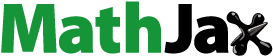
Abstract
Vibrotactile feedback training may be used as a complementary strategy to reduce time in demanding postures in manual handling. This study evaluated the short- and medium-term effects of concurrent posture-correction vibrotactile feedback training on trunk inclination exposure in real manual sorting work. Fifteen warehouse workers completed the training and the follow-up sessions. Trunk inclination angles were recorded using the ambulatory Smart Workwear System. Questionnaires were used for assessing system usability, perceived physical exertion, and work ability. The results showed reduced time in trunk inclination >30°, >45°, and >60°, and reductions in the 90th, 95th, and 99th percentile trunk inclination angles, when receiving feedback and immediately after feedback withdrawal. No significant reduction was retained after one and three weeks. The wearer's comfort was scored high, and the feedback did not increase the perceived cognitive demands. No significant effects attributed to changed trunk inclination exposure were observed for perceived physical exertion or work ability. The training program has the potential of contributing to reduced trunk inclination exposure in the short term. Future studies are needed to evaluate if improvements in the feedback training can transfer the short-term results to retained median- and long-term effects.
Practitioner summary: A two-day training program with concurrent posture-correction vibrotactile feedback can contribute to reduced exposure of trunk inclination in real manual sorting work in the short term. More research is needed on how to design the feedback training programs in order to be effective in the long term.
1. Introduction
Work-related Diseases and disorders constitute a major global health issue affecting societies, organisations, and individuals. The economic cost has been estimated to be 3.3% of the Gross Domestic Product in the EU and 3.9% globally (Tompa et al. Citation2019). Musculoskeletal disorders (MSDs) represent the largest category of work-related health disorders and account for 40% of the global compensation costs of occupational- and work-related accidents and diseases (ILO Citation2015). Globally, low back pain (LBP) attributed to work, has been estimated to yearly cause about 22 million disability-adjusted life years (Driscoll et al. Citation2014). Heavy and repetitive manual handling and awkward postures are considered risk factors for MSDs (Sluiter, Rest, and Frings-Dresen Citation2001; Lötters et al. Citation2003; van Rijn et al. Citation2010; Punnett Citation2014). The risk of developing chronic LBP is further exacerbated when manual handling occurs in combination with an inclination of the trunk (Holtermann et al. Citation2013). Further, trunk flexion >30° has been associated with LBP-related work absence when occurring more than 10% of the worktime (Hoogendoorn et al. Citation2002), and trunk flexion >45° has been associated with LBP when occurring more than 45 minutes per week (Jansen, Morgenstern, and Burdorf Citation2004). Despite this, manual handling remains frequent in the working population despite increased automation in the handling of goods (Eurofound Citation2012, Citation2016). Due to its low investment costs and high level of flexibility, manual handling may continue to be a common part of material handling processes for the foreseeable future, despite increased industrial automation (Baker and Halim Citation2007; Marchet, Melacini, and Perotti Citation2015). Due to the large burden of LBP and MSDs, measures targeting a reduction in biomechanical exposures related to manual handling are needed. Often, such measures focus on engineering controls and organisational aspects. As a complement, measures may additionally be introduced at the individual level targeting an increase in the physical capacity of the employees through strength-training programs or work technique training. The latter often comprise e.g. education on body mechanics and back care, and training on lifting technique and on mechanical lifting devices (Yassi et al. Citation2001; Denis et al. Citation2020; Sundstrup et al. Citation2020).
However, several systematic literature reviews have questioned the effectiveness of work technique training (Clemes, Haslam, and Haslam Citation2010; Verbeek et al. Citation2011; Hogan, Greiner, and O'sullivan Citation2014) to reduce MSDs. The lack of evidence for training effectiveness may partly be attributed to insufficient transfer of the training to retain behavioural changes (Hogan, Greiner, and O'sullivan Citation2014), and to the fact that previous reviews did not sufficiently consider the quality of the training itself (Denis et al. Citation2020). As proposed by Denis et al. (Citation2020) work technique training programs can be more effective if carried out in a real work setting. The training should also allow for practice in a range of work situations that represent the variability that workers usually are exposed to. Also, sufficient practice time is needed to enable a retained change in motor performance (motor learning) attributed to the training (Sigrist et al. Citation2013; Lind et al. Citation2020c).
In recent years, several systems have been developed which can generate automated risk assessments based on kinematics using accelerometers or inertial measurement units (IMUs) (Battini, Persona, and Sgarbossa Citation2014; Alberto et al. Citation2018; Huang et al. Citation2020; Stefana et al. Citation2021). Some of these systems have incorporated features that provide work technique training using augmented (extrinsic) feedback (Vignais et al. Citation2013; Lind, Forsman, and Rose Citation2020a). To date, various types of augmented feedback have been used, including visual, auditory or tactile feedback (Sigrist et al. Citation2013). In addition, the timing of feedback varies from terminal feedback, which is provided after a task sequence, to concurrent (real-time) feedback, which is provided directly (concurrent to) when certain preset threshold levels are exceeded. Augmented feedback has been used in a wide range of application areas such as sports (Galloway Citation2011; Kos and Umek Citation2018), rehabilitation (Ribeiro et al. Citation2011; Araujo, Scholl Schell, and Ribeiro Citation2017; Wang et al. Citation2017), computer work (Madeleine et al. Citation2006; de Korte et al. Citation2008), surveillance operations (Bazazan et al. Citation2019) and manual handling (Vignais et al. Citation2013; Lind et al. Citation2020c; Kamachi, Owlia, and Dutta Citation2021).
In a systematic literature review, Ribeiro et al. (Citation2011) found support for the effectiveness of augmented feedback in the prevention of low back pain (LBP), whilst there are still single studies reporting no significant effects on reducing MSDs prevalence (Meijer, Sluiter, and Frings-Dresen Citation2009) or discomfort (de Korte et al. Citation2008). Several studies indicate that posture-correction feedback (error amplification feedback), i.e. which provides feedback signals to the operator when performing undesired movements, can be effective to induce motor learning (Sigrist et al. Citation2013; Liu, Li, and Lamontagne Citation2018), especially among skilled users (Milot et al. Citation2010; Sigrist et al. Citation2013). However, concurrent correction feedback may hamper the bodýs intrinsic feedback system and can reinforce feedback dependence if provided too often or for too long (Lam et al. Citation2011; Ribeiro et al. Citation2011; Buchanan and Wang Citation2012; Patterson, Carter, and Hansen Citation2013). Doss et al. (Citation2018) studied the combined effect of verbal feedback and concurrent posture-correction audio feedback in three different types of simulated patient transfer tasks. The study included 10 female nursing students, and the audio feedback was provided when the forward trunk inclination angle exceeded 45°. Directly after feedback training, a significant mean reduction in the trunk inclination angle by 2.1–7.6° was observed for the different scenarios. Boocock et al. (Citation2019) used auditory feedback to reduce trunk flexion in a simulated lifting task. When compared to a control group, those receiving auditory feedback had significantly lower trunk flexion angles and which also remained lower during the entire 20-minute lifting session.
Lind et al. (Citation2020a) found, in comparison to baseline, significantly lower times with elevated arms in simulated post sorting during concurrent posture-correction vibrotactile feedback. Lind et al. (Citation2020c) studied the effect of concurrent posture-correction vibrotactile feedback on trunk inclination and arm elevation in simulated material kitting among industrial personnel. A 10-minute feedback training session reduced the accumulated time in trunk inclination significantly, both during and directly after training. Vignais et al. (Citation2013) evaluated concurrent feedback in manual operations simulating industrial manufacturing tasks. When compared to the control group, those receiving the augmented feedback significantly lowered their biomechanical load score derived from the RULA method (McAtamney and Corlett Citation1993). None of these studies did, however, evaluate the retained effects after one or several weeks, so the medium- and long-term effects of the feedback training are unknown. To assess the retained effects of feedback training, Agruss, Williams, and Fathallah (Citation2004) evaluated acceleration-based verbal and EMG-based audible feedback with a fading feedback schedule to reduce spinal compression force in a simulated lifting task. At the post-training session one week after the baseline, those participants receiving verbal feedback training at baseline had significantly lower spinal compression force during the post-training session when compared to the control group. In another study targeting a reduction in finger type force levels in computer typing tasks, Gerard et al. (Citation2002) observed a significant decline in finger type force levels one- and two weeks after the participants had received auditory feedback to reduce the finger force levels. In a recent laboratory-based study aiming at reducing peak spinal flexion in patient transfer using auditory feedback, Kamachi, Owlia, and Dutta (Citation2021) observed that the initial reduction in peak sagittal spinal flexion following the training remained after both 2 weeks and 2 months after the initial training. Hence, although limited there is some support that augmented feedback can contribute to sustained improvements in the work technique.
Previous research has demonstrated that both high perceived physical exertion and low work ability are related to adverse health outcomes. For example, a dose-response relation between perceived physical exertion and long-term sickness absence was observed among health care workers in which moderate and strenuous physical exertion were associated with long-term sickness absence at a hazard ratio of 1.28 and 1.53, respectively (Andersen et al. Citation2012). Work ability, as defined as the balance between the resources of individual workers and work demands, has been associated with sick leave and early retirement in various occupational groups when perceived as moderate to poor (Alavinia et al., Citation2009; Ahlström et al. Citation2010; Hallman et al. Citation2019). Lastly, the usability of such feedback systems regarding wearer comfort after multiple days and the impact on cognitive demand in a real work setting is still unknown.
Several research gaps can be identified in the literature. First, it remains unclear whether augmented feedback training is effective in real-life settings since many of the studies have been conducted on simulated single tasks (Breen, Nisar, and Olaighin Citation2009; Causo et al. Citation2011; Tino et al. Citation2011; Bark et al. Citation2015; Lind et al. Citation2020a; Kamachi, Owlia, and Dutta Citation2021) or multi-tasks (Vignais et al. Citation2013; Lind et al. Citation2020c). Second, while most studies have only tested the short-term retention effects (Vignais et al. Citation2013; Lind et al. Citation2020c), some studies suggest that improvements in reduced postural load can be retained over longer periods of time (Gerard et al. Citation2002; Agruss, Williams, and Fathallah Citation2004; Kamachi, Owlia, and Dutta Citation2021). Third, to the best of our knowledge, there is a lack of information on the effects of changes in postural load after a work technique training program on secondary health outcomes (e.g. perceived work ability and physical exertion).
