macrocognition (Fiore et al. Citation2010; Roberts and Stanton Citation2018) and interactive team cognition (Cooke Citation2015; Cooke et al. Citation2013).
The empirical literature supporting the role of team cognition and processes in general and emergent states on valued team and individual outcomes is abundant and mature, with numerous supporting meta-analyses (Mathieu et al. Citation2017). Therefore, it is unsurprising that the various measurement instruments available assess either individual and group processes or emergent states, or a combination thereof. Furthermore, TDIs are generally employed with the principal aim of improving specific team processes or emergent states, with the hope of enhancing overall team performance. However, given the number of frameworks of team effectiveness available and lack of consensus on the precise factors that teamwork consists of, the measurement and development of teamwork is correspondingly diverse.
Teamwork measurement
The measurement of teamwork and team performance underlies the capacity for organisations to manage, improve, and sustain high levels of team effectiveness. If teamwork is not well defined and measured, it cannot be improved in any systematic way (Salas, Rosen, Held, et al. Citation2009). The wide range of processes assumed to facilitate teamwork compounded by the lack of single, unifying theory of the exact dimensions of teamwork, has made measurement challenging as associated measures are correspondingly diverse (Valentine, Nembhard, and Edmondson Citation2015). Instruments such as the Team Climate Inventory (TCI; Anderson and West Citation1996) measure a collection of team related competencies (e.g. participative safety, task orientation), whereas others, such as the widely utilised Psychological Safety questionnaire (Edmondson Citation1999), measures specific processes or emergent states. A number of reviews have been conducted to collate the various measurement tools available (see for an overview of a selection of these articles). Collectively these reviews identified well over 220 measures of teamwork. Although a diverse and vast set of measures are discussed, these reviews demonstrate an over reliance on self-report measures, inconsistent reporting of reliability and validity testing, and an almost exclusive focus on the measurement of teamwork in healthcare settings.
Table 2. Overview of reviews conducted on teamwork and associated measures.
The utilisation of self-report inventories has proliferated in recent decades, but a number of additional methods exist to assess teamwork and team performance. These include behavioural observations (e.g. Mishra, Catchpole, and McCulloch Citation2009 - some rate behaviours of individual team members whereas others rate behaviours of the team as a whole), event-based measurement which generates behavioural checklists that are linked to scenario events (e.g. Fowlkes et al. Citation1994), and automatic performance measurement such as audio recording (e.g. Andersson, Rankin, and Diptee Citation2017). The relative strengths and weaknesses of each measure type, as well as some examples of state-of-the-art measures in each category, are presented in and discussed in the following sections.
Table 3. Comparison of teamwork measures.
Self-report measures
Questionnaires and rating scales are a common method of assessing both individual and team-level teamwork processes; particularly valuable for those qualities that are more affective (e.g. trust) in nature and not readily observable (Rosen et al. Citation2012). Instruments such as the Team Climate Inventory (TCI; Anderson and West Citation1996), which appeared most frequently in the reviews documented in , assess multiple teamwork competencies. Other tools measure single constructs associated with teamwork, such as the Psychological Safety scale (Edmondson Citation1999). This scale appears to be one of the most widely utilised measures of team functioning, having been cited in excess of 7000 times (Google Scholar, 2020). A significant challenge for the assessment of teams using self-report instruments is the aggregation or translation of individual responses into team-level characteristics. Even with a detailed theoretical understanding and careful analysis of the respective constructs, the various methods of aggregation are likely to yield very different results that fail to capture the unique variance in individual responses. In addition, self-report measures often yield inflated scores, as individuals are likely to rate themselves more favourably than an observer might (Marlow, Bisbey, et al. Citation2018). Self-report measures are often administered pre- or post-team tasks, failing to capture the dynamic nature of team performance that occurs whilst a team is performing their taskwork (Rosen et al. Citation2012).
Observational measures
Observational measures, on the other hand, can be collected in real-time or with video recordings of activities, thus having the potential to capture the dynamic nature of team functioning. Indeed, accurately capturing observable behaviours is considered to be fundamental to assessing the attributes of a team (Salas, Reyes, and Woods Citation2017). Behaviourally anchored rating scales (BARS) represent an observational technique typically employed; consisting of brief descriptions of behaviours that serve as anchors for excellent, acceptable, and poor performance. These behaviours can be assessed at an individual level and aggregated to a team score or at a team level. If the former method is used, the same problems of aggregation discussed above apply. The Non-technical Skills system for rating pilots’ behaviour in a crew setting (NOTECHS; Flin et al. Citation2003) is a good example of a widely utilised BARS that has been applied to the assessment of team functioning in healthcare settings (Mishra, Catchpole, and McCulloch Citation2009). Whilst the concrete descriptions provided contribute to more accurate ratings, there is also a risk of observers becoming blinkered and focussing only on the behaviours on the list (Murphy and Constans Citation1987). Behavioural observation scales (BOS), alternatively, avoid such problems through the application of Likert-type scales to rate the frequency of certain team processes (e.g. Brown and Latham Citation2002; Thomas, Sexton, and Helmreich Citation2004; Burtscher et al. Citation2010). This type of measure is summative, relying on the memory of the observer to evaluate performance across time, potentially making it susceptible to recency and primacy effects (Rosen et al. Citation2012). Observational measures are not free from bias. To mitigate such issues any observational measurement system requires a plan for developing and calculating inter-rater reliability, including rater training and scoring guides (Weaver et al. Citation2010).
Event-based measurement
Event-based measurement (EBAT) is a structured observation technique that is commonly applied to team simulation scenarios. Through the systematic introduction of events into training exercises, EBAT provides opportunities to observe specific behaviours of interest that are then marked as being either present or absent (Fowlkes et al. Citation1998). For example, situation awareness might be assessed in an aviation scenario by staging an event where the aircraft is deliberately steered off course by the trainer. The target behavioural response would be for the pilot to call a code word for ‘check navigation’ (Fowlkes et al. Citation1994), which would be scored as a hit or a miss. Through the use of such an approach, learning objectives can be clearly quantified, and the specific focal set of teamwork competencies to be trained can be defined. This is particularly valuable, as observers are only able to accurately assess four to five team-based constructs before they start to correlate and become redundant (Salas, Reyes, and Woods Citation2017). However, due to the staging of specific events, EBAT can only be used in training scenarios and not in real-world performance contexts. Furthermore, the development of EBAT measures is both time-consuming, and unique to the context and scenario for which they have been designed.
Automated measurement
Whilst observational methods offer some degree of unobtrusive assessment, the holy grail of team measurement are tools that capture the affective, behavioural, and cognitive properties of teamwork unobtrusively and dynamically, in real-time (Gorman et al. Citation2012; Salas, Reyes, and Woods Citation2017). Automated assessment collects data pertaining to a team through the computer systems they interact with and has the benefit of reducing disruption, minimising measurement errors, and lessening experimenter resources (Cooke et al. Citation2004). This type of measurement has most frequently and successfully been applied to the assessment of team performance (e.g. Martin and Foltz Citation2004; Frank et al. Citation2008; LaVoie et al. Citation2008). For example, LaVoie et al. (Citation2008) analysed recorded speech via statistical machine learning technologies to determine military unit performance and identify critical incidents that could be included in after-action reviews. Whilst such measures reflect effectiveness in their simulated environments, ideally, embedded measures should also evaluate team behaviours.
Communication, as one of the most critical team behaviours (Marlow, Lacerenza, et al. Citation2018), lends itself well to embedded measurement, as it can be automatically and continuously recorded during team tasks (Stanton and Roberts Citation2020). Measurement of communication can pertain to the physical properties (i.e. frequency, duration, volume), content (what is being said), or sequential flow of information exchange between team members (Kiekel et al. Citation2002). Research has failed to demonstrate a consistent relationship between communication frequency and team outcomes (Marlow, Lacerenza, et al. Citation2018), suggesting that it is communication quality (i.e. the extent to which communication adequately distributes pertinent information among team members) that is more integral to team performance than frequency (Marks, Zaccaro, and Mathieu Citation2000; Manser et al. Citation2013). This can only be assessed by analysing the content and flow of communication. Therefore, the derivation of any meaningful metrics from communication requires an element of human processing and can best be described as semi-automated (Granåsen 2018). A further consideration is the evaluation of the extent to which the physiological state of an operator impacts their cognitive function and in turn, affects their capacity to communicate Roberts and Cole Citation2018). Whilst such an approach may not be an explicit teamwork measure it can certainly help to promote understanding and prediction of how and when communications breakdowns are likely to occur.
Communication analysis has also been used to infer other team-based properties such as team cognition. The theory of interactive team cognition reasons that through interactions, team members coordinate cognitively with one another and create knowledge by integrating ideas (Cooke Citation2015). Therefore, cognition can be inferred through observing the processes and interactions between members (Cooke et al. Citation2013). Cooke and colleagues have leveraged communication data and interaction analysis to assess team cognition. The observation of team behaviour and communication in the face of unexpected change was used to measure team situation awareness (Gorman, Cooke, and Winner Citation2006). Research employing these measures reveals that high-performing teams exhibit consistent patterns of communication both in what they say (content) and who they say it to (flow).
Social network analysis (SNA) encompasses a set of methodological techniques that describe and explore patterns apparent in the social relationships between individuals and groups (Wasserman and Faust Citation1994). SNA has the potential to be developed as an automated measure, the raw data can certainly be captured with a high degree of autonomy but at present, it typically relies upon a degree of human data processing and interpretation of the output. SNA has also been utilised to study team communications and various other team-related constructs. It is worth noting that SNA has become popular as a measure of teamwork in ergonomics applications, with recent applications in emergency service operations (Houghton et al. Citation2006), healthcare (Barth, Schraagen, and Schmettow Citation2015; Salwei et al. Citation2019), railway maintenance (Walker et al. Citation2006), aviation (Stanton, Plant, Revell, et al. Citation2019), and defence (Stanton Citation2014). In addition, Stanton and colleagues have built upon the idea of networks to develop the Event Analysis of Systemic Teamwork (EAST; Stanton, Plant, Revell, et al. Citation2019) framework for measuring team performance as well as aspects of team cognition such as distributed situation awareness. EAST involves the use of three related networks for understanding teamwork and distributed cognition: task, social and information. Stanton (Citation2014) shows how these networks explain the work of the team (task networks), the relationships between the agents in the team (the social network documents communications between human and technological agents) and the content of what is communicated in pursuance of the tasks (the information network). For each of these networks, the global and nodal SNA metrics have been applied, which is an extension of SNA beyond the social network. Additionally, the efficiency gains for teams produced by restructuring the networks may be demonstrated (Stanton and Roberts Citation2020).
Evidently, the application of technology represents the future of team process and performance measurement due to the automated, dynamic, and unobtrusive nature of data collection afforded. Novel applications of the technology include the use of digital trace data (e.g. analysis of electronic mail data; Anderson and Kieliszewski Citation2018), wearable sensors (e.g. sociometric badges which use accelerometers, microphones, and/or optical sensors; Zhang et al. Citation2018), and computer-based laboratory simulations which emulate the behaviour of systems of interest (e.g. Kennedy and McComb Citation2014). These innovative approaches can all offer unique insight into team dynamics but pose new ethical challenges regarding the collection and management of ‘big data’ (Rosen et al. Citation2015), can be costly, and require rigorous construct validation prior to application (cf. Braun and Kuljanin Citation2015).
