Abstract
Interest in Maritime Autonomous Surface Ships (MASS) is increasing as it is predicted that they can bring improved safety, performance and operational capabilities. However, their introduction is associated with a number of enduring Human Factors challenges (e.g. difficulties monitoring automated systems) for human operators, with their ‘remoteness’ in shore-side control centres exacerbating issues. This paper aims to investigate underlying decision-making processes of operators of uncrewed vehicles using the theoretical foundation of the Perceptual Cycle Model (PCM). A case study of an Unmanned Aerial Vehicle (UAV) accident has been chosen as it bears similarities to the operation of MASS through means of a ground-based control centre. Two PCMs were developed; one to demonstrate what actually happened and one to demonstrate what should have happened. Comparing the models demonstrates the importance of operator situational awareness, clearly defined operator roles, training and interface design in making decisions when operating from remote control centres.
Practitioner Summary: To investigate underlying decision-making processes of operators of uncrewed vehicles using the Perceptual Cycle Model, by using an UAV accident case study. The findings showed the importance of operator situational awareness, clearly defined operator roles, training and interface design in making decisions when monitoring uncrewed systems from remote control centres.
1. Introduction
Interest in Maritime Autonomous Surface Ships (MASS) is rapidly growing as they bring many benefits including improvements to safety and reducing operational costs by removing the need for onboard operators (Ahvenjärvi Citation2016; Burmeister et al. Citation2014b; Chang et al. Citation2021; Kretschmann, Burmeister, and Jahn Citation2017; Thieme and Utne Citation2017). Uncrewed ships would also bring benefits to the operators by giving them a better and safer working environment from a shore-based or host-ship Remote Control Centre (RCC; Wahlström et al. Citation2015). Their introduction may also reduce the number of operators required for each ship and operators may be able to monitor multiple MASS from the RCC (Mackinnon et al. Citation2015). MASS applications include search and rescue (Mendonça et al. Citation2016), recovery of oil spills (Wang et al. Citation2016), hydrographic surveys (Dobref et al. Citation2018; Giordano et al. Citation2015) and military operations (Deng et al. Citation2018; Osga and McWilliams Citation2015).
The International Maritime Organisation (IMO) has outlined four degrees of autonomy for MASS (International Maritime Organisation Citation2021):
Degree one: ships with automated systems and decision support systems that are used by onboard personnel.
Degree two: the ships are remotely controlled with seafarers still onboard.
Degree three: the ships are uncrewed and remotely controlled.
Degree four: full autonomous ships where the system will make decisions on its own and choose the appropriate action.
Within these degrees of autonomy different levels of automation may be used ranging from the operator manually controlling the ship to the ship’s systems acting autonomously without the human operator’s input, these levels are not necessarily hierarchical and a MASS may use multiple levels during a voyage (International Maritime Organisation Citation2021). However, these degrees of autonomy do not necessarily fully describe the human involvement in the system, the European Defence Agency’s Safety and Regulations for European Unmanned Maritime Systems (SARUMS) group have defined six levels of control (see ) which could be used operating a MASS with degree three of autonomy. These levels of control could range from level one (directly operated by a human operator) to level five (the system operates autonomously with no human involvement) (Maritime UK 2020). However, it is expected that to meet regulatory requirements that there will still need to be a human in the system, the operator would then be responsible for monitoring the automated systems and intervening if required (Huang et al. Citation2020; Ramos, Utne, and Mosleh Citation2019).
Table 1. Levels of control for MASS operation (Maritime UK 2020).
The level of control used is important as it describes the system’s ability to operate without human input, showing that at higher levels of control or automation the operator may be less involved in the decision-making process as the systems are more highly automated (Scharre Citation2015; Vagia, Transeth, and Fjerdingen Citation2016). Whether the intention is for the MASS decision-making to have a high potential impact (e.g. collision avoidance navigation) or as an operator aid (e.g. proposing actions for a human operator to approve) it is critical to ensure that the information available to the operator is appropriate (Scharre Citation2015). It will be necessary to consider the level of automation of a ship when designing its systems, as it will affect the information required by human operators to maintain their situational awareness and what their responsibilities within the system are (Man et al. Citation2018). It has been found that automating decision-making functions can have a detrimental effect on a system’s performance (Parasuraman Citation2000).