The main aim of this study was to evaluate whether concurrent posture-correction vibrotactile feedback can be effective in reducing trunk forward inclination in real manual sorting work, when the feedback is received, immediately after the feedback training (the short-term effects), and about one and three weeks after the feedback training (the median-term effects). The second aim was to evaluate the usability of the system regarding the wearer's comfort and its impact on cognitive demands within the study period. The third aim was to evaluate whether changes in trunk inclination angle also result in improved work ability and reduced physical exertion within the study period.
2. Methods
An intervention study over a period of four weeks (September – October 2020) was conducted to investigate the short- and medium-term effects of concurrent posture-correction vibrotactile feedback training in a real work setting. On five workdays, the work postures of warehouse staff were monitored for up to one hour using a technical measurement system – the Smart Workwear System (Lind et al. Citation2019b; Lind et al. Citation2020a). On two training days, concurrent posture-correction vibrotactile feedback (hereafter referred to as vibrotactile feedback) was provided with the system when the participants bend their trunks forward above predefined threshold levels. In addition to work postures, the usability of the system was evaluated on two separate measurement days, and the intervention effect on physical exertion and work ability was evaluated on all measurement days.
2.1. Technical measurements – trunk postures
Trunk postures were measured with the Smart Workwear System vibrotactile feedback module (Lind et al. Citation2020a) which can be used to measure postural exposure as a basis for work design improvements and to provide feedback to facilitate work technique training (Lind et al. Citation2019b; Lind et al. Citation2020c). The vibrotactile feedback module system comprises a customised workwear t-shirt with embedded pockets, IMUs (LPMS-B2 IMU, LP Research, Tokyo, Japan, size: 39 × 39 × 8 mm, mass: 12 g), vibration units, an Android smartphone with the application ‘ErgoRiskLogger’. The system records and processes kinematics data from the IMUs in real-time and initiates vibrotactile feedback signals of the vibration motor units via Bluetooth (). A previous study found sufficient accuracy for trunk inclination measurement during forwarding bending and lifting tasks (Yang, Borgström, and Forsman Citation2019).
Figure 1. (A) Conceptual description of the Smart Workwear System vibrotactile feedback module including an IMU, a vibration unit, a smartphone device with the smartphone application. The black arrows indicate the data or feedback signal flow, and the white block arrows illustrate the wireless (Bluetooth) communication flow. (B) Photo of the system vibrotactile feedback module showing an IMU, a vibration unit, and the smartphone application ErgoRiskLogger. (C) Photo of the system on a person.
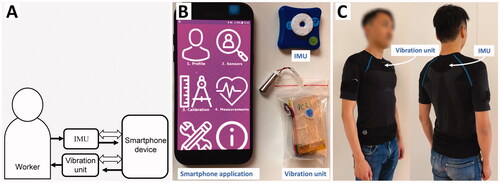
The feedback can be provided concurrently with less than half a second delay through the vibration motor unit or can be provided after a set time period (terminal feedback) such as every 1–10 minutes. For this study, the concurrent feedback option was used, and the vibrotactile feedback was provided for trunk postures only. The vibration unit was placed in an embedded pocket of the workwear t-shirt located at the sternum. The IMU to record trunk postures was placed in an embedded pocket located at the upper back at the level of the thoracic vertebrae 1–2 (Korshoj et al. Citation2014). The IMU was configured to a 25 Hz sampling frequency with the 3-axis accelerometer with a range of ±4 G and the 3-axis gyroscope with a range of ±500°/s, both recorded with a 16-bit resolution. The trunk sagittal inclination angle (flexion/extension) was calculated as the relative segment angle to the reference position (0°) in the sagittal plane by using the quaternion signals, which incorporated accelerometer and gyroscope data using the sensor's built-in fusion algorithm. The reference position was obtained before each measurement session having the participants standing straight in a relaxed position with the arms hanging down and the palms facing towards the body. Due to the likelihood of magnetic interference in industrial environments, the magnetometers in the IMU were disabled.
2.2. Questionnaires
2.2.1. System usability
Cognitive demands were measured at baseline and after the feedback training 2 when participants had used the system with feedback for two training sessions, using three items of the cognitive demand dimension from the Copenhagen Psychosocial Questionnaire II (COPSOQ II): ‘eyes on lots of things’, ‘remember a lot’ and ‘difficult decisions’. The items were measured on a five-level scale ranging from ‘always’ to ‘never’. Wearer comfort was measured on the follow-up 1 and 2 after a longer period of use of the system, using the Comfort Rating Scale (CRS) (Knight and Baber Citation2005), which includes the six dimensions: ‘emotion’, ‘attachment’, ‘harm’, ‘perceived change’, ‘movement’, and ‘anxiety’. The dimensions were measured on a 21-level scale with verbal anchors at each end: ‘low’ (0), and ‘high’ (20). The participants’ general experience of wearing the system and receiving vibration feedback was assessed after the feedback training 1 and 2 sessions using semi-open questions. The questions asked were targeted: whether the participants had perceived the vibrotactile feedback, their general opinion on the system, if they understood the purpose of the feedback, as well as whether and in what ways they experienced that the feedback had influenced their work technique. The answers to the semi-open questions were analysed by using content analysis (Hsieh and Shannon Citation2005). This design with a slightly varied (reduced) set of questions after each session was chosen in order to minimise survey fatigue.
2.2.2. Self-rated work ability, physical exertion, and productivity demands
On all measurement days, the participants rated their current work ability using the single-item question on work ability (Ahlström et al. Citation2010) where the current work ability is compared with the lifetime best on a scale from 0 (‘completely unable to work’) to 10 (‘work ability at its best’). Physical exertion was measured using the Borg RPE scale (Borg Citation1998) where the participants rated their physical exertion level from 6 to 20 for both the current workday and for a typical workday. In addition, self-rated productivity demands (hereafter referred to as productivity demands) were measured each measurement day by asking the participants to rate: ‘How was the amount of work today compared to a normal workday’, on a 7-level scale: from ‘much less’ (1), ‘less’ (2), ‘slightly less’ (3), ‘about the same’ (4) ‘slightly more’ (5), ‘more’ (6), to ‘much more’ (7).
2.3. Participants
For this study, 20 participants (19 men and 1 woman) were recruited from a working population pool of 30 warehouse workers in Belgium. Exclusion criteria were disorders or pain that would prevent the person from performing their regular work. Five of the twenty participants did not participate in all the five measurement days due to sickness absence (n = 3), leave of absence (n = 1), and cancelling the participation (n = 1). These five were therefore excluded from the final study population. Hence, the final study population comprised 15 full-time employed staff (14 men and 1 woman) who had a mean (SD) age of 30.8 (11.5) years, body mass of 77.1 (11.3) kg, a stature of 179.3 (8.1) cm, and BMI of 24.0 (3.1). All but one were right-hand dominant. The participants had a median work experience duration in manual order picking at the company of 10 months (range: 1 month to 15 years). All participants received written and verbal information about the study and were informed that the participation was voluntary and that they could terminate their participation at any time. Written informed consent was obtained prior to participation in the study. The study was conducted according to the guidelines of the Declaration of Helsinki and approved by the board of the Post Master’s Program at the Odisee University of Applied Sciences (date of approval: June 26, 2019).
2.3.1. Musculoskeletal complaints, quantitative demands and work pace
The participants reported the 12-month prevalence of musculoskeletal complaints (‘pain, aches or discomfort’) and work-disabling musculoskeletal complaints at baseline using the Nordic Musculoskeletal Questionnaire (Kuorinka et al. Citation1987) and on a 5-level scale from ‘never’ to ‘almost every day’ (). For the body regions: neck, shoulders, wrist/hands, upper back, lower back and ankle/feet – having musculoskeletal complaints at least once during the last 12 months was experienced by a majority of the participants. The most common body regions experiencing musculoskeletal complaints at least once a month during the last 12 months were: the lower back (n = 7), the upper back (n = 5), the neck (n = 4), the knees (n = 4), and the ankle/feet (n = 4). For the work-disabling musculoskeletal complaints, the most common body regions experiencing complaints at least once a month during the last 12 months were: the lower back (n = 2), the ankles/feet (n = 2), the upper back (n = 1), the wrists/hands (n = 1), and the knees (n = 1).
Table 1. The number of participants (n = 15) reporting musculoskeletal complaints and work-disabling musculoskeletal complaints (in parentheses) during the last 12 months at baseline.
The quantitative demands and the work pace were measured at the baseline using the quantitative demands dimension and the work pace dimension from COPSOQ II (Pejtersen et al. Citation2010). The quantitative demands dimension comprised of the items: ‘work piles up’, ‘complete task’, ‘get behind’ and ‘enough time’. The work pace dimension comprised of the items: ‘work fast’, ‘high pace’ and ‘high pace necessary’. The items ‘high pace’ and ‘high pace necessary’ were measured using a five-scale interval ranging from: ‘to a very large extent’ to ‘to a very small extent’. The remaining items were measured using the five-level scale ranging from: ‘always’ to ‘never’. An index (0–100) was calculated for each dimension based on the average score of the included items with a high score indicating high demands (Berthelsen, Westerlund, and Kristensen Citation2014). The quantitative demands at work (normally distributed) had a mean (SD) score of 38.8 (17.1). The work pace (non-normally distributed) had a median (IQR) score of 75 (29.2).
2.4. Study settings
The study was carried out in the warehouse of a multinational company located in Belgium where manual sorting of packages was performed. The task comprised manual sorting of externally delivered packages that arrived in metal containers that were placed on carts (). The packages were registered twice using a hand scanner attached to a shoulder strap: once in the incoming metal container and afterwards, when sorted in the cardboard storage container. The packages varied in both mass (about 3–15 kg) and dimension (L × W × H, from about 29 × 11 × 20 cm to about 125 × 60 × 39 cm). The packages were picked from the metal containers from a horizontal distance of between underarm distance to about ¾ of arms reach, and from a vertical location of about 33 cm to about 109 cm. They were then sorted and placed in cardboard containers () at about the same vertical and horizontal ranges. About 3–6 packages were sorted each minute depending on the distance from the arrival metal container to the location of each specific cardboard storage container. When the storage containers had been filled (mass: ≤350 kg), they were manually pushed 5–20 metres to a conveyor belt for transport to a storage location in the warehouse (2H–2J). Thereafter, the worker pushed the empty metal containers about 5–10 metres to a conveyor for the empties. Each worker performed the sorting task for a full shift, which equals 7 hours.