State of science: teamwork measurement
Traditional measurement approaches (i.e. surveys and observations) are problematic and ill-equipped to capture emergent team processes and process dynamics (Kozlowski and Chao Citation2018; Salmon, Clacy, and Dallat Citation2017). New technologies have the potential to address many of the aforementioned limitations inherent in traditional methods, yet the relative infancy of these automated measures necessitates a more considered method of assessment. The requirements for demonstrating validity may need to adapt in order to capitalise on the use of big data (Braun and Kuljanin Citation2015). Research needs to focus on more than just the processes subsumed within an IPO conceptualisation of team effectiveness to better capture the dynamic nature of teams. For example, research using sociometric badge data has demonstrated that uniform speaking time across members, proximal contact, and proximity time all predict team performance (Olguín and Pentland Citation2010). This new direction of research suggests that understanding patterns of team behaviour may reveal more about team performance than merely assessing static representations of teamwork processes. For example, Stanton, Walker, and Sorensen (Citation2012) showed how teams shifted dynamically between ‘attractors’ in phases space for an intelligence analysis task.
As with many HFE challenges, there does not appear to be a simple solution to the measurement of team functioning; because not all teams are created equally. Therefore, a one-size-fits-all approach would be inappropriate. For example, in future teams that include robots and artificial intelligence, self-report on the part of the technology is not feasible at least in its more traditional form. In terms of an optimal approach to measuring teamwork in ergonomics applications, it is clear that there are many options available, and that the methods adopted depend on various factors including the specific aims of the assessment, the resources available, and the level of access to the team in question. It is possible, however, to provide some general guidance. It is likely that a toolkit approach (such as the TeamSTEPPS approach developed by King et al. Citation2008) will be useful whereby various methods are used to capture data on the different team processes of interest. A toolkit approach has also worked well with the EAST framework (Stanton Citation2014) and indeed the concept could be pursued in future research to develop a toolkit of methods that cover each of the Big Five teamwork behaviours and coordinating mechanisms (Salas, Sims, and Burke Citation2005).
In terms of study design, many authors have also offered recommendations for the measurement of team performance (cf. Marlow, Bisbey, et al. Citation2018; Salas, Reyes, and Woods Citation2017), the most pertinent of these are summarised by Kozlowski and Chao (Citation2018) as follows:
Collect data unobtrusively;
Collect data from multiple sources;
Measure teams over time; and
Test the reliability and validity of measures.
To continue to progress the science of teamwork, future research needs to adopt these four principles.
Team development interventions
Teamwork is essential for the success of organisations across a variety of contexts (Lacerenza et al. Citation2018). However, teams often do not have the requisite skills to perform effectively as a collective from the outset. In addition, team members change over time, and even well-established teams may, over time, start to fail or underperform (Langan-Fox, Cooper, and Klimoski Citation2007). Given the potential consequences of teamwork failures (e.g. miscommunication), there is a compelling need to employ psychologically sound, empirically tested, TDIs. Team development interventions are defined as systematic activities designed to improve team competencies, processes, and effectiveness, generally falling within the categories of Team Building (TB) or Team Training (TT; Lacerenza et al. Citation2018). Whilst the principle aims of TB and TT are similar, the terms are not synonymous. Team building interventions have a more prominent focus on interpersonal relations and social interactions and are often employed to solve task-related problems (Klein et al. Citation2009; Shuffler, DiazGranados, and Salas Citation2011). Common TB strategies include goal setting, interpersonal relationship management, role clarification, and problem-solving. All have been found to have a moderately positive effect on team outcomes, but role clarification appears to be the most effective (Klein et al. Citation2009). More recently, however, TB has been used as a catchall for a variety of team-based interventions that have very little scientific underpinning (Shuffler et al. Citation2018). Team training interventions, conversely, adopt a more structured approach to developing the relevant knowledge, skills, and attitudes that underlie effective teamwork (Tannenbaum, Salas, and Cannon-Bowers Citation1996). It is perhaps unsurprising, therefore, that in all the TDI research, the evidence for TT is the strongest. For this reason, the present review focuses on TT rather than TB interventions.
Team training interventions
Various TT strategies have been employed across organisations, military, education, and healthcare, with those most commonly cited in the literature detailed in .
Table 4. Commonly cited team training interventions.
The research pertaining to TT interventions is broad and varied, with in excess of 60 scientific reviews undertaken to understand precisely what interventions work, for whom, and in what context. For example, one of the first meta-analyses regarding the efficacy of TT examined the relative contribution of four different TT strategies (CRM, TCAT, CT, and GTSC) on performance (Salas, Nichols, and Driskell Citation2007). Evaluated collectively, TT demonstrated a significant, small to moderate tendency to improve team performance. Specifically, TCAT was found to make the most significant contribution to the effectiveness of TT. Consequently, many of the TT interventions delivered since this meta-analysis bear the hallmarks of TCAT (e.g. teaches teamwork skills and tests them in a variety of challenging scenarios) without necessarily terming the intervention as such. Indeed, CRM is considered to be one of the most well-known and well-researched forms of team coordination training (Weaver et al. Citation2010). CRM, originally designed in response to a number of fatal aviation accidents, is a skills training programme that aims to normalise error and generate strategies for the management of error principally through the improvement of teamwork (Flin, O’Connor, and Mearns Citation2002; Kanki, Anca, and Chidester Citation2019). Meta-analyses have shown that CRM can have a significant positive effect on knowledge, attitudes, and behaviour in both the aviation (O’Connor et al. Citation2008) and healthcare (O’Dea, O’Connor, and Keogh Citation2014) industries.
CRM programmes were designed to increase the use of non-technical skills (the cognitive, social and personal resources that complement technical skills) to improve safety-critical behaviours on the flight deck. These principles have subsequently been applied within the medical profession in the form of non-technical skills training. Non-technical skills training is broader than TT in that it focuses on improving situation awareness, decision-making, teamwork, leadership, and the management of stress and fatigue. Nonetheless, evidence suggests that nontechnical skills training courses reduce the rate of surgical errors, improve teamwork and communication skills within the operating theatre, and improve understanding of leadership (Wood et al. Citation2017).
Guided team self-correction training tends to fall under the guise of team debriefs or after-action reviews (AAR) in the modern TDI literature, which are often included as part of a broad, multifaceted intervention (Shuffler et al. Citation2018). Debriefs, pioneered by the military decades ago, encourage teams to discuss, interpret, and learn from recent team events (Allen et al. Citation2018). There is robust evidence for the effectiveness of team debriefs, with a meta-analysis revealing a 25% improvement in team performance following the application of appropriately structured debriefs (Tannenbaum and Cerasoli Citation2013). The meta-analysis recommends that AARs should promote active self-learning, have a developmental intent, focus on specific events, and use multiple information sources in order to have the greatest impact on teamwork and performance.
The application of systematic programmes designed to enhance leader knowledge, skills, and abilities represent an important TDI with the potential to enhance overall team performance (Day Citation2000; Avolio, Walumbwa, and Weber Citation2009). A recent meta-analysis suggests that Leadership Training (LT) is more effective than previously thought; likely to improve team outcomes by up to 29% regardless of design, delivery, or elements trained (Lacerenza et al. Citation2017). The most effective LT programmes are based on an analysis of the needs of the team leader, the provision of feedback, and the use of multiple delivery methods (information, demonstration, and practice) (Lacerenza et al. Citation2017).
Collectively, the state of science on TDIs provides meta-analytic evidence that TT improves teamwork and team performance in medical (Hughes et al. Citation2016), organisational (McEwan et al. Citation2017), and military contexts (Goodwin, Blacksmith, and Coats Citation2018). Team debriefs can improve team performance (Tannenbaum and Cerasoli Citation2013), and LT improves leader capabilities and provides numerous positive outcomes for followers, teams, and organisations (Lacerenza et al. Citation2017).
Team training delivery methods
Whilst there is strong empirical support for TT, the practice of TT is extremely broad, encompassing a range of learning strategies, methods and teamwork competencies (Cannon-Bowers and Salas Citation1997). The means by which TT programs are delivered has been found to be a significant moderator of effectiveness (McEwan et al. Citation2017; Weaver, Dy, and Rosen Citation2014). These are described in detail in .
Table 5. Team training methods.
Although there is reasonable evidence to suggest that classroom-based TT interventions can improve teamwork processes (Weaver, Dy, and Rosen Citation2014), recent research has found that TT interventions that targeted didactic instruction alone did not result in significant improvements in teamwork (McEwan et al. Citation2017). Demonstration methods, despite representing more active forms of learning, are not widely employed, with only 35% of TT programs reporting demonstration-based activities (Weaver et al. Citation2010). Conversely, simulation-based training, adopted in 68% of TT programs, is widely considered the most critical TT delivery method (Weaver et al. Citation2010). Compared with no intervention, simulation-based training has been found to improve knowledge, technical skills, and behavioural learning (Cook et al. Citation2011). Consequently, simulation is considered a powerful tool to enhance teamwork (Salas, Reyes, and McDaniel Citation2018). Simulation training is considered a subset of the broader practice-based TT methods, yet simulation activities predominate the literature and have the strongest evidence of effectiveness (Buljac-Samardzic, Doekhie, and van Wijngaarden Citation2020). As such, the term is commonly used to represent all experiential activities that enable teams to enact teamwork skills (as is the case in McEwan et al. Citation2017), thus the term is adopted accordingly throughout this review.
State of science: team development interventions
Team training works when ‘done right’ and appropriately informed by the scientific literature (Salas, Reyes, and Woods Citation2017). This requires an initial assessment of the individual and teamwork behaviours necessary for successful team performance (Shuffler et al. Citation2018). Whilst there are some team-generic, transportable teamwork competencies (e.g. Smith-Jentsch et al. Citation2008), no two teams are the same. Therefore, a thorough team-level needs analysis should be undertaken to ensure that the TDI delivered appropriately targets the specific team competencies needed for success. Various ergonomics methods have been used to support this, including Work Domain Analysis (WDA; Naikar Citation2013), Hierarchical Task Analysis (HTA; Stanton Citation2006), and Team Task Analysis (TTA; Burke Citation2004). Multifaceted TDIs appear to be most successful at improving team performance, as long as they focus on the most pertinent, context-specific teamwork skills. Furthermore, the most effective TDIs adopt a multi-method delivery approach, including appropriate instructional strategies, demonstration of skills, simulation of teamwork activities, and team debriefs. Finally, the application of robust measurement tools is required for the diagnosis of team functioning, and evaluation of overall effectiveness (Driskell, Salas, and Driskell Citation2018; Salas, Reyes, and McDaniel Citation2018).
Many recent reviews of the teamwork literature discuss the relative value of novel, technology-based measures of teamwork. However, there does not appear to be a corresponding interest in the utilisation of technology in TDIs. Distance learning, computer-based training, and computer-assisted instruction (Salas, Reyes, and Woods Citation2017) are examples of methods that require further evaluation. Many teams are geographically dispersed (especially during the current COVID-19 pandemic), thus advances in technology need to be exploited in order to provide TT solutions that cater to the increasing complexity and diversity of teams.
