Abstract
Besides radically altering work, advances in automation and intelligent technologies have the potential to bring significant societal transformation. These transitional periods require an approach to analysis and design that goes beyond human-machine interaction in the workplace to consider the wider sociotechnical needs of envisioned work systems. The Sociotechnical Influences Space, an analytical tool motivated by Rasmussen’s risk management model, promotes a holistic approach to the design of future systems, attending to societal needs and challenges, while still recognising the bottom-up push from emerging technologies. A study explores the concept and practical potential of the tool when applied to the analysis of a large-scale, ‘real-world’ problem, specifically the societal, governmental, regulatory, organisational, human, and technological factors of significance in mixed human-artificial agent workforces. Further research is needed to establish the feasibility of the tool in a range of application domains, the details of the method, and the value of the tool in design.
Practitioner summary: Emerging automation and intelligent technologies are not only transforming workplaces, but may be harbingers of major societal change. A new analytical tool, the Sociotechnical Influences Space, is proposed to support organisations in taking a holistic approach to the incorporation of advanced technologies into workplaces and function allocation in mixed human-artificial agent teams.
Introduction
Advances in automation and intelligent technologies are not only changing the nature of human work (Drury Citation2008; Moray Citation2000), but have the potential to bring about significant societal transformation (Dolata Citation2008, Citation2013). Driverless cars, for example, are expected to become a commonality on our roads in the future and can bring marked changes to our technological, social, and political structures (Bissell et al. Citation2020; Brady Citation2019; Hancock Citation2019). In addition, emerging technologies in defence, healthcare, education, finance, law, media, and the service sectors have the potential to bring widespread change (e.g. Leahy, Holland, and Ward Citation2019; Shaw and Chisholm Citation2020; Wirtz, Weyerer, and Geyer Citation2019).
During periods of major technological innovation, a top-down approach to the design of envisioned work systems is needed. The focus of design must expand beyond considerations relating solely to human interaction with machines in the workplace to one that encompasses wider considerations for people, organisations, and society. A holistic perspective is needed to ensure the transformation of people’s work and lives occurs on the basis of real needs or informed choices and desires, rather than being driven solely or predominantly by the bottom-up push from emerging technologies.
The need to take a broader perspective in human factors and ergonomics, or holistic or systems approach, is widely recognised in the literature (Bentley et al. Citation2021; Carayon Citation2006; Carayon et al. Citation2015; Dekker, Hancock, and Wilkin Citation2013; Hancock Citation2019; Hollnagel Citation2011; Moray Citation2000; Thatcher et al. Citation2018; Vicente Citation2004). However, contributions in this area are still largely theoretical and lack significant practical application (Carayon et al. Citation2015), perhaps because of funding and time constraints or the perception that broad-ranging problems exceed the competencies of practitioners (Thatcher et al. Citation2018; Wilson Citation2014). Whatever the reasons for this paucity, social orders and political and economic systems and activities are intimately related to overall quality of life, and generate problems addressed by human factors and ergonomics (Wilkin Citation2010), suggesting the need for a more holistic approach in design.
When considering the large-scale incorporation of advanced automation and intelligent technologies into predominantly human work systems, wide-ranging matters, such as those relating to wealth creation, privacy, security, responsibility, discrimination, and boredom among others (e.g. Floridi et al. Citation2018; Streitz Citation2019; Torresen Citation2018), must not be ignored in workplace design because of the potential consequences for workers and transformative effects for people and society. The capacity of such technologies to generate public benefit and economic value, and to provide opportunities for freeing up time for humans, are expected to act as significant drivers for their uptake (e.g. Cave and ÓhÉigeartaigh Citation2018; Russell, Dewey, and Tegmark Citation2015). Yet, it is argued, and generally well accepted, that people derive meaning, dignity, and purpose from their work (e.g. Floridi et al. Citation2018; Leveringhaus Citation2018), an observation that gains greater poignancy as artificial intelligence becomes more capable. Further, the risks of reduced human control and engagement (e.g. Dignum Citation2017; Streitz et al. Citation2019), such as the diminished ability of humans to prevent or rectify errors or the gradual degradation of human expertise to the point where the adoption of intelligent or automated technologies becomes a foregone conclusion, and our reliance on technology becomes a significant vulnerability, must also be considered.
Ultimately, decisions about such factors can shape the world we live in, gradually building to form the societal response to such global questions as what kind of culture we want to perpetuate or foster, who can or will bear responsibility for errors or adverse occurrences, and what are the consequences for the competencies and occupations of humans. For this reason, higher-level social, governmental, and regulatory concerns—external to organisations—must not be seen simply as providing the context for mixed human-technology work systems but must be explored as an integral part of system design (see also Thatcher et al. Citation2018). Further, while the sources of these concerns may be viewed as falling beyond the purview of the workplaces in consideration, and outside of their apparent control or influence, organisations are embedded in a wider sociotechnical system and must be responsive to public, political, and legal concerns in order to remain viable or effective.
Although cultural, social, psychological, physical, and technological concerns have not been neglected in the development and exploration of artificial technologies (e.g. Acemoglu and Restrepo Citation2018; Hoffman Citation2017; Misselhorn Citation2018; Theodorou and Dignum Citation2020), different factors tend to be studied by scholars in distinct fields or disciplines. For instance, human factors researchers have generally focussed on concerns relating to human performance, competencies, and trust (see also Bentley et al. Citation2021); philosophers and ethicists have tended to examine ethical or moral dilemmas; and economists have concentrated for the most part on productivity or monetary trends. Such focussed efforts are necessary to foster in-depth examination of the relevant issues. However, breaking problem spaces into parts and studying them in isolation risks overlooking important interactions between factors that fall within the scopes of different disciplines or that cannot be deduced solely by examining components of the system separately. Therefore, in designing complex work systems, it is also important to take account of the aggregation of factors, and the dynamic interrelationships between them, so that their integrated implications for workplace outcomes can be considered.
The design of envisioned systems poses special challenges for analysts and designers. Described as the ‘envisioned world problem’ by Woods and Dekker (Citation2000) in the context of their studies of future air traffic management, a key challenge relates to suitable methods for analysis and design, given that the sociotechnical system does not yet exist in the envisioned form and therefore cannot be easily observed. Woods and Dekker rely on descriptive methods, whereby specific incidents are selected for study and then users’ behaviours and work practices with novel technologies explored in this context. Such studies are definitely useful and needed in the exploration of envisioned worlds, but for a number of reasons they are not well suited to the current problem of large-scale incorporation of advanced automation and intelligent technologies into predominantly human work systems.
First, the method is better suited to cases where a specific technology proposal is under consideration and the details of the technological innovation reasonably well defined, rather than to cases where numerous, wide-ranging technological possibilities are still emerging and are largely unspecified. Moreover, it is important to recognise that behaviours and work practices that may emerge in the envisioned world with people’s continuing use of, and increasing familiarity with, technologies over time cannot be easily comprehended upfront. It is also well established that technologies intended to support people’s current or observed behaviours generally lead to changes in those behaviours once those technologies are introduced into the work setting in a never-ending task-artifact cycle (Carroll and Rosson Citation1992).
Another challenge is that predicting situations of use is difficult, as Woods and Dekker (Citation2000) acknowledge. This problem is compounded by the fact that, in complex sociotechnical systems, one cannot hope to predict all of the situations of use. That is, even if many ‘credible’ situations can be defined and explored, actors in the system will still be confronted with novel events, or events that have not been—and cannot be—anticipated by analysts or designers, and therefore by definition cannot be observed or studied ahead of time (Rasmussen, Pejtersen, and Goodstein Citation1994; Vicente Citation1999). Moreover, novel or unforeseen situations are widely recognised as posing the greatest threats to system performance and safety (Perrow Citation1984; Rasmussen Citation1969; Reason Citation1990; Vicente Citation1999). For these reasons, preparing systems to deal with not only ongoing change, but also novel situations is a significant challenge.
Cognitive work analysis (Rasmussen, Pejtersen, and Goodstein Citation1994; Vicente Citation1999) offers an alternative approach to the envisioned world problem (Naikar et al. Citation2003), which focuses on the boundaries of successful operation as a key point of reference. These boundaries are limits on behaviour that must be respected by actors for successful performance, regardless of the circumstances or situations of use, but within these constraints, actors still have many possibilities for behaviour. The idea therefore is that by basing designs on these fundamental boundaries or constraints on action, actors can be supported in adapting their behaviours to a wide range of situations, including novel or unforeseen events, without jeopardising system productivity or safety. Such a design approach also allows the human-machine system to evolve over time, within certain boundaries (Naikar et al. Citation2021).
The value of cognitive work analysis for design has been demonstrated by a large body of experimental studies (Vicente Citation2002) and several industrial case studies (Naikar Citation2013). In addition, cognitive work analysis has previously been applied to envisioned world problems (e.g. Bisantz et al. Citation2003; Elix and Naikar Citation2021; Militello et al. Citation2019; Naikar and Sanderson Citation2001), with tangible impact on practice in industrial cases. However, in all of these applications, specific technological innovations were under consideration, and their consequences within the workplace were relatively straightforward. Furthermore, there was no clear need to consider consequences beyond the workplace explicitly, as the resulting designs did not have revolutionary implications for organisational practices or society more generally. For example, in one case, cognitive work analysis was used to develop a team design for a first-of-a-kind military aircraft (Naikar et al. Citation2003), but, as the focus was on human crews, there were no radical implications for broader societal matters such as ethical frameworks, education curricula, or regulatory frameworks setting industry standards, which become more pressing when designing teams or workforces with humans and artificial intelligence, especially on a large scale.
In principle, cognitive work analysis may be applied to design questions spanning from the workplace to social issues. However, in cases where there is potential for significant transformation at the level of frontline workers, the organisation, and beyond, a means for conceptualising the wider sociotechnical problem would also be useful for supporting the transformation of the workplace in a way that takes account of the wider consequences or challenges, particularly in large-scale endeavours where the technological and social possibilities are wide-ranging, emerging, and unspecified.
In this paper, we present an analytical tool for modelling multi-level sociotechnical factors that must be considered in designing envisioned work systems during periods of rapid technological and social change (Brady and Naikar Citation2020). We explain how the proposed tool, the Sociotechnical Influences Space, is motivated by Rasmussen’s (Citation1997) model of risk management, and we discuss the key features of this tool, its similarities and differences with the AcciMap method, and its construction and potential applications in the context of a proof of concept study. We use the term proof of concept to mean an exploration of the concept and practical potential of the tool when applied to the analysis of a large-scale, ‘real-world’ problem, a stage of concept or method development distinct from analytical or empirical evaluations, which are intended to assess the value of the resulting analysis for design (Elix and Naikar Citation2021; Rouse Citation1991; Vicente Citation2002). The system selected for this study is the Royal Australian Air Force’s future workforce, although we stress that this is only a hypothetical study in that the organisation has not yet made a decision to replace its current workforce design with one incorporating human-artificial agent work systems on a large-scale in the future.
Sociotechnical frameworks
Many methods and tools exist for the analysis, design, and evaluation of sociotechnical systems (for reviews, see Carayon et al. Citation2015; Stanton et al. Citation2013; Waterson et al. Citation2015). Generally, these techniques are regarded as sociotechnical in orientation in that they are concerned with the coupling of people with technology or, in other words, with the interaction of technological and social subsystems. However, the majority of approaches by far limit their focus to the workplace. This narrow span of view means that needs and goals beyond the workplace are largely neglected (Carayon et al. Citation2015), such that the viability and consequences of designs within the wider societal system in which workplaces are embedded are insufficiently considered. In addition, inadequate attention is given to interactions across levels of analysis (Waterson et al. Citation2015), thus risking oversight of important or emergent relationships. Existing methods and tools therefore provide a limited basis for the analysis, design, and evaluation of sociotechnical systems, especially during periods of major social and technological change.
Two widely recognised and relatively mature frameworks that do place significant emphasis on sociotechnical factors beyond the workplace are Rasmussen’s (Citation1997) risk management model and Leveson’s (Citation2004) systems-theoretic accident model and processes (STAMP) method. Both frameworks encompass multiple sociotechnical system levels in considering the possible causes of accidents or control failures involved in accidents. Given this focus, the two frameworks are commonly associated with the analysis and design of existing systems and, more specifically, with safety outcomes in those systems.
Newer frameworks for forecasting risks and accidents include the networked hazard analysis and risk management system (Dallat, Salmon, and Goode Citation2018), which combines hierarchical task analysis with systematic human error reduction and prediction, and the event analysis of systemic teamwork broken-links approach (Stanton and Harvey Citation2017). These frameworks are concerned with identifying foreseeable risks in existing systems, rather than accounting for unpredictable contingencies in future systems.
A more recent development that considers multiple sociotechnical system levels in the design of future systems is the systems-theoretic early concept analysis (STECA) method (Fleming and Leveson Citation2016), which involves performing hazard analysis on a concept of operations. Like its precursors, namely STAMP (Leveson Citation2004) and the risk management model (Rasmussen Citation1997), STECA focuses on safety outcomes. In addition, STECA seems to assume a relatively stable system, where the goals and control actions required for safely achieving those goals can be well defined or specified ahead of the system being put into operation. If we accept that the goals of actors can only be conceived in the context of specific situations, or classes of situation (Rasmussen, Pejtersen, and Goodstein Citation1994; Vicente Citation1999), then it is unclear to what extent the method is robust in the event that the system is faced with novel or unforeseen situations.