Figure 2. Photos displaying a typical work cycle comprising of sorting and scanning of packages arriving in a metal container (A–E), placing and scanning the packages in a destination (cardboard) container (F–G), and pushes the container to a conveyor belt (H–J).
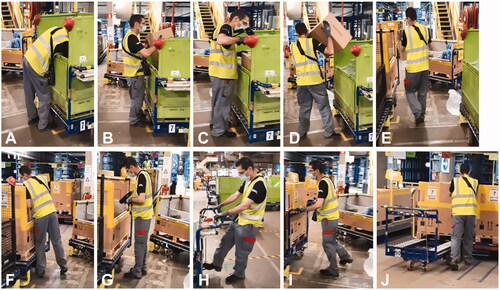
Each participant was monitored for five workdays within four weeks using the Smart Workwear System (). The participants were informed that the system would register their work postures. On the feedback training days, the participants were additionally informed that the system would provide them vibrotactile feedback if they bend their trunk forward above predefined threshold levels and that they should aim to reduce demanding postures. The vibrotactile feedback was set at two threshold levels for the trunk forward inclination angle: (i) above 30° with intermittent vibration, and (ii) above 45° with continuous vibration. The vibrotactile feedback was stopped as soon as the trunk inclination dropped below 30°. The threshold levels were based on the RAMP Tool (Lind, Forsman, and Rose Citation2019a; Lind, Forsman, and Rose Citation2020b; Rose et al. Citation2020), in which trunk inclination exceeding 20° for 1 hour per workday and trunk inclination exceeding 45° for 30 minutes per workday is considered hazardous and requires risk-reduction measures. However, for this type of work, it was not considered possible to eliminate most trunk inclination angles <30°, therefore the lower threshold level of 30° was used. Hence, the training targeted the ‘peak’ trunk inclination angles.
Figure 3. Illustration of the study setting. Five measurement days were performed, including the baseline, feedback training session 1, feedback training session 2 and post-training session (on the same day), and follow-ups 1 and 2. Surveys were collected on all measurement days with specific topics as illustrated in the boxes under each measurement day.
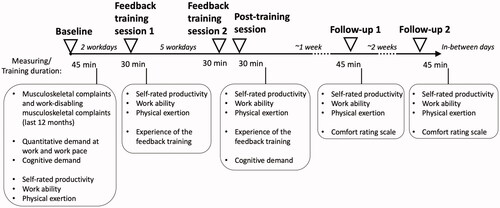
At the baseline, the participants performed their normal sorting tasks and were monitored for about 45 minutes. Two workdays after the baseline, the participants performed their tasks with concurrent vibrotactile feedback for about 30 minutes (feedback training session 1). After five workdays, the participants received the same concurrent vibrotactile feedback for about 30 minutes (feedback training session 2), directly followed by about 30 minutes of monitoring without feedback (post-training session). To evaluate the medium-term effects, the participants were monitored on two follow-up occasions without receiving vibrotactile feedback at about one and three weeks after the post-feedback training (follow-up 1 and follow-up 2). Half of the enrolled participants worked day shifts (6 AM to 2 PM), and the other half worked evening shifts (2 PM to 10 PM). Five participants were monitored simultaneously. To minimise any potential source of variability due to e.g. work tasks or fatigue, each participant was monitored at the same time during the measurement days. Additional follow-up days were arranged for those participants who were unavailable on the prescheduled follow-up occasions. During the measurement days, the participants were instructed to work at their own normal work pace.
2.5. Statistical analysis
The Shapiro-Wilk test was used to check the normality of the data. For normally distributed data, mean and standard deviation (SD) values were presented and paired t-tests were used for comparison. For non-normally distributed data, median and interquartile range (IQR) were presented, and the Wilcoxon-Signed Rank tests were used.
The effect of feedback training on trunk postures (aim 1) was evaluated using within-subject comparisons using the repeated measures analysis of variance (ANOVA) for normally distributed data sets, and the Friedman test for non-normally distributed data sets. For the repeated measures ANOVA, the Greenhouse–Geisser correction was used for data sets violating Mauchly's Test of Sphericity. For post-hoc tests, paired t-tests were applied for normally distributed data and the Wilcoxon-Signed Rank test for non-normally distributed data. The following parameters were compared between the scenarios: the proportion of time in forwarding trunk inclination ≥20°, ≥30°, ≥45°, and ≥60°, the 90th, 95th, and 99th percentile angles, and the 10th–90th percentile range.
The system usability (aim 2) was evaluated using descriptive data for the comfort rating scales. Secondly, the cognitive demands scores from baseline and feedback training session 2 were compared using the paired t-test. The effect of changes in trunk inclination angle on individual perceived work ability and physical exertion (aim 3) were evaluated using longitudinal multilevel models applying a within-subject pairwise design. For this, the 95th percentile of trunk inclination angles was used as a measure for changes in trunk inclination angle; hereafter referred to as postural load.
A series of two-level hierarchical multilevel models were estimated with measurement occasions (level 1) nested within individuals (level 2). First, intercept-only models () were estimated to calculate the variance over time (level 1) and between individuals (level 2) for both perceived work ability and physical exertion. Second, unconditional growth models with non-linear time trends (i.e.
and
) were estimated by including a time dummy variable using three categories: reference (baseline), feedback (feedback training sessions 1 and 2), and follow-up (follow-up sessions 1 and 2). Finally, conditional growth models including postural load
as our time-varying variable of interest and productivity demands
as a control variable. These final models can be described by the following equation with
being the residual error terms at the highest level (individual level) and
the residual errors at the lowest level (time level):
The gammas represent the parameter estimates and the X variables are the observed variables in the data. For the gamma parameters, the first subscript is the same as its corresponding variable and the second subscript refers to the level in the data. As an example,
is the parameter of the second variable on the lowest level (i.e. time level). For the X variables, the subscript j indicates individuals (j = 1 … 13) (the RPE-ratings of two participants were excluded due to extreme values, see also the Discussion section Effects of trunk posture on physical exertion and work ability), and the subscript i indicates time points (i = 1 … 5). Model parameters were estimated using the maximum likelihood estimation which utilises the iterative generalised least squares estimator (Goldstein Citation2003).
The statistical analyses were performed using SPSS (IBM SPSS Statistics 27, New York, United States) and R (R Foundation for Statistical Computing, Vienna, Austria, version 4.0.3). P-values < 0.05 were considered statistically significant.
3. Results
3.1. Trunk postures
The repeated measures ANOVA tests and the Friedman tests were all statistically significant (p < 0.05). As shown by the descriptive data ( and ), a significantly (p < 0.05) lower proportion of time was spent in trunk forward inclination ≥45° and ≥60° when receiving the vibrotactile feedback during feedback training 1 compared to the baseline, which corresponded to a median intra-individual percentage reduction in time by 34% for inclination angles ≥45° and 80% for inclination angles ≥60°. A significant (p < 0.05) reduction in the proportion of time in trunk inclination ≥30° ≥45° and ≥60° was also recorded during both feedback training 2 and post-feedback training sessions. The median intra-individual reduction in the proportion of time in trunk inclination ≥30° ≥45° and ≥60° ranged from 60 to 89% when compared to the baseline. For follow-up 1 and follow-up 2, there were no significant differences in the trunk postural load compared to the baseline. During feedback training 1, the 95th and 99th percentile trunk inclination angles, as well as the 10th–90th percentile range, declined significantly (p < 0.05) compared to the baseline values. During feedback training 2 and post-feedback training, the group 90th, 95th, and 99th percentile trunk inclination angles and the 10th–90th percentile range were significantly reduced (p < 0.01) compared to the baseline. As an example, the median of the 90th percentile trunk inclination angle declined from 31° at the baseline, to 21.4° at feedback training 2 and to 19.3° at post-feedback training. The 95th percentile trunk inclination angle declined from 40° at the baseline to 26.8° at feedback training 2 and 28.6° at post-feedback training. Furthermore, at the group level, the (median) 99th percentile inclination angle declined from 60° at baseline to 50° during feedback training 1, to 47.2° at feedback training 2, and to 40.1°at post-feedback training. For the medium-term retention test, there were no significant differences for the 90th, 95th, and 99th percentile trunk inclination angles. For follow-up 1 and follow-up 2, only a slight tendency of decline in the 90th, 95th percentile trunk inclination angles and the 10th–90th percentile range was observed.
Figure 4. (A) Accumulated proportion of time of trunk forward inclination ≥30°, ≥45°, and ≥60° at baseline, the feedback training sessions 1 and 2, post-training session, and the follow-up sessions 1 and 2. (B) the 90th, 95th and 99th percentile trunk inclination angles, and the 10–90th percentile range for trunk inclination. Statistically significant (p < 0.05, Wilcoxon signed-rank test) differences between the scenarios are displayed with their corresponding p-values.
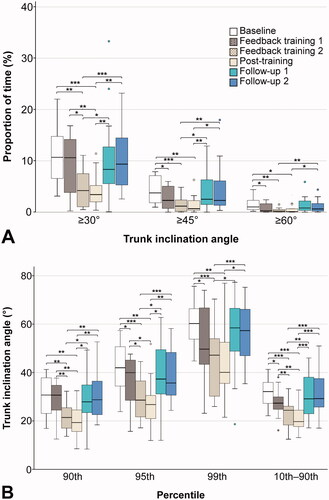
Table 2. Group median values and median intra-individual percentage differences (diff) (compared to the baseline) in the proportion of time in trunk inclination ≥30°, ≥45°, and ≥60°, and the group 90th, 95th, and 99th percentile angles and 10th–90th percentile range of trunk forward inclination in the feedback training sessions 1 and 2, the post-feedback training and the follow-up sessions 1 and 2.