Another concern that has not received a great deal of research attention is the sustainability of changes in teamwork. Currently very little is understood about the half-life of interventions, or indeed the methods and techniques required to ‘top up’ both individual and team-based competencies. Feedback, as a critical feature of TT (Weaver et al. Citation2010) has been identified as a potential means of providing individuals and teams with ongoing insight into their performance. However, it is unknown whether this would be sufficient to maintain any improvements in teamwork obtained as a result of TT.
Those responsible for implementing TDIs also need to consider the relative value of delivering individual versus collective TT. Ellis et al. (Citation2005) provided evidence that generic teamwork skills training could promote team effectiveness, yet teams were trained and assessed as a collective. It would be particularly insightful to compare the effects of members trained individually or collectively and to evaluate these approaches when members are placed back into either established or augmented teams. Large-scale military training exercises could, potentially, utilise synthetic team-mates so that individuals can get TT anytime, anywhere without having to utilise mass human and technology resources (Myers et al. Citation2019).
Finally, there is a growing field of literature dedicated to understanding multi-team systems (MTS: see Stanton et al. Citation2010) and the ways in which such systems are similar and/or different to standalone teams in regard to the teamwork competencies required for success. An MTS is defined as a network of teams that interact to complete a general collective goal, while simultaneously pursuing various immediate and interdependent goals (Fleştea et al. Citation2017). An interesting avenue of research is evolving around the development of these nested, hierarchical structures, and whether the scientific principles of TDIs can be applied to improve the performance of MTS.
On the future of teams and teamwork
A secondary aim of the current review was to evaluate the extent to which existing models and measures are suitable to support team design, operation and evaluation in future systems. Advanced automation, robotics AI are already with us (Hancock Citation2019), and the next generation of AI, AGI, may soon arrive (Salmon, Stevens, and Carden Citation2018; Johnson and Vera, Citation2019). Teams are changing as a result. In road transport, for example, we already have a driver and automated vehicle teams (Banks and Stanton Citation2019), and this will soon expand to teams comprising multiple connected vehicles (Banks et al. Citation2018). Likewise, in defence non-human agent team members are increasingly being introduced to teams in areas such as land warfare and aviation (Ball et al. Citation2010). The capabilities of these non-human agents are also increasing dramatically. With AGI, for example, non-human team members will be able to perform all of the intellectual tasks that humans can, and they will have the capacity to learn, solve problems, self-improve, and undertake tasks that they were not originally designed for (Everitt, Lea, and Hutter Citation2018; Gurkaynak, Yilmaz, and Haksever Citation2016; Kaplan and Haenlein Citation2019). There is an opportunity to enhance and optimise teams and teamwork through such technologies; however, it is important to question whether existing human-focussed models can direct this evolution, or whether they will in fact restrict it. A clear observation from the current review is that there is a dearth of literature concerning how contemporary models and approaches to the measurement of teamwork may be applied to teams incorporating non-human agents. That is not to say that human-machine teaming research does not exist, it clearly does. However, such work is typically focussed on task work and/or does not appear to build upon existing knowledge of human-human teamwork processes.
There is controversy concerning whether AI or robots (embodied AI) are tools or team-mates (Groom and Nass Citation2007; Klein et al. Citation2004; Seeber et al. Citation2020). A large part of the concern is over the degree of human control that is possible over nonhuman teammates (Shneiderman Citation2020). Taking into account the aforementioned definition of a team – a group of individuals with specific roles who interact interdependently towards a common goal – it is not clear that AGI is the answer. Why replicate humans when what we need is for AI to do the jobs that humans are either incapable of doing or do not want to do because the task is dull, dirty, or dangerous? In addition, to speak to the tool vs. team-mate argument, it has been acknowledged that there are teams of humans and animals (e.g. bomb-sniffing dogs and dolphins that find ordinance) and so it may be time to start thinking about technology as a team member of a different species. This makes a great difference regarding how we assess these teammates and intervene to improve these teams. Some of our models, theories, measures, and interventions for teamwork need to evolve to accommodate these new team-mates.
In the case of teamwork models, it seems apparent that work is required both to test models and to extend them to enable the consideration of technology-based team members. With the Big Five model, for example, it is not clear how well the five behaviours and three coordinating mechanisms apply to human and non-human agent teams. Indeed, problems have been found when applying the Big Five model to consider only the most basic of non-human agent team members such as radio communications technology (Neville, Salmon, and Read Citation2018). For more advanced technologies such as AI, AGI and robotics, there has been no examination of how behaviours such as team orientation and backup behaviour should occur. Likewise, the sharing of mental models between human and non-human agents is difficult to either achieve or test. In addition, it may be that new important behaviours and coordinating mechanisms need to be introduced. Trust (Lee Citation2019), reliability (Hancock Citation2019) and automation transparency (Kunze et al. Citation2019), for example, will become far more important and transactions in situation awareness between human and non-human agents will also be prevalent (Stanton et al. Citation2006, Citation2017; Stanton, Plant, Roberts, et al. Citation2019). It is logical then to argue that further work is required to build on state-of-the-art models and ensure that they remain fit for purpose. In the case of Salas and colleagues’ model, it is likely that there will be more than just a Big Five for human and non-human agent teams.
A route forward for describing and measuring human-automation/AI-agent teaming may initially be via the effective synthesis of research with seemingly different objectives, a route well-trodden by Human Factors. The success of an automated system artefact is reliant on a successful partnership between a human operator and automation (Endsley Citation2017). The disuse of automation refers to circumstances in which automation is underutilised by an operator or there is a rejection of its capabilities (Lee and See Citation2004; Parasuraman and Riley Citation1997). The correct usage of automation depends on the attitudes of the operator, such as trust, knowledge of the automation, but also features of the automation itself, such as data feedback, reliability and usability. Trust in this context refers to the attitude that an automated agent will help achieve an individual’s goals in a situation characterised by uncertainty and vulnerability (Lee and See Citation2004). Operators’ subjective trust in automation is largely based upon their perceptions of its competence (Muir and Moray Citation1996). This certainly appears to have parallels with the manner in which trust is cultivated in human teams. The usability of a system is defined in ISO 9241-11 as the extent to which a product can be used by specified users to achieve specified goals with effectiveness, efficiency and satisfaction in a specified context of use (Jokela et al. Citation2003). The concept of usability is multifaceted and includes constructs such as simplicity, visual acuity, feedback, learnability, efficiency, satisfaction and memorability. The vast amount of such constructs centre around the efficacy of communication. It certainly appears that factors such as trust and communication which play a significant role in human-human teamwork efficacy are also fundamental considerations during the design and integration of automated agents. A question concerns whether the application of such work could be extended to serve as a framework within which human- AI-agent teamwork can be evaluated. Is an automated agent with a high degree of usability considered to be a better team member, or even have higher team orientation? Is the manner in which automation state data is communicated to a human operator more likely to engage performance monitoring behaviours, leading to back-up behaviours? Can the construct of human trust in technology provide insights into the establishment of bi-directional trust between human and AI agents working in a team based environment? It is clear that at present we are left with more questions than answers. However, consideration of how to train and measure effective human–automation interactions and assess human performance in systems with varying degrees of automation will continue to grow (Roberts et al. Citation2020).
The need for an extension is even more critical with teamwork measures. The reliance to date on self-report measures raises pertinent questions regarding the capacity to assess teamwork in human and non-human agent teams. Indeed, questions can be legitimately raised regarding the applicability of existing human-centred measures to teams comprising human and increasingly intelligent non-human team members. For example, it will be difficult to gather self-report ratings of teamwork from non-human team members unless this is explicitly considered when designing them. Important dimensions of teamwork will also become critical measures, such as trust and transparency. Likewise, the capacity of non-human team members to gather data during team performance (e.g. communications logs, sensing data) creates new possibilities for additional measures, including new automated measures using big data.
Conclusions
The literature review has revealed that a vast number of frameworks of team effectiveness are available and a lack of consensus exists with respect to the precise factors that underpin teamwork. Regardless of the framework adopted, team processes and emergent states have received the most frequent research attention to date. These mediating variables, which are often cognitive in nature enable the integration of individuals’ effort towards the accomplishment of shared goals and represent the very essence of teamwork (Kozlowski Citation2018). It is clear from the review that team training works when ‘done right’ and is appropriately informed by the scientific literature (Salas, Reyes, and Woods Citation2017). This requires an initial assessment of the individual and teamwork behaviours necessary for successful team performance. The state of science on TDIs provides meta-analytic evidence that TT improves teamwork and team performance across a wealth of domains and contexts. Team debriefs can improve team performance (Tannenbaum and Cerasoli Citation2013), and LT improves leader capabilities and provides numerous positive outcomes for followers, teams, and organisations.
The vast number of frameworks of team effectiveness available and lack of consensus on the precise factors that teamwork consists of, has resulted in the measurement and development of teamwork being correspondingly diverse. The various measurement instruments available assess either individual and group processes or emergent states, or a combination thereof. Well over 220 measures of teamwork were identified with a number of general issues identified. This included an over-reliance on self-report measures, inconsistent reporting of reliability and validity testing, and an almost exclusive focus on the measurement of teamwork in healthcare settings. Traditional measurement approaches (i.e. surveys and observations) are problematic and ill-equipped to capture emergent team processes and process dynamics (Kozlowski and Chao Citation2018; Salmon, Clacy, and Dallat Citation2017). New technologies and novel measurement approaches (e.g. SNA) have the potential to address many of the shortfalls inherent in traditional methods, yet the relative infancy of these automated measures necessitate a more considered method of assessment.
A secondary aim of the review was to determine whether state-of-the-art teamwork models and measures remain fit for purpose given the changing nature of teams, teamwork, and the environments in which they operate. The current review has indicated that the models (such as IMIO and the Big Five) that are being used to describe human teams will need to change in order for them to describe the work of human-AI-agent teams. New factors and interactions will need to be identified and defined to account for human-AI-agent teaming and teamwork. It is perhaps on the topic of teamwork measurement that the greatest synergy can be found with respect to the current state of science in human teams and future requirements for teams containing non-human agents. The current review of the literature suggested that the tools which capture the affective, behavioural, and cognitive properties of teamwork unobtrusively and dynamically are the holy grail of teamwork measurement (Gorman et al. Citation2012; Salas, Reyes, and Woods Citation2017). Automated assessment collects data pertaining to a team through the computer systems they interact with and has the benefit of reducing disruption, minimising measurement errors, and lessening experimenter resources (Cooke et al. Citation2004). The drive towards technology-supported measurement of teamwork and team performance will not only help to promote greater efficacy, objectivity and reliability to advance contemporary understanding of human-centred teams. It will also provide a window of opportunity to identify and develop our understanding of new factors and interactions to account for human-AI-agent teaming and teamwork.
Abbreviations | ||
TDI | = | team development intervention |
IPO | = | input process outcome |
IMOI | = | input mediator output input |
STEPPS | = | team strategies and tools to enhance performance and patient safety |
TCI | = | team climate inventory |
NOTECHS | = | nontechnical skills |
TB | = | team building |
TT | = | team training |
CRM | = | crew resource management |
MTS | = | multi team systems |
Acknowledgements
The authors would like to thank Sarah Twist, Daniel Fay and Kiome Pope for their help compiling literature and reviewing earlier document drafts. Professor Neville A Stanton’s time on this review has been supported by the Underwater Systems Programme of the UK Ministry of Defence Science & Technology Research Programme, contract TIN 3.113.
Disclosure statement
No potential conflict of interest was reported by the author(s).
