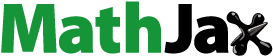
Abstract
Many forestry roles have changed from being manual tasks with a high physical workload to being a machine operator task with a high mental workload. Automation can support a decrease in mental fatigue by removing tasks that are repetitive and monotonous for the operators. Cable yarding presents an ideal opportunity for early adoption of automation technology; specifically the carriage movement along a defined corridor. A Valentini V-850 cable yarder was used in an Italian harvesting setting, in order to gauge the ergonomic benefit of carriage control automation. The study showed that automating yarder carriage movements improved the ergonomic situation of the workers directly involved in the related primary tasks. However, the caveat is that improving one work task may negatively affect the other work tasks, and therefore introducing automation to a worksite must be done after considering all impacts on the whole system.
Practitioner summary: Automation decreased the winch operator’s mental workload while improving overall productivity. At the same time, the mental and physiological workload of the operator tasked with bucking were slightly increased. Ideally, winch automation should be coupled with bucking mechanisation to balance the intervention and boost both operator well-being and productivity.
1. Introduction
Mechanisation has been a dominating trend in forest operations for over a century, at least. A long time has passed since the introduction of the first “steam donkeys’ in the late 1800s (Conway Citation1972), but this trend has continued unabated since then. During all this time, the basic method and aspiration have remained essentially the same: increasing worker productivity by equipping them with more powerful tools. What changed has rather been the specific mode of implementation, which has adapted to technology evolution and to changes in the surrounding physical and social environment. For instance, promising solutions that were too complicated and too costly to implement at an earlier time, such as communication and tracking technologies, have later become a widespread commercial product after low-cost reliable electronics emerged in the 1990s. At the same time, the almost exclusive emphasis on increased productivity has been mitigated by a growing concern for operator safety and general well-being, thus expanding the goals and perspectives of technological progress in forest engineering (Spinelli, Magagnotti, and Labelle Citation2020).
In any case, mechanisation has eventually diminished the physical effort required from logging tasks, while increasing the cognitive and intellectual demands. Modern loggers operate relatively sophisticated machines and generally possess a high level of technical skills, often resulting from extensive formal training. Yet, what was gained in terms of reduced physiological workload may have been partly lost in terms of aggravated mental workload. In particular, the operation of complex equipment often requires a very intensive handling of controls (Gellerstedt Citation2002), which may quickly lead to mental overload. At the same time, performance of monotonous repetitive tasks may generate boredom and frustration, with negative effects on performance and operator well-being (Xie and Salvendy Citation2000; Grzywiński and Hołota Citation2006).
The solution to both problems lays with automation, which may smooth out the peaks and dips of mental demand, by simplifying procedures and delegating to the machine itself the execution of replicates in repetitive tasks. Furthermore, automation can also mitigate the workload derived from multi-tasking, which has become increasingly common due to the growing cost of labour and the consequent need for downsizing logging crews (Baker et al. Citation2014; Di Fulvio et al. Citation2017). As mechanisation increases work capacity, the same worker is asked to perform additional tasks that were originally carried out by other workers. Most often, a machine allows dispensing with an assistant and makes the main operator self-sufficient. However, machines do not have the same capacity as humans for independent intelligent action, and therefore replacement is never complete. The main operator cannot delegate sub-tasks as entirely as he could have done with a human assistant, and therefore he needs to take charge of additional decisional tasks. Multi-tasking may impose a variable strain on an individual’s cognitive abilities, depending on task difficulty and the level of resource overlap (Wickens Citation2008), but the eventual outcome is dictated by simple economics: when demand exceeds capacity, performance will break down (Navon and Gopher Citation1979). Automation can restore the economic balance between effort and work capacity, thus preventing performance decline and operator exhaustion.
Over the past decades, forest technology has made significant progress in the field of machine automation: advanced electronics (e.g. sensors, measuring tools, video feeds) are now an integral component of many forest machines, designed to increase work quality and resource efficiency. On-board electronics also allow automating specific functions and help relieving operator mental workload (Olivera et al. Citation2016). As enabling technologies keep developing, so will the automation of mechanised harvesting systems, eventually leading to the introduction of robots (Thor Citation2014). Today, advanced automation concepts are being explored for specific tasks, such as the development of unmanned forwarders and other ground-based forest technology (Hellström et al. Citation2009; Ringdahl, Hellström, and Lindroos Citation2012; Parker, Bayne, and Clinton Citation2016).
However, the high complexity of the forest environment and of most forest management practices represent a serious challenge to the development of autonomous forest machines (Billingsley, Visala, and Dunn Citation2008). Encouraging results have been obtained with partially-autonomous forwarders, where the driver stores all driving instruction on the on-board computer during the first trip and the machine repeats automatically the same path in the following trips, until the job is completed and a new path must be programmed (Hellstrom and Ringdahl Citation2006). Obviously, autonomous or semi-autonomous operation is easier with such simple tasks as following a pre-defined route in low-traffic areas. After all, that is why most other successful examples of autonomous driving come from mining e.g. Anonymous (Citation2020).