The need to build systems with the adaptive capacity to contend with contingencies that have not been, and cannot be, anticipated by analysts or designers was recognised by Rasmussen and his colleagues as the fundamental objective of design, most clearly within the cognitive work analysis framework (Rasmussen Citation1986; Rasmussen, Pejtersen, and Goodstein Citation1994). In relation to risk or safety management, tools within this framework, such as the abstraction hierarchy, were seen primarily as a means for developing information systems and visualisations for actors who, through their normal activities, could shape the flow of events in accident scenarios. Specifically, the intent was to make visible their local boundaries of acceptable performance and the side effects of their decisions on other actors through the design of a cooperative work system, so that they could adapt their behaviours to unfolding events, which may be unanticipated, without compromising system performance and safety (Rasmussen and Svedung Citation2000). In this context, the AcciMap method provides a basis for identifying the set of interacting actors associated with the causal factors of accidents across a range of scenarios, and cognitive work analysis is fundamental to a strategy for proactive risk management in a dynamic society.
Although Rasmussen and his colleagues (Rasmussen Citation1986; Rasmussen, Pejtersen, and Goodstein Citation1994) conceived cognitive work analysis primarily as a basis for developing designs to support actors in existing systems, the value of this framework for designing envisioned work systems has since been demonstrated. However, as discussed earlier in this paper, prior studies were concerned with workplace design problems that could be narrowly bounded, such as design of the training systems or crewing concepts for first-of-a-kind military aircraft (Elix and Naikar Citation2021; Naikar et al. Citation2003). To support organisational transformation during periods of major technological and social change, an analysis of the wider sociotechnical problem would also be helpful, promoting consideration of factors both within and beyond the workplace with the potential to affect or influence a broad range of outcomes, not just safety, in the envisioned system.
In this paper, we focus on Rasmussen’s (Citation1997) risk management framework as the fundamental building block for a proposed approach for analysing multi-level sociotechnical factors in the design of envisioned work systems, namely the Sociotechnical Influences Space. Rasmussen was the first to propose the integration of sociotechnical considerations beyond the workplace in the analysis and design of complex systems (Carayon et al. Citation2015), and his framework has since served as a foundational basis for contemporary analysis tools. In addition, we see the lack of taxonomies for guiding analysis and modelling in Rasmussen’s framework (Salmon, Cornelissen, and Trotter Citation2012) as a strength of this approach, as even generic taxonomies, such as those incorporated in STAMP, could serve to constrain or limit consideration of needs and desires in the design of envisioned systems within prescribed or artificial boundaries.
Risk management framework
Rasmussen’s (Citation1997) approach to risk management was motivated by the recognition that the high degree of integration and coupling of systems in contemporary society had made it “increasingly difficult to explain accident causation by analysis of local factors within a work system” (Svedung and Rasmussen Citation2002, 398). Rather, the causes of accidents were to be found at multiple levels of the sociotechnical system, and not just at the level of frontline operators. Consequently, safety and risk management efforts must consider not just the hazardous work processes of the system under consideration, but also actions taken at different sociotechnical system levels that can cause an accidental flow of events ().
Figure 1. Rasmussen’s (Citation1997) model of risk management. Adapted from Rasmussen (1997) with permission from Elsevier.
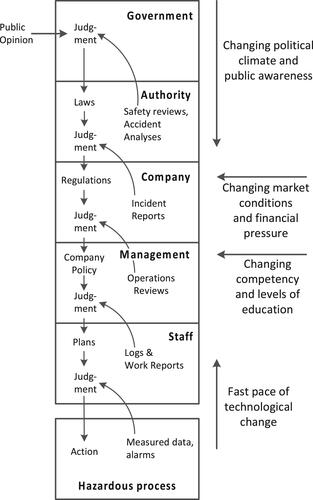
Rasmussen (Citation1997) further advocated a proactive approach to risk management in which safety interventions are not based solely on past accidents or incidents, as he recognised that in a rapidly changing or evolving society, confluences of new kinds of events or circumstances may form the basis of novel sorts of accidents. Rather, risk management should be based on the identification of decision makers at all levels of the sociotechnical system who exert control over hazardous work processes through their normal activities, and on supporting the communication flow between these decision makers so that any potentially dangerous side effects of their activities, or the potential causes of accidents, can be recognised and managed, as they arise, through continuing adaptation to the evolving circumstances.
For proactive risk management then the risk management model in is used in a series of graphical notations or ‘maps’ (Rasmussen and Svedung Citation2000; Svedung and Rasmussen Citation2002) as the underlying basis for modelling the following: (1) the conditions, actions, and decisions shaping the flow of events in an actual accident (basic AcciMap); (2) the general conditions, actions, and decisions shaping the flow of events across a representative set of accidents (generic AcciMap, which is produced by aggregating a number of basic AcciMaps); (3) the organisational bodies and individual actors who, through their normal activities, could shape the flow of events in the plausible accident scenarios (Actor Map); and (4) the information flow among these decision makers during their normal work (InfoFlow Map). The key objective is to design information systems to make decision makers at different sociotechnical system levels aware of any potentially hazardous side effects of their activities, in view of the objectives, values, and constraints of other decision makers, and thus facilitate the communication flow necessary among these decision makers for preventing accidents. presents a basic AcciMap for the transport of dangerous goods (Svedung and Rasmussen Citation2002) to illustrate how the risk management model () underpins the graphical notations for proactive safety control.
Figure 2. Basic AcciMap for an accident scenario involving the transport of hazardous goods. Reproduced from Svedung and Rasmussen (Citation2002) with permission from Elsevier.
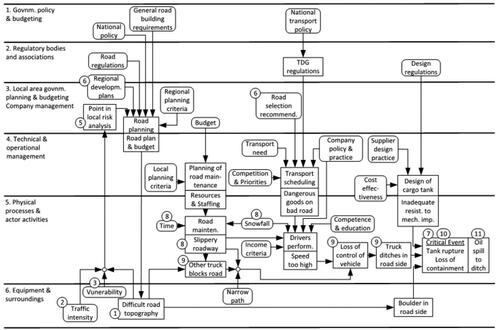
Although Rasmussen (Citation1997) advocated a proactive strategy for risk management, his model has been utilised primarily as a basis for conventional accident or incident analysis (Branford, Naikar, and Hopkins Citation2009). Specifically, the basic AcciMap has been used either for explaining how past accidents have eventuated or as a system for reporting the details of adverse events as they occur (see Waterson et al. (Citation2017) for a review). Likewise, variations of the AcciMap approach have focussed predominantly on the analysis of specific accident or incident sequences (Trotter, Salmon, and Lenné Citation2014).
Sociotechnical influences space
The sociotechnical influences space (SIS) is motivated by Rasmussen’s (Citation1997) risk management model, particularly in that it recognises the importance of multiple sociotechnical system levels in shaping workplace outcomes. However, in contrast to common perceptions and applications of the risk management model, specifically those associated with the AcciMap method, it is proposed that, in the design of envisioned work systems, the sociotechnical landscape in Rasmussen’s model need not be, or should not be, construed narrowly as simply one “in which accidents may unfold” (Svedung and Rasmussen Citation2002, 398), or as a field of risks. Rather, it may be viewed more completely as a landscape in which a variety of desirable workplace outcomes may be seeded or cultivated, and thus more broadly as a field of influences. Consequently, whereas the risk management model is commonly perceived as a foundation for mapping possible causes of accidents with the intent of preventing further accidents in an existing system, specifically in the form of the AcciMap method, the SIS conceives the risk management model as the foundation for mapping possible influences on desirable workplace outcomes, principally—although not solely—with the intent of designing an envisioned system.
Specifically, the SIS recognises that, in designing future systems during periods of rapid change, sociotechnical factors at multiple system levels must be aligned for desirable outcomes to be realised—not just for the workplace under study, but for society as well. If, for example, the pace of technological development outstrips the pace of change of organisational structures, workplace legislation, or government policy, which is possible during periods of significant societal transformation (Rasmussen Citation1997), the potential benefits of change may not be fully realised and, worse still, adverse consequences may be experienced. Consequently, the dynamic interactions between factors at different sociotechnical levels are important considerations in the design of future work systems.
The SIS therefore analyses multi-level sociotechnical influences that must be considered in design to cultivate desirable outcomes for workplaces and for people and society more generally. By sociotechnical, we mean social, psychological, physical, cultural, or technological in nature, and by influences, we mean factors that have a capacity to have an effect on the outcome. The outcomes may relate not just to safety, but also to such criteria as productivity, employee wellbeing, and acceptability or conduciveness to the continuing development or betterment of society.
illustrates the representational scheme of the SIS with sociotechnical system levels and workplace outcomes we have found useful in a proof of concept study, which is the focus of the next section. The factors at each level are shown in boxes. The desired workforce outcomes are shown in ovals. The directional links signify that one factor can be reasonably expected to have an effect on, or influence, another factor or outcome in the model.
Figure 3. Representational scheme of the sociotechnical influences space (SIS).
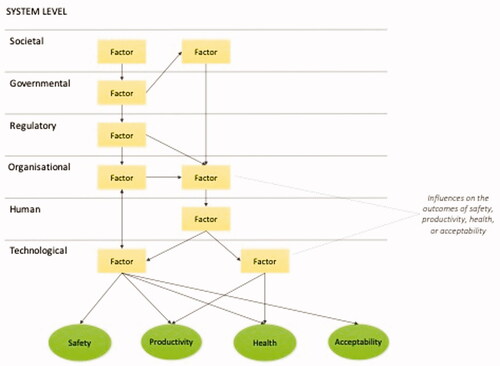
The inclusion of the societal level in the SIS recognises the explicit consideration that must be given to societal or human needs and goals, or what Vicente (Citation2004, 45) refers to as “a problem worth solving”. Focussing on technological innovation, without due concern for the physical, psychological, social, and cultural implications of their introduction, risks erosion of human wellbeing and the overall quality of life. Nevertheless, the SIS also incorporates a technological level, recognising the materiality inherent in social systems (Law and Singleton Citation2013) and that, throughout history, radical changes in societal structures have commonly coincided with the development of novel technologies (Wright Citation2000). The SIS therefore incorporates both a top-down and bottom-up perspective on design.
Further, the SIS recognises that the outcomes designers strive to attain with their solutions must expand beyond considerations relating to performance and safety and workers’ health or wellbeing (Dul et al. Citation2012) to encompass results beyond the workplace, as decisions taken within the work system can shape the world we live in (see also Wilkin Citation2010). Considering multiple outcomes—even if they are interrelated—is important in the design of work systems encompassing physical, cultural, social, psychological, and technological factors, as each outcome can provide both a different perspective for examining the work system and a distinct criterion for evaluating system success (Vicente Citation1999). It has been suggested that, at the very least, “a truly effective system should not pose a safety threat, should be economically viable, and should enhance the quality of life of its workers” (Vicente Citation1999, 20). However, the need for workplace designs to contribute to the continuing evolution or development of a flourishing, progressive society is also an important goal—in a truly holistic, problem-driven approach.
Finally, by incorporating multiple sociotechnical levels within a single, integrated representation, the SIS underscores the importance of interactions between factors at different system levels in the design of work systems. Dynamic interactions between parts of a system can exert a tremendous influence on its performance, and even small changes in one part can create reverberations throughout the system and make a significant difference to the outcomes (Vicente Citation2004). Consequently, the design of work systems must account for dynamic relationships between multiple system factors in shaping workplace results. Notably, however, the SIS differentiates between relationships that support the inclusion of factors and outcomes in the representation of the sociotechnical system, which are empirical, logical, or hypothetical in nature, and the actual interactions that occur in the sociotechnical system, which are dynamic. Only actors in the sociotechnical system can account for, or shape or influence, these dynamic interactions. These and other key features of the SIS are discussed in the next section in the context of a specific example of a SIS for a complex sociotechnical system.
Sociotechnical influences space of an envisioned work system
The framework provided by the SIS was used to analyse and construct a representation of an envisioned work system. To explore the concept and its practical potential for addressing large-scale, ‘real-world’ problems, the system selected for this proof of concept study was the Royal Australian Air Force’s future workforce. We repeat that this is a hypothetical study in that the organisation has not yet made a decision to replace its current human workforce with human-artificial agent work systems on a large scale in the future. Rather, the motivation for this research is the long-term possibility of incorporating sophisticated artificial agents into a predominantly human workforce. Some of the envisaged benefits of introducing advanced automation and intelligent technologies into the future workforce include reduced risk to human lives and enhanced or novel military capability.