Additional information
Funding
References
- Abdallah, M., M. A. Talib, S. Feroz, Q. Nasir, H. Abdalla, and B. Mahfood. 2020. “Artificial Intelligence Applications in Solid Waste Management: A Systematic Research Review.” Waste Management 109: 231–246.
- Allen, J. A., R. Reiter-Palmon, J. Crowe, and C. Scott. 2018. “Debriefs: Teams Learning from Doing in Context.” American Psychologist 73 (4): 504–516. doi:https://doi.org/10.1037/amp0000246.
- Anderson, L. C., and C. A. Kieliszewski. 2018. “Using Digital Trace Analytics to Understand and Enhance Scientific Collaboration.” Paper presented at the International Conference on Applied Human Factors and Ergonomics, Orlando, FL, July 22–26.
- Anderson, N., and M. A. West. 1996. “The Team Climate Inventory: Development of the TCI and Its Applications in Teambuilding for Innovativeness.” European Journal of Work and Organizational Psychology 5 (1): 53–66. doi:https://doi.org/10.1080/13594329608414840.
- Andersson, D., A. Rankin, and D. Diptee. 2017. “Approaches to Team Performance Assessment: A Comparison of Self-Assessment Reports and Behavioral Observer Scales.” Cognition, Technology & Work 19 (2–3): 517–528. doi:https://doi.org/10.1007/s10111-017-0428-0.
- Annett, J. A., and N. A. Stanton. 2000. “Team Work-A Problem For Ergonomics?” Ergonomics 43 (8): 1045–1051. doi:https://doi.org/10.1080/00140130050084860.
- Aronson, M. 2019. Rising Water: The Story of the Thai Cave Rescue. New York, NY: Simon and Schuster.
- Avolio, B. J., F. O. Walumbwa, and T. J. Weber. 2009. “Leadership: Current Theories, Research, and Future Directions.” Annual Review of Psychology 60: 421–449. doi:https://doi.org/10.1146/annurev.psych.60.110707.163621.
- Ball, J., C. Myers, A. Heiberg, N. J. Cooke, M. Matessa, M. Freiman, and S. Rodgers. 2010. “The Synthetic Teammate Project.” Computational and Mathematical Organization Theory 16 (3): 271–299. doi:https://doi.org/10.1007/s10588-010-9065-3.
- Banks, V. A., and N. A. Stanton. 2019. “Analysis of Driver Roles: Modelling the Changing Role of the Driver in Automated Driving Systems Using EAST.” Theoretical Issues in Ergonomics Science 20 (3): 284–300. doi:https://doi.org/10.1080/1463922X.2017.1305465.
- Banks, V. A., N. A. Stanton, G. Burnett, and S. Hermawati. 2018. “Distributed Cognition on the Road: Using EAST to Explore Future Road Transportation Systems.” Applied Ergonomics 68: 258–266. doi:https://doi.org/10.1016/j.apergo.2017.11.013.
- Barth, S., J. M. Schraagen, and M. Schmettow. 2015. “Network Measures for Characterising Team Adaptation Processes.” Ergonomics 58 (8): 1287–1302. doi:https://doi.org/10.1080/00140139.2015.1009951.
- Bell, S. T. 2007. “Deep-Level Composition Variables as Predictors of Team Performance: A Meta-Analysis.” The Journal of Applied Psychology 92 (3): 595–615. doi:https://doi.org/10.1037/0021-9010.92.3.595.
- Bell, S. T., S. G. Brown, A. Colaneri, and N. Outland. 2018. “Team Composition and the ABCs of Teamwork.” American Psychologist 73 (4): 349–362. doi:https://doi.org/10.1037/amp0000305.
- Benishek, L. E., and E. H. Lazzara. 2019. “Teams in a New Era: Some Considerations and Implications.” Frontiers in Psychology 10: 1006. doi:https://doi.org/10.3389/fpsyg.2019.01006.
- Braun, M. T., and G. Kuljanin. 2015. “Big Data and the Challenge of Construct Validity.” Industrial and Organizational Psychology 8 (4): 521–527. doi:https://doi.org/10.1017/iop.2015.77.
- Brennan, S. E., M. Bosch, H. Buchan, and S. E. Green. 2013. “Measuring Team Factors Thought to Influence the Success of Quality Improvement in Primary Care: A Systematic Review of Instruments.” Implementation Science: IS 8 (20): 20–17. doi:https://doi.org/10.1186/1748-5908-8-20.
- Brown, T. C., and G. P. Latham. 2002. “The Effects of Behavioural Outcome Goals, Learning Goals, and Urging People to Do Their Best on an Individual’s Teamwork Behaviour in a Group Problem-Solving Task.” Canadian Journal of Behavioural Science 34 (4): 276–285. doi:https://doi.org/10.1037/h0087180.
- Buljac-Samardzic, M., K. D. Doekhie, and J. D. H. van Wijngaarden. 2020. “Interventions to Improve Team Effectiveness within Health Care: A Systematic Review of the past Decade.” Human Resources for Health 18 (1): 2. doi:https://doi.org/10.1186/s12960-019-0411-3.
- Burke, C. S. 2004. “Team Task Analysis.” In Handbook of Human Factors and Ergonomics Methods, edited by N. Stanton, A. Hedge, K. Brookhuis, E. Salas, and H. W. Hendrick, 552–561. Boca Raton, FL: CRC Press.
- Burke, C. S., K. C. Stagl, E. Salas, L. Pierce, and D. Kendall. 2006. “Understanding Team Adaptation: A Conceptual Analysis and Model.” Journal of Applied Psychology 91 (6): 1189–1207. doi:https://doi.org/10.1037/0021-9010.91.6.1189.
- Burtscher, M. J., J. Wacker, G. Grote, and T. Manser. 2010. “Managing Nonroutine Events in Anesthesia: The Role of Adaptive Coordination.” Human Factors 52 (2): 282–294.
- Cannon-Bowers, J. A., and C. Bowers. 2011. “Team Development and Functioning.” In APA handbook of industrial and organizational psychology, Vol. 1. Building and Developing the Organization, edited by S. Zedeck, 597–650. Washington, DC: American Psychological Association.
- Cannon-Bowers, J. A., and E. Salas. 1990Training. “Cognitive Psychology and Team Training: Shared Mental Models in Complex Systems.” Human Factors Society Bulletin 33 (12): 1–4.
- Cannon-Bowers, J. A., and E. Salas. 1997. “A Framework for Developing Team Performance Measures in Training.” In Team Performance Assessment and Measurement, edited by M. T. Brannick, E. Salas, and C. Prince, 57–74. Hillsdale, NJ: Lawrence Erlbaum Associates.
- Cannon-Bowers, J. A., E. Salas, E. Blickensderfer, and C. A. Bowers. 1998. “The Impact of Cross-Training and Workload on Team Functioning: A Replication and Extension of Initial Findings.” Human Factors 40 (1): 92–101. doi:https://doi.org/10.1518/001872098779480550.
- Cannon-Bowers, J. A., E. Salas, and S. Converse. 1993. “Shared Mental Models in Expert Team Decision Making.” In Current Issues in Individual and Group Decision Making, edited by J. Castellan Jr., 221–246. Hillsdale, NJ: Lawrence Erlbaum.
- Carron, A. V., S. R. Bray, and M. A. Eys. 2002. “Team Cohesion and Team Success in Sport.” Journal of Sports Sciences 20 (2): 119–126. doi:https://doi.org/10.1080/026404102317200828.
- Carron, A. V., L. J. Martin, and T. M. Loughead. 2012. “Teamwork and Performance.” In The Oxford Handbook of Sport and Performance Psychology, edited by S. N. Murphy, 309–327. New York, NY: Oxford University Press.
- Cohen, S. G., and D. E. Bailey. 1997. “What Makes Teams Work: Group Effectiveness Research from the Shop Floor to the Executive Suite.” Journal of Management 23 (3): 239–290. doi:https://doi.org/10.1177/014920639702300303.
- Cook, D. A., R. Hatala, R. Brydges, B. Zendejas, J. H. Szostek, A. T. Wang, P. J. Erwin, and S. J. Hamstra. 2011. “Technology-Enhanced Simulation for Health Professions Education: A Systematic Review and Meta-Analysis.” JAMA 306 (9): 978–988. doi:https://doi.org/10.1001/jama.2011.1234.
- Cooke, N. J. 2015. “Team Cognition as Interaction.” Current Directions in Psychological Science 24 (6): 415–419. doi:https://doi.org/10.1177/0963721415602474.
- Cooke, N. J., J. C. Gorman, C. W. Myers, and J. L. Duran. 2013. “Interactive Team Cognition.” Cognitive Science 37 (2): 255–285. doi:https://doi.org/10.1111/cogs.12009.
- Cooke, N. J., E. Salas, J. A. Cannon-Bowers, and R. Stout. 2000. “Measuring Team Knowledge.” Human Factors 42 (1): 151–173.
- Cooke, N. J., E. Salas, P. A. Kiekel, and B. Bell. 2004. “Advances in Measuring Team Cognition.” In Team Cognition: Understanding the Factors That Drive Process and Performance, edited by E. Salas and S. M. Fiore, 83–106. Washington, DC: American Psychological Association.
- Costa, A. C., R. A. Roe, and T. Taillieu. 2001. “Trust within Teams: The Relation with Performance Effectiveness.” European Journal of Work and Organizational Psychology 10 (3): 225–244. doi:https://doi.org/10.1080/13594320143000654.
- Coultas, C. W., T. Driskell, C. S. Burke, and E. Salas. 2014. “A Conceptual Review of Emergent State Measurement: Current Problems, Future Solutions.” Small Group Research 45 (6): 671–703. doi:https://doi.org/10.1177/1046496414552285.
- Dalenberg, S., A. L. Vogelaar, and B. Beersma. 2009. “The Effect of a Team Strategy Discussion on Military Team Performance.” Military Psychology 21 (2): S31–S46. doi:https://doi.org/10.1080/08995600903249107.
- Day, D. V. 2000. “Leadership Development: A Review in Context.” The Leadership Quarterly 11 (4): 581–613. doi:https://doi.org/10.1016/S1048-9843(00)00061-8.
- De Crespigny, R. 2012. QF32: Life Lessons from the Cockpit of QF32. New York, NY: Macmillan Publishers.
- Dekker, S. W. A. 2000. “Crew Situation Awareness in High-Tech Settings: Tactics for Research into an Ill-Defined Phenomenon.” Transportation Human Factors 2 (1): 49–62. doi:https://doi.org/10.1207/STHF0201_7.
- Delice, F., M. Rousseau, and J. Feitosa. 2019. “Advancing Teams Research: What, When, and How to Measure Team Dynamics over Time.” Frontiers in Psychology 10: 1324. doi:https://doi.org/10.3389/fpsyg.2019.01324.
- Dietz, A. S., P. J. Pronovost, K. N. Benson, P. A. Mendez-Tellez, C. Dwyer, R. Wyskiel, and M. A. Rosen. 2014. “A Systematic Review of Behavioural Marker Systems in Healthcare: What Do we Know about Their Attributes, Validity and Application?” BMJ Quality & Safety 23 (12): 1031–1039. doi:https://doi.org/10.1136/bmjqs-2013-002457.
- Donadio, F., J. Frejaville, S. Larnier, and S. Vetault. 2018. “Artificial Intelligence and Collaborative Robot to Improve Airport Operations.” In Online Engineering & Internet of Things, 973–986. Cham, Switzerland: Springer.