Based on their inherent simplicity, cable yarder set ups offer ideal conditions for partial automation, including a fixed pre-defined route, a repetitive travelling cycle and a relatively short distance that facilitates remote exchanging of large volumes of data between connected devices. That has encouraged experiments with yarder automation at a very early date (Yamada Citation1990; Numata et al. Citation1995). Today, all main European yarder producers already offer automated versions of their machines (Erber and Spinelli Citation2020). Therefore, while the designers of ground-based forest equipment still tackle the technical challenges of developing autonomous versions of their machines, modern yarders could actually be used for testing the benefits of automation, under real work conditions. In particular, yarder operation could offer the opportunity of gauging the workload reduction benefits of loggers engaged with wood extraction tasks.
Therefore, the goal of this study is to determine if the automation of carriage travel in a commercial yarding operation has any effect on the mental workload of workers engaged with yarder operation and other connected tasks. The null hypothesis is that mental workload is the same under manual and automated operation.
2. Materials
2.1. Site
The study took place in a spruce-dominated (Picea abies Karst.) stand in the Eastern Italian Alps, near Arta Terme (Udine, Friuli Venezia-Giulia), coordinates 46.489976°N, 13.021182°E ().
Figure 1. The study location near Arta Terme (Udine, Friuli Venezia-Giulia).
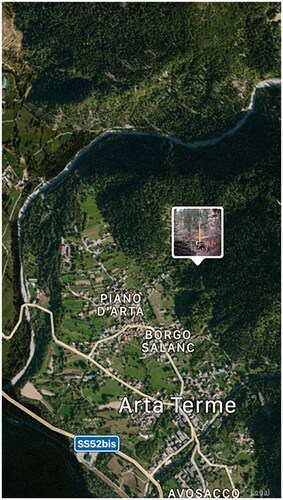
The stand covered 14.7 ha and grew at a mean elevation of 650 m asl. The treatment was a selection cut where all mature spruce trees (average DBH of 33 cm) were removed, while leaving all young trees and mature fir (Abies alba L.) trees. The operation was selected because it was considered generally representative of the environmental, technological and social conditions encountered in the Italian Alps, and with some more approximation of the Alpine region in general (Spinelli, Magagnotti, and Facchinetti Citation2013).
2.2. Equipment
The yarder used on the study site was a Valentini V850 M3 trailer-mounted tower model (), which was a very popular yarder model and had the general technical characteristics of similar models built by competing manufacturers in the region.
Figure 2. Valentini V850 M3 trailer-mounted tower model used to perform the study (photo credit: Giovanna Ottaviani Aalmo).
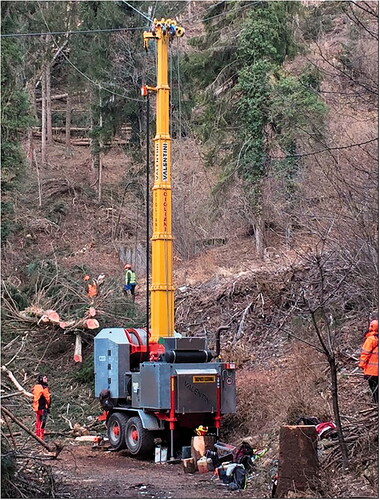
Like all modern yarders, the machine was equipped with a dedicated electronic radio-control system capable of automatic path programming. The system included two receivers installed on the yarder and two transmitters assigned to the main workstations: one transmitter was with the breaker-out at the loading station, and the other with the winch operator at the unloading station (), whereby the receivers were on the carriage (for long distance transmission) and on the Human Machine Interface (HMI) display installed on the winch and designed for adjusting all work settings. The system allowed for programming carriage stops at predefined points along the line, as well as for setting carriage speed and speed ramps on any given skyline segment. All the settings could be monitored on the HMI ().
Figure 3. (a) Details of the transmitter (3a) mounted on the operator’s belt; the monitoring interface on the machine (3b). (b) Details of the transmitter (3a) mounted on the operator’s belt; the monitoring interface on the machine (3b).
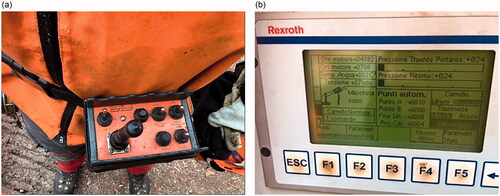
Path-programming allowed launching the carriage and then letting it take care of itself until it reached its predefined stop along the skyline. In turn, that allowed operators to tend other business in the meantime, instead of manually controlling carriage operation all along. For the purpose of the study, the yarder was run each day alternately under the path-programmed mode and the manual-control mode (henceforth: automated and manual, respectively).
The productivity of the system in m3 per productive day is reported in .
Table 1. Productivity of the system in m3/work day (approx. 8 h).
2.3. Operators
Five forest operators (all males; ages ranging from 22 to 49) with sufficient experience and no known health related issues, agreed in participating to this study. Details of the volunteers are reported in . Four to five workers usually composed the crew, but because of family and other work engagements, the crew was reduced to three for 3 occurrences in the eight study days i.e. for the morning’s data collection or for the afternoon’s data collection (for details see showing the crews’ composition for each half-day).