3.2. System usability
3.2.1. Cognitive demands
The cognitive demands had a median (IQR) score of 65.6 (13.3) at baseline. There was a tendency for lower scores at feedback training 2 with a score of 59.4 (15.1), but the difference was not significant (p = 0.151).
3.2.2. Wearer comfort
The participants perceived the system as rather comfortable to wear, as shown by their low scores on the Comfort Rating Scale (). Four of the six dimensions had a median (IQR) score of 0 (0) on both assessed days. For emotion, one participant rated a maximum of 6 on follow-up 1, which decreased to 2 on follow-up 2. For harm, all but one participant rated 0 and one participant rated 1 on follow-up 2. For a perceived change, one participant rated a maximum score of 6 on follow-up 1, which also decreased to 2 on follow-up 2. For anxiety, all but one participant rated 0 and one participant rated 6 on follow-up 1. For the other two dimensions, attachment and movement, the scores were rated higher with a median (IQR) score above ranging from 0 to 5.
Table 3. Median (IQR) ratings of the 21-point Comfort Rating Scale with verbal anchors at each end: ‘Low’ (0), and ‘High’ (20).
3.2.3. General experience of the vibrotactile feedback
The results from the questionnaire showed that all 15 participants felt the vibration during their work in feedback training 1 and all but one during feedback training 2. For both feedback training sessions, 14 of 15 participants reported experiencing the system as nonintrusive and that it did not interfere with how they carried out their work tasks. One participant experienced mild itching and one mentioned that the customised t-shirt was too large. All participants responded that they understood the purpose of the feedback, and 10 and 13 participants described, in feedback training sessions 1 and 2, respectively – that the vibrotactile feedback had affected them in the way that they tried to correct their posture. For example, 3 participants reported that the feedback made them bend their knees more often, 1 participant mentioned avoiding bending the back forward too much, and 12 participants stated they paid more attention to and tried to correct their postures (among them were 7 after feedback training 1, and 10 after feedback training 2). In contrast, 5 and 2 participants stated that the vibrotactile feedback had not affected them in feedback training sessions 1 and 2, respectively. A reason for not being able to use the feedback to correct posture was the requirement to maintain a high work pace which was mentioned by 2 participants at feedback training 1.
3.3. Self-rated work ability, physical exertion and productivity demands
The work ability was at baseline rated with a median (IQR) score of 8.0 (2.0). The same median score was obtained on all measurement days, except for the feedback training 2 where the work ability had a median (IQR) score of 9.0 (2.0). Although work ability was higher during the feedback condition ( = 0.47, p < 0.05), the change in workability was not related to the change in postural load (
= 0.006, p = 0.42). The majority (76%) of the differences in self-rated work ability were due to differences between individual workers, and not as a consequence of changes over time.
For the perceived physical exertion (n = 13), the median RPE score across all measurement days was 13.0 (i.e. ‘somewhat hard’), and the median score for a typical workday was 15.0 (‘hard’). When compared to the median (IQR) RPE score at a baseline of 14.0 (2.5), a tendency for lower median scores was recorded for all workdays of 13.0 (3.0–4.5), with the exception of the follow-up 1 session where a median score of 15.0 (3.5) was recorded. For the perceived physical exertion (n = 13), the median RPE score across all measurement days was 13.0 (i.e. ‘somewhat hard’), and the median score for a typical workday was 15.0 (‘hard’). When compared to the median (IQR) RPE score at a baseline of 14.0 (2.5), a tendency for lower median RPE scores was recorded for all workdays of 13.0 (3.0–4.5), with the exception of the follow-up 1 session where a median score of 15.0 (3.5) was recorded. When testing the influence of postural load on perceived physical exertion, the postural load did not have a significant impact on the RPE score ( = −0.002, p = 0.90). In contrast, the majority (89%) of the differences in perceived exertion were due to changes over time (within individual workers).
Regarding the productivity demands, a majority (n = 9) rated the productivity demands at baseline to be ‘about the same’ as for a normal workday, while the others rated it to be ‘slightly less’ (n = 2), ‘less’ (n = 2) and ‘much less’ (n = 2). When compared to the mean productivity demand score of 3.2 at baseline, there was a tendency of increased productivity demands for each measurement day, where a mean score of 3.53 was recorded for feedback training 1, a score of 3.87 for feedback training 2, a score of 4.13 for follow-up 1, and a score of 3.47 for the follow-up session 2. Still, half or more of the participants assessed the productivity demands on each measurement day to be about the same as for a normal workday. In this context, the time-varying variable, i.e. productivity demands showed that a one-unit increase in product demand level, such as from ‘slightly more’ to ‘more’, was associated with 1.07 units increase in perceived exertion ( = 1.07, p < 0.01).
4. Discussion
4.1. Trunk posture
This study evaluated the effect of concurrent vibrotactile feedback training using the Smart Workwear System to reduce the time in adverse trunk forward inclination of 15 warehouse workers in real manual sorting work. During the feedback training, the warehouse workers significantly reduced the time in demanding trunk postures and the greatest magnitude of the reduction occurred for a time in trunk inclinations ≥45° and ≥60°. A reduction in the proportion of time in trunk inclination ≥30°, ≥45° and ≥60° also occurred directly after the feedback was withdrawn, i.e. at the post-training session (short-term retention test). However, no significant reduction in the time in demanding trunk postures occurred during the two follow-up sessions about one week and three weeks after the post-training session. As a result, this study provides support that vibrotactile feedback training, which consisted of two 30-minute training sessions, can be effective in reducing the trunk inclination loads during and immediately after the training. However, these reductions in exposure did not retain significant after about one and three weeks, indicating that this short training program using concurrent vibrotactile feedback may not be effective in reducing trunk inclination exposure over a longer time (such as after one or several months).
To the best of our knowledge, this is the first study that has evaluated the effect of using short-term vibrotactile feedback training to reduce trunk postural load in a real work setting on warehouse workers, and to evaluate the effects at both the short-term – during and directly after training, and medium-term after more than a week.
When compared to previous research on the effect of vibrotactile feedback training targeting the trunk and upper arm postural load in order pickers, Lind et al. (Citation2020c) reported similar proportions of reductions as observed in the current study. Whereas Lind et al. (Citation2020c) observed an intra-individual reduction in time in trunk inclination ≥30° by 54% and a reduction by 92% for time trunk inclination angles ≥45° – the current study observed a reduction in time of 68% for trunk inclination ≥30° and 80% for trunk inclination ≥45°. The proportional reduction for the 90th and 99th percentile angles were similar between the studies when receiving the vibrotactile feedback, i.e. declines by 31% and 37%, respectively for the 90th and 99th percentile inclination angle in Lind et al. (Citation2020c) – while the corresponding declines in the current study were 34% and 36%, respectively. For both studies, the decline in trunk postural load remained quite consistent for the post-training immediately after the feedback was withdrawn. However, Lind et al. (Citation2020c) did not study the medium-term retention effects of the vibrotactile feedback. Such medium-term effects were studied by Kamachi, Owlia, and Dutta (Citation2021), who observed retained reductions in the peak trunk angle after both two weeks and two months. However, Kamachi, Owlia, and Dutta (Citation2021) only observed significant reductions in the peak trunk angle for those few simulated tasks that were included in the training program. For an additional task not included in the training (to test the skill transfer), there was no significant reduction in the peak trunk angle when compared to the control group. Hence, more studies on manual handling tasks are needed to evaluate training programs that are useful for skill transfer in a longer time perspective. Another difference between the studies was that Kamachi, Owlia, and Dutta (Citation2021) only included novice participants, who performed simulated work tasks – while the current study included trained participants in a real working environment (hence, more complex) which both can affect retention (Nembhard Citation2000; Nembhard and Osothsilp Citation2002).
The trunk forward inclination exposure at the baseline was somewhat close to those of several other occupational groups that perform manual handling tasks. At the baseline, the group median 90th percentile trunk inclination angle of 30.7°, is close to that of paper mill workers (mean of 29.2°) (Heiden, Zetterberg, and Mathiassen Citation2019), but slightly higher when compared to previous studies of order picking tasks (26° in both) (Christmansson et al. Citation2002; Lind et al. Citation2020c). Further, the 10th–90th percentile range (here: 32.1°), was about the same as for baggage handlers performing sorting (31.9°) (Wahlström et al. Citation2016), but higher than industrial order picking tasks (24°) (Christmansson et al. Citation2002; Lind et al. Citation2020c), assembly tasks (25.4°), machinery operating (21.4°), and maintenance (29.6°) (Schall et al. Citation2021) – but lower when compared to paper mill workers (35.1°) (Heiden, Zetterberg, and Mathiassen Citation2019) and to baggage handlers working at the ramp (39.0°) (Wahlström et al. Citation2016). The 90th and 99th percentiles trunk inclination angles in the current study (i.e. 30.7° and 60.2°) were similar to those of paper mill workers (29.2° and 60.2°) and slightly lower than for baggage handlers performing sorting, i.e. 32.2° and 62.7° (Wahlström et al. Citation2016). Despite these similarities in trunk inclination exposures, the possibilities of reducing trunk inclination exposure from work technique training may be highly dependent on the exact work conditions (Lind et al. Citation2020c). Hence the possible effectiveness of work technique training may vary. Still, multifaceted measures that additionally consider engineering controls and organisation strategies are important to reduce exposure to adverse postures (Nelson et al. Citation2006). Therefore, to effectively reduce adverse exposures, work technique training may be used as a complement to primary measures after first focussing at eliminating or minimising the hazards at the source. The trunk posture was measured by IMUs in the present studies. Although angular velocities can differ between inclinometers based on accelerometers only and those based on both accelerometers and gyroscopes, the difference within each percentile is small (<2°) for postures (Fan et al. Citation2021). Hence, the comparisons above with other occupational groups of sorting tasks and manual handling seem justified.
4.2. System usability
Regarding the second aim, this study supports that the Smart Workwear System has high wearer comfort based on the comfort rating scores. Also, the concurrent posture-correction vibrotactile feedback did not increase the cognitive demands. Most participants considered the system as nonintrusive and that it did not interfere with how they carried out their work tasks, and all participants reported that they could understand the feedback provided. In addition, a majority of the participants reported that they increased their awareness of their own work postures after the feedback training.