- Driskell, J. E., E. Salas, and T. Driskell. 2018. “Foundations of Teamwork and Collaboration.” American Psychologist 73 (4): 334–348. doi:https://doi.org/10.1037/amp0000241.
- Dyer, J. L. 1984. “Team Research and Team Training: A State-of-the-Art Review.” Human Factors Review 26: 285–323.
- Edmondson, A. 1999. “Psychological Safety and Learning Behavior in Work Teams.” Administrative Science Quarterly 44 (2): 350–383. doi:https://doi.org/10.2307/2666999.
- Ellis, A. P., B. S. Bell, R. E. Ployhart, J. R. Hollenbeck, and D. R. Ilgen. 2005. “An Evaluation of Generic Teamwork Skills Training with Action Teams: Effects on Cognitive and Skill‐Based Outcomes.” Personnel Psychology 58 (3): 641–672. doi:https://doi.org/10.1111/j.1744-6570.2005.00617.x.
- Endsley, M. R. 2017. “From Here to Autonomy: Lessons Learned from Human–Automation Research.” Human Factors 59 (1): 5–27. doi:https://doi.org/10.1177/0018720816681350.
- Everitt, T., G. Lea, and M. Hutter. 2018. AGI safety literature review. International Joint Conference on Artificial Intelligence (IJCAI), arXiv preprint arXiv:1805.01109.
- Fiore, S., M. A. Rosen, K. A. Smith-Jentsch, E. Salas, M. Letsky, and N. Warner. 2010. “Toward an Understanding of Macrocognition in Teams: Predicting Processes in Complex Collaborative Contexts.” Human Factors 52 (2): 203–224. doi:https://doi.org/10.1177/0018720810369807.
- Fleştea, A. M., O. C. Fodor, P. L. Curşeu, and M. Miclea. 2017. “‘We Didn’t Know Anything, it was a Mess!’ Emergent Structures and the Effectiveness of a Rescue Operation Multi-Team System.” Ergonomics 60 (1): 44–58.
- Fletcher, G., R. Flin, P. McGeorge, R. Glavin, N. Maran, and R. Patey. 2003. “Anaesthetists’ Non‐Technical Skills (ANTS): Evaluation of a Behavioural Marker System.” British Journal of Anaesthesia 90 (5): 580–588. doi:https://doi.org/10.1093/bja/aeg112.
- Flin, R., and N. Maran. 2004. “Identifying and Training Non-Technical Skills for Teams in Acute Medicine.” Quality and Safety in Health Care 13 (1): i80–i84. doi:https://doi.org/10.1136/qshc.2004.009993.
- Flin, R., L. Martin, K.-M. Goeters, H.-J. Hörmann, R. Amalberti, C. Valot, and H. Nijhuis. 2003. “Development of the NOTECHS (Non-Technical Skills) System for Assessing Pilots’ CRM Skills.” Human Factors and Aerospace Safety 3 (2): 97–119.
- Flin, R., P. O’Connor, and K. Mearns. 2002. “Crew Resource Management: Improving Teamwork in High Reliability Industries.” Team Performance Management 8 (3–4): 68–78. doi:https://doi.org/10.1108/13527590210433366.
- Ford, K., M. Menchine, E. Burner, S. Arora, K. Inaba, D. Demetriades, and B. Yersin. 2016. “Leadership and Teamwork in Trauma and Resuscitation.” The Western Journal of Emergency Medicine 17 (5): 549–556. doi:https://doi.org/10.5811/westjem.2016.7.29812.
- Fouse, S., N. J. Cooke, J. C. Gorman, I. Murray, M. Uribe, and A. Bradbury. 2011. “Effects of Role and Location Switching on Team Performance in a Collaborative Planning Environment.” Paper presented at the 55th Annual Conference of the Human Factors and Ergonomics Society, Santa Monica, CA. doi:https://doi.org/10.1177/1071181311551300.
- Fowlkes, J., D. J. Dwyer, R. L. Oser, and E. Salas. 1998. “Event-Based Approach to Training (EBAT).” The International Journal of Aviation Psychology 8 (3): 209–221. doi:https://doi.org/10.1207/s15327108ijap0803_3.
- Fowlkes, J. E., N. E. Lane, E. Salas, T. Franz, and R. Oser. 1994. “Improving the Measurement of Team Performance: The TARGETs Methodology.” Military Psychology 6 (1): 47–61. doi:https://doi.org/10.1207/s15327876mp0601_3.
- Frank, G., N. Evens, R. Hubal, and B. Whiteford. (2008). “Automated, Interactive AARs: A Positive Spin.” Paper presented at the 2008 Interservice/Industry Training, Simulation, and Education Conference (I/ITSEC). Orlando, FL, December 1–8.
- Goodwin, G. F., N. Blacksmith, and M. R. Coats. 2018. “The Science of Teams in the Military: Contributions from over 60 Years of Research.” American Psychologist 73 (4): 322–333. doi:https://doi.org/10.1037/amp0000259.
- Gordon, M., D. Darbyshire, and P. Baker. 2012. “Non‐Technical Skills Training to Enhance Patient Safety: A Systematic Review.” Medical Education 46 (11): 1042–1054. doi:https://doi.org/10.1111/j.1365-2923.2012.04343.x.
- Gorman, J. C., N. J. Cooke, and P. G. Amazeen. 2010. “Training Adaptive Teams.” Human Factors 52 (2): 295–307.
- Gorman, J. C., N. J. Cooke, and J. L. Winner. 2006. “Measuring Team Situation Awareness in Decentralized Command and Control Systems.” Ergonomics 49 (12–13): 1312–1325.
- Gorman, J. C., E. E. Hessler, P. G. Amazeen, N. J. Cooke, and S. M. Shope. 2012. “Dynamical Analysis in Real Time: Detecting Perturbations to Team Communication.” Ergonomics 55 (8): 825–839. doi:https://doi.org/10.1080/00140139.2012.679317.
- Granåsen, M. 2019. Exploring C2 Capability and Effectiveness in Challenging Situations: Interorganizational Crisis Management, Military Operations and Cyber Defence. Vol. 1836. Linköping, Sweden: Linköping University Electronic Press.
- Groom, V., and C. Nass. 2007. “Can Robots Be Teammates?: Benchmarks in Human-Robot Teams.” Interaction Studies 8 (3): 285–301. doi:https://doi.org/10.1075/gest.8.3.02str.
- Grote, G., M. Kolbe, and M. J. Waller. 2018. “The Dual Nature of Adaptive Coordination in Teams: Balancing Demands for Flexibility and Stability.” Organizational Psychology Review 8 (2–3): 125–148. doi:https://doi.org/10.1177/2041386618790112.
- Gurkaynak, G., I. Yilmaz, and G. Haksever. 2016. “Stifling Artificial Intelligence: Human Perils.” Computer Law & Security Review 32 (5): 749–758. doi:https://doi.org/10.1016/j.clsr.2016.05.003.
- Hancock, P. A. 2019. “Some Pitfalls in the Promises of Automated and Autonomous Vehicles.” Ergonomics 62 (4): 479–495. doi:https://doi.org/10.1080/00140139.2018.1498136.
- Havyer, R. D. A., M. T. Wingo, N. I. Comfere, D. R. Nelson, A. J. Halvorsen, F. S. McDonald, and D. A. Reed. 2013. “Teamwork Assessment in Internal Medicine: A Systematic Review of Validity Evidence and Outcomes.” Journal of Internal Medicine 29 (6): 894–910. doi:https://doi.org/10.1007/s11606-013-2686-8.
- Health and Safety Executive. 2015a. “Factual Report.” Accessed 12 November 2021. https://www.whatdotheyknow.com/request/379886/response/916683/attach/3/HSE%20Factual%20Report%20of%20The%20Smiler%20Ride%20incident.pdf
- Health and Safety Executive. 2015b. “Expert Witness Report.” Accessed 12 November 2021. https://www.whatdotheyknow.com/request/379886/response/916683/attach/html/4/Expert%20witness%20report%20from%20Steven%20Flanagan.pdf.html
- Hessler, G., and K. H. Baringhaus. 2018. “Artificial Intelligence in Drug Design.” Molecules 23 (10): 2520. doi:https://doi.org/10.3390/molecules23102520.
- Hinski, S., N. Cooke, N. McNeese, A. Sen, and B. Patel. 2016. “A Human Factors Approach to Building High-Performance Multi-Professional Cardiac Arrest Teams: Developing a Code Blue Team Performance Metric.” Paper presented at the HFES 2016 International Symposium on Human Factors and Ergonomics in Health Care, San Diego, CA, April 15.
- Hoegl, M., and H. G. Gemuenden. 2001. “Teamwork Quality and the Success of Innovative Projects: A Theoretical Concept and Empirical Evidence.” Organization Science 12 (4): 435–449. doi:https://doi.org/10.1287/orsc.12.4.435.10635.
- Houghton, R. J., C. Baber, R. McMaster, N. A. Stanton, P. Salmon, R. Stewart, and G. Walker. 2006. “Command and Control in Emergency Services Operations: A Social Network Analysis.” Ergonomics 49 (12-13): 1204–1225. doi:https://doi.org/10.1080/00140130600619528.
- Hughes, A. M., M. E. Gregory, D. L. Joseph, S. C. Sonesh, S. L. Marlow, C. N. Lacerenza, L. E. Benishek, H. B. King, and E. Salas. 2016. “Saving Lives: A Meta-Analysis of Team Training in Healthcare.” The Journal of Applied Psychology 101 (9): 1266–1304. doi:https://doi.org/10.1037/apl0000120.
- Hutchins, E. 1995. Cognition in the Wild. Cambridge, MA: MIT Press.
- Ilgen, D. R., J. R. Hollenbeck, M. Johnson, and D. Jundt. 2005. “Teams in Organizations: From Input-Process-Output Models to IMOI Models.” Annual Review of Psychology 56 (1): 517–543. doi:https://doi.org/10.1146/annurev.psych.56.091103.070250.
- Jenkins, D. P., P. M. Salmon, N. A. Stanton, and G. H. Walker. 2010. “A Systemic Approach to Accident Analysis: A Case Study of the Stockwell Shooting.” Ergonomics 53 (1): 1–17. doi:https://doi.org/10.1080/00140130903311625.
- Johnson, M., and A. Vera. 2019. “No AI is an Island: The Case for Teaming Intelligence.” AI Magazine 40 (1): 16–28. doi:https://doi.org/10.1609/aimag.v40i1.2842.
- Jokela, T., N. Iivari, J. Matero, and M. Karukka. 2003. “The Standard of User-Centered Design and the Standard Definition of Usability: Analyzing ISO 13407 against ISO 9241-11.” Paper presented at the Proceedings of The Latin American Conference on Human-Computer Interaction, Rio de Janeiro, Brazil, August 17–20.
- Kanki, B. G., J. Anca, and T. R. Chidester, eds. 2019. Crew Resource Management. Waltham, MA: Academic Press.
- Kaplan, A., and M. Haenlein. 2019. “Siri, Siri, in my Hand: Who’s the Fairest in the Land? On the Interpretations, Illustrations, and Implications of Artificial Intelligence.” Business Horizons 62 (1): 15–25. doi:https://doi.org/10.1016/j.bushor.2018.08.004.