Given this potential for revolutionary change in the workforce design, a SIS was constructed to understand the range of social, psychological, cultural, and technological factors that must be considered and managed, so that the result is a workforce that is safe, productive, healthy, and acceptable to the continuing development of society. This analysis would provide a representation of the wider sociotechnical problem space, including factors both internal and external to the workplace, which could serve as a basis for organisational decision making. Specifically, by identifying factors at multiple system levels that could shape workplace outcomes, the SIS could provide a more comprehensive basis for making decisions about the distribution of work, or function allocation, between humans and machines in the future workforce, beyond the usual considerations for human performance. In addition, similar to the goals of Rasmussen’s (Citation1997) risk management framework, the SIS could provide a basis for identifying organisational units or actors within Air Force who, through their spheres of influence and responsibility over particular factors in the representation, could shape the outcomes that are reached over time, specifically by managing the dynamic interactions between factors at different sociotechnical levels in this rapidly evolving problem space.
The following sections present the SIS developed for these overarching objectives. Subsequently, this example is used to explore the concepts and potential contributions of the SIS, and to examine the similarities and differences with Rasmussen’s (Citation1997) risk management framework.
SIS development
The SIS was developed by examining a wide range of studies addressing the psychological, social, cultural, or technological issues and consequences of incorporating higher levels of advanced automated and intelligent agents into workplaces and society. The aim was to identify factors that have been either reasoned or demonstrated to shape the performance of human-machine systems. Although other methods, such as knowledge elicitation with subject matter experts, field observations of workplaces with a mix of human and machine workers, and experimental studies could also have been used to identify factors of significance, it was considered important, and pragmatic, to first establish the knowledge already available in the published literature, which is methodologically diverse and spans a range of scientific disciplines.
The method adopted for this study is best described as a narrative review, whereby relevant information was identified from a variety of sources and forward and backward citation used to uncover further information. This approach is suitable when one is considering methodologically distinct studies on many different, though related, topics, usually with the intent of reinterpretation or interconnection (Baumeister and Leary Citation1997). The aim is to assess a broad range of issues on a given subject and thus obtain a holistic perspective. summarises the strategies adopted for the narrative review.
Table 1. Strategies for the narrative review.
The structured search noted in utilised EBSCOhost to explore a range of databases across different subject areas over a 5-year period from 2014 to 2018. A variety of keywords were trialled and the results assessed for relevance to the domain of interest before the final set was selected. This set combined interchangeable terms for intelligent technologies (artificial intelligence; autonomous system; robot; human-automation; human-robot; autonomous weapon) with interchangeable terms for concepts relating to work and various dimensions of workplace performance including both general terms as well as terms specific to the SIS levels (workforce; workplace; work; culture; cultural; social; psychology; psychological; cognition; cognitive; physical; society; societal; government; governmental; regulatory; regulation; organisation; organisational; technology; technological; human). The search formula combined the keywords within each set with the Boolean operator ‘OR’ and linked the two sets with the Boolean operator ‘AND’, promoting the identification of a wide range of documents across diverse disciplines, consistent with the strategy of canvassing the literature as broadly as possible at first and then narrowing down rapidly with a broad inspection based on the specific aims of the SIS.
Limiting the results to those written in English, an initial search returned a total of 204,636 documents of which 30,453 were from academic journals and 718 were reviews. The decision was made to restrict the search to reviews, and as the final search returned 1242 review articles, only reviews identified by Scopus, of which there were 725, were examined for pragmatic reasons. For each of these reviews, the title, abstract, and keywords were assessed for mention of concepts that could plausibly shape the performance of human-machine systems in the Air Force. Review articles that were assessed to be irrelevant by the analyst performing the search were excluded, leaving 51 articles requiring further analysis.
Further to the structured search, the narrative review incorporated additional strategies for identifying pertinent articles, including background knowledge, colleagues, and semi-structured database searches (). Forward and backward citation searching were also conducted to collect further articles from the pool of studies identified through these strategies. No date range constraints were applied to these strategies, and articles were only analysed if they contained relevant content as judged by either of the two analysts.
An iterative approach to analysing the literature was utilised to define concepts relevant to the SIS, specifically factors relevant to the performance of human-machine systems in the Air Force. The literature was read and revisited as new insights or interpretations became evident to the analysts, leading to the generation or development of provisional concepts. As new concepts became apparent, targeted semi-structured database searches were performed to collect further information for assessing the suitability of including specific concepts within the SIS. These semi-structured searches were performed through Google, Google Scholar, and EBSCOhost databases. Keywords combined synonyms for intelligent technologies as noted above with terms relating to specific concepts being considered for inclusion in the SIS (e.g. privacy; accountability; reliability), using Boolean operators (i.e. ‘AND’, ‘OR’) as appropriate.
Concepts were assigned and grouped into system levels as they emerged, largely informed by Rasmussen’s (Citation1997) risk management model but with iterative modifications made in response to shifts in analyst insight and understanding, ultimately leading to the addition of two system levels. The scope and focus of specific concepts similarly evolved throughout the process, resulting in their refinement through splitting, combining, or discarding of concepts. This iterative process of analysis continued until analysts exhausted attempts to uncover any further novel concepts or factors and were satisfied that the factors and system levels provided a convincing and comprehensive representation of the problem space. Labels and descriptions of the factors were written to aid understanding and to indicate some of the key reasons for their inclusion in the SIS. However, we expect that, like many other models, the SIS must necessarily evolve through inclusion, elaboration, or clarification of concepts over time to remain a solid foundation for design throughout the lifetime of a program (Flach et al. Citation2008).
Resulting representation
The SIS produced from the analysis described above is presented in . This figure shows that six sociotechnical system levels were necessary for modelling the social, psychological, cultural, and technological factors of significance in a future workforce with mixed human and artificial agents in Air Force, namely societal, governmental, regulatory, organisational, human, and technological. provides brief descriptions of these six levels.
Figure 4. SIS of envisioned work system.
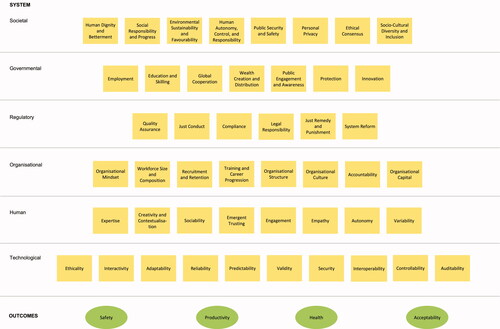
Table 2. Description of sociotechnical system levels in the SIS.
also shows the factors at each of the six levels in the SIS. These factors can plausibly shape the performance of human-machine systems in Air Force, by having an effect on or influencing any one or more of the workplace outcomes of safety, productivity, health, and acceptability, whether directly or mediated through other factors in the representation. Appendix A provides brief summaries of all of the factors as well as expanded examples of some of the factors at each level to illustrate the different foci at each level.
In addition to the system levels and factors in the SIS, incorporates the outcomes of safety, productivity, health, and acceptability. These four outcomes encapsulate that, for the Air Force to remain successful and viable with mixed human and artificial agent work systems into the future, the organisation and its activities will need to be safe for its workers, the public, and the environment; be productive to remain economically viable, enable faster decision cycles and response capability, and gain competitive edge over potential adversaries; ensure the mental and physical health of its workers; and be morally acceptable to Australian society and responsive to public concerns.
depicts a sample of links relevant to the factors and outcomes in the SIS, illustrating that three kinds of relationships are accommodated in the representation. Empirical relations are derived from historical, experimental, field, or case study observations. Logical relations are established on the basis of reasoned arguments, or on relationships that are deliberated rather than observed. Hypothetical relations are hypothesised relationships derived through analysis, synthesis, and modelling of prior knowledge or findings within the framework provided by the SIS. Brief descriptions of the links shown in are provided in Appendix B to provide examples of each of these three kinds of relationships.
SIS construction and potential applications
Having presented the SIS of an envisioned work system, in this section we consider a number of points relating to its development and explore some potential applications of the resulting representation.
Domain differences
The SIS presented above may seem relevant to many different systems beyond the Air Force domain that was the motivation for its development. One reason for any potential commonalities is that the SIS was deliberately developed to take advantage of observations, arguments, or experiences in other domains, where these alluded to factors that could plausibly shape the performance of human-machine systems in the Air Force. In addition, many studies are not specific to particular domains. Although the descriptions of factors could have been written more specifically for the Air Force, care was taken to avoid referring to specificities, such as particular cases, scenarios, or examples, as the intent was to produce a representation that could facilitate thinking about many different situations or circumstances over time, consistent with the ideas discussed earlier in this paper. Nevertheless, the descriptions were written in a style that accommodates an Air Force audience.
In any case, it is conceivable that the unique purposes, functions, and structures of other systems will manifest differently in a SIS compared with that developed for Air Force. While the nature of these differences cannot be known unequivocally without comprehensive analyses, several likely distinctions can be anticipated. In a healthcare context, for example, both clinicians and patients have the potential to shape the utilisation of artificial agents in that domain, perhaps through the development of trusting relations (Ahmad, Stoyanov, and Lovat Citation2020; Gerke, Minssen, and Cohen Citation2020). However, patients are not human workers and so fall outside the bounds of the system levels or factors included in the SIS of Air Force. Similarly, in office work, interactions with clients or customers may be important to model explicitly. Another potential point of difference is that factors relating to profit motives are integral to privately funded organisations, and arguably also to organisations receiving mixed funding, such as healthcare systems, whereas the SIS of Air Force, which is a publicly funded organisation, does not incorporate such factors.
Road transport provides a further illustration of how differences in the fundamental nature of systems can translate into distinct representations. For example, in the case of intelligent technologies developed for use in the private sector, factors relating to organisational principles and priorities may be less important. Similarly, where driverless vehicles are utilised in commercial road transport, factors relating to human performance may require different kinds of considerations.
Finally, while the Air Force bears greater resemblance to the Army and Navy organisations in Australia, there are important distinctions between these systems, arising from their unique operating environments as well as their particular purposes, functions, and structures. For example, by virtue of operating primarily in the land domain, the Army is likely to demonstrate a continued reliance on the dismounted soldier and to integrate highly mobile robots for activities such as load-carrying support or scouting and reconnaissance. Consequently, it is plausible that Army personnel may interact with intelligent technologies in more intimate or physically interactive ways than either Air Force or Navy, who operate large aircraft and ships with requisite technical infrastructure to support heavy, computationally-intense technologies. As such, in a SIS of Army, it may be important to consider more explicitly the physical appearance or movement patterns and gestures of intelligent robots given the human tendency for anthropomorphic or zoomorphic interpretations of machine behaviour (de Visser et al. Citation2016; Sandry Citation2015). Likewise, owing to the Navy’s unique role in humanitarian assistance and disaster relief operations as a first responder and provider of bulk supplies and manpower (Cong Citation2019), it is conceivable that Navy personnel may have special needs in frontline interactions with civilian populations. Consequently, it may be important to consider more explicitly the dynamic between Navy personnel, civilians, and intelligent agents in a SIS of this domain. Finally, the unique purposes, priorities, and structures of military forces of other nations may manifest differently in a SIS.
Plausibility and value
One way of exploring the plausibility and potential value of the SIS is to juxtapose its results with those from other similar initiatives. In this section, we consider two studies conducted by Australian government research agencies, one specific to Defence and the other with a national scope. Potentially, these studies could also be utilised by the Air Force in making decisions about the incorporation of intelligent technologies into the future workforce. While a direct, detailed comparison of the results is not possible, because of differences in the objectives of the work, a broad scan is informative. We do not offer an appraisal of studies or reviews in the wider literature, other than to note that, to our knowledge, the SIS covers a broad range of sociotechnical system levels and factors, whereas other studies offer detailed consideration of some of the issues, in keeping with their specific objectives.
The first study we consider sought “to develop a pragmatic and evidence-based ethical methodology for AI projects in Defence” (Devitt et al. Citation2020, i). In that its overarching intent is “to ensure that the introduction of the technology does not result in adverse outcomes” (i), its goals are broadly similar to those of the SIS, although the study may be more concerned with moral and ethical issues and less with safety, productivity, or health outcomes. The method for the study was a three-day workshop, supplemented by a virtual platform enabling remote participation. The workshop was attended by 104 domestic and international subject matter experts from 45 organisations, including representatives from Defence, other Australian government agencies, civil society, universities, and industry. The attendees were encouraged to consult a wide variety of literature before, during, and for 30 days after the workshop. The workshop itself comprised group discussions and oral presentations by experts in the fields of ethics of war; ethics of data and artificial intelligence; autonomous systems in Defence; adaptive autonomy; human factors that affect human-autonomy teaming; and assurance of autonomy.
The resulting report, which incorporated insights from further consultation with Defence stakeholders and examination of some existing published frameworks, identified five facets and 20 subtopics to be explored when considering the use of artificial intelligence in Defence. As described in Devitt et al. (Citation2020), the five facets are: responsibility (who is responsible for AI); governance (how is AI controlled); trust (how can AI be trusted); law (how can AI be used lawfully); and traceability (how are the actions of AI recorded). The discussion in the report is organised in terms of these five facets and 20 subtopics.
The facets, subtopics, and associated issues that are noted briefly or discussed in more depth in the report with the potential to impact workplace outcomes correspond with factors at different levels of the SIS, with an emphasis on regulatory concerns (e.g. quality assurance; compliance; legal responsibility) and technological performance (e.g. interactivity; validity; security; auditability). Concerns around organisational accountability and human trust are also captured in depth in the report, mirroring some of the more prominent or well-researched themes in the literature. The SIS appears to consider more explicitly various societal needs and concerns (e.g. human dignity and betterment; social responsibility and progress; environmental sustainability and favourability), government policy imperatives (e.g. employment; education and skilling; wealth creation and distribution), and human-level concerns (e.g. sociability; empathy; autonomy). These aspects are relatively underrepresented in the literature.