The wearer's comfort was evaluated from ratings of experienced workers performing their daily work in a real work setting, and the wearer used the system for several workdays before providing the wearer comfort ratings. This can be seen as a strength compared to ratings by Knight and Baber (Citation2005) in simulated work conditions and to Lind et al. (Citation2020c) who studied the participants in a factory lab environment on a single occasion. Knight and Baber (Citation2005) assessed the wearer comfort using the CRS on two versions of the SensVest, designed to house components for measurements of physiological and kinematics data. For the more finalised version, i.e. SensVest 2, the highest mean score (i.e. lowest wearer comfort) was reported for the attachment dimension (10), while for the current study the Smart Workwear System had a mean score for the attachment dimension of 4.3 (SD 4.2) when taking the average value from the two days for each person (data not shown in ). The corresponding values for the movement dimension were about 6 for the SensVest 2, and 3.8 (SD 4.8) for the Smart Workwear System. For a perceived change, the corresponding mean score for the SensVest 2 was about 8, while the mean score for the Smart Workwear System was 0.5 (SD 1.2). The low score for the perceived change dimension in the current study indicates that the wearer did not feel physically different or upset by wearing smart workwear. Besides the physical dimensions, emotional components of comfort, examined in the emotion and anxiety dimensions, were also scored low for the Smart Workwear, hence, indicating that wearing the device did not make the workers emotional concerned about their appearance or make them worry about their safety while wearing the device. In agreement with Lind et al. (Citation2020c) who also evaluated the Smart Workwear System, the highest scores (indicating relatively lower wearer comfort) were given for the wearable comfort dimension ‘movement’, i.e. ‘the device affects the way I move’. While a high rating in this dimension is seen as an indication of low wearer comfort by Knight and Baber (Citation2005), this is the main purpose of the system although it should not inhibit or restrict the wearers’ movements which are also included in the dimension (see ). In line with Lind et al. (Citation2020c), the scores were low for the other wearer comfort dimensions, indicating a high wearer comfort.
Wearer (or clothing) comfort covers more qualities than included by the CRS, nonetheless, the CRS-dimensions include a range of dimensions that were judged as relevant to the type of application targeted in the current study. In addition to evaluating whether the system inhibits or restricts movement, the impact on work performance and an overall question on the comfort (Beeler et al. Citation2018) might need future investigations. It should be noted that the period of time did not exceed two hours in the current study nor in the study by Lind et al. (Citation2020c) since the aim of these two studies focussed on the short period training programs. Hence, the evaluation of wearer comfort may not entirely represent the wearer comfort if being used for full workdays and on a daily basis. Also, the assessment of the wearer comfort in the current study was not performed on the workdays when the participants were receiving the vibrotactile feedback. Hence, this limits the possibilities of studying this specific factor. As noted in Lind et al. (Citation2020c) a few participants (2/15) reported complaints that the concurrent vibrotactile feedback provided in that study was given too much of the time. Based on these complaints, it may be inadvisable to use concurrent vibrotactile feedback continuously for longer time periods than those used in Lind et al. (Citation2020c) of about 10–15 minutes and about 30 minutes in the current study. When used for longer periods of time, other types of feedback such as terminal feedback may be preferable, or faded feedback frequency to limit potential feedback dependency (Ribeiro et al. Citation2011).
The median cognitive demands scores at baseline in the current study of 65.6 (IQR 13.3) indicated a normal level of cognitive demands, and which is close to the level among a representative sample of 3517 Danish workers reporting a mean score of 63.9 (SD 18.7) (Pejtersen et al. Citation2010). Also, instead of a potential increase in the perceived cognitive demands when receiving the feedback training as might be expected, there was no increase in the scores. This suggests that when workers are given this type of concurrent vibrotactile feedback using a single vibrotactile unit, the perceived cognitive demands may not need to be increased. This can be contrasted to visual feedback which requires continuous visual control (Sigrist et al. Citation2013), and hence, might increase the cognitive demands in this type of work. The quantitative demands in this study (mean score 38.8), were similar to those of the Danish workers (mean score 40.2) (Pejtersen et al. Citation2010). However, a substantially higher work pace score was reported at baseline in the current study (median score 75) when compared to the Danish workers (mean score of 59.5). This indicates a relatively high work pace of the manual sorting work performed in the current study, and which was also stated by some participants as having restricted their ability to change the work technique based on the vibrotactile feedback.
4.3. Effects of trunk posture on physical exertion and work ability
Concerning the third aim, the longitudinal analysis showed that postural load (i.e. the 95th percentile trunk inclination angle), did not have a significant effect on either self-rated work ability nor perceived physical exertion during the 4-week timeframe of the study. When compared to the baseline, no significant differences in perceived exertion were observed during the feedback training sessions as well as during the follow-up sessions. The perceived exertion varied from day to day (intra-class correlation = 89%), but it did not follow the changes in inclination.
It is likely workers’ perceived exertion is determined also by other factors such as productivity demands. This was confirmed by the longitudinal model showing that changes in self-rated productivity demands were positively associated with changes in perceived exertion.
A common strategy to reduce trunk inclination is to adopt a squat or semi-squat which includes bending the knees. This strategy can, however, increase the physiological workload since the individual has to move a larger part of the body, and hence, a larger mass (Garg & Herrin Citation1979; van Dieën, Hoozemans, and Toussaint Citation1999). Also, some individuals may have insufficient physiological capacity or muscle strength to continue this strategy for an entire workday, without first being physically conditioned to such a changed strategy (Asfour, Ayoub, and Mital Citation1984; Genaidy et al. Citation1994; Knapik Citation1997). Since a changed work strategy may introduce new adverse exposures, it was considered important to, in addition to measurements of trunk inclination angles, include an overall (global) subjective measure such as perceived physical exertion and work ability. As displayed earlier, no significant effect of the perceived physical exertion was observed between the measurement days when compared to the baseline, hence the strategy to reduce trunk inclination did not result in either a significant lower nor higher perceived physical exertion level. Further, it can be hypothesised that for workers experiencing low back pain, a reduction in the time in trunk inclination could have improved the perceived work ability in the longer term. Although there was a tendency of improved work ability at feedback training 2 (and post-training) sessions, this was not significantly related to the change in the 95th percentile trunk inclination. In similarity, in the study by Boocock et al. (Citation2019), no significant difference in RPE-score was reported among those receiving auditory feedback and who adopted a more physiologically demanding lifting technique compared to the control group. It was hypothesised that the absence of significant change in the RPE score in that study may have been influenced by being confounded by perceived local fatigue. In the current study, the RPE ratings of two participants were excluded because they reported extremely low scores (‘6’ i.e. ‘no exertion at all’) or extremely high scores (‘20’ i.e. ‘maximal exertion’). Such extreme ratings indicated that these two persons did not interpret the verbal anchors of the RPE scale correctly. Additionally, all participants were asked to rate their perceived physical exertion during a typical workday. Interestingly, for each measurement day, the RPE score for a typical workday was consistently higher than all ‘current’ measured workdays except for follow-up I, which also had a significantly higher self-reported productivity demand level. When pooling all RPE scores for the ‘current’ workday (mean: 13.0) against the scores for a ‘typical’ workday (mean: 15.0), the difference was significant (p < 0.01). Reporting a higher score for a ‘typical’ workday compared to the ‘current’ workday is consistent with other studies among garbage collectors, cleaners, and home care service workers (Forsman et al. Citation2020). Assuming a higher validity when such rating is collected for the current workday, this points to that rating for a typical workday may be slightly overestimated.
4.4. Methodological considerations
This study applied a within-subjects design where each participant served as their own control instead of having a control group. The advantages of the repeated measures design, which was chosen in this study, include a higher statistical power by controlling variability between subjects, and fewer subjects were required. One disadvantage of this design was the order effects, which could be affected by the order of the tested condition or changes due to learning over time. Still, the results showed that the effects on postural exposure improvement increased during and directly after the training sessions and reduced during follow-ups 1 and 2. This pattern of the changes did not support a learning effect; on the contrary, it supported that the changes were mainly due to the feedback training provided.
Given that a short training period of two 30-min feedback sessions was chosen, concurrent feedback was used since it may more directly indicate which specific events exceed the predetermined angular thresholds when compared to terminal feedback provided after each completed work cycle. According to a previous study (Lind et al. Citation2020c), short feedback training of 30 min may be effective for reducing the time in demanding postures – at least in the short term, and the feedback may be disturbing if used for a longer period. Concurrent feedback will also produce a larger number of feedback events (i.e. as soon as the wearer exceeds the predetermined angular thresholds) when compared to terminal feedback. However, concurrent feedback has some drawbacks when used for longer time periods, since it may interfere with the own body’s intrinsic feedback, and thus contribute to the user being dependent on the external feedback (Ribeiro et al. Citation2011). To overcome this drawback, some studies have applied faded feedback (Kamachi, Owlia, and Dutta Citation2021).
Since the study was performed during real work as opposed to during controlled simulated work, it was not possible to control the amount of performed work. However, the work consisted of fairly repetitive work performed at a high work pace. The participants were asked to rate the amount of work they performed during each day (‘self-rated productivity’). As presented in section Self-rated work ability, physical exertion and productivity demands, the training days were rated slightly higher than the baseline. The baseline had the lowest mean (self-rated) productivity, while the first follow-up occasion had the highest. Hence, if the work pace was slightly different on different occasions, it would rather attenuate the positive effects of the feedback training than the opposite. The measures of the trunk inclination angles were recorded using an inertial measurement sensor incorporated into the Smart Workwear System. The system has been validated for trunk inclination measurement (Yang, Borgström, and Forsman Citation2019). Even though it may be assumed that the t-shirt and the sensor are placed in the same position on each measurement day, there is, however, always a variability between the obtained reference positions on different days. To minimise such effects, each participant wore the same individually assigned t-shirt and a standardised reference posture was applied (Hansson et al. Citation2006) which in previous validations have observed between-days variations of less than 2°. Such small between-day variations should not have a significant effect on the study results.