- Kendall, D. L., and E. Salas. 2004. “Measuring Team Performance: Review of Current Methods and Consideration of Future Needs.” In The Science and Simulation of Human Performance, edited by J. W. Ness, V. Tepe, and D. R. Ritzer. 307–326. Bradford, UK: Emerald Group Publishing Limited.
- Kennedy, D. M., and S. A. McComb. 2014. “When Teams Shift among Processes: Insights from Simulation and Optimization.” The Journal of Applied Psychology 99 (5): 784–815. doi:https://doi.org/10.1037/a0037339.
- Kiekel, P. A., N. J. Cooke, P. W. Foltz, J. C. Gorman, and M. J. Martin. 2002. “Some Promising Results of Communication-Based Automatic Measures of Team Cognition.” Paper presented at the Human Factors and Ergonomics Society Annual Meeting, Baltimore, MD, September 29 to October 4.
- King, H. B., J. Battles, D. P. Baker, A. Alonso, E. Salas, J. Webster, Salisbury, M. 2008. “TeamSTEPPS™: Team Strategies and Tools to Enhance Performance and Patient Safety.” In Advances in Patient Safety: New Directions and Alternative Approaches, edited by K. Henriksen, J. B. Battles, and M. A. Keyes, Vol. 3. Rockville, MD: Agency for Healthcare Research and Quality.
- Klein, C., D. DiazGranados, E. Salas, H. Le, C. S. Burke, R. Lyons, and G. F. Goodwin. 2009. “Does Team Building Work?” Small Group Research 40 (2): 181–222. doi:https://doi.org/10.1177/1046496408328821.
- Klein, G., D. D. Woods, J. J. M. Bradshaw, R. R. Hoffman, and P. J. P. Feltovich. 2004. “Ten Challenges for Making Automation a “Team Player” in Joint Human-Agent Activity.” Human-Centered Computing 19: 91–95.
- Kozlowski, S. W. 2018. “Enhancing the Effectiveness of Work Groups and Teams: A Reflection.” Perspectives on Psychological Science : a Journal of the Association for Psychological Science 13 (2): 205–212. doi:https://doi.org/10.1177/1745691617697078.
- Kozlowski, S. W. J., and B. S. Bell. 2003. “Work Groups and Teams in Organizations.” In Handbook of Psychology: Industrial and Organizational Psychology, edited by W. C. Borman, D. R. Ilgen, and R. J. Klimoski, Vol. 12, 333–375. Hoboken, NJ: John Wiley & Sons Inc.
- Kozlowski, S. W. J., and G. T. Chao. 2018. “Unpacking Team Process Dynamics and Emergent Phenomena: Challenges, Conceptual Advances, and Innovative Methods.” American Psychologist 73 (4): 576–592. doi:https://doi.org/10.1037/amp0000245.
- Kozlowski, S. W. J., J. A. Grand, S. K. Baard, and M. Pearce. 2015. “Teams, Teamwork, and Team Effectiveness: Implications for Human Systems Integration.”. In APA Handbooks in Psychology®. APA Handbook of Human Systems Integration, edited by D. A. Boehm-Davis, F. T. Durso, and J. D. Lee, 555–571. Washington, DC: American Psychological Association.
- Kunze, A., S. J. Summerskill, R. Marshall, and A. J. Filtness. 2019. “Automation Transparency: Implications of Uncertainty Communication for Human-Automation Interaction and Interfaces.” Ergonomics 62 (3): 345–360. doi:https://doi.org/10.1080/00140139.2018.1547842.
- Lacerenza, C. N., S. L. Marlow, S. I. Tannenbaum, and E. Salas. 2018. “Team Development Interventions: Evidence-Based Approaches for Improving Teamwork.” American Psychologist 73 (4): 517–531. doi:https://doi.org/10.1037/amp0000295.
- Lacerenza, C. N., D. L. Reyes, S. L. Marlow, D. L. Joseph, and E. Salas. 2017. “Leadership Training Design, Delivery, and Implementation: A Meta-Analysis.” The Journal of Applied Psychology 102 (12): 1686–1718. doi:https://doi.org/10.1037/apl0000241.
- Langan-Fox, J., C. L. Cooper, and R. J. Klimoski, eds. 2007. Research Companion to the Dysfunctional Workplace: Management Challenges and Symptoms. Cheltenham, UK: Edward Elgar Publishing.
- LaVoie, N., P. Foltz, M. Rosenstein, R. Oberbreckling, R. Chatham, and J. Psotka. 2008. “Automated Support for AARs: Exploiting Communication to Assess Team Performance.” Paper presented at the Interservice/Industry Training, Simulation and Education Conference, Orlando, FL, December 1–5.
- Lee, J. D. 2019. “Trust and the Teleology of Technology.” Ergonomics 62 (4): 500–501. doi:https://doi.org/10.1080/00140139.2019.1563332.
- Lee, J. D., and K. A. See. 2004. “Trust in Automation: Designing for Appropriate Reliance.” Human Factors 46 (1): 50–80.
- LePine, J. A., R. F. Piccolo, C. L. Jackson, J. E. Mathieu, and J. R. Saul. 2008. “A Meta‐Analysis of Teamwork Processes: Tests of a Multidimensional Model and Relationships with Team Effectiveness Criteria.” Personnel Psychology 61 (2): 273–307. doi:https://doi.org/10.1111/j.1744-6570.2008.00114.x.
- Lisbon, D., D. Allin, C. Cleek, L. Roop, M. Brimacombe, C. Downes, and S. K. Pingleton. 2016. “Improved Knowledge, Attitudes, and Behaviours after Implementation of TeamSTEPPS Training in an Academic Emergency Department: A Pilot Report.” American Journal of Medical Quality 31 (1): 86–90. doi:https://doi.org/10.1177/1062860614545123.
- Lucia, B., M. A. Vetter, and O. Moroz. 2021. “The Rhetoric of Google Lens: A Postsymbolic Look at Locative Media.” Rhetoric Review 40 (1): 75–89. doi:https://doi.org/10.1080/07350198.2020.1841452.
- Malakis, S., T. Kontogiannis, and B. Kirwan. 2010. “Managing Emergencies and Abnormal Situations in Air Traffic Control (Part II): Teamwork Strategies.” Applied Ergonomics 41 (4): 628–635. doi:https://doi.org/10.1016/j.apergo.2009.12.018.
- Manser, T., S. Foster, R. Flin, and R. Patey. 2013. “Team Communication during Patient Handover from the Operating Room: More than Facts and Figures.” Human Factors 55 (1): 138–156. doi:https://doi.org/10.1177/0018720812451594.
- Marks, M. A., J. E. Mathieu, and S. J. Zaccaro. 2001. “A Temporally Based Framework and Taxonomy of Team Processes.” Academy of Management Review 26 (3): 356–376. doi:https://doi.org/10.5465/amr.2001.4845785.
- Marks, M. A., S. J. Zaccaro, and J. E. Mathieu. 2000. “Performance Implications of Leader Briefings and Team-Interaction Training for Team Adaptation to Novel Environments.” The Journal of Applied Psychology 85 (6): 971–986. doi:https://doi.org/10.1037/0021-9010.85.6.971.
- Marlow, S., T. Bisbey, C. Lacerenza, and E. Salas. 2018. “Performance Measures for Health Care Teams: A Review.” Small Group Research 49 (3): 306–356. doi:https://doi.org/10.1177/1046496417748196.
- Marlow, S. L., C. N. Lacerenza, J. Paoletti, C. S. Burke, and E. Salas. 2018. “Does Team Communication Represent a One-Size-Fits-All Approach?: A Meta-Analysis of Team Communication and Performance.” Organizational Behavior and Human Decision Processes 144: 145–170. doi:https://doi.org/10.1016/j.obhdp.2017.08.001.
- Martin, M. J., and P. W. Foltz. 2004. “Automated Team Discourse Annotation and Performance Prediction using LSA.” Paper presented at the Human Language Technologies and North American Association for Computational Linguistics annual meeting, Boston, MA, May 2–7.
- Mathieu, J. E., J. R. Hollenbeck, D. van Knippenberg, and D. R. Ilgen. 2017. “A Century of Work Teams in the Journal of Applied Psychology.” The Journal of Applied Psychology 102 (3): 452–467. doi:https://doi.org/10.1037/apl0000128.
- Mathieu, J., M. T. Maynard, T. Rapp, and L. Gilson. 2008. “Team Effectiveness 1997-2007: A Review of Recent Advancements and a Glimpse into the Future.” Journal of Management 34 (3): 410–476. doi:https://doi.org/10.1177/0149206308316061.
- Mayer, C. M., L. Cluff, W.-T. Lin, T. S. Willis, R. E. Stafford, C. Williams, R. Saunders, K. A. Short, N. Lenfestey, H. L. Kane, and J. B. Amoozegar. 2011. “Evaluating Efforts to Optimize TeamSTEPPS Implementation in Surgical and Pediatric Intensive Care Units.” Joint Commission Journal on Quality and Patient Safety 37 (8): 365–374. doi:https://doi.org/10.1016/S1553-7250. (11)37047-X
- McEwan, D., and M. R. Beauchamp. 2014. “Teamwork in Sport: A Theoretical and Integrative Review.” International Review of Sport and Exercise Psychology 7 (1): 229–250. doi:https://doi.org/10.1080/1750984X.2014.932423.
- McEwan, D., G. R. Ruissen, M. A. Eys, B. D. Zumbo, and M. R. Beauchamp. 2017. “The Effectiveness of Teamwork Training on Teamwork Behaviors and Team Performance: A Systematic Review and Meta-Analysis of Controlled Interventions.” PLoS One 12 (1): e0169604. doi:https://doi.org/10.1371/journal.pone.0169604.
- McGrath, J. E. 1964. Social Psychology: A Brief Introduction. New York, NY: Holt, Rinehart and Winston.
- McNeese, N. J., M. Demir, N. J. Cooke, and C. Myers. 2018. “Teaming with a Synthetic Teammate: Insights into Human-Autonomy Teaming.” Human Factors 60 (2): 262–273.
- Merritt, S. M., and D. R. Ilgen. 2008. “Not All Trust is Created Equal: Dispositional and History-Based Trust in Human-Automation Interactions.” Human Factors 50 (2): 194–210. doi:https://doi.org/10.1518/001872008X288574.
- Mishra, A., K. Catchpole, and P. McCulloch. 2009. “The Oxford NOTECHS System: Reliability and Validity of a Tool for Measuring Teamwork Behaviour in the Operating Theatre.” Quality & Safety in Health Care 18 (2): 104–108. doi:https://doi.org/10.1136/qshc.2007.024760.
- Muir, B. M., and N. Moray. 1996. “Trust in Automation. Part II. Experimental Studies of Trust and Human Intervention in a Process Control Simulation.” Ergonomics 39 (3): 429–460. doi:https://doi.org/10.1080/00140139608964474.
- Murphy, K. R., and J. I. Constans. 1987. “Behavioral Anchors as a Source of Bias in Rating.” Journal of Applied Psychology 72 (4): 573–577. doi:https://doi.org/10.1037/0021-9010.72.4.573.
- Myers, C. W., J. T. Ball, N. J. Cooke, M. D. Freiman, M. Caisse, S. M. Rodgers, M. Demir, and N. J. McNeese. 2019. “Autonomous Intelligent Agents for Team Training: Making the Case for Synthetic Teammates.” IEEE Intelligent Systems 34 (2): 3–14. doi:https://doi.org/10.1109/MIS.2018.2886670.