Table 2. Anthropometric characteristics of the five volunteers participating to the study.
Table 3. Scheme of the operators (OP) under observation per task (T) and day.
2.4. Task description
The workers performed the three tasks described below in rotations, so as to have at least two people performing the same type of task:
Task 1 (T1): Yarding – The yarder operator was positioned at the unloading station. He controlled the yarder and unhooked the loads upon arrival. Under the manual treatment he had to use the joysticks on the remote control to bring the carriage to the unloading station, park it over the unloading point and then drop the load; in contrast, under the automated treatment he only had to push a button to authorise unloading, after the carriage had led itself to the unloading station and stopped over the unloading point.
Task 2 (T2): felling/chocker setting – This operator was positioned at the loading station. He felled the trees with a chainsaw and then set the chokers around their butts. Under the manual treatment, he also had to manoeuvre the carriage to the loading position, activate the slack-pulling function and then reverse these commands to hoist the load after setting the chokers. Under the automated treatment, he did not need to maintain constant communication with the winch operator to pass the carriage control, but just tend to his felling business while waiting for the carriage to reach the loading station and park itself over the loading point. That would allow him to focus on other tasks and complete them without urgent time pressure or distraction.
Task 3 (T3): motor-manual processing – This operator stationed at the unloading point. He delimbed and bucked trees using a chainsaw, then stacked the logs with a 12-t Hitachi Zaxis 110 excavator-based loader. Treatment made no visible differences in the demands posed on this operator, who was monitored as a general reference for checking whether operator mental workload was being affected by other factors than treatment. However, this operator could still be indirectly affect by automation, if that changed work pace (increased time pressure) or gave additional time to the winch operator to help with bucking (decreased workload).
The operators performed the task in a rotation starting from the task in which they usually worked. Each day they were changing tasks following the numeric sequence if the safety requirements was allowing it. The crew composition per day and per task is reported in .
The crew only operated under good weather conditions and in the absence of heavy rain, because of safety reasons. That is why data collection was broken into two separate bouts of four days each in the months of February-March, with two weeks in between. Adverse environmental conditions during those two weeks might have confounded the results – and especially Heart Rate Variability (HRV) readings – because sudden slip, trips or falls on slippery terrain would have increased operator strain in a way that was not related to treatment. The average temperature for the days and the hours in which the study took place was 4 °C.
3. Methods
All procedures performed in this study were in accordance with the ethical standards of the Norwegian Research Committee/Regional forskningsetisk komite (REK) and with the 1964 Helsinki Declaration and its later amendments. Because of the non-invasiveness of the procedures and the scope of the study (non-medical), REK did not require an ethical approval but only to implement procedures for informed consent and data storage. All operators agreed to participate in the test voluntarily. They were entitled to withdraw at any time or decline to answer specific questions or complete specific tasks, if desired. Informed consent was obtained from all individual participants involved in the study.
Mental workload was determined with objective and subjective methods.
3.1. EEG-based workload measures (objective)
All participants were provided with the wearable heart rate monitor Bodyguard2® (Firstbeat Citation2015), which they wore 24/7 throughout the two study periods (4 + 4 days) (). The devices were removed only when the participants were showering. The participants were instructed on how to change the patches. Before applying the devices on them for the first time, they were asked to sign an informed consent so that they were aware that they could freely withdraw from the study at any moment.
Figure 4. Positioning of the Bodyguard2® on the operators’ upper body.
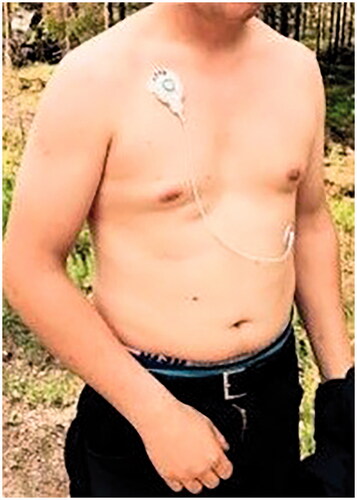
Heart rate (HR) and HRV were measured to assess workload level. HR was measured continuously and recorded at one second intervals. HRV was reported as RMSSD (Root-Mean Square Differences of Successive R-R intervals i.e. the time elapsed between two successive R waves of the QRS signal on the electrocardiogram). RMSSD was elected among all other measures of HRV, because it is identical to SD1 (non-linear measure of HRV; proxy of the unpredictability of a time series) but combines both traditional and non-linear HRV, which makes this indicator more reliable also on short-term measurements (Ciccone et al. Citation2017). Additionally, for readings beyond the 24 h RMSSD is strongly correlated to pNN50 – or the mean number of times an hour in which the change in successive normal sinus (NN) intervals exceeds 50 ms (Bigger et al. Citation1989).