The second study, which had a national scope, sought to identify “key principles and measures that can be used to achieve the best possible results from AI, while keeping the well-being of Australians as the top priority” (Dawson et al. Citation2019, 4). Although this study focuses on civilian applications, it emphasises the need to consider the health of workers and complex moral issues, as well as incorporating issues relating to system safety and productivity. To develop the ethics framework, Dawson et al. conducted workshop sessions, engaged advisory and technical expert groups, and sought public consultation to generate feedback on a discussion paper. The workshops brought together 91 invited delegates from industry, universities, and government, and were conducted across four Australian capital cities. Participants were given the opportunity to share their perspectives and to interrogate the proposed ethics framework. Public consultation was sought via written submissions.
The resulting framework incorporates eight core principles for guiding organisations in the use or development of intelligent technologies. As described by Dawson et al. (Citation2019), these core principles are: (1) generates net-benefits, (2) do no harm, (3) regulatory and legal compliance, (4) privacy protection, (5) fairness, (6) transparency and explainability, (7) contestability, and (8) accountability. The associated issues are discussed by exploring a number of case studies and trends, in three chapters titled ‘data governance’, ‘automated decisions’, and ‘predicting human behaviour’. However, issues considered within the SIS are also described in two other chapters in the report covering ‘existing frameworks, principles and guidelines on AI ethics’ and ‘current examples of AI in practice’.
Overall, issues noted briefly or discussed in greater depth in the report with the potential to impact workplace outcomes correspond with factors at different levels of the SIS, with an emphasis on societal needs (e.g. personal privacy; ethical consensus; socio-cultural diversity and inclusion), governmental considerations (e.g. public engagement and awareness; protection), and regulatory concerns (e.g. just conduct; compliance; legal responsibility). However, we note that, as the report considers issues in the context of domains outside of Defence, some of the topics, such as trusting relations between consumers and organisations, cannot be precisely captured by the SIS we developed for a military domain. The SIS, on the other hand, appears to consider more explicitly various organisational factors (e.g. organisational mindset; organisational structure) and human-level considerations (e.g. expertise; creativity and contextualisation; sociability; engagement; empathy; and variability). These human-level concerns, which emphasise how unique human abilities can be harnessed, and in fact are needed, to complement artificial intelligence in realising positive outcomes in human-machine systems, are themes that are relatively underrepresented in the general literature.
The intent of the preceding discussion is not to establish whether the SIS is better or worse than other approaches, but rather to demonstrate that the method adopted for the development of the SIS results in a reasonably comprehensive representation. Further, although we do not claim that the SIS is complete and considers all potentially relevant factors, it may be argued that the structure provided by the SIS, specifically the sociotechnical levels and outcomes, promotes consideration of a broad range of factors. The SIS may therefore provide a useful framework for studies utilising alternative methods, such as knowledge elicitation with subject matter experts or systematic reviews, in guiding the types of experts or stakeholders consulted as well as the kinds of factors investigated in depth. Moreover, the SIS provides a graphical representation that may be useful, particularly for providing a visual summary of the issues and the domains of responsibility or interest of different stakeholders.
Perhaps most importantly, the SIS organises information in a way that emphasises how factors at a number of sociotechnical system levels, both internal and external to the workplace, can shape desirable outcomes. The levels, factors, and outcomes are clearly differentiated and provide different frames of reference for assessing workplace effectiveness. In addition, the incorporation of multiple systems enables factors and levels to be examined within the context of the broader problem space. Given the focus on designing envisioned work systems, this framing of the problem space underscores the interconnecting factors and levels, and associated stakeholders, that must be considered systematically in design to maximise positive outcomes, not just for the workplace under consideration but more broadly for society as well. Misalignments in the sociotechnical system can compromise the outcomes.
Potential applications
The SIS of Air Force presented above was developed in view of the possibility of incorporating sophisticated artificial agents into a predominantly human workforce on a large scale in the future. While artificial technologies have already demonstrated value in many aspects of military operations, such as removing humans from danger zones, the future military environment is expected to challenge the speed and capacity of human decision makers to unprecedented levels (Australian Defence Force Citation2018). Personnel are expected to be confronted with a deluge of information that is variable in terms of its quality, relevance, and trustworthiness, creating significant uncertainty in decision making. In addition, new forms of conflict, such as cyberattacks, and emerging technologies, such as hypersonic weapons, will limit the time available for decision making.
The development of the SIS reinforced the view that the large-scale introduction of advanced intelligent agents, with high levels of autonomy, into predominantly human workforces is still a research endeavour and a long-term possibility, although the potential for a disruptive step-change or leap-ahead capability cannot be ignored and must be monitored. Many factors in the SIS are based on emerging ideas and early indications of issues or concerns relevant to the successful utilisation of intelligent technologies as opposed to long-held or highly researched notions. Notably, there are still considerable uncertainties in the capabilities of emerging technologies and prediction of the specific timing and location of effects is difficult. Also, jobs and roles will continue to evolve with the introduction of artificial technologies into workplaces. The organisational strategy in workforce design must therefore necessarily be one where Air Force leaders monitor and shape developments in artificial intelligence (e.g. in emerging technologies, in laws and regulations, in government policy, in education and training needs, in public pressures and concerns, and in cost) and continue to adjust the future workforce model, including possibilities for distributing work across human and machine actors, as required.
Potentially, the SIS of Air Force provides a useful tool for supporting organisational leaders during this process. Specifically, the SIS provides a framework for understanding the problem space and keeping track of where emerging ideas, sentiments, and developments in artificial intelligence, at all levels of the sociotechnical system, may have implications for the landscape of interacting factors in the SIS. In addition, the SIS provides a means for conceptualising how these developments and interactions may widen or narrow the space of possibilities for incorporating intelligent technologies into the future workforce successfully and, more specifically, for organising work across human and machine actors effectively, through their effects on the outcomes of safety, productivity, health, and acceptability. The SIS may also support Air Force leaders in making decisions about where to invest resources in shaping the landscape of interacting factors, for instance through research and development or through cooperation with other actors, once again at all levels of the sociotechnical system, with the intent of broadening the space of possibilities for the effective utilisation of intelligent technologies in the future workforce. Some examples of these ideas are provided later in this section.
To this end, consistent with the intent the AcciMap, the SIS provides a framework for systematically identifying and mapping Air Force actors and stakeholders, who through their spheres of influence or responsibility over particular factors in the representation—both within and beyond the organisation—have the potential to shape the outcomes that are reached. In addition, the SIS provides a means for identifying responsibility gaps, if any, in the management of specific factors and defining actors, stakeholders, or other initiatives for managing those gaps in the organisational strategy. Finally, the SIS emphasises the coordination needed between organisational actors and stakeholders at different system levels in shaping the various factors and thus cultivating desirable outcomes in the design of the future workforce.
These potential applications of the SIS recognise that the effective utilisation of intelligent agents and distribution of work in the future workforce may change and evolve over time, with the possibilities widening or narrowing, shaped by the decisions of actors in the sociotechnical system. For example, there is still considerable debate about the nature of the legal framework needed for the governance of intelligent technologies, including whether existing laws and regulations will suffice; whether they need to be amended and, if so, how; or whether new codes of conduct are necessary. It is therefore plausible that the introduction of new laws or regulations, emphasised by factors such as Just Conduct or Legal Responsibility at the regulatory level of the SIS, may rule out certain opportunities for the incorporation of intelligent agents into the future workforce, despite heavy investments in technology development over many years and thus high levels of readiness in relation to such factors as Reliability and Validity, emphasised at the technological level of the SIS. Such scenarios or developments must be continually evaluated and accommodated, for instance in ongoing organisational planning for Workforce Size and Composition and Training and Career Progression, emphasised at the organisational level of the SIS, and also considered in ongoing organisational cooperation with actors represented at the regulatory and governmental levels of the SIS.
The SIS is also consistent with recent research on cognitive work analysis to support emergent work distribution in agile human-machine teams (Naikar et al. Citation2021) and human crews (Elix and Naikar Citation2021). In contrast to both fixed and dynamic approaches to function allocation (e.g. Kaber Citation2018; Parasuraman and Wickens Citation2008; Wright, Dearden, and Fields Citation2000), this approach is not necessarily concerned with specifying a priori optimal allocation of functions between actors, whether humans or machines, given assumptions about how work should be distributed in relation to anticipated variations in situational parameters. Rather, it is concerned with bounding the possibilities for work organisation between human and artificial agents, with the actual distribution emerging in situ from their respective spaces of possibilities for action, which may be overlapping, thus creating redundancy in the system. This approach, which recognises that many events or contingencies and the details or nuances of those situations cannot be anticipated ahead of time by analysts or designers, is consistent with the intent of designing for agility to accommodate environments with high levels of instability, uncertainty, and unpredictability.
Whereas cognitive work analysis is intended to support detailed design considerations in the context of specific technologies and domains of use, the SIS is intended to support broader design considerations, where there are numerous, unspecified technologies, the details of which are still emerging. However, the intent of both approaches is to broaden the space of possibilities for action, within certain boundaries of successful operation, supporting flexible, adaptive action by actors in situ. Accordingly, decisions taken by actors within the bounds of successful action articulated in cognitive work analysis may be reflected in the conceptualisation of the wider sociotechnical problem space provided by the SIS, and vice versa. For example, intelligent technologies may not be utilised by actors on every operation, with the intent of preserving Expertise and promoting Human Dignity and Betterment, emphasised at the human and societal levels of the SIS, respectively. However, actors may take decisions in situ to utilise artificial intelligence in view of terrain or aerodynamic constraints and the need for precision or efficiency, with the intent of ensuring Public Security and Safety or Environmental Sustainability and Favourability, represented at the societal level of the SIS. Such values and considerations may be accommodated in the design of the sociotechnical system, for instance in laws or regulations, emphasised at the regulatory level of the SIS, or in organisational policies for Training and Career Progression, represented at the organisational level.
Similarly, intrinsic human Variability in preferences for working with intelligent technologies, emphasised at the human level of the SIS, can be accommodated in design, as a function of the circumstances. For example, when time constraints rule out humans from successful action, intelligent technologies may be utilised, but the possibility of humans taking carriage of the work on other occasions, according to their preferences, can be supported in the design of the human-machine partnering strategy and the wider sociotechnical system. In such ways, the need to support emergence in the sociotechnical system to account for events that have not been—and cannot be—anticipated by analysts or designers, while at the same time fostering the outcomes of safety, productivity, health, and acceptability, is accommodated by the SIS, and consistent with the more detailed design framework provided by cognitive work analysis (Rasmussen, Pejtersen, and Goodstein Citation1994; Vicente Citation1999). Consequently, the space of possibilities for action of human and machine actors in the system, conceptualised by the SIS and cognitive work analysis, is constantly changing shape as a function of the circumstances and the decisions or actions taken by actors at all levels of the sociotechnical system.
Finally, we note that while basic visual representations of the SIS provide adequate support for analysts in constructing and evaluating the model, these visualisations may be insufficient for imbuing end-user understanding or for driving further development. It is therefore reasonable to expect that Air Force, as the end-user of the SIS, may benefit from access to sophisticated visualisation tools with interactive capabilities. A novel software tool is currently being developed to address this need, hosted on a web-based platform integrating applications for knowledge elicitation, data analysis, and information visualisation (Tieu and Ong Citation2021). Aside from providing a platform for presenting and exploring descriptions of the factors and empirical, logical, and hypothetical relationships in a visual context, the SIS visualisation tool provides significant opportunities for collaboration with Air Force. Specifically, the tool allows users to insert comments, questions, or links to relevant documents, and to map new information onto the model. Thus the visualisation tool provides a mechanism for Air Force to contribute its domain expertise to the development of the SIS, building upon and complementing the use of published literature in informing the construction of the model. Further, a potential application involves superimposing actors or stakeholders with requisite authority or expertise onto specific factors or system levels as a way of identifying individuals or groups—internal or external to Air Force—with the ability to provide consultation in relation to specific concerns or to shape the outcomes of the work system. Such an application would also likely be useful in identifying any responsibility or knowledge gaps tied to specific factors or levels, which if overlooked could threaten the successful incorporation of advanced technologies into the Air Force.
Comparison with risk management framework
Having discussed a specific example of a SIS for an envisioned work system, in this section we compare some of its key features with Rasmussen’s (Citation1997) risk management framework, specifically the risk management model and AcciMap method. In particular, we consider similarities and differences in the intent, outcomes, levels, factors, and relations.
Intent
As the SIS is motivated by Rasmussen’s (Citation1997) risk management framework, it has many commonalities with the risk management model and AcciMap method, and arguably any dissimilarities may be explained largely by differences in the intent. Underpinning the risk management model is the goal of safety improvement, and the fundamental realisation that actions at multiple levels of the sociotechnical system can contribute to accidents, not just actions at the level of frontline operators. Accordingly, the AcciMap method identifies the causal factors of accidents in a specific domain at multiple levels of the sociotechnical system. Further, in a proactive approach to risk management, generic AcciMaps are created based on generalisation across multiple accidents, and the resulting representation is used as a basis for identifying actors who can shape the course of events in future scenarios through closed-loop adaptive control (Rasmussen and Svedung Citation2000).