A strength of this study was that it was carried out in a real work setting and included order-pickers performing the normal work. This improves the ecological validity when compared to being performed in simulated work in laboratory settings or in a field settings. A sample size of 20 participants was targeted, expecting a drop-out rate of 10–20%. The final study population that completed all session were 15 (25% drop-out rate), resulting in a slightly larger study population than the 13 included in a previous study that observed statistically significant difference using an earlier version of the system (Lind et al. Citation2020c). The sample size is close to those of similar studies targeting postural load change in short-term feedback training using technical measurement instruments to record postures, e.g. 10–16 participants (Fethke, Gant, and Gerr Citation2011; Vignais et al. Citation2013; Doss et al. Citation2018; Harris-Adamson et al. Citation2019; Lind et al. Citation2020a). A larger proportion of female participants was also targeted, but due to the re-location of workers in the facility only one female was included in the final study population. Consequently, it was not possible to evaluate any potential difference in the effect of the feedback training attributed to sex. Therefore, we encourage such potential effects to be evaluated in future studies.
4.4. Clinical relevance
At the baseline, the workers were exposed to trunk inclination over 30° for 10.7% of the worktime (group median), which was reduced to 3.4% post-training when the feedback was withdrawn. Hoogendoorn et al. (Citation2002) found that working with the trunk in forwarding flexion ≥30° for more than 10% of the worktime was significantly associated with sickness absence over 7 days due to LBP (OR 3.21), but no significant association was observed when it occurred 5–10% of the worktime. The baseline trunk inclination ≥45° of 3.7% corresponds to 89 min per week for 8 hours workdays, assuming a similar exposure to work tasks for the work week. In comparison, working with the trunk forward flexed ≥45° for 90 min per week has been associated with an increased risk of LBP/low back disorders (RR 2.82, 95% CI 1.16–6.86) in elderly care and nursing home workers (Jansen, Morgenstern, and Burdorf Citation2004). Directly after the feedback training, the trunk inclination ≥45° declined to 0.7% which corresponds to 17 min per week and thus stays below the reference level in Jasen et al. (Citation2004). To summarise, this points to that the concurrent vibrotactile feedback in the short term contributed to a time reduction in trunk inclination of a magnitude that could be of clinical relevance if maintained for a longer time and it did not seem to introduce new additional adverse exposures as noted by a non-increase in perceived physical exertion, work ability or perceived cognitive demands. In the medium-term, however, no significant sustained time reductions in trunk inclination nor peak angles were observed. Hence, future studies are needed to evaluate if improvements in the feedback training can transfer the short-term improvements to retained median- and long-term effects.
5. Conclusion
In this study, the concurrent posture-correction vibrotactile feedback used for work technique training in real manual handling work contributed to reduced time in trunk inclination >30°, >45°, and >60°, and reductions in the 90th, 95th, and 99th percentile trunk inclination angles, when receiving feedback and immediately after feedback withdrawal. The significant retained reductions of time in demanding trunk postures in the short term indicate a short-term learning effect. For the medium-term effect, the study observed tendencies of reduced exposure, but they were not significant. This points to the that the training program used may not be effective over a longer time period without it being continuously repeated. The system had overall high wearer comfort and its use did not increase workers’ perceived cognitive demands. No significant effects attributed to changed trunk inclination exposure were observed for the physical exertion level and the work ability within the study period. Future studies are needed to determine whether vibrotactile feedback can be effective to reduce postural exposure in real work over a longer time period, or under different training conditions, considering factors such as the duration and frequency of the training provided, feedback modality type, and the timing of the feedback.
Abbreviations | ||
RULA | = | Rapid Upper Limb Assessment |
NMQ | = | the Nordic Musculoskeletal Questionnaire |
CRS | = | the Comfort Rating Scale |
COPSOQ II | = | the Copenhagen Psychosocial Questionnaire II. |
Acknowledgments
The authors thank the participants for participating in the study.
Disclosure statement
The authors declare no conflict of interest.
Additional information
Funding
References
- Agruss, C. D., K. Williams, and F. A. Fathallah. 2004. “The Effect of Feedback Training on Lumbosacral Compression during Simulated Occupational Lifting.” Ergonomics 47 (10): 1103–1115. doi:10.1080/00140130410001686375.
- Ahlström, L., A. Grimby-Ekman, M. Hagberg, and L. Dellve. 2010. “The Work Ability Index and Single-Item Question: associations with Sick Leave, Symptoms, and Health-a Prospective Study of Women on Long-Term Sick Leave.” Scandinavian Journal of Work, Environment & Health 36 (5): 404–412. doi:10.5271/sjweh.2917.
- Alavinia, S. M., T. I. van den Berg, C. van Duivenbooden, L. A. Elders, and A. Burdorf. 2009. “Impact of Work-Related Factors, Lifestyle, and Work Ability on Sickness Absence among Dutch Construction Workers.” Scandinavian Journal of Work, Environment & Health 35 (5): 325–333. doi:10.5271/sjweh.1340.
- Alberto, R., F. Draicchio, T. Varrecchia, A. Silvetti, and S. Iavicoli. 2018. “Wearable Monitoring Devices for Biomechanical Risk Assessment at Work: Current Status and Future Challenges-a Systematic Review.” International Journal of Environmental Research and Public Health 15 (9): 2001. doi:10.3390/ijerph15092001.
- Andersen, L. L., T. Clausen, R. Persson, and A. Holtermann. 2012. “Dose-Response Relation between Perceived Physical Exertion during Healthcare Work and Risk of Long-Term Sickness Absence.” Scandinavian Journal of Work, Environment & Health 38 (6): 582–589. doi:10.5271/sjweh.3310.
- Araujo, F. X. D., M. Scholl Schell, and D. C. Ribeiro. 2017. “Effectiveness of Physiotherapy Interventions plus Extrinsic Feedback for Neck Disorders: A Systematic Review with Meta-Analysis.” Musculoskeletal Science & Practice 29: 132–143. doi:10.1016/j.msksp.2017.04.005.
- Asfour, S. S., M. M. Ayoub, and A. Mital. 1984. “Effects of an Endurance and Strength Training Programme on Lifting Capability of Males.” Ergonomics 27 (4): 435–442. doi:10.1080/00140138408963507.
- Baker, P., and Z. Halim. 2007. “An Exploration of Warehouse Automation Implementations: Cost, Service and Flexibility Issues.” Supply Chain Management: An International Journal 12 (2): 129–138. doi:10.1108/13598540710737316.
- Bark, K., E. Hyman, F. Tan, E. Cha, S. A. Jax, L. J. Buxbaum, and K. J. Kuchenbecker. 2015. “Effects of Vibrotactile Feedback on Human Learning of Arm Motions.” IEEE Transactions on Neural Systems and Rehabilitation Engineering 23 (1): 51–63. doi:10.1109/TNSRE.2014.2327229.
- Battini, D., A. Persona, and F. Sgarbossa. 2014. “Innovative Real-Time System to Integrate Ergonomic Evaluations into Warehouse Design and Management.” Computers & Industrial Engineering 77: 1–10. doi:10.1016/j.cie.2014.08.018.
- Bazazan, A., I. Dianat, N. Feizollahi, Z. Mombeini, A. M. Shirazi, and H. I. Castellucci. 2019. “Effect of a Posture Correction-Based Intervention on Musculoskeletal Symptoms and Fatigue among Control Room Operators.” Applied Ergonomics 76: 12–19. doi:10.1016/j.apergo.2018.11.008.
- Beeler, N., L. Roos, S. K. Delves, B. J. Veenstra, K. Friedl, M. J. Buller, and T. Wyss. 2018. “The Wearing Comfort and Acceptability of Ambulatory Physical Activity Monitoring Devices in Soldiers.” IISE Transactions on Occupational Ergonomics and Human Factors 6 (1): 1–10. doi:10.1080/24725838.2018.1435431.
- Berthelsen, H. H. Westerlund, and T. Kristensen. 2014. Copsoq ii - en uppdatering och språklig validering av den svenska versionen av en enkät för kartläggning av den psykosociala arbetsmiljön på arbetsplatser Stockholm, (SWE): Stressforskningsinstitutet, Stockholms universitet.
- Boocock, M., Y. Naudé, S. Taylor, J. Kilby, and G. Mawston. 2019. “Influencing Lumbar Posture through Real-Time Biofeedback and Its Effects on the Kinematics and Kinetics of a Repetitive Lifting Task.” Gait & Posture 73: 93–100. doi:10.1016/j.gaitpost.2019.07.127.
- Borg, G. 1998. Borg’s Perceived Exertion and Pain Scales. Champaign, IL, USA: Human Kinetics.
- Breen, P. P., A. Nisar, and G. Olaighin. 2009. “Evaluation of a Single Accelerometer Based Biofeedback System for Real-Time Correction of Neck Posture in Computer Users.” Conference of the IEEE Engineering in Medicine and Biology Society, 7269–7272.
- Buchanan, J. J., and C. Wang. 2012. “Overcoming the Guidance Effect in Motor Skill Learning: Feedback All the Time Can Be Beneficial.” Experimental Brain Research 219 (2): 305–320. doi:10.1007/s00221-012-3092-x.
- Causo, A. Z. Ding, S. H. Yeo, and I. Chen. 2011. “Strategies in Vibrotactile Feedback for Improved Upper Arm Posture Mapping and Replication Using Inertia Sensors.”. Proceedings of the 2011 IEEE/ASME International Conference on Advanced Intelligent Mechatronics. 683–688.
- Christmansson, M., L. Medbo, G. Å. Hansson, K. Ohlsson, J. Unge Byström, T. Möller, and M. Forsman. 2002. “A Case Study of a Principally New Way of Materials Kitting - an Evaluation of Time Consumption and Physical Workload.” International Journal of Industrial Ergonomics 30 (1): 49–65. doi:10.1016/S0169-8141(02)00077-X.
- Clemes, S. A., C. O. Haslam, and R. A. Haslam. 2010. “What Constitutes Effective Manual Handling Training? A Systematic Review.” Occupational Medicine (Oxford, England) 60 (2): 101–107. doi:10.1093/occmed/kqp127.