3.2. Self-reported workload measures (subjective)
In order to corroborate the results of the EEG-based measures, a subjective assessment was also implemented, assuming a direct relationship between increasing mental effort and its perception (Longo Citation2017). Self-assessment is supported by many practitioners, based on the notion that only the individual concerned with the task can produce an accurate assessment of the workload he/she has experienced. The NASA Task Load Index (Hart and Staveland Citation1988) method was selected for this purpose. This method accounts for within-task differences between participants, and between-task differences for the same participant. The method uses six subscales to assess mental workload: mental demand, physical demand, temporal demand, performance, effort, and frustration. Each of these subscales was described in detail and presented to the tested subjects before they underwent the test. This study adopted a modified version of the test called ‘RAW-TLX’ which eliminates the weighting process (Hart Citation2006). This was done to simplify the test administration. In this TLX test modification, ratings can be added or averaged, and in this study the latter was preferred. The RAW Nasa TLX test was administered after each work shift.
3.3. Characteristics of the dataset
The dataset was collected over a total of eight days split in two periods of four days each, with a gap of two weeks in between. This was mainly due to adverse weather conditions that hindered the operation.
The original dataset for HR and RMSSD contained 13,641 observations. It was not possible to consider all these observations as independent from each other since they were taken at one second intervals. To reduce this inter-correlation problem, the dataset was reduced by averaging the two response variables (HR and RMSSD) over periods of a half day, with the lunch break in between. That yielded a combination of 16 half-days, 5 people, and 6 treatments. Accounting for the fact that in three cases only 3 people were observed and that not all the 5 people worked the same amount of half-days, consolidation of results yielded 72 observations that were retained for the final analysis. All tasks were performed by one single person not to affect the workload. TIn addition to the heart rate (AVERAGEHR) and the heart rate variability (RMSSD) registered for each volunteer recorded as a dummy variable (SUBJECT), the dataset comprises several other factorial variables to define whether the recording was happening in the morning (AM) or after the lunch break (PM); in which test day the recording was taking place (DAY) and finally which treatment the volunteer were undergoing, with A meaning automated and M manual and 1, 2, 3 being the tasks described above (TREATMENT A1-2-3, M1-2-3). A summary of the dataset is given in .
Table 4. Summary statistics of the variables in the dataset.
3.4. Data analysis
Due to missing combinations, it was impossible to adopt a balanced factorial design, and therefore data was analysed using a model that contained only the main effects of day, time of day and treatment and introduced operator as random factor. That was made on the reasonable assumption that the selected operators were representatives of the local operator population (which is how they were selected) and made it less critical that observations from the same person would be correlated. Therefore, a combination treatment was generated with 6 levels, corresponding to the intersection of machine treatment (manual or automated) with task (T1, T2 and T3).
All data were analysed using a mixed effect model implemented in the R statistical software (Team Citation2013). The following packages were those mostly used to perform the data analysis: lme4 (Bates et al. Citation2014), ggplot2 (Wickham Citation2009) and car (Fox Citation2019). The mixed effect model allowed for modelling the independent variables using the person as a random factor.
We followed the principle of parsimony and designed a model with only the main effects of the fixed factors (day, time of day and treatment) and of the random factor (person), as follows:
where Y was either HR or RMSSD, β0 was a constant (an unknown parameter estimated using the data), ‘Day’ was the effect of day, ‘AM_PM’ was the effect of the time of day, ‘Treatment’ was the effect of treatment (i.e. manual M or automated A), ‘Subject’ was the effect of the person and ɛ was the error term. In addition, the assumptions that were common in such linear mixed models were made: Day, AM-PM and Treatment were fixed factors, Subject was a random factor, by modelling the operators as random factors we accounted for the fact that observations from the same person could be correlated and we underlined the fact that we were not particularly interested in these particular five people involved in this study, but we wanted to look at them as random representatives from a population for which we want the results of the statistical analysis to apply. In all analyses, the elected significance level was α < 0.05.
For the NASA TLX, the median (interquartile range, IQR) score of each of the six workload dimensions was calculated. Each dimension score could range from 0 to10, with 0 being the lowest and 10 the highest for mental demand, physical demand, temporal demand, effort, and frustration, and for performance. 0 equated to perfect and 10 to failure. Overall, workload was calculated for each survey response as the mean of all dimension scores. The Raw-TLX scores were compared using the analysis of variance (ANOVA) with repeated measures and the Bonferroni post-hoc test.
Results
The results of association between treatment and RMSSD estimated by the mixed effect models are shown in . In we report the results for the association between Treatment and AVERAGEHR.
Table 5. Regression results for RMSSD.
Table 6. Regression results for AVERAGEHR.
The RMSSD levels modelled are significantly lower in the manual setting for Task 1 (−8.4022 msec) while for Task 2 was −3.2665 msec and Task 3 was −4.1243 msec. This suggests that operators experienced a higher mental workload when manually operating the yarder and this finding was confirmed by the results of the subjective analysis () where the levels indicated for the overall perceived workload were higher when the setting was manual.
Table 7. NASA TLX Index for Task 1 of the winch operator.
Conversely, operators engaged in Task 3 (log bucking) experienced a reduction of the RMSSD by −6.1449 ms under the automated setting (). This could be explained by the fact that the automatic setting increased work pace in the bucking task.