The SIS, on the other hand, is motivated by the goal of designing systems, particularly envisioned systems, that are not only safe, but also productive, healthy, and acceptable places to work. It is based on the recognition that the sociotechnical system levels at the heart of Rasmussen’s (Citation1997) risk management framework may be construed more broadly as a field of influences shaping a variety of desirable consequences, not just as a field of risks shaping the release of accidents. Given this scope, the SIS cannot be based solely on the analysis of accidents, and cannot be viewed straightforwardly as a map of contributing factors to accidents, or AcciMap. Instead, the SIS must accommodate factors revealed in productive, healthy, and acceptable patterns of work as well. Nevertheless, as is the case with the AcciMap, the resulting representation may serve as a basis for identifying organisational actors who, through their spheres of responsibility or influence over contributing factors, can steer the system to desirable outcomes through closed-loop adaptive control. More specifically, the SIS may be used to support design decisions about the distribution of work in human-artificial agent work systems, as discussed above. Still, regardless of any differences in the intent, the SIS may be viewed simply as an addition to the suite of tools comprising the risk management framework, specifically as a design tool to support organisational transformation during periods of major technological and social change.
Outcomes
In line with the differences in intent, the risk management model and AcciMap method direct attention to safety outcomes, whereas the SIS emphasises a broader range of outcomes. In the case of future workforce design incorporating humans and artificial agents in the Air Force, outcomes relating to productivity, health, and acceptability, as well as safety, were found to be important. Historical evidence demonstrates changes to productivity occurring as a result of introducing automation and artificial intelligence into workplaces (Acemoglu and Restrepo Citation2018; Autor Citation2015). In addition, the physical and mental health of workers has been shown to suffer as a result of decreased personal autonomy in the workplace (Demerouti et al. Citation2001; Karasek and Theorell Citation1990), although automation and intelligent technologies also have the potential to give workers greater autonomy, for instance, by enabling them to focus on their uniquely human capabilities and interests (Calvo et al. Citation2020). Finally, the moral and ethical acceptability of artificial agents may also determine the ways in which these technologies are utilised in the workplace (Sjöberg and Drottz-Sjöberg Citation2001; Taebi Citation2017).
Levels
The risk management model focuses attention on a number of sociotechnical system levels, ranging from government to hazardous work process (), and the AcciMap method in turn typically incorporates comparable levels for accident or incident analysis (). The SIS is based on this foundation, but also incorporates a societal level for the analysis of an envisioned work system. This inclusion reflects the greater emphasis required on the needs and goals of humankind when there is potential for significant societal transformation, as well as the recognition that societal level influences can plausibly affect other factors and outcomes. For example, societal needs and concerns relating to the dignity people derive from their work, privacy and security breaches, and racial or religious discrimination are pressing, high-profile considerations in wide-ranging discussions on the large-scale utilisation of artificial agents in workplaces and society. Furthermore, public opinions or pressures in relation to such concerns can significantly shape political activities or government policies and organisational priorities relating to the incorporation of artificial agents into workforces.
Second, the SIS incorporates a technological level, reflecting the inherent materiality of social systems (Law and Singleton Citation2013) and the critical role of technological innovation in societal transformation throughout history (Wright Citation2000). In addition, historical experience and research show that technological characteristics of automated or intelligent agents can have considerable impact on workplace outcomes, both transformative and catastrophic (e.g. National Transportation Safety Board Citation2019).
Finally, in contrast to the risk management model and AcciMap method, the SIS does not incorporate levels modelling specific process or activity sequences, such as the physical processes and actor activities and the equipment and surroundings levels in the AcciMap in . While these levels may be important for accident or incident analysis, and are not irrelevant to envisioned systems, the details usually associated with these levels typically haven’t yet been defined for envisioned systems, and may in fact be the object or goal of the design activity. Nevertheless, the intent behind these levels is accommodated in the SIS of Air Force in that specific considerations relating to physical processes, actor activities, and equipment and surroundings are incorporated within the representation, where these aspects have the potential to affect workplace outcomes in the envisioned system.
Factors
The factors in a SIS and AcciMap reflect their respective intent, levels, and outcomes. Consistent with the intent of accident analysis and safety improvement, the AcciMap method focuses on modelling factors of significance in prior accident or incident sequences. The factors are regarded as significant in that they signify possible causes of undesirable occurrences in an existing system. In contrast, the SIS focuses on modelling factors of significance in the design of envisioned work systems. In this case, the factors are regarded as significant in that they have the potential to shape or influence desirable outcomes in the future system. Accordingly, the SIS is not limited to causal factors revealed in accidents or incidents, but incorporates factors revealed in successful performance. Further, the SIS is not limited to factors manifested in existing systems, but accommodates factors that are reasoned or hypothesised to plausibly affect outcomes in the envisioned system.
Relations
Consistent with their different objectives, the links in an AcciMap reflect empirical relationships revealed in prior accident or incident scenarios, whereas the SIS accommodates empirical observations of successful performance. The AcciMap method may also include logical relations, which were not observed in past accidents or incidents, but could be reasoned to have played a role in those undesirable occurrences. The SIS in addition accommodates logical relations that may not necessarily have a basis in an existing system, but may be reasoned to shape results in an envisioned system. Finally, the SIS also accommodates hypothetical relationships that appear plausible to hypothesise based on analysis of empirical or logical relationships in the representation. In these ways, the SIS considers relationships between factors that may not yet have manifested or been established as relevant in the past in an existing system, but which may nonetheless shape performance in the future in an envisioned system. As emphasised above, the empirical, logical, and hypothetical links in the SIS support the inclusion of particular factors in the representation, but do not necessarily reflect the dynamic relationships between factors arising from the actions or decisions of actors in the envisioned system.
General discussion
In view of recent advances in intelligent and automated technologies, in this paper we have presented the SIS, a tool for considering multi-level sociotechnical influences in the design of envisioned work systems. We have explained the basic concepts of this analysis and design approach and we have demonstrated the plausibility of constructing such a representation of a complex sociotechnical system. Specifically, given the long-term possibility of incorporating higher levels of artificial intelligence into a predominantly human workforce in the Royal Australian Air Force, the SIS was used to define the societal, governmental, regulatory, organisational, human, and technological factors of significance in this case, and to discuss some potential applications of the resulting representation, primarily for supporting organisational transformation and function allocation decisions in design.
Given the potential for widespread societal and workplace transformation arising from developments in artificial intelligence, the SIS presents a holistic approach to analysis and design that goes beyond the usual considerations for human performance in the workplace to consider the wider sociotechnical problem, attending to societal needs and challenges while recognising the bottom-up push from emerging technologies. As such, the SIS encompasses multiple system levels, factors, and outcomes as an integral part of system design, and promotes consideration of factors both internal and external to the workplace in decisions relating to the incorporation of advanced technologies into the future workforce and distribution of work across humans and machines. Consequently, irrespective of whether or not a SIS of a complex sociotechnical system can be regarded as complete, it leads to consideration of a much broader range of factors than standard approaches to function allocation, whether fixed or dynamic (e.g. Kaber Citation2018; Parasuraman and Wickens Citation2008; Wright, Dearden, and Fields Citation2000).
Further, the SIS is consistent with cognitive work analysis (Rasmussen, Pejtersen, and Goodstein Citation1994; Vicente Citation1999) and its use in designing agile human-machine teams (Naikar et al. Citation2021) and human crews (Elix and Naikar Citation2021), in that it expressly promotes an approach to design that accommodates emergence in the sociotechnical system to account for events that have not been—and cannot be—anticipated by analysts or designers, while at the same time fostering the outcomes of safety, productivity, health, and acceptability. Whereas cognitive work analysis provides a detailed design framework for use with specific technologies and operating domains, the SIS supports consideration of the wider sociotechnical problem and is especially well suited to cases where there are numerous, unspecified technologies, the details of which are still emerging. However, the intent of both approaches is to broaden the space of possibilities for action in design, given certain boundaries, so that actors are supported in adapting their behaviours to the nuances of specific situations, which cannot be foreseen, and to novel events. Such methods are needed in the design of envisioned systems, where technological innovations and social possibilities are still being forged, and in supporting actors in task environments with high levels of instability, uncertainty, and unpredictability.
By looking beyond concerns within the workplace, the breadth of sociotechnical factors that can shape design decisions becomes clear. For instance, the SIS presented in this paper suggests the need to consider whether potential distributions of work between humans and intelligent technologies are morally preferable in view of the proposition that humans derive a sense of dignity from their work. Further, the need or desire for sociocultural or socioeconomic heterogeneity may play a role in function allocation decisions, so that workplaces and society can continue to benefit from diverse perspectives and ideas in the daily conduct of life and work. Likewise, employment and economic priorities may be considered in function allocation decisions, with the intent of preserving stability in overall employment and providing people with sufficient opportunities for obtaining paid, desirable work.
As emphasised at the outset, the SIS was motivated by Rasmussen’s (Citation1997) risk management framework, and it therefore shares many features with the risk management model and AcciMap method. Although there are differences in the outcomes, levels, factors, and relations, arguably any dissimilarities may be explained primarily by differences in the intent. Given that, the SIS may be seen as an addition to the suite of tools comprising the risk management framework, with its specific contribution being to support the design of envisioned systems, particularly during periods of major technological and social change.
While the discussion thus far has focussed on the design of envisioned systems, the SIS may also be applied to the design of existing systems, particularly in times of rapid technological change or social turmoil. For example, contemporary events relating to climate change, pandemics, or terrorism may force change simultaneously at multiple sociotechnical system levels, and lack of vertical alignment or integration across levels has the potential to throw systems into chaos (Vicente Citation2004). Further, the SIS has potential application in times of relative social and technological stability. By considering the needs and concerns of humankind at multiple system levels, the SIS offers a broad view that accommodates long-standing social problems, for example in environmental sustainability, poverty, and sociocultural inequality. In earlier work, an expansion of the traditional scope of human factors and ergonomics to consider global problems has been advocated (Lange-Morales, Thatcher, and García-Acosta Citation2014; Moray Citation2000), and a lack of adequate tools for analysis and design has been reported (Thatcher, Nayak, and Waterson Citation2020). While the suitability of the SIS for meeting these calls to action is yet to be demonstrated, it provides a promising avenue for future research.
It is also worth emphasising that the intent of the SIS is not to design systems based on specific or detailed conceptualisations of the future, or likely future events, but rather to support the emergence of novel work systems, the exact components of which cannot be envisaged or specified ahead of time. Like the diagram of work organisation possibilities (Elix and Naikar Citation2021; Naikar and Elix Citation2016, Citation2021), the SIS recognises that the possibilities are infinite. The intent therefore is not to predict a system’s future state, but rather to design to enable emergence—to enable the system to evolve to a future state. Accordingly, the SIS seeks continuing multi-level fit or adaptation of social, political, organisational, and technological structures to constant change by supporting the self-organising behaviours of actors in the sociotechnical system, through the design of both hard and soft technologies.
Finally, one limitation of the current study is that while it has been demonstrated that the SIS can be applied to the analysis of a complex sociotechnical system, its value for design has not yet been established. Some steps have been outlined for the potential use of the SIS in future design activities, specifically as a tool for supporting organisational transformation and function allocation decisions. Such ideas should be implemented in future research so that the strengths and limitations of the SIS can be examined further and modifications or refinements to the concepts or methods identified. Moreover, as the current study focuses on a single domain and design application, the value of the SIS in other domains and for different kinds of design activities, including for examining existing rather than envisioned systems as well as other technologies and social questions, needs to be explored.
Abbreviations | ||
AcciMap | = | accident mapping |
SIS | = | sociotechnical influences space |
STAMP | = | systems-theoretic accident model and processes |
STECA | = | systems-theoretic early concept analysis |
Acknowledgements
We thank the following people from the Defence Science and Technology Group, Melbourne, Australia: Dr Julia Chadwick for her assistance in sourcing information relevant to some of the factors in the Sociotechnical Influences Space for future workforce design; Dr Niem Tri, Mr Martin Cross, Mr Jacob Koehler, and Mr Andrew Cruickshank for their constructive comments on earlier writing and presentations of this work; Ms Rebecca Ong for discussions about her conceptualisation of the future Defence environment; and Dr Seng Boey for his valuable feedback and continued support of this research. In addition, we are grateful to four anonymous reviewers for their thoughtful and detailed comments, which have been instrumental in helping us to improve our manuscript.
Disclosure statement
No potential conflict of interest was reported by the author(s).
References
- Abrassart, C., Y. Bengio, G. Chicoisne, N. de Marcellis-Warin, M.-A. Dilhac, S. Gambs, V. Gautrais, M. Gibert, L. Langlois, F. Laviolette, P. Lehoux, J. Maclure, M. Martel, J. Pineau, P. Railton, C. Régis, C. Tappolet, and N. Voarino. 2018. “Montreal declaration for a responsible development of artificial intelligence.” https://www.montrealdeclaration-responsibleai.com/reports-of-montreal-declaration.