- De Korte, E. M., H. De Kraker, P. M. Bongers, and P. Van Lingen. 2008. “Effects of a Feedback Signal in a Computer Mouse on Movement Behaviour, Muscle Load, Productivity, Comfort and User Friendliness.” Ergonomics 51 (11): 1757–1775. doi:10.1080/00140130802327177.
- Denis, D., M. Gonella, M. Comeau, and M. Lauzier. 2020. “Questioning the Value of Manual Material Handling Training: A Scoping and Critical Literature Review.” Applied Ergonomics 89: 103186–103186. doi:10.1016/j.apergo.2020.103186.
- Doss, R., J. Robathan, D. Abdel-Malek, and M. W. R. Holmes. 2018. “Posture Coaching and Feedback during Patient Handling in a Student Nurse Population.” IISE Transactions on Occupational Ergonomics and Human Factors 6 (3-4): 116–127. doi:10.1080/24725838.2018.1428838.
- Driscoll, T., G. Jacklyn, J. Orchard, E. Passmore, T. Vos, G. Freedman, S. Lim, and L. Punnett. 2014. “The Global Burden of Occupationally Related Low Back Pain: Estimates from the Global Burden of Disease 2010 Study.” Annals of the Rheumatic Diseases 73 (6): 975–981. doi:10.1136/annrheumdis-2013-204631.
- Eurofound. 2012. Fifth European Working Conditions Survey. Luxembourg, LUX: Publications Office of the European Union.
- Eurofound. 2016. Sixth European Working Conditions Survey Luxembourg, LUX: Publications Office of the European Union.
- Fan, X., C. M. Lind, I. M. Rhen, and M. Forsman. 2021. “Effects of Sensor Types and Angular Velocity Computational Methods in Field Measurements of Occupational Upper Arm and Trunk Postures and Movements.” Sensors )21 (16): 5527. doi:10.3390/s21165527.
- Fethke, N. B., L. C. Gant, and F. Gerr. 2011. “Comparison of Biomechanical Loading during Use of Conventional Stud Welding Equipment and an Alternate System.” Applied Ergonomics 42 (5): 725–734. doi:10.1016/j.apergo.2010.11.007.
- Forsman, M., Målqvist, I. S., Alderling, M., Bergman Rentzhog, A., Lundin, A., Savlin, P., Lindahl Norberg, A., Yang, L., Berglund, K., Linden, A., Abtahi, F., Lind, C.M, and Hillert, L. 2020. Hemtjänstpersonalens Arbetsförhållanden – Identifiering av Hälsoriskfaktorer Samt Åtgärdsförslag Stockholm, SWE: Institute of Environmental Medicine, Karolinska Institutet.
- Galloway, S. M. 2011. “The Effect of Biofeedback on Tennis Service Accuracy.” International Journal of Sport and Exercise Psychology 9 (3): 251–266. doi:10.1080/1612197X.2011.614851.
- Garg, Arun., and Gary D. Herrin. 1979. “Stoop or Squat: A Biomechanical and Metabolic Evaluation.” A I I E Transactions 11 (4): 293–302. doi:10.1080/05695557908974474.
- Genaidy, A., N. Davis, E. Delgado, S. Garcia, and E. Al-Herzalla. 1994. “Effects of a Job-Simulated Exercise Programme on Employees Performing Manual Handling Operations.” Ergonomics 37 (1): 95–106. doi:10.1080/00140139408963627.
- Gerard, M. J., T. J. Armstrong, D. A. Rempel, and C. Woolley. 2002. “Short Term and Long Term Effects of Enhanced Auditory Feedback on Typing Force, Emg, and Comfort While Typing.” Applied Ergonomics 33 (2): 129–138. doi:10.1016/S0003-6870(01)00062-X.
- Goldstein, H. 2003. Multilevel Statistical Models (3rd ed.). London, UK: Hodder Arnold.
- Hansson, G.Å., I. Arvidsson, K. Ohlsson, C. Nordander, S. E. Mathiassen, S. Skerfving, and I. Balogh. 2006. “Precision of Measurements of Physical Workload during Standardised Manual Handling. Part ii: Inclinometry of Head, Upper Back, Neck and Upper Arms.” Journal of Electromyography and Kinesiology 16 (2): 125–136. doi:10.1016/j.jelekin.2005.06.009.
- Harris-Adamson, C., E. Lam, F. Fathallah, A. D. Tong, S. Hill, and A. Smith. 2019. “The Ergonomic Impact of a Mattress Lift Tool and Bottom Sheet Type on Hotel Room Cleaners While Making Beds.” Applied Ergonomics 81: 102880. doi:10.1016/j.apergo.2019.102880.
- Heiden, M., C. Zetterberg, and S. E. Mathiassen. 2019. “Trunk and Upper Arm Postures in Paper Mill Work.” Applied Ergonomics 76: 90–96. doi:10.1016/j.apergo.2018.12.004.
- Hallman, D. M., A. Holtermann, A. Dencker-Larsen, M. Birk Jørgensen, and C. D. Nørregaard Rasmussen. 2019. “Are Trajectories of Neck-Shoulder Pain Associated with Sick Leave and Work Ability in Workers? A 1-Year Prospective Study.” BMJ Open 20, 9(3). doi:10.1136/bmjopen-2018-022006.
- Hogan, D. A., B. A. Greiner, and L. O'sullivan. 2014. “The Effect of Manual Handling Training on Achieving Training Transfer, Employee's Behaviour Change and Subsequent Reduction of Work-Related Musculoskeletal Disorders: A Systematic Review.” Ergonomics 57 (1): 93–107. doi:10.1080/00140139.2013.862307.
- Holtermann, A., T. Clausen, B. Aust, O. S. Mortensen, and L. L. Andersen. 2013. “Risk for Low Back Pain from Different Frequencies, Load Mass and Trunk Postures of Lifting and Carrying among Female Healthcare Workers.” International Archives of Occupational and Environmental Health 86 (4): 463–470. doi:10.1007/s00420-012-0781-5.
- Hoogendoorn, W. E., P. M. Bongers, H. C. W. De Vet, G.a.M. Ariëns, W. Van Mechelen, and L. M. Bouter. 2002. “High Physical Work Load and Low Job Satisfaction Increase the Risk of Sickness Absence Due to Low Back Pain: Results of a Prospective Cohort Study.” Occupational and Environmental Medicine 59 (5): 323–328. doi:10.1136/oem.59.5.323.
- Hsieh, H. F., and S. E. Shannon. 2005. “Three Approaches to Qualitative Content Analysis.” Qualitative Health Research 15 (9): 1277–1288. doi:10.1177/1049732305276687.
- Huang, C., W. Kim, Y. Zhang, and S. Xiong. 2020. “Development and Validation of a Wearable Inertial Sensors-Based Automated System for Assessing Work-Related Musculoskeletal Disorders in the Workspace.” International Journal of Environmental Research and Public Health 17 (17): 6050. doi:10.3390/ijerph17176050.
- Ilo . 2015. Global trends on occupational accidents and diseases. World day for safety and health at work.
- Jansen, J. P., H. Morgenstern, and A. Burdorf. 2004. “Dose-Response Relations between Occupational Exposures to Physical and Psychosocial Factors and the Risk of Low Back Pain.” Occupational and Environmental Medicine 61 (12): 972–979. doi:10.1136/oem.2003.012245.
- Kamachi, M., M. Owlia, and T. Dutta. 2021. “Evaluating a Wearable Biofeedback Device for Reducing End-Range Sagittal Lumbar Spine Flexion among Home Caregivers.” Applied Ergonomics 97: 103547. doi:10.1016/j.apergo.2021.103547.
- Knapik, J. J. 1997. “The Influence of Physical Fitness Training on the Manual Material Handling Capability of Women.” Applied Ergonomics 28 (5-6): 339–345. doi:10.1016/S0003-6870(97)00004-5.
- Knight, J. F., and C. Baber. 2005. “A Tool to Assess the Comfort of Wearable Computers.” Human Factors 47 (1): 77–91. doi:10.1518/0018720053653875.
- Korshoj, M., J. H. Skotte, C. S. Christiansen, P. Mortensen, J. Kristiansen, C. Hanisch, J. Ingebrigtsen, and A. Holtermann. 2014. “Validity of the Acti4 Software Using ActiGraph GT3x + Accelerometer for Recording of Arm and Upper Body Inclination in Simulated Work Tasks.” Ergonomics 57 (2): 247–253. doi:10.1080/00140139.2013.869358.
- Kos, A., and A. Umek. 2018. “Smart Sport Equipment: Smartski Prototype for Biofeedback Applications in Skiing.” Personal and Ubiquitous Computing 22 (3): 535–544. doi:10.1007/s00779-018-1146-1.
- Kuorinka, I., B. Jonsson, A. Kilbom, H. Vinterberg, F. Biering-Sørensen, G. Andersson, and K. Jørgensen. 1987. “Standardised Nordic Questionnaires for the Analysis of Musculoskeletal Symptoms.” Applied Ergonomics 18 (3): 233–237. doi:10.1016/0003-6870(87)90010-X.
- Lam, C. F., D. S. Derue, E. P. Karam, and J. R. Hollenbeck. 2011. “The Impact of Feedback Frequency on Learning and Task Performance: Challenging the “More is Better” Assumption.” Organizational Behavior and Human Decision Processes 116 (2): 217–228. doi:10.1016/j.obhdp.2011.05.002.
- Lind, C. M., J. A. Diaz-Olivares, K. Lindecrantz, and J. Eklund. 2020a. “A Wearable Sensor System for Physical Ergonomics Interventions Using Haptic Feedback.” Sensors 20 (21): 6010. doi:10.3390/s20216010.
- Lind, C. M., M. Forsman, and L. M. Rose. 2019a. “Development and Evaluation of RAMP I - a Practitioner's Tool for Screening of Musculoskeletal Disorder Risk Factors in Manual Handling.” International Journal of Occupational Safety and Ergonomics 25 (2): 165–180. doi:10.1080/10803548.2017.1364458.
- Lind, C. M., M. Forsman, and L. M. Rose. 2020b. “Development and Evaluation of RAMP II - a Practitioner's Tool for Assessing Musculoskeletal Disorder Risk Factors in Industrial Manual Handling.” Ergonomics 63 (4): 477–504. doi:10.1080/00140139.2019.1710576.