Figure 5. (a) Boxplot showing the RMSSD and AVEARGEHR responses for each treatment. (b) Boxplot showing the RMSSD (ms) and AVEARGEHR (Bpm) responses for each treatment.
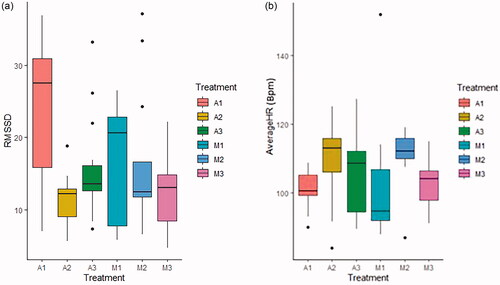
Even if the effect was found not statistically significant, RMSSD was lower in the afternoon, suggesting an increased mental workload regardless of treatment and subjects ().
Table 8. NASA RAW-TLX results for treatment 1 under Manual (M) and Automated (A) conditions for each of the 6 dimensions.
The three different tasks were not associated with a significant increase in heart rate under any of the two machine settings, meaning that the automated treatment did not affect physiological workload (HR), as opposed to mental workload (HRV). Task 2 (felling with chainsaw) incurred a larger HR reading than the average for the other tasks, or +9.2 and +9.9 bpm under the automatic and the manual setting, respectively (). That is most likely related to the heavier physical effort required by the job of feller and breaker-out, both of which are considered extremely tiresome.
The intercept of this model was quite high, as the data analysed corresponded to readings obtained during effective working hours and excluded those readings taken during lunch breaks. No statistically significant association was found between HR readings and the time of the day (AM/PM). Even so, the results suggested that the physiological workload was lower in the afternoon (β = −3.61), probably because work pace slowed down in the afternoon due to fatigue.
The subjective assessment done through the raw NASA-tlx test showed that there was no significant difference between the automated and manual setting of Task 2 (t = 0.18, p = 0.85) and the automated and manual setting of Task 3 (t = 1.89, p = 0.46) and that the six dimensions assessed with this method (Mental Demand, Physical Demand, Temporal Demand, Performance, Effort and Frustration) were perceived at the same intensity. Results for the six dimensions of the Nasa TLX showed higher values for the manual setting (M = 6.3025; SD = 0.0637) then for the automatic (M = 4.3275; SD = 1.6355). Conversely, participants engaged with Task 1 showed a statistically significant difference in their assessment of perceived mental workload (t = −3.19, p = 0.01), which was higher under the manual treatment. Automated winch operation seemed to produce a significant benefit in terms of perceived lower mental demand, physical demand and frustration.
The Bonferroni post hoc test for the six dimension of Task 1 is showed no statistically significant difference in the mean values of the following pairs:
‘Mental Demand’ and ‘Performance,’ ‘Physical Demand’ and ‘Temporal Demand,’ ‘Physical demand’ and ‘Frustration,’ ‘Temporal Demand’ and ‘Effort,’ and also ‘Temporal Demand’ and ‘Frustration.’ For the other possible comparisons, significant differences between means were identified.
Discussion
In the present study, we investigated how forest machine automation could improve the wellbeing of operators. To reach our goal, we have assessed with qualitative and quantitative methods the physical and mental workload experienced by a cable logging crew engaged with three different tasks.
Automation could reduce human mental workload (Young and Stanton Citation1997), which has been confirmed in this study for specific tasks. Alleviating mental workload is crucial to safety: if the mental workload imposed on the operators exceeds their capacity, then the operation becomes uncontrolled and potentially dangerous (Tsang and Vidulich Citation2006). On the other hand, selective alleviation for specific tasks may also lead to an unbalance in task allocation and physical workload, especially when working as a crew. The balance between task demands and operator resources is essential to the safety of any operations (Jorna Citation1991), and such balance must be achieved for the whole team, not just for selected team members.
Furthermore, the study also assessed the physiological workload of operators, since that is likely impacted by automation and may have a crucial effect on safety and wellbeing. Results showed that there was no significant difference in physiological workload between the two treatments for any of the involved operators. In fact, this specific automation measure was not expected to affect physiological workload directly, although it may have done so indirectly – but that was not the case.
Workload unbalance was anticipated because of the availability of additional free time for some of the operators under the automated treatment, which would allow them to engage with additional tasks. Physical overload was expected for tasks one and two, during the time after the operators were pressing the button to automatically move the carriage; once the ‘go’ button was pressed, the carriage would move to the predetermined position without requiring further attention from the operators, who could use this time to perform other tasks, thus increasing their physiological workload, and impacting that of their fellow crew members, due to the effect of assistance or additional time pressure.
For the operators occupied with task three, a physiological overload was anticipated, due to the higher productivity of the system working under automated conditions (Spinelli, Visser, et al. Citation2020). However, the complementarities and proximity of the work stations for Task 1 and Task 3 kept the operators engaged with Task 3 from increasing their physiological workload as they were getting support from the operators assigned to Task 1 when the latter were not occupied with unloading.