- Acemoglu, D., and P. Restrepo. 2018. “Artificial Intelligence, Automation, and Work.” In The Economics of Artificial Intelligence: An Agenda, edited by A. Agrawal, J. Gans, and A. Goldfarb. Chicago, IL: University of Chicago Press.
- Agrawal, A., J. Gans, and A. Goldfarb. 2019. “Economic Policy for Artificial Intelligence.” Innovation Policy and the Economy 19: 139–159. doi:https://doi.org/10.1086/699935.
- Ahmad, O. F., D. Stoyanov, and L. B. Lovat. 2020. “Barriers and Pitfalls for Artificial Intelligence in Gastroenterology: Ethical and Regulatory Issues.” Techniques and Innovations in Gastrointestinal Endoscopy 22 (2): 80–84. doi:https://doi.org/10.1016/j.tgie.2019.150636.
- Aleksander, I. 2017. “Partners of Humans: A Realistic Assessment of the Role of Robots in the Foreseeable Future.” Journal of Information Technology 32 (1): 1–9. doi:https://doi.org/10.1057/s41265-016-0032-4.
- Augusto, J. C., V. Callaghan, D. Cook, A. Kameas, and I. Satoh. 2013. “Intelligent Environments: A Manifesto.” Human-Centric Computing and Information Sciences 3 (1): 1–18. doi:https://doi.org/10.1186/2192-1962-3-12.
- Australian Defence Force. 2018. ADF Concept for Command and Control of the Future Force. Canberra, Australia: Commonwealth of Australia.
- Autor, D. H. 2015. “Why Are There Still So Many Jobs? The History and Future of Workplace Automation.” Journal of Economic Perspectives 29 (3): 3–30. doi:https://doi.org/10.1257/jep.29.3.3.
- Bainbridge, L. 1983. “Ironies of Automation.” Automatica 19 (6): 775–779. doi:https://doi.org/10.1016/0005-1098(83)90046-8.
- Baumeister, R. F., and M. R. Leary. 1997. “Writing Narrative Literature Reviews.” Review of General Psychology 1 (3): 311–320. doi:https://doi.org/10.1037/1089-2680.1.3.311.
- Behymer, K. J., and J. M. Flach. 2016. “From Autonomous Systems to Sociotechnical Systems: Designing Effective Collaborations.” She Ji: The Journal of Design, Economics, and Innovation 2 (2): 105–114.
- Bentley, T., N. Green, D. Tappin, and R. Haslam. 2021. “State of Science: The Future of Work - Ergonomics and Human Factors Contributions to the Field.” Ergonomics 64 (4): 427–439.
- Bisantz, A. M., E. Roth, B. Brickman, L. L. Gosbee, L. Hettinger, and J. McKinney. 2003. “Integrating Cognitive Analyses in a Large-Scale System Design Process.” International Journal of Human-Computer Studies 58 (2): 177–206. doi:https://doi.org/10.1016/S1071-5819(02)00130-1.
- Bissell, D., T. Birtchnell, A. Elliott, and E. L. Hsu. 2020. “Autonomous Automobilities: The Social Impacts of Driverless Vehicles.” Current Sociology 68 (1): 116–134. doi:https://doi.org/10.1177/0011392118816743.
- Boden, M. A. 1998. “Creativity and Artificial Intelligence.” Artificial Intelligence 103 (1–2): 347–356. doi:https://doi.org/10.1016/S0004-3702(98)00055-1.
- Boden, M. A. 2016. AI: Its Nature and Future. New York: Oxford University Press (Incorporated).
- Boden, M., J. Bryson, D. Caldwell, K. Dautenhahn, L. Edwards, S. Kember, P. Newman, V. Parry, G. Pegman, T. Rodden, T. Sorrell, M. Wallis, B. Whitby, and A. Winfield. 2017. “Principles of Robotics: Regulating Robots in the Real World.” Connection Science 29 (2): 124–129. doi:https://doi.org/10.1080/09540091.2016.1271400.
- Brady, A., and N. Naikar. 2020. “An approach for modelling sociotechnical influences in mixed human-artificial agent workforce design [Extended abstract].” Proceedings of the Ergonomics and Human Factors 2020 Virtual Conference in Conjunction with the 13th International Organisational Design and Management Conference. Loughborough, UK: CIEHF.
- Brady, M. 2019. “Is Australian Law Adaptable to Automated Vehicles?” Griffith Journal of Law & Human Dignity : 35–71. [Special Issue].
- Branford, K., N. Naikar, and A. Hopkins. 2009. “Guidelines for AcciMap Analysis.” In Learning from High Reliability Organisations, edited by A. Hopkins, 193–212. Sydney, Australia: CCH Australia.
- Burch, G. F., A. A. Bennett, R. H. Humphrey, J. H. Batchelor, and A. H. Cairo. 2016. “Unraveling the Complexities of Empathy Research: A Multi-Level Model of Empathy in Organizations.” In Research on Emotions in Organizations: Emotions and Organizational Governance, edited by N. M. Ashkanasy, C. E. J. Härtel, and W. J. Zerbe, Vol. 12, 169–189. Bingley, UK: Emerald Group Publishing Limited.
- Burrell, J. 2016. “How the Machine ‘Thinks’: Understanding Opacity in Machine Learning Algorithms.” Big Data & Society 3 (1): 205395171562251. doi:https://doi.org/10.1177/2053951715622512.
- Calvo, R. A., D. Peters, K. Vold, and R. M. Ryan. 2020. “Supporting Human Autonomy in AI Systems: A Framework for Ethical Enquiry.” In Ethics of Digital Well-Being: A Multidisciplinary Approach, edited by, C. Burr and L. Floridi, Vol. 140, 31–54. Cham: Springer.
- Carayon, P. 2006. “Human Factors of Complex Sociotechnical Systems.” Applied Ergonomics 37 (4): 525–535. doi:https://doi.org/10.1016/j.apergo.2006.04.011.
- Carayon, P., P. Hancock, N. Leveson, I. Noy, L. Sznelwar, and G. van Hootegem. 2015. “Advancing a Sociotechnical Systems Approach to Workplace safety-developing the conceptual framework.” Ergonomics 58 (4): 548–564.
- Carroll, J. M., and M. B. Rosson. 1992. “Getting Around the Task-Artifact Cycle: How to Make Claims and Design by Scenario.” ACM Transactions on Information Systems 10 (2): 181–212. doi:https://doi.org/10.1145/146802.146834.
- Casner, S. M., R. W. Geven, M. P. Recker, and J. W. Schooler. 2014. “The Retention of Manual Flying Skills in the Automated Cockpit.” Human Factors 56 (8): 1506–1516.
- Cath, C., S. Wachter, B. Mittelstadt, M. Taddeo, and L. Floridi. 2017. “Artificial Intelligence and the ‘Good Society’: The US, EU, and UK Approach.” Science Engineering and Ethics 24: 505–528.
- Cave, S., and S. S. ÓhÉigeartaigh. 2018. “An AI Race for Strategic Advantage: Rhetoric and Risks.” AI Ethics and Society 1.
- Clarke, R. 2019. “Regulatory Alternatives for AI.” Computer Law & Security Review 35 (4): 398–409. doi:https://doi.org/10.1016/j.clsr.2019.04.008.
- Cockburn, I. M., R. Henderson, and S. Stern. 2018. “The Impact of Artificial Intelligence on Innovation: An Exploratory Analysis.” In The Economics of Artificial Intelligence: An Agenda, edited by A. Agrawal, J. Gans, and A. Goldfarb. Chicago, IL: University of Chicago Press.
- Coeckelbergh, M. 2020. “Artificial Intelligence, Responsibility Attribution, and a Relational Justification of Explainability.” Science and Engineering Ethics 26 (4): 2051–2068. doi:https://doi.org/10.1007/s11948-019-00146-8.
- Cong, B. 2019. When Disaster Strikes. Assessing the Royal Australian Navy’s Preparedness for Humanitarian Assistance and Disaster Relief. Fyshwick, Australia: Sea Power Centre.
- Dallat, C., P. M. Salmon, and N. Goode. 2018. “Identifying Risks and Emergent Risks Across Sociotechnical Systems: The NETworked Hazard Analysis and Risk Management System (NET-HARMS).” Theoretical Issues in Ergonomics Science 19 (4): 456–482. doi:https://doi.org/10.1080/1463922X.2017.1381197.
- Davis, S. H. H. 2019. “The Legal Personality of the Commonwealth of Australia.” Federal Law Review 47 (1): 3–30.
- Dawson, D., E. Schleiger, J. Horton, J. McLaughlin, C. Robinson, G. Quezada, J. Scowcroft, and S. Hajkowicz. 2019. Artificial Intelligence: Australia’s Ethics Framework. Sydney, Australia: Data61 CSIRO.
- Dekker, S. W. A., P. A. Hancock, and P. Wilkin. 2013. “Ergonomics and Sustainability: Towards an Embrace of Complexity and Emergence.” Ergonomics 56 (3): 357–364.
- Demerouti, E., A. B. Bakker, F. Nachreiner, and W. B. Schaufeli. 2001. “The Job Demands—Resources Model of Burnout.” Journal of Applied Psychology 86 (3): 499–512. doi:https://doi.org/10.1037/0021-9010.86.3.499.
- de Visser, E. J., S. S. Monfort, R. McKendrick, M. A. B. Smith, P. E. McKnight, F. Krueger, and R. Parasuraman. 2016. “Almost Human: Anthropomorphism Increases Trust Resilience in Cognitive Agents.” Journal of Experimental Psychology. Applied 22 (3): 331–349.
- Devitt, K., M. Gan, J. Scholz, and R. Bolia. 2020. A Method for Ethical AI in Defence. DSTG Technical Report (DSTG-TR-3786). Canberra, Australia: Defence Science & Technology Group.
- Dignum, V. 2017. “Responsible Artificial Intelligence: Designing AI for Human Values.” ITU Journal: ICT Discoveries: 1-8. [Special Issue].
- Dolata, U. 2008. The Transformative Capacity of New Technologies. How Innovations Affect Sectoral Change: Conceptual Considerations. MPIfG Discussion Paper (08/2). Cologne, Germany: Max Plank Institute for the Study of Societies.
- Dolata, U. 2013. The Transformative Capacity of New Technologies: A Theory of Sociotechnical Change. New York: Routledge.
- Drury, C. G. 2008. “The Future of Ergonomics/the Future of Work: 45 Years After Bartlett (1962).” Ergonomics 51 (1): 14–20. doi:https://doi.org/10.1080/00140130701800894.
- Dul, J., R. Bruder, P. Buckle, P. Carayon, P. Falzon, W. S. Marras, J. R. Wilson, and B. van der Doelen. 2012. “A Strategy for Human Factors/Ergonomics: Developing the Discipline and Profession.” Ergonomics 55 (4): 377–395. doi:https://doi.org/10.1080/00140139.2012.661087.
- Ekelhof, M. A. C. 2018. “Lifting the Fog of Targeting: “Autonomous Weapons” and Human Control Through the Lens of Military Targeting.” Naval War College Review 71 (3): 61–94.
- Elix, B., and N. Naikar. 2021. “Designing for Adaptation in Workers’ Individual Behaviors and Collective Structures with Cognitive Work Analysis: Case Study of the Diagram of Work Organization Possibilities.” Human Factors: The Journal of the Human Factors and Ergonomics Society 63 (2): 274–295. doi:https://doi.org/10.1177/0018720819893510.
- Flach, J., D. Schwartz, A. Bennett, S. Russell, and T. Hughes. 2008. “Integrated Constraint Evaluation: A Framework for Continuous Work Analysis.” In Applications of Cognitive Work Analysis, edited by A. M. Bisantz and C. M. Burns, 273–297. Boca Raton, FL: CRC Press; London: Taylor & Francis Group.
- Fleming, C. H., and N. G. Leveson. 2016. “Early Concept Development and Safety Analysis of Future Transportation Systems.” IEEE Transactions on Intelligent Transportation Systems 17 (12): 3512–3523. doi:https://doi.org/10.1109/TITS.2016.2561409.
- Floridi, L., J. Cowls, M. Beltrametti, R. Chatila, P. Chazerand, V. Dignum, C. Luetge, R. Madelin, U. Pagallo, F. Rossi, B. Schafer, P. Valcke, and E. Vayena. 2018. “AI4People—an Ethical Framework for a Good AI Society: Opportunities, Risks, Principles, and Recommendations.” Minds and Machines 28 (4): 689–707.
- Furman, J., and R. Seamans. 2019. “AI and the Economy.” Innovation Policy and the Economy 19: 139–161–191. doi:https://doi.org/10.1086/699936.
- Gasser, U., and V. A. F. Almeida. 2017. “A Layered Model for AI Governance.” IEEE Internet Computing 21 (6): 58–62. doi:https://doi.org/10.1109/MIC.2017.4180835.
- Gerke, S., T. Minssen, and G. Cohen. 2020. “Ethical and Legal Challenges of Artificial Intelligence-Driven Healthcare.” In Artificial Intelligence in Healthcare, edited by A. Bohr and K. Memarzadeh, 295–336. Cambridge, MA: Academic Press.