- Naikar, N. 2013. Work Domain Analysis: Concepts, Guidelines, and Cases. Boca Raton, FL: CRC Press.
- Neville, T. J., P. M. Salmon, and G. J. Read. 2018. “Radio Gaga? Intra-Team Communication of Australian Rules Football Umpires - Effect of Radio Communication on Content, Structure and Frequency.” Ergonomics 61 (2): 313–328. doi:https://doi.org/10.1080/00140139.2017.1353140.
- O’Connor, P., J. Campbell, J. Newon, J. Melton, E. Salas, and K. A. Wilson. 2008. “Crew Resource Management Training Effectiveness: A Meta-Analysis and Some Critical Needs.” The International Journal of Aviation Psychology 18 (4): 353–368. doi:https://doi.org/10.1080/10508410802347044.
- O’Dea, A., P. O’Connor, and I. Keogh. 2014. “A Meta-Analysis of the Effectiveness of Crew Resource Management Training in Acute Care Domains.” Postgraduate Medical Journal 90 (1070): 699–708. doi:https://doi.org/10.1136/postgradmedj-2014-132800.
- Ohland, M. W., M. L. Loughry, D. J. Woehr, L. G. Bullard, R. M. Felder, C. J. Finelli, R. A. Layton, H. R. Pomeranz, and D. G. Schmucker. 2012. “The Comprehensive Assessment of Team Member Effectiveness: Development of a Behaviorally Anchored Rating Scale for Self-and Peer Evaluation.” Academy of Management Learning & Education 11 (4): 609–630. doi:https://doi.org/10.5465/amle.2010.0177.
- Olguín, D. O., and A. Pentland. 2010. “Assessing Group Performance from Collective Behavior.” Paper presented at the 2010 ACM Conference on Computer Supported Cooperative Work, Savannah, GA, February 6–10.
- Onwochei, D. N., S. Halpern, and M. Balki. 2017. “Teamwork Assessment Tools in Obstetric Emergencies: A Systematic Review.” Simulation in Healthcare 12 (3): 165–176. doi:https://doi.org/10.1097/SIH.0000000000000210.
- Parasuraman, R., and V. Riley. 1997. “Humans and Automation: Use, Misuse, Disuse, Abuse.” Human Factors 39 (2): 230–253. doi:https://doi.org/10.1518/001872097778543886.
- Paris, C. R., E. Salas, and J. A. Cannon-Bowers. 2000. “Teamwork in Multi-Person Systems: A Review and Analysis.” Ergonomics 43 (8): 1052–1075.
- Pitardi, V., H. R. Marriott. 2021. “Alexa, She’s Not Human but… Unveiling the Drivers of Consumers’ Trust in Voice‐Based Artificial Intelligence.” Psychology & Marketing 38 (4): 626–642. doi:https://doi.org/10.1002/mar.21457.
- Rafferty, L. A., and N. A. Stanton. 2012. The Human Factors of Fratricide. 1st ed. London, UK: CRC Press.
- Rauschecker, A. M., J. D. Rudie, L. Xie, J. Wang, M. T. Duong, E. J. Botzolakis, A. M. Kovalovich, J. Egan, T. C. Cook, R. N. Bryan, I. M. Nasrallah, S. Mohan, and J. C. Gee. 2020. “Artificial Intelligence System Approaching Neuroradiologist-Level Differential Diagnosis Accuracy at Brain MRI.” Radiology 295 (3): 626–637. doi:https://doi.org/10.1148/radiol.2020190283.
- Roberts, A. P., and J. C. Cole. 2018. “Naturalistic Decision Making: Taking a (Cognitive) Step Back to Take Two Steps Forward in Understanding Experience-Based Decisions.” Journal of Applied Research in Memory and Cognition 7 (1): 70–81. doi:https://doi.org/10.1016/j.jarmac.2018.01.003.
- Roberts, A. P. J., and N. A. Stanton. 2018. “Macrocognition in Submarine Command and Control: A Comparison of Three Simulated Operational Scenarios.” Journal of Applied Research in Memory and Cognition 7 (1): 92–105. doi:https://doi.org/10.1016/j.jarmac.2018.01.006.
- Roberts, A. P., N. A. Stanton, K. L. Plant, D. T. Fay, and K. A. Pope. 2020. “You Say It is Physical, I Say It is Functional; Let us Call the Whole Thing off! Simulation: An Application Divided by Lack of Common Language.” Theoretical Issues in Ergonomics Science 21 (5): 507–536. doi:https://doi.org/10.1080/1463922X.2019.1683913.
- Rogers, W. C., S. L. Rogers, and G. Gregston. 1992. “Storm Center: The USS Vincennes and Iran Air Flight 655: A Personal Account of Tragedy and Terrorism.” Naval Inst Press.
- Rosen, M. A., A. S. Dietz, T. Yang, C. E. Priebe, and P. J. Pronovost. 2015. “An Integrative Framework for Sensor-Based Measurement of Teamwork in Healthcare.” Journal of the American Medical Informatics Association 22 (1): 11–18. doi:https://doi.org/10.1136/amiajnl-2013-002606.
- Rosen, M. A., N. Schiebel, E. Salas, T. S. Wu, S. Silvestri, and H. B. King. 2012. “How Can Team Performance Be Measured, Assessed, and Diagnosed.”. In Improving Patient Safety through Teamwork and Team Training, edited by E. Salas and K. Frush, 59–79. New York, NY: Oxford University Press.
- Rosenman, E. D., J. S. Ilgen, J. R. Shandro, A. L. Harper, and R. Fernandez. 2015. “A Systematic Review of Tools Used to Assess Team Leadership in Health Care Action Teams.” Academic Medicine 90 (10): 1408–1422. doi:https://doi.org/10.1097/ACM.0000000000000848.
- Rousseau, V., C. Aubé, and A. Savoie. 2006. “Teamwork Behaviors: A Review and Integration of Frameworks.” Small Group Research 37 (5): 540–570. doi:https://doi.org/10.1177/1046496406293125.
- Salas, E., N. Cooke, and M. A. Rosen. 2008. “On Teams, Teamwork, and Team Performance: Discoveries and Developments.” Human Factors 50 (3): 540–547. doi:https://doi.org/10.1518/001872008X288457.
- Salas, E., T. L. Dickinson, S. A. Converse, and S. Tannenbaum. 1992. “Toward an Understanding of Team Performance and Training.” In Teams: Their Training and Performance, edited by R. W. Swezey and E. Salas, 3–29. Westport, CT: Ablex Publishing.
- Salas, E. and S. M. Fiore, eds. 2004. Team Cognition: Understanding the Factors That Drive Process and Performance. Washington, DC: American Psychological Association.
- Salas, E., D. R. Nichols, and J. E. Driskell. 2007. “Testing Three Team Training Strategies in Intact Teams: A Meta-Analysis.” Small Group Research 38 (4): 471–488. doi:https://doi.org/10.1177/1046496407304332.
- Salas, E., D. L. Reyes, and S. H. McDaniel. 2018. “The Science of Teamwork: Progress, Reflections, and the Road Ahead.” American Psychologist 73 (4): 593–600. doi:https://doi.org/10.1037/amp0000334.
- Salas, E., D. L. Reyes, and A. L. Woods. 2017. “The Assessment of Team Performance: Observations and Needs.” In Innovative Assessment of Collaboration, edited by A. A. von Davier, M. Zhu, and P. C. Kyllonen, 21–36. Cham, Switzerland: Springer.
- Salas, E., M. A. Rosen, C. S. Burke, and G. F. Goodwin. 2009. “The Wisdom of Collectiveness in Organizations: An Update of Competencies.” In Team Effectiveness in Complex Organizations: Cross-Disciplinary Perspectives and Approaches. London, UK: Routledge.
- Salas, E., M. A. Rosen, J. D. Held, and J. J. Weissmuller. 2009. “Performance Measurement in Simulation-Based Training: A Review and Best Practices.” Simulation & Gaming 40 (3): 328–376. doi:https://doi.org/10.1177/1046878108326734.
- Salas, E., M. A. Rosen, and H. King. 2007. “Managing Teams Managing Crises: Principles of Teamwork to Improve Patient Safety in the Emergency Room and Beyond.” Theoretical Issues in Ergonomics Science 8 (5): 381–394. doi:https://doi.org/10.1080/14639220701317764.
- Salas, E., D. E. Sims, and C. S. Burke. 2005. “Is There a “Big Five” in Teamwork?” Small Group Research 36 (5): 555–599. doi:https://doi.org/10.1177/1046496405277134.
- Salas, E., K. A. Wilson, C. S. Burke, and D. C. Wightman. 2006. “Does Crew Resource Management Training Work? An Update, an Extension, and Some Critical Needs.” Human Factors 48 (2): 392–412. doi:https://doi.org/10.1518/001872006777724444.
- Salas, E., S. Zajac, and S. L. Marlow. 2018. “Transforming Health Care One Team at a Time: Ten Observations and the Trail Ahead.” Group & Organization Management 43 (3): 357–381. doi:https://doi.org/10.1177/1059601118756554.
- Salmon, P. M., T. Carden, and N. J. Stevens. 2018. “Breaking Bad Systems: Using Work Domain Analysis to Identify Strategies for Disrupting Terrorist Cells.” Proceedings of Ergonomics and Human Factors; Birmingham, UK.
- Salmon, P. M., A. Clacy, and C. Dallat. 2017. “It’s Not All about the Bike: Distributed Situation Awareness and Teamwork in Elite Women’s Cycling Teams.” Proceedings from Contemporary Ergonomics & Human Factors 2017: 240–248.
- Salmon, P. M., G. H. Walker, and N. A. Stanton. 2016. “Pilot Error versus Sociotechnical Systems Failure: A Distributed Situation Awareness Analysis of Air France 447.” Theoretical Issues in Ergonomics Science 17 (1): 64–79. doi:https://doi.org/10.1080/1463922X.2015.1106618.
- Salwei, M. E., P. Carayon, A. S. Hundt, P. Hoonakker, V. Agrawal, P. Kleinschmidt, J. Stamm, D. Wiegmann, and B. W. Patterson. 2019. “Role Network Measures to Assess Healthcare Team Adaptation to Complex Situations: The Case of Venous Thromboembolism Prophylaxis.” Ergonomics 62 (7): 864–816. doi:https://doi.org/10.1080/00140139.2019.1603402.
- Schmutz, J. B., L. L. Meier, and T. Manser. 2019. “How Effective is Teamwork Really? The Relationship between Teamwork and Performance in Healthcare Teams: A Systematic Review and Meta-Analysis.” BMJ Open 9 (9): e028280. doi:https://doi.org/10.1136/bmjopen-2018-028280.
- Seeber, I., E. Bittner, R. O. Briggs, T. de Vreede, G.-J. de Vreede, A. Elkins, R. Maier, A. B. Merz, S. Oeste-Reiß, N. Randrup, G. Schwabe, and M. Söllner. 2020. “Machines as Teammates: A Research Agenda on AI in Team Collaboration.” Information & Management 57 (2): 103174. doi:https://doi.org/10.1016/j.im.2019.103174.