For mental workload it was found that the operators engaged in Task 1 experienced an increased mental workload under the manual treatment. In that case, the operators had to devote much attention to proper carriage control, especially when visibility was poor and the carriage was far. In all cases, the operators engaged with Tasks 1 and 2 had to be in constant radio communication, which was paramount to safe and efficient operation but required committing additional cognitive resources, especially in an outdoor environment with obstacles to wave transmission and the confounding background noise of a 175 kW diesel engine. Under those conditions, safety is always a main concern and winch operators must pay the utmost attention not to make any manoeuvring errors. Accidents and injuries are quite common in cable yarding operations: the most recent estimates account for 36 events per million m3 of wood (Tsioras, Rottensteiner, and Stampfer Citation2011) for an Austrian public company known for its high safety standards. Therefore, such a high accident rate is likely higher for private contractors who experience a stronger and more direct financial pressure (Montorselli et al. Citation2010). Any actions leading to a more tolerable level of mental workload across the working day will reduce fatigue, distraction and the risk of accidents. In that regard, it is interesting to look at the variable impact of automation on the different dimensions of mental workload, as expressed by the NASA TLX rating system. The automation measure introduced with the study had a marked impact on physical demand, mental demand and especially frustration – the latter likely derived from the difficulties frequently experienced with visibility and radio communication under operational field conditions. In contrast, very little effect was visible for effort and temporal demand, probably because the effort of manually manoeuvring the carriage was minimal (the controls were simple and commands were sent by pushing buttons on a remote) and the temporal demand was dictated by the other operators’ work pace, rather than by the machine itself. Equally interesting was the fact that the perceived overall performance pressure was higher under the automated treatment, which matched the operator’s expectations of a higher productivity and was actually achieved (Spinelli, Visser, et al. Citation2020).
If the automated treatment had no impact on the mental workload of operators engaged with Task 2 that was because the new treatment did not change their task in any significant way. They would have the carriage delivered near to their station in any case – whether the carriage travelled autonomously or was manoeuvred by someone else. After that, the loading sequence was manual in both cases, and the carriage would be sent back without any further controlling on the part of the operators at the loading site, since the controlling was done by the crew member at the winch (Task 1).
Essentially, the automation measure tested in the study did alleviate the task of the operators it substituted, i.e. those engaged with driving the carriage between the loading and the unloading site. This was the main impact by design; all other impacts were indirect and secondary. This much one would expect: the study just confirmed that the operation was successful in relieving the mental workload of the winch driver, and produced subjective and objective measures for its magnitude. The study also detected the existence and significance of indirect secondary effects on the other team members, which was also important information. Every action determines a chain of reactions – primary and secondary – and a properly conducted impact assessment must account for all of them. In this case, the relief experienced by operators engaged with Task 1 was eroded in small part by an aggravation for the operators engaged with Task 3, due to the indirect effect of a faster work pace. This could be potentially solved by introducing better equipment for the bucking station (Task 3) so that the operator assigned to this task could more easily cope with the increased work flow. In particular, one may envisage fitting the excavator-based loader with a processor head to mechanise all bucking, in which case Task 3 would proceed very fast and with minimal physiological workload for the operators engaged with it. Ideally, one may combine carriage automation and mechanised bucking and merge the two work stations into one, with one single operator performing Tasks 1 and 3, that is: driving the bucking machine once he has sent the carriage on its way, and interrupting the bucking task once the carriage comes back loaded and prompts the request for unloading. This would definitely cut cost and would sound attractive to most entrepreneurs with the financial capacity for purchasing the new machine, but further studies should determine whether the mental aggravation caused by multi-tasking (Task 1 and 3 assigned to the same operator) will be offset by a simpler work procedure and a more comfortable work station.
The study also hints at generally increased mental workload levels in the afternoon, hypothetically related to difficulties in concentration derived from the accumulation of fatigue and/or the classic after-lunch energy level drop. This is an important warning sign, consistent with the findings of Tsioras, Rottensteiner, and Stampfer (Citation2011), who observed a spike in cable logging accident events between 2:00 and 3:00 pm. If the root cause of the observed accident peak is fatigue accumulation, any measures to reduce fatigue may help with prevention. However, more general preventive measures will likely yield a general reduction of accident rates, not a targeted smoothing of spikes, which must be achieved by manipulating the rest pause schedule.
Finally, we need to issue a caveat on the small number of operators gauged in this study: ideally, one should have included a larger number of subjects in order to warrant safe generalisation of results. While all test operators were chosen for being generally representative of the larger pool available in the region, they were not administered specific psychological tests and therefore one cannot be sure that there would be no differences in psychological behaviour and load tolerance between the different operators recruited for the study, and between them and the larger pool of operators under investigation. For that reason, readers are invited to use the results of this study with some caution, until validated by future larger follow-up studies.
Conclusions
Automation can increase team productivity, reduce operator fatigue and improve work safety, and this study offers just one more witness to that general rule by using a very simple intervention that can be implemented by most entrepreneurs at a moderate cost. With the limitations related to the number of volunteers in this study, our research has shown that there is a significant difference in the mental workload measurements under manual and automated operation unlikely what stated in the null-hypothesis.