- Grossi, D., L. Royakkers, and F. Dignum. 2007. “Organizational Structure and Responsibility: An Analysis in a Dynamic Logic of Organized Collective Agency.” Artificial Intelligence and Law 15 (3): 223–249. doi:https://doi.org/10.1007/s10506-007-9054-0.
- Gula, A., C. Ellis, S. Bhattacharya, and L. Fiondella. 2020. “Software and System Reliability Engineering for Autonomous Systems Incorporating Machine Learning.” Annual Reliability and Maintainability Symposium (RAMS). Piscataway, NJ: IEEE.
- Hancock, P. A. 2019. “Some Pitfalls in the Promises of Automated and Autonomous Vehicles.” Ergonomics 62 (4): 479–495. doi:https://doi.org/10.1080/00140139.2018.1498136.
- Hand, D. J., and S. Khan. 2020. “Validating and Verifying AI Systems.” Patterns (New York, N.Y.) 1 (3): 100037. doi:https://doi.org/10.1016/j.patter.2020.100037.
- Harley, B. 1999. “The Myth of Empowerment: Work Organisation, Hierarchy and Employee Autonomy in Contemporary Australian Workplaces.” Work, Employment, & Society 13 (1): 41–66. doi:https://doi.org/10.1177/09500179922117782.
- Hoffman, R. R. 2017. “A Taxonomy of Emergent Trusting in the Human–Machine Relationship.” In Cognitive Systems Engineering: The Future for a Changing World, edited by P. J. Smith and R. R. Hoffman, 137–163. Boca Raton, FL: Taylor & Francis.
- Hollnagel, E. 2007. “From Overcoming Limitations to Securing Safety: Defining the New Mission of Human-Machine Systems.” IFAC Proceedings Volumes 40 (16): 23–30. doi:https://doi.org/10.3182/20070904-3-KR-2922.00005.
- Hollnagel, E. 2011. “The Diminishing Relevance of Human-Machine Interaction.” In The Handbook of Human-Machine Interaction: A Human-Centered Design Approach, edited by G. A. Boy, 417–429. New York: CRC Press.
- Horowitz, M. C., and P. Scharre. 2015. Meaningful Human Control in Weapons Systems: A Primer [Working Paper]. Washington, DC: Center for New American Security.
- Huang, M., R. Rust, and V. Maksimovic. 2019. “The Feeling Economy: Managing in the Next Generation of Artificial Intelligence (AI).” California Management Review 61 (4): 43–65. doi:https://doi.org/10.1177/0008125619863436.
- Johnson, D. G. 2015. “Technology with No Human Responsibility?” Journal of Business Ethics 127 (4): 707–715. doi:https://doi.org/10.1007/s10551-014-2180-1.
- Kaber, D. 2018. “Issues in Human–Automation Interaction Modeling: Presumptive Aspects of Frameworks of Types and Levels of Automation.” Journal of Cognitive Engineering and Decision Making 12 (1): 7–24. doi:https://doi.org/10.1177/1555343417737203.
- Karasek, R., and T. Theorell. 1990. Healthy Work: Stress, Productivity, and the Reconstruction of Working Life. New York: Basic Books.
- Kerasidou, A. 2020. “Artificial Intelligence and the Ongoing Need for Empathy, Compassion and Trust in Healthcare.” Bulletin of the World Health Organization 98 (4): 245–250. doi:https://doi.org/10.2471/BLT.19.237198.
- Khan, M. A., and L. S. Law. 2018. “The Role of National Cultures in Shaping the Corporate Management Cultures: A Three-Country Theoretical Analysis.” In Organizational Culture, edited by J. Vveinhardt. London, UK: IntechOpen.
- Klein, G., D. D. Woods, J. M. Bradshaw, R. R. Hoffman, and P. J. Feltovich. 2004. “Ten Challenges for Making Automation a “Team Player” in Joint Human-Agent Activity.” IEEE Intelligent Systems 19 (06): 91–95. doi:https://doi.org/10.1109/MIS.2004.74.
- Klein, G. cited in Colmer, A. 2020. Agile Command and Control Insights Paper. Emerging Disruptive Assessment Symposium. Canberra, Australia: Department of Defence, Commonwealth of Australia.
- Kleinlogel, E. P., and J. Dietz. 2014. “Ethical Decision Making in Organizations: The Role of Empathy.” In Organizing Through Empathy, edited by K. Pavlovich and K. Krahnke, 115–130. Oxon, UK: Routledge.
- Kroll, J. A. 2018. “The Fallacy of Inscrutability.” Philosophical Transactions of the Royal Society A 376: 20180084.
- Lange-Morales, K., A. Thatcher, and G. García-Acosta. 2014. “Towards a Sustainable World through Human Factors and Ergonomics: It is All About Values.” Ergonomics 57 (11): 1603–1615.
- Langfred, C. W., and N. A. Moye. 2004. “Effects of Task Autonomy on Performance: An Extended Model considering Motivational, Informational, and Structural Mechanisms.” Journal of Applied Psychology 89 (6): 934–945. doi:https://doi.org/10.1037/0021-9010.89.6.934.
- Langfred, C. W., and K. W. Rockmann. 2016. “The Push and Pull of Autonomy: The Tension between Individual Autonomy and Organizational Control in Knowledge Work.” Group & Organization Management 41 (5): 629–657. doi:https://doi.org/10.1177/1059601116668971.
- Law, J., and V. Singleton. 2013. “ANT and Politics: Working in and on the World.” Qualitative Sociology 36 (4): 485–502. doi:https://doi.org/10.1007/s11133-013-9263-7.
- Leahy, S. M., C. Holland, and F. Ward. 2019. “The Digital Frontier: Envisioning Future Technologies Impact on the Classroom.” Futures 113: 102422. doi:https://doi.org/10.1016/j.futures.2019.04.009.
- Lehne, M., J. Sass, A. Essenwanger, J. Schepers, and S. Thun. 2019. “Why Digital Medicine Depends on Interoperability.” NPJ Digital Medicine 2: 79. doi:https://doi.org/10.1038/s41746-019-0158-1.
- Leveringhaus, A. 2018. “Developing Robots: The Need for an Ethical Framework.” European View 17 (1): 37–43. doi:https://doi.org/10.1177/1781685818761016.
- Leveson, N. 2004. “A New Accident Model for Engineering Safer Systems.” Safety Science 42 (4): 237–270. doi:https://doi.org/10.1016/S0925-7535(03)00047-X.
- Luengo-Oroz, M., K. Hoffman Pham, J. Bullock, R. Kirkpatrick, A. Luccioni, S. Rubel, C. Wachholz, M. Chakchouk, P. Biggs, T. Nguyen, T. Purnat, and B. Mariano. 2020. “Artificial Intelligence Cooperation to Support the Global Response to COVID-19.” Nature Machine Intelligence 2 (6): 295–297. doi:https://doi.org/10.1038/s42256-020-0184-3.
- Makarius, E. E., D. Mukherjee, J. D. Fox, and A. K. Fox. 2020. “Rising with the Machines: A Sociotechnical Framework for Bringing Artificial Intelligence into the Organization.” Journal of Business Research 120: 262–273. doi:https://doi.org/10.1016/j.jbusres.2020.07.045.
- Mikalef, P., S. O. Fjørtoft, and H. Y. Torvatn. 2019. “Developing an Artificial Intelligence Capability: A Theoretical Framework for Business Value.” In Business Information Systems Workshops, edited by W. Abramowicz, and R. Corchuelo, Vol 373, 409–416. Cham: Springer.
- Militello, L. G., E. M. Roth, S. Scheff, K. Ernst, C. Sushereba, and D. Klein. 2019. Crew Configuration Analysis for Future Airborne Reconnaissance Operations. Cincinnati, OH: Applied Decision Science, LLC.
- Misselhorn, C. 2018. “Artificial Morality. Concepts, Issues and Challenges.” Society 55 (2): 161–169. doi:https://doi.org/10.1007/s12115-018-0229-y.
- Moray, N. 2000. “Culture, Politics and Ergonomics.” Ergonomics 43 (7): 858–868. doi:https://doi.org/10.1080/001401300409062.
- Mulgan, G. 2016. “A Machine Intelligence Commission for the UK: How to Grow Informed Public Trust and Maximise the Positive Impact of Smart Machines.” Nesta.
- Naikar, N. 2013. Work Domain Analysis: Concepts, Guidelines, and Cases. Boca Raton, FL: Taylor & Francis.
- Naikar, N., and B. Elix. 2016. “Integrated System Design: Promoting the Capacity of Sociotechnical Systems for Adaptation Through Extensions of Cognitive Work Analysis.” Frontiers in Psychology 7 (962): 962–964.
- Naikar, N., and B. Elix. 2021. “Designing for Self-Organisation in Sociotechnical Systems: Resilience Engineering, Cognitive Work Analysis, and the Diagram of Work Organisation Possibilities.” Cognition, Technology & Work 23 (1): 23–37. doi:https://doi.org/10.1007/s10111-019-00595-y.
- Naikar, N., and P. M. Sanderson. 2001. “Evaluating Design Proposals for Complex Systems with Work Domain Analysis.” Human Factors 43 (4): 529–542. doi:https://doi.org/10.1518/001872001775870322.
- Naikar, N., G. Moy, H. Kwok, and A. Brady. 2021. “Designing for “Agility” in Envisioned Worlds: Concepts for Collaborative Intelligence in Human-Machine Teams.” Naturalistic Decision Making and Resilience Engineering Symposium 2021. Toulouse, France: Naturalistic Decision Making, Resilience Engineering Association, and Fondation pour une culture de sécurité industrielle.
- Naikar, N., B. Pearce, D. Drumm, and P. M. Sanderson. 2003. “Designing Teams for First-of-a-Kind, Complex Systems Using the Initial Phases of Cognitive Work Analysis: Case Study.” Human Factors 45 (2): 202–217. doi:https://doi.org/10.1518/hfes.45.2.202.27236.
- National Transportation Safety Board. 2019. Assumptions Used in the Safety Assessment Process and the Effects of Multiple Alerts and Indications on Pilot Performance. Washington, DC: National Transportation Safety Board.
- Nishant, R., M. Kennedy, and J. Corbett. 2020. “Artificial Intelligence for Sustainability: Challenges, Opportunities, and a Research Agenda.” International Journal of Information Management 53: 102104. doi:https://doi.org/10.1016/j.ijinfomgt.2020.102104.
- Parasuraman, R., and C. D. Wickens. 2008. “Humans: Still Vital after All These Years of Automation.” Human Factors: The Journal of the Human Factors and Ergonomics Society 50 (3): 511–520. doi:https://doi.org/10.1518/001872008X312198.
- Parker, S. K., and G. Grote. 2020. “Automation, Algorithms, and beyond: Why Work Design Matters More than Ever in a Digital World.” Applied Psychology: 1–45. doi:https://doi.org/10.1111/apps.12241.
- Parkinson, S., P. Ward, K. Wilson, and J. Miller. 2017. “Cyber Threats Facing Autonomous and Connected Vehicles: Future Challenges.” IEEE Transactions on Intelligent Transportation Systems 18 (11): 2898–2915. doi:https://doi.org/10.1109/TITS.2017.2665968.
- Perrow, C. 1984. Normal Accidents: Living with High-Risk Technologies. New York: Basic Books.
- Petit, N. 2017. Law and Regulation of Artificial Intelligence and Robots: Conceptual Framework and Normative Implications. Rochester, NY. [Working paper].
- Pradhan, M., A. Tiderko, and D. Ota. 2017. “Approach towards achieving interoperability between military land vehicle and robotic systems.” Proceedings of the International Conference on Military Communications and Information Systems, 1–7. Atlanta, GA.
- Pumplun, L., C. Tauchert, and M. Heidt. 2019. “A new organizational chassis for artificial intelligence – exploring organizational readiness factors.” Proceedings of the 27th European Conference on Information Systems (ECIS), Stockholm & Uppsala, Sweden.
- Rasmussen, J. 1969. Man-Machine Communication in the Light of Accident Records (Report S-1-69). Roskilde, Denmark: Danish Atomic Energy Commission, Research Establishment Risø.
- Rasmussen, J. 1986. Information Processing and Human-Machine Interaction: An Approach to Cognitive Engineering. New York: North-Holland.
- Rasmussen, J. 1997. “Risk Management in a Dynamic Society: A Modelling Problem.” Safety Science 27 (2-3): 183–213. doi:https://doi.org/10.1016/S0925-7535(97)00052-0.
- Rasmussen, J., A. M. Pejtersen, and L. P. Goodstein. 1994. Cognitive Systems Engineering. New York: John Wiley & Sons.
- Rasmussen, J., and I. Svedung. 2000. Proactive Risk Management in a Proactive Society. Karlstad: Swedish Rescue Services Agency.
- Reason, J. 1990. Human Error. Cambridge: Cambridge University Press.
- Relling, T., M. LüTzhöft, R. Ostnes, and H. P. Hildre. 2018. “A Human Perspective on Maritime Autonomy.” In Augmented Cognition: Users and Contexts, edited by D. D. Schmorrow & C. M. Fidopiastic, 350–362. Cham: Springer.