- Shneiderman, B. 2020. “Human-Centered Artificial Intelligence: Reliable, Safe & Trustworthy.” International Journal of Human–Computer Interaction 36 (6): 495–504. doi:https://doi.org/10.1080/10447318.2020.1741118.
- Shoemaker, S. J., M. L. Parchman, K. K. Fuda, J. Schaefer, J. Levin, M. Hunt, and R. Ricciardi. 2016. “A Review of Instruments to Measure Interprofessional Team-Based Primary Care.” Journal of Interprofessional Care 30 (4): 423–432. doi:https://doi.org/10.3109/13561820.2016.1154023.
- Shuffler, M. L., D. Diazgranados, M. T. Maynard, and E. Salas. 2018. “Developing, Sustaining, and Maximizing Team Effectiveness: An Integrative, Dynamic Perspective of Team Development Interventions.” Academy of Management Annals 12 (2): 688–724. doi:https://doi.org/10.5465/annals.2016.0045.
- Shuffler, M. L., D. DiazGranados, and E. Salas. 2011. “There’s a Science for That: Team Development Interventions in Organizations.” Current Directions in Psychological Science 20 (6): 365–372. doi:https://doi.org/10.1177/0963721411422054.
- Smith-Jentsch, K. A., J. A. Cannon-Bowers, S. I. Tannenbaum, and E. Salas. 2008. “Guided Team Self-Correction: Impacts on Team Mental Models, Processes, and Effectiveness.” Small Group Research 39 (3): 303–327. doi:https://doi.org/10.1177/1046496408317794.
- Smith-Jentsch, K. A., R. L. Zeisig, B. Acton, and J. A. McPherson. 1998. “Team Dimensional Training: A Strategy for Guided Team Self-Correction.” In Making Decisions under Stress: Implications for Individual and Team Training, edited by J. A. Cannon-Bowers and E. Salas, 271–297. Washington, DC: American Psychological Association.
- Snook, S. A. 2000. Friendly Fire: The Accidental Shootdown of U.S. Black Hawk Helicopters over Norther Iraq. Woodstock, UK: Princeton University Press.
- Stanton, N. A. 2006. “Hierarchical Task Analysis: Developments, Applications, and Extensions.” Applied Ergonomics 37 (1): 55–79. doi:https://doi.org/10.1016/j.apergo.2005.06.003.
- Stanton, N. A. 2014. “Representing Distributed Cognition in Complex Systems: How a Submarine Returns to Periscope Depth.” Ergonomics 57 (3): 403–418. doi:https://doi.org/10.1080/00140139.2013.772244.
- Stanton, N. A., and M. J. Ashleigh. 2000. “A Field Study of Team Working in a New Human Supervisory Control System.” Ergonomics 43 (8): 1190–1209. doi:https://doi.org/10.1080/00140130050084941.
- Stanton, N. A., K. L. Plant, K. M. Revell, T. G. Griffin, S. Moffat, and M. Stanton. 2019. “Distributed Cognition in Aviation Operations: A Gate-to-Gate Study with Implications for Distributed Crewing.” Ergonomics 62 (2): 138–155. doi:https://doi.org/10.1080/00140139.2018.1520917.
- Stanton, N. A., K. L. Plant, A. P. Roberts, and C. K. Allison. 2019. “Use of Highways in the Sky and a Virtual Pad for Landing Head up Display Symbology to Enable Improved Helicopter Pilots Situation Awareness and Workload in Degraded Visual Conditions.” Ergonomics 62 (2): 255–267. doi:https://doi.org/10.1080/00140139.2017.1414301.
- Stanton, N. A., L. A. Rafferty, P. M. Salmon, K. M. A. Revell, R. McMaster, A. Caird-Daley, and C. Cooper-Chapman. 2010. “Distributed Decision Making in Multi-Helicopter Teams: Case Study of Mission Planning and Execution from a Non-Combatant Evacuation Operation Training Scenario.” Journal of Cognitive Engineering and Decision Making 4 (4): 328–353. doi:https://doi.org/10.1177/155534341000400405.
- Stanton, N. A., and A. P. Roberts. 2020. “Better Together? Investigating New Control Room Configurations and Reduced Crew Size in Submarine Command and Control.” Ergonomics 63 (3): 307–323. doi:https://doi.org/10.1080/00140139.2019.1654137.
- Stanton, N. A., P. M. Salmon, and G. H. Walker. 2019. Systems Thinking in Practice: Applications of the Event Analysis of Systemic Teamwork Method. Boca Raton, FL: CRC Press.
- Stanton, N. A., P. M. Salmon, G. H. Walker, E. Salas, and P. A. Hancock. 2017. “State-of-Science: Situation Awareness in Individuals, Teams and Systems.” Ergonomics 60 (4): 449–466. doi:https://doi.org/10.1080/00140139.2017.1278796.
- Stanton, N. A., R. Stewart, D. Harris, R. J. Houghton, C. Baber, R. McMaster, P. Salmon, G. Hoyle, G. Walker, M. S. Young, M. Linsell, R. Dymott, and D. Green. 2006. “Distributed Situation Awareness in Dynamic Systems: Theoretical Development and Application of an Ergonomics Methodology.” Ergonomics 49 (12–13): 1288–1311. doi:https://doi.org/10.1080/00140130600612762.
- Stanton, N. A., G. H. Walker, and L. J. Sorensen. 2012. “It’s a Small World after All: Contrasting Hierarchical and Edge Networks in a Simulated Intelligence Analysis Task.” Ergonomics 55 (3): 265–281. doi:https://doi.org/10.1080/00140139.2011.642006.
- Sullenberger, C. B., and J. Zaslow. 2009. Highest Duty: My Search for What Really Matters. New York, NY: Harper Collins.
- Svensson, J., and J. Andersson. 2006. “Speech Acts, Communication Problems, and Fighter Pilot Team Performance.” Ergonomics 49 (12–13): 1226–1237. doi:https://doi.org/10.1080/00140130600612671.
- Tannenbaum, S. I., and C. P. Cerasoli. 2013. “Do Team and Individual Debriefs Enhance Performance? A Meta-Analysis.” Human Factors 55 (1): 231–245. doi:https://doi.org/10.1177/0018720812448394.
- Tannenbaum, S. I., E. Salas, and J. A. Cannon-Bowers. 1996. “Promoting Team Effectiveness.” In Handbook of Work Group Psychology, edited by M. A. West, 503–529. Hoboken, NJ: Wiley-Blackwell.
- Thomas, E. J., J. B. Sexton, and R. L. Helmreich. 2004. “Translating Teamwork Behaviours from Aviation to Healthcare: Development of Behavioural Markers for Neonatal Resuscitation.” Quality and Safety in Health Care 13 (1): i57–i64. doi:https://doi.org/10.1136/qshc.2004.009811.
- Trotter, M., P. M. Salmon, and M. G. Lenne. 2014. “Impromaps: Applying Rasmussen’s Risk Management Framework to Improvisation Incidents.” Safety Science 64: 60–70. doi:https://doi.org/10.1016/j.ssci.2013.11.021.
- Valentine, M. A., I. M. Nembhard, and A. C. Edmondson. 2015. “Measuring Teamwork in Health Care Settings: A Review of Survey Instruments.” Medical Care 53 (4): e16–e30. doi:https://doi.org/10.1097/MLR.0b013e31827feef6.
- Volpe, C. E., J. A. Cannon-Bowers, E. Salas, and P. E. Spector. 1996. “The Impact of Cross-Training on Team Functioning: An Empirical Investigation.” Human Factors 38 (1): 87–100. doi:https://doi.org/10.1518/001872096778940741.
- Walker, G. H., H. Gibson, N. A. Stanton, C. Baber, P. Salmon, and D. Green. 2006. “Event Analysis of Systemic Teamwork (EAST): A Novel Integration of Ergonomics Methods to Analyse C4i Activity.” Ergonomics 49 (12–13): 1345–1369. doi:https://doi.org/10.1080/00140130600612846.
- Walker, G. H., P. M. Salmon, M. Bedinger, and N. A. Stanton. 2017. “Quantum Ergonomics: Shifting the Paradigm of the Systems Agenda.” Ergonomics 60 (2): 157–166. doi:https://doi.org/10.1080/00140139.2016.1231840.
- Wasserman, S., and K. Faust. 1994. Social Network Analysis: Methods and Applications. Vol. 8. Cambridge, UK: Cambridge University Press.
- Waterson, P., M. M. Robertson, N. J. Cooke, L. Militello, E. Roth, and N. A. Stanton. 2015. “Defining the Methodological Challenges and Opportunities for an Effective Science of Sociotechnical Systems and Safety.” Ergonomics 58 (4): 565–599. doi:https://doi.org/10.1080/00140139.2015.1015622.
- Weaver, S. J., S. M. Dy, and M. A. Rosen. 2014. “Team-Training in Healthcare: A Narrative Synthesis of the Literature.” BMJ Quality & Safety 23 (5): 359–372. doi:https://doi.org/10.1136/bmjqs-2013-001848.
- Weaver, S. J., M. A. Rosen, E. Salas, K. D. Baum, and H. B. King. 2010. “Integrating the Science of Team Training: Guidelines for Continuing Education.” The Journal of Continuing Education in the Health Professions 30 (4): 208–220. doi:https://doi.org/10.1002/chp.20085.
- Weingart, S. N., O. Yaghi, M. Wetherell, and M. Sweeney. 2018. “Measuring Medical Housestaff Teamwork Performance Using Multiple Direct Observation Instruments: Comparing Apples and Apples.” Academic Medicine 93 (7): 1064–1070. doi:https://doi.org/10.1097/ACM.0000000000002238.
- Weld, L. R., M. T. Stringer, J. S. Ebertowski, T. S. Baumgartner, M. C. Kasprenski, J. C. Kelley, D. S. Cho, E. A. Tieva, and T. E. Novak. 2016. “TeamSTEPPS Improves Operating Room Efficiency and Patient Safety.” American Journal of Medical Quality 31 (5): 408–414. doi:https://doi.org/10.1177/1062860615583671.
- Whittaker, G., H. Abboudi, M. S. Khan, P. Dasgupta, and K. Ahmed. 2015. “Teamwork Assessment Tools in Modern Surgical Practice: A Systematic Review.” Surgery Research and Practice 2015: 494827. doi:https://doi.org/10.1155/2015/494827.
- Wood, T. C., N. Raison, S. Haldar, O. Brunckhorst, C. McIlhenny, P. Dasgupta, and K. Ahmed. 2017. “Training Tools for Nontechnical Skills for Surgeons—a Systematic Review.” Journal of Surgical Education 74 (4): 548–578. doi:https://doi.org/10.1016/j.jsurg.2016.11.017.
- Yule, S., R. Flin, S. Paterson-Brown, and N. Maran. 2006. “Non-Technical Skills for Surgeons in the Operating Room: A Review of the Literature.” Surgery 139 (2): 140–149. doi:https://doi.org/10.1016/j.surg.2005.06.017.
- Zhang, Y., J. Olenick, C. H. Chang, S. W. Kozlowski, and H. Hung. 2018. “TeamSense: Assessing Personal Affect and Group Cohesion in Small Teams through Dyadic Interaction and Behavior Analysis with Wearable Sensors.” Proceedings of the ACM on Interactive, Mobile, Wearable and Ubiquitous Technologies 2 (3): 1–22. doi:https://doi.org/10.1145/3264960.