Within this context, the study illustrates quite effectively two fundamental points that have a strong impact on efficiency and safety, and namely: first, that a single intervention may not be able to release the full potential of automation, and second, that the impact of any intervention must evaluated for the whole operation, not the individual work station. While automated path programming did speed up the operation, it also moved the strain (and the risk) from the winch operator (Task 1) to the processor operator (Task 3), which could only be resolved by improving the processor operator work station with the introduction of a modern mechanical processor. Hence, a more effective automation intervention would have resulted from the introduction of automated path programming together with that of a new processor, which would have amounted to improving the automation of both Task 1 and Task 3.
Despite technology advancement, forestry is still an industry where worldwide 170,000 accidents are happening yearly, leading to a reduced work capacity directly and indirectly as the industry becomes less appealing for younger generations (Garland Citation2018). There is a need for developing new non-technical skills (Flin, O'Connor, and Crichton Citation2008) and for adopt tools for fatigue assessment and reduction, so that the forest industry may retain the existing workforce and attract new recruits.
Disclosure statement
No potential conflict of interest was reported by the author(s).
References
- Anonymous. 2020. “The Fully Autonomus Scania AXL.” https://www.scania.com/uk/en/home/experience-scania/features/axl.html.
- Baker, S.A., B. Mei, T.G. Harris, and W.D. Greene. 2014. “An Index for Logging Cost Changes across the US South.” Journal of Forestry 112 (3): 296–301. doi:10.5849/jof.13-055.
- Bates, D., M. Mächler, B. Bolker, and S. Walker. 2014. “Fitting Linear Mixed-Effects Models Using lme4.” arXiv preprint arXiv:1406.5823
- Bigger, J.T., Jr, P. Albrecht, R.C. Steinman, L.M. Rolnitzky, J.L. Fleiss, and R.J. Cohen. 1989. “Comparison of Time-and Frequency Domain-Based Measures of Cardiac Parasympathetic Activity in Holter Recordings after Myocardial Infarction.” The American Journal of Cardiology 64 (8): 536–538. doi:10.1016/0002-9149(89)90436-0.
- Billingsley, J., A. Visala, and M. Dunn. 2008. “Robotics in Agriculture and Forestry.” In Springer handbook of robotics, edited by B. Siciliano and O. Khatib. Berlin: Springer.
- Ciccone, A.B., J.A. Siedlik, J.M. Wecht, J.A. Deckert, N.D. Nguyen, and J.P. Weir. 2017. “Reminder: RMSSD and SD1 Are Identical Heart Rate Variability Metrics.” Muscle & Nerve 56 (4): 674–678. doi:10.1002/mus.25573.
- Conway, M. 1972. “A Forest Service Approach to Planning.” National Parks Planning Symposium20-23 Ago 1970Canterbury (Nueva Zelandia), Department of Land and Survey, Wellington (Nueva Zelandia).
- Di Fulvio, F., D. Abbas, R. Spinelli, M. Acuna, P. Ackerman, and O. Lindroos. 2017. “Benchmarking Technical and Cost Factors in Forest Felling and Processing Operations in Different Global Regions during the Period 2013–2014.” International Journal of Forest Engineering 28 (2): 94–105. doi:10.1080/14942119.2017.1311559.
- Erber, G, and R. Spinelli. 2020. “Timber Extraction by Cable Yarding on Flat and Wet Terrain: A Survey of Cable Yarder Manufacturer’s Experience.” Silva Fennica 54 (2): 10211. doi:10.14214/sf.10211.
- Firstbeat. 2015. Accessed 14 February 2015. http://www.firstbeat.com/work-well-being/product-overview.
- Flin, R.H., P. O'Connor, and M. Crichton. 2008. Safety at the Sharp End: A Guide to Non-Technical Skills. Farnham: Ashgate Publishing, Ltd.
- Fox, J. 2019. “John Fox and Sanford Weisberg.” An R Companion to Applied Regression. 3rd ed. Thousand Oaks, CA: Sage Publications.
- Garland, J.J. 2018. “Accident Reporting and Analysis in Forestry: Guidance on Increasing the Safety of Forest Work.” Forestry Working Paper FAO 2.
- Gellerstedt, S. 2002. “Operation of the Single-Grip Harvester: motor-Sensory and Cognitive Work.” International Journal of Forest Engineering 13 (2): 35–47. doi:10.1080/14942119.2002.10702461.
- Grzywiński, W, and R. Hołota. 2006. “Subjective Assessment of the Fatigue of Forest Workers Based on Japanese Questionnaire.” Acta Scientiarum Polonorum Silvarum Colendarum Ratio et Industria Lignaria 5 (1): 27–37.
- Hart, S.G. 2006. “NASA-Task Load Index (NASA-TLX); 20 Years Later.” Proceedings of the Human Factors and Ergonomics Society Annual Meeting 50 (9): 904–908. doi:10.1177/154193120605000909.
- Hart, S.G, and L.E. Staveland. 1988. “Development of NASA-TLX (Task Load Index): Results of Empirical and Theoretical Research.” Human Mental Workload 1: 139–183.