- Lind, C. M. L. Sandsjö, N. Mahdavian, D. Högberg, L. Hanson, J. A. Diaz Olivares, L. Yang, and M. Forsman. 2019b. “Prevention of Work Related Musculoskeletal Disorders Using Smart Workwear – the Smart Workwear Consortium.”. In Ahram, T., Karwowski, W. & Taiar, R. eds. Human Systems Engineering and Design. Cham, FRA: Springer, 477–483
- Lind, C. M., L. Yang, F. Abtahi, L. Hanson, K. Lindecrantz, K. Lu, M. Forsman, and J. Eklund. 2020c. “Reducing Postural Load in Order Picking through a Smart Workwear System Using Real-Time Vibrotactile Feedback.” Applied Ergonomics 89: 103188. doi:10.1016/j.apergo.2020.103188.
- Liu, L. Y., Y. Li, and A. Lamontagne. 2018. “The Effects of Error-Augmentation versus Error-Reduction Paradigms in Robotic Therapy to Enhance Upper Extremity Performance and Recovery Post-Stroke: A Systematic Review.” Journal of Neuroengineering and Rehabilitation 15 (1): 65. doi:10.1186/s12984-018-0408-5.
- Lötters, F., A. Burdorf, J. Kuiper, and H. Miedema. 2003. “Model for the Work-Relatedness of Low-Back Pain.” Scandinavian Journal of Work, Environment & Health 29 (6): 431–440. doi:10.5271/sjweh.749.
- Madeleine, Pascal., Pernille. Vedsted, Anne Katrine. Blangsted, Gisela. SjøGaard, and Karen. Søgaard. 2006. “Effects of Electromyographic and Mechanomyographic Biofeedback on Upper Trapezius Muscle Activity during Standardized Computer Work.” Ergonomics 49 (10): 921–933. doi:10.1080/00140130600665828.
- Marchet, G., M. Melacini, and S. Perotti. 2015. “Investigating Order Picking System Adoption: A Case-Study-Based Approach.” International Journal of Logistics Research and Applications 18 (1): 82–98. doi:10.1080/13675567.2014.945400.
- Mcatamney, L., and E. N. Corlett. 1993. “RULA: A Survey Method for the Investigation of Work-Related Upper Limb Disorders.” Applied Ergonomics 24 (2): 91–99. doi:10.1016/0003-6870(93)90080-S.
- Meijer, E. M., J. K. Sluiter, and M. H. W. Frings-Dresen. 2009. “Effectiveness of a Feedback Signal in a Computer Mouse on Upper Extremity Musculoskeletal Symptoms: A Randomised Controlled Trial with an 8-Month Follow-up.” Occupational and Environmental Medicine 66 (5): 305–311. doi:10.1136/oem.2008.041483.
- Milot, M.-H., L. Marchal-Crespo, C. Green, S. Cramer, and D. Reinkensmeyer. 2010. “Comparison of Error-Amplification and Haptic-Guidance Training Techniques for Learning of a Timing-Based Motor Task by Healthy Individuals.” Experimental Brain Research 201 (2): 119–131. doi:10.1007/s00221-009-2014-z.
- Nelson, A., M. Matz, F. Chen, K. Siddharthan, J. Lloyd, and G. Fragala. 2006. “Development and Evaluation of a Multifaceted Ergonomics Program to Prevent Injuries Associated with Patient Handling Tasks.” International Journal of Nursing Studies 43 (6): 717–733. doi:10.1016/j.ijnurstu.2005.09.004.
- Nembhard, D. A. 2000. “The Effects of Task Complexity and Experience on Learning and Forgetting: A Field Study.” Human Factors 42 (2): 272–286. doi:10.1518/001872000779656516.
- Nembhard, D. A., and N. Osothsilp. 2002. “Task Complexity Effects on between-Individual Learning/Forgetting Variability.” International Journal of Industrial Ergonomics 29 (5): 297–306. doi:10.1016/S0169-8141(01)00070-1.
- Patterson, J. T., M. J. Carter, and S. Hansen. 2013. “Self-Controlled kr Schedules: Does Repetition Order Matter?” Human Movement Science 32 (4): 567–579. doi:10.1016/j.humov.2013.03.005.
- Pejtersen, J. H., T. S. Kristensen, V. Borg, and J. B. Bjorner. 2010. “The Second Version of the Copenhagen Psychosocial Questionnaire.” Scandinavian Journal of Public Health 38 (3 Suppl): 8–24. doi:10.1177/1403494809349858.
- Punnett, L. 2014. “Musculoskeletal Disorders and Occupational Exposures: How Should we Judge the Evidence concerning the Causal Association?” Scandinavian Journal of Public Health 42 (13 Suppl): 49–58. doi:10.1177/1403494813517324.
- Ribeiro, D. C., G. Sole, J. H. Abbott, and S. Milosavljevic. 2011. “Extrinsic Feedback and Management of Low Back Pain: A Critical Review of the Literature.” Manual Therapy 16 (3): 231–239. doi:10.1016/j.math.2010.12.001.
- Rose, L. M., J. Eklund, L. Nord Nilsson, L. Barman, and C. M. Lind. 2020. “The RAMP Package for Msd Risk Management in Manual Handling - A Freely Accessible Tool, with Website and Training Courses.” Applied Ergonomics 86: 103101. doi:10.1016/j.apergo.2020.103101.
- Schall, M. C., X. Zhang, H. Chen, S. Gallagher, and N. B. Fethke. 2021. “Comparing Upper Arm and Trunk Kinematics between Manufacturing Workers Performing Predominantly Cyclic and Non-Cyclic Work Tasks.” Applied Ergonomics 93: 103356. doi:10.1016/j.apergo.2021.103356.
- Sigrist, R., G. Rauter, R. Riener, and P. Wolf. 2013. “Augmented Visual, Auditory, Haptic, and Multimodal Feedback in Motor Learning: A Review.” Psychonomic Bulletin & Review 20 (1): 21–53. doi:10.3758/s13423-012-0333-8.
- Sluiter, J. K., K. M. Rest, and M. H. W. Frings-Dresen. 2001. “Criteria Document for Evaluating the Work-Relatedness of Upper-Extremity Musculoskeletal Disorders.” Scandinavian Journal of Work Environment & Health 7 (Suppl. 1): 1–102.
- Stefana, E., F. Marciano, D. Rossi, P. Cocca, and G. Tomasoni. 2021. “Wearable Devices for Ergonomics: A Systematic Literature Review.” Sensors 21 (3): 777. doi:10.3390/s21030777.
- Sundstrup, E., K. G. V. Seeberg, E. Bengtsen, and L. L. Andersen. 2020. “A Systematic Review of Workplace Interventions to Rehabilitate Musculoskeletal Disorders among Employees with Physical Demanding Work.” Journal of Occupational Rehabilitation 30 (4): 588–612. doi:10.1007/s10926-020-09879-x.
- Tino, A. M. Carvalho, N. F. Preto, and K. M. Mcconville. 2011. “Wireless Vibrotactile Feedback System for Postural Response Improvement.”. Proceedings of the Annual International Conference of the IEEE Engineering in Medicine and Biology Society. 2012/01/19, 5203–5206.
- Tompa, E. A. Mofidi, S. Van Den Heuvel, T. Van Bree, F. Michaelsen, Y. Jung, L. Porsch, and M. Van Emmerik. 2019. The Value of Occupational Safety and Health and the Societal Costs of Work-Related Injuries and Diseases Luxembourg, LUX: Publications Office of the European Union.
- Van Dieën, J. H., M. J. Hoozemans, and H. M. Toussaint. 1999. “Stoop or Squat: A Review of Biomechanical Studies on Lifting Technique.” Clinical Biomechanics (Bristol, Avon) 14 (10): 685–696. doi:10.1016/s0268-0033(99)00031-5.
- Van Rijn, R. M., B. M. Huisstede, B. W. Koes, and A. Burdorf. 2010. “Associations between Work-Related Factors and Specific Disorders of the Shoulder-a Systematic Review of the Literature.” Scandinavian Journal of Work, Environment & Health 36 (3): 189–201. doi:10.5271/sjweh.2895.
- Verbeek, J. H., K. P. Martimo, J. Karppinen, P. P. Kuijer, E. Viikari-Juntura, and E. P. Takala. 2011. “Manual Material Handling Advice and Assistive Devices for Preventing and Treating Back Pain in Workers.” Cochrane Database of Systematic Reviews 15(6). doi:10.1002/14651858.CD005958.pub3.
- Vignais, N., M. Miezal, G. Bleser, K. Mura, D. Gorecky, and F. Marin. 2013. “Innovative System for Real-Time Ergonomic Feedback in Industrial Manufacturing.” Applied Ergonomics 44 (4): 566–574. doi:10.1016/j.apergo.2012.11.008.
- Wahlström, J., E. Bergsten, C. Trask, S. E. Mathiassen, J. Jackson, and M. Forsman. 2016. “Full-Shift Trunk and Upper Arm Postures and Movements among Aircraft Baggage Handlers.” The Annals of Occupational Hygiene 60 (8): 977–990. doi:10.1093/annhyg/mew043.
- Wang, Q., P. Markopoulos, B. Yu, W. Chen, and A. Timmermans. 2017. “Interactive Wearable Systems for Upper Body Rehabilitation: A Systematic Review.” Journal of Neuroengineering and Rehabilitation 14 (1): 20. doi:10.1186/s12984-017-0229-y.
- Yang, L. D. Borgström, and M. Forsman. 2019. “Validation and Comparison of Three Positioning Protocols of Inertial Measurement Units for Measuring Trunk Movement.”. In Bagnara, S., Tartaglia, R., Albolino, S., Alexander, T. & Fujita, Y. eds. Proceedings of the 20th Congress of the International Ergonomics Association (IEA 2018). Cham: Springer International Publishing, 205–211
- Yassi, A., J. E. Cooper, R. B. Tate, S. Gerlach, M. Muir, J. Trottier, and K. Massey. 2001. “A Randomized Controlled Trial to Prevent Patient Lift and Transfer Injuries of Health Care Workers.” Spine (Phila Pa 1976) 26 (16): 1739–1746.