- Rodrigues, R. 2020. “Legal and Human Rights Issues of AI: Gaps, Challenges and Vulnerabilities.” Journal of Responsible Technology 4: 100005. doi:https://doi.org/10.1016/j.jrt.2020.100005.
- Romme, A. G. L. 2019. “Climbing up and down the Hierarchy of Accountability: Implications for Organization Design.” Journal of Organization Design 8 (1): 1–14. doi:https://doi.org/10.1186/s41469-019-0060-y.
- Roth, E. M., E. P. DePass, R. Scott, R. Truxler, S. F. Smith, and J. L. Wampler. 2017. “Designing Collaborative Planning Systems: Putting Joint Cognitive Systems Principles to Practice.” In Cognitive Systems Engineering: The Future for a Changing World, edited by P. J. Smith and R.R. Hoffman, 247–268. London, UK: Taylor & Francis.
- Roth, E., B. DePass, J. Harter, R. Scott, and J. Wampler. 2018. “Beyond Levels of Automation: Developing More Detailed Guidance for Human Automation Interaction Design.” Proceedings of the Human Factors and Ergonomics Society Annual Meeting 62 (1): 150–154. doi:https://doi.org/10.1177/1541931218621035.
- Rouse, W. B. 1991. Design for Success: A Human-Centered Approach to Designing Successful Products and Systems. New York: Wiley.
- Russell, S., D. Dewey, and M. Tegmark. 2015. “Research Priorities for Robust and Beneficial Artificial Intelligence.” AI Magazine 36 (4): 105–114. doi:https://doi.org/10.1609/aimag.v36i4.2577.
- Ryan, R. M., and E. L. Deci. 2000. “Self-Determination Theory and the Facilitation of Intrinsic Motivation, Social Development, and Well-Being.” American Psychologist 55 (1): 68–78. doi:https://doi.org/10.1037/0003-066X.55.1.68.
- Salmon, P. M., M. Cornelissen, and M. J. Trotter. 2012. “Systems-Based Accident Analysis Methods: A Comparison of Accimap, HFACS, and STAMP.” Safety Science 50 (4): 1158–1170. doi:https://doi.org/10.1016/j.ssci.2011.11.009.
- Sandry, E. 2015. “Re-Evaluating the Form and Communication of Social Robots.” International Journal of Social Robotics 7 (3): 335–346. doi:https://doi.org/10.1007/s12369-014-0278-3.
- Schaefer, K. E., J. Y. C. Chen, J. L. Szalma, and P. A. Hancock. 2016. “A Meta-Analysis of Factors Influencing the Development of Trust in Automation: Implications for Understanding Autonomy in Future Systems.” Human Factors 58 (3): 377–400.
- Scherer, M. U. 2016. “Regulating Artificial Intelligence Systems: Risks, Challenges, Competencies, and Strategies.” Harvard Journal of Law & Technology 29 (2): 353–400.
- Schuller, A. L. 2017. “At the Crossroads of Control: The Intersection of Artificial Intelligence in Autonomous Weapon Systems with International Humanitarian Law.” Harvard National Security Journal 8 : 379–425.
- Sehrawat, V. 2017. “Autonomous Weapon System: Law of Armed Conflict (LOAC) and Other Legal Challenges.” Computer Law & Security Review 33 (1): 38–56. doi:https://doi.org/10.1016/j.clsr.2016.11.001.
- Shaw, B., and O. Chisholm. 2020. “Creeping Through the Backdoor: Disruption in Medicine and Health.” Frontiers in Pharmacology 11: 818. doi:https://doi.org/10.3389/fphar.2020.00818.
- Shirani, A. 2019. “Upskilling and Retraining in Data Analytics: A Skill-Adjacency Analysis for Career Paths.” Issues in Information Systems 20 (4): 65–74.
- Shrestha, Y. R., S. M. Ben-Menahem, and G. von Krogh. 2019. “Organizational Decision-Making Structures in the Age of Artificial Intelligence.” California Management Review 61 (4): 66–83. doi:https://doi.org/10.1177/0008125619862257.
- Sjöberg, L., and B.-M. Drottz-Sjöberg. 2001. “Fairness, Risk and Risk Tolerance in the Siting of a Nuclear Waste Repository.” Journal of Risk Research 4 (1): 75–101. doi:https://doi.org/10.1080/136698701456040.
- Smids, J., S. Nyholm, and H. Berkers. 2020. “Robots in the Workplace: A Threat to—or Opportunity for—Meaningful Work?” Philosophy & Technology 33 (3): 503–522. doi:https://doi.org/10.1007/s13347-019-00377-4.
- Spiekermann, S., J. Korunovska, and M. Langheinrich. 2019. “Inside the Organization: Why Privacy and Security Engineering is a Challenge for Engineers.” Proceedings of the IEEE 107 (3): 600–615. doi:https://doi.org/10.1109/JPROC.2018.2866769.
- Stanton, N. A., and C. Harvey. 2017. “Beyond Human Error Taxonomies in Assessment of Risk in Sociotechnical Systems: A New Paradigm with the EAST ‘Broken-Links’ Approach.” Ergonomics 60 (2): 221–233. doi:https://doi.org/10.1080/00140139.2016.1232841.
- Stanton, N. A., P. M. Salmon, L. A. Rafferty, G. H. Walker, C. Baber, and D. P. Jenkins. 2013. Human Factors Methods: A Practical Guide for Engineering and Design. London, UK: CRC Press.
- Strauch, B. 2018. “Ironies of Automation: Still Unresolved After All These Years.” IEEE Transactions on Human-Machine Systems 48 (5): 419–433. doi:https://doi.org/10.1109/THMS.2017.2732506.
- Streitz, N. 2019. “Beyond ‘Smart-Only’ Cities: Redefining the ‘Smart-Everything’ Paradigm.” Journal of Ambient Intelligence and Humanized Computing 10 (2): 791–812. doi:https://doi.org/10.1007/s12652-018-0824-1.
- Streitz, N., D. Charitos, M. Kaptein, and M. Böhlen. 2019. “Grand Challenges for Ambient Intelligence and Implications for Design Contexts and Smart Societies.” Journal of Ambient Intelligence and Smart Environments 11 (1): 87–107. doi:https://doi.org/10.3233/AIS-180507.
- Svedung, I., and J. Rasmussen. 2002. “Graphic Representation of Accident Scenarios: Mapping System Structure and the Causation of Accidents.” Safety Science 40 (5): 397–417. doi:https://doi.org/10.1016/S0925-7535(00)00036-9.
- Taebi, B. 2017. “Bridging the Gap Between Social Acceptance and Ethical Acceptability.” Risk Analysis: An Official Publication of the Society for Risk Analysis 37 (10): 1817–1827. doi:https://doi.org/10.1111/risa.12734.
- Thatcher, A., R. Nayak, and P. Waterson. 2020. “Human Factors and Ergonomics Systems-Based Tools for Understanding and Addressing Global Problems of the Twenty-First Century.” Ergonomics 63 (3): 367–387.
- Thatcher, A., P. Waterson, A. Todd, and N. Moray. 2018. “State of Science: Ergonomics and Global Issues.” Ergonomics 61 (2): 197–213.
- Theodorou, A., and V. Dignum. 2020. “Towards Ethical and Socio-Legal Governance in AI.” Nature Machine Intelligence 2 (1): 10–12. doi:https://doi.org/10.1038/s42256-019-0136-y.
- Tieu, A. M., and R. Ong. 2021. Visualisation Library: An Accessible, Customisable and Extensible Information Visualisation Suite for Defence. Canberra, Australia: Modelling and Simulation Society of Australia and New Zealand Inc. Manuscript submitted for publication.
- Torresen, J. 2018. “A Review of Future and Ethical Perspectives of Robotics and AI.” Frontiers in Robotics and AI 4: 75. doi:https://doi.org/10.3389/frobt.2017.00075.
- Trotter, M., P. M. Salmon, and M. G. Lenné. 2014. “Impromaps: Applying Rasmussen’s Risk Management Framework to Improvisation Incidents.” Safety Science 64: 60–70. doi:https://doi.org/10.1016/j.ssci.2013.11.021.
- Truxler, R., E. Roth, R. Scott, S. Smith, and J. Wampler. 2012. “Designing Collaborative Automated Planners for Agile Adaptation to Dynamic Change.” Proceedings of the Human Factors and Ergonomics Society Annual Meeting 56 (1): 223–227. doi:https://doi.org/10.1177/1071181312561065.
- Tubella, A. A., A. Theodorou, F. Dignum, and V. Dignum. 2019. “Governance by Glass-Box: Implementing Transparent Moral Bounds for AI Behaviour.” Proceedings of the Twenty-Eighth International Joint Conference on Artificial Intelligence (IJCAI-19), 5787–5793. San Francisco, CA.
- United Nations Educational, Scientific and Cultural Organization. 2005. “Universal declaration on bioethics and human rights.” http://portal.unesco.org/en/ev.phpURL_ID=31058&URL_DO=DO_TOPIC&URL_SECTION=201.html
- United Nations. 2018. “Report of the 2018 session of the group of governmental experts on emerging technologies in the area of lethal autonomous weapons systems.” https://www.unog.ch/80256EDD006B8954/(httpAssets)/20092911F6495FA7C125830E003F9A5B/$file/CCW_GGE.1_2018_3_final.pdf
- Vicente, K. 1999. Cognitive Work Analysis: Toward Safe, Productive, and Healthy Computer-Based Work. Boca Raton, FL: CRC Press.
- Vicente, K. 2004. The Human Factor: Revolutionizing the Way People Live with Technology. New York: Routledge.
- Vicente, K. J. 2002. “Ecological Interface Design: Progress and Challenges.” Human Factors 44 (1): 62–78. doi:https://doi.org/10.1518/0018720024494829.
- Waterson, P., D. P. Jenkins, P. M. Salmon, and P. Underwood. 2017. “Remixing Rasmussen’: The Evolution of Accimaps within Systemic Accident Analysis.” Applied Ergonomics 59 (Pt B): 483–503.
- Waterson, P., M. M. Robertson, N. J. Cooke, L. Militello, E. Roth, and N. A. Stanton. 2015. “Defining the Methodological Challenges and Opportunities for an Effective Science of Sociotechnical Systems and Safety.” Ergonomics 58 (4): 565–599.
- Whitby, B. 2015. “Automating Medicine the Ethical Way.” In Machine Medical Ethics. Intelligence Systems, Control and Automation: Science and Engineering, edited by S. van Rysewyk and M. Pontier, Vol. 74, 223–232. Cham: Springer.
- Wilkin, P. 2010. “The Ideology of Ergonomics.” Theoretical Issues in Ergonomics Science 11 (3): 230–244. doi:https://doi.org/10.1080/14639220802609895.
- Wilson, J. R. 2014. “Fundamentals of Systems Ergonomics/Human Factors.” Applied Ergonomics 45 (1): 5–13. doi:https://doi.org/10.1016/j.apergo.2013.03.021.
- Winfield, A. 2019. “Ethical Standards in Robotics and AI.” Nature Electronics 2 (2): 46–48. doi:https://doi.org/10.1038/s41928-019-0213-6.
- Winfield, A. F. T., and M. Jirotka. 2018. “Ethical Governance is Essential to Building Trust in Robotics and Artificial Intelligence Systems.” Philosophical Transactions of the Royal Society A 376: 1–13.
- Winkler, J. D., T. Marler, M. N. Posard, R. S. Cohen, and M. L. Smith. 2019. Reflections on the Future Warfare and Implications for Personnel Policies of the US Department of Defence. Santa Monica, CA: RAND Corporation.
- Wirtz, B. W., J. C. Weyerer, and C. Geyer. 2019. “Artificial Intelligence and the Public Sector—Applications and Challenges.” International Journal of Public Administration 42 (7): 596–615. doi:https://doi.org/10.1080/01900692.2018.1498103.
- Wong-Rieger, D., and F. Rieger. 1989. “The Influence of Societal Culture on Corporate Culture, Business Strategy, and Performance in the International Airline Industry.” In Organizational Science Abroad, edited by C. A. B. Osigweh, 229–265. Boston, MA: Springer.
- Woods, D., and S. Dekker. 2000. “Anticipating the Effects of Technological Change: A New Era of Dynamics for Human Factors.” Theoretical Issues in Ergonomics Science 1 (3): 272–282. doi:https://doi.org/10.1080/14639220110037452.
- Wright, P., A. Dearden, and B. Fields. 2000. “Function Allocation: A Perspective from Studies of Work Practice.” International Journal of Human-Computer Studies 52 (2): 335–355. doi:https://doi.org/10.1006/ijhc.1999.0292.
- Wright, R. 2000. Nonzero: The Logic of Human Destiny. New York, NY: Vintage Books.
- Wu, C.-H., M. A. Griffin, and S. K. Parker. 2015. “Developing Agency through Good Work: Longitudinal Effects of Job Autonomy and Skill Utilization on Locus of Control.” Journal of Vocational Behavior 89: 102–108. doi:https://doi.org/10.1016/j.jvb.2015.05.004.
- Yampolskiy, R. 2020. On Controllability of AI. Louisville, KY: University of Louisville. https://philarchive.org/archive/YAMOCO.