- Hellstrom, T, and O. Ringdahl. 2006. “Follow the past: A Path-Tracking Algorithm for Autonomous Vehicles.” International Journal of Vehicle Autonomous Systems 4 (2/3/4): 216–224. doi:10.1504/IJVAS.2006.012208.
- Hellström, T., P. Lärkeryd, T. Nordfjell, and O. Ringdahl. 2009. “Autonomous Forest Vehicles: Historic, Envisioned, and State-of-the-Art.” International Journal of Forest Engineering 20 (1): 31–38. doi:10.1080/14942119.2009.10702573.
- Jorna, P. 1991. “Operator Workload as a Limiting Factor in Complex Systems.” In Automation and Systems Issues in Air Traffic Control, edited by J. A. Wise, V. David Hopkin, and M. L. Smith, 281–292. Cham: Springer.
- Longo, L. 2017. “Subjective Usability, Mental Workload Assessments and Their Impact on Objective Human Performance.” IFIP Conference on Human-Computer Interaction, Springer.
- Montorselli, N.B., C. Lombardini, N. Magagnotti, E. Marchi, F. Neri, G. Picchi, and R. Spinelli. 2010. “Relating Safety, Productivity and Company Type for Motor-Manual Logging Operations in the Italian Alps.” Accident Analysis & Prevention 42 (6): 2013–2017. doi:10.1016/j.aap.2010.06.011.
- Navon, D, and D. Gopher. 1979. “On the Economy of the Human-Processing System.” Psychological Review 86 (3): 214–255. doi:10.1037/0033-295X.86.3.214.
- Numata, K., K. Kanzaki, Y. Suzuki, and D. Chu. 1995. “Application of Automatic Cable System to Forest Environment Observation – Positioning Precision of Carriage and Gondola with Instrument Units.” Japanese Forestry Society 106: 545–548.
- Olivera, A., R. Visser, M. Acuna, and J. Morgenroth. 2016. “Automatic GNSS-Enabled Harvester Data Collection as a Tool to Evaluate Factors Affecting Harvester Productivity in a Eucalyptus spp. Harvesting Operation in Uruguay.” International Journal of Forest Engineering 27 (1): 15–28. doi:10.1080/14942119.2015.1099775.
- Parker, R., K. Bayne, and P.W. Clinton. 2016. “Robotics in Forestry.” NZ Journal of Forestry 60 (4): 9.
- Ringdahl, O., T. Hellström, and O. Lindroos. 2012. “Potentials of Possible Machine Systems for Directly Loading Logs in Cut-to-Length Harvesting.” Canadian Journal of Forest Research 42 (5): 970–985. doi:10.1139/x2012-036.
- Spinelli, R., N. Magagnotti, and D. Facchinetti. 2013. “Logging Companies in the European Mountains: An Example from the Italian Alps.” International Journal of Forest Engineering 24 (2): 109–120. doi:10.1080/14942119.2013.838376.
- Spinelli, R., N. Magagnotti, and E.R. Labelle. 2020. “The Effect of New Silvicultural Trends on Mental Workload of Harvester Operators.” Croatian Journal of Forest Engineering 41 (2): 177–190. doi:10.5552/crojfe.2020.747.
- Spinelli, R., R. Visser, N. Magagnotti, C. Lombardini, and G.O. Aalmo. 2020. “The Effect of Partial Automation on Cable Yarder Productivity and Cost.” Annals of Forest Research 63 (2): 3–14. doi:10.15287/afr.2020.1883.
- Team, R.C. 2013. “R: A Language and Environment for Statistical Computing.” http://www.R-project.org/
- Thor, M. 2014. Prospects and Challenges for Forest Harvesting Technologies in Europe. Gerardmere, France: FEC2014.
- Tsang, P.S, and M.A. Vidulich. 2006. “Mental Workload and Situation Awareness.” In Handbook of Human Factors and Ergonomics, edited by G. Salvendy, pp. 243–268. New York: John Wiley & Sons, Inc.
- Tsioras, P.A., C. Rottensteiner, and K. Stampfer. 2011. “Analysis of Accidents during Cable Yarding Operations in Austria 1998–2008.” Croatian Journal of Forest Engineering: Journal for Theory and Application of Forestry Engineering 32 (2): 549–560.
- Wickens, C.D. 2008. “Multiple Resources and Mental Workload.” Human Factors 50 (3): 449–455. doi:10.1518/001872008X288394.
- Wickham, H. 2009. “Elegant Graphics for Data Analysis.” Media 35 (211): 10–1007.
- Xie, B, and G. Salvendy. 2000. “Review and Reappraisal of Modelling and Predicting Mental Workload in Single-and Multi-Task Environments.” Work & Stress 14 (1): 74–99. doi:10.1080/026783700417249.
- Yamada, S. 1990. “A Full-Automatic Yarder System Equipped with Electronic Devices.” Journal of the Forest Mechanization Society 440: 23–28.
- Young, M.S, and N.A. Stanton. 1997. “Automotive Automation: Investigating the Impact on Drivers’ Mental Workload.” International Journal of Cognitive Ergonomics, 1 (4), 325–336.