Whilst uncrewed MASS are expected to bring many benefits (e.g. Norris Citation2018; Wahlström et al. Citation2015), there are also Human Factors challenges related to their operation (Hoem Citation2020; Man et al. Citation2018; Wahlström et al. Citation2015). For instance, the introduction of MASS has the potential to reduce operator workload (Porathe, Prison, and Man Citation2014; Wahlström et al. Citation2015). However, MASS may increase operator workload due to the vast amounts of data that will become available from all the ship’s sensors (Porathe, Prison, and Man Citation2014; Wahlström et al. Citation2015). Further, an operator’s workload may also be affected by the usability of the automated systems, and so it will be important that they are designed to support operators’ decision-making (Karvonen and Martio Citation2019). As MASS will be remotely operated or monitored from a shore-side or host ship RCC, operators may have limited situational awareness due to no longer being on board affecting their ability to make appropriate decisions (Mackinnon et al. Citation2015; Porathe, Prison, and Man Citation2014).
Another issue for decision-making could be less time for operators to make decisions at an RCC due to the potential delay in receiving information from the ship (Ramos et al. Citation2020). There may be a delay in the transmission of information from the ship because of the distance between the ship and the RCC and the time taken for the systems to process data transmitted from the onboard sensors (Wahlström et al. Citation2015). It has been suggested that the human-machine interface (HMI) will be critical for performance and safety, ensuring the operator maintains their situational awareness and, in the loop, should they need to take over (Ahvenjärvi Citation2016). There may be challenges in supporting the operator monitor the MASS’ automated systems if they do not have an appropriate level of reliance on the automation (Parasuraman and Riley Citation1997). Human operators may rely on the automated system too heavily if they believe the automated system is more capable and/or reliable than it actually is, so they may believe that the system does not have to be monitored as rigorously (Parasuraman and Riley Citation1997).
It is envisaged that automated systems will be more reliable and can respond more quickly to situations than their human counterparts (Ahvenjärvi Citation2016; Norris Citation2018; Ramos et al. Citation2020). However, despite uncrewed systems having the potential to reduce error as the onboard human operator is removed, there is some concern that automation may create new avenues for error (e.g. Ahvenjärvi Citation2016; Burmeister et al. Citation2014b). This is because the role of the human operator is not entirely removed from the system, it is simply changed from an active operator to a supervisor of the system (Ahvenjärvi Citation2016; Hoem Citation2020). The errors that might be introduced may be new and potentially more complex, due to the complexity of the automated systems involved (Ahvenjärvi Citation2016; Burmeister et al. Citation2014b; Hoem Citation2020). This may have safety implications for MASS as it may then be harder for the human operator to diagnose and respond appropriately to a system failure or unexpected situation, so it may not be as simple as removing the human element from onboard to make systems safer (Sandhåland, Oltedal, and Eid Citation2015).
Similar challenges and issues have already been seen in Unmanned Aerial Vehicles (UAVs) operation due to their similar operation to MASS (Wahlström et al. Citation2015). MASS and UAV have various operational similarities:
The uncrewed vehicles are operated from RCCs, ground RCCs for UAVs and shore-side or host-ship RCCs for MASS (Ahvenjärvi Citation2016; Gregorio et al. Citation2021; Man, Lundh, and MacKinnon Citation2018; Skjervold Citation2018)
There is a lack of proximity between the uncrewed vehicle and the operator, operators rely on sensor feedback (Karvonen and Martio Citation2019; Pietrzykowski, Pietrzykowski, and Hajduk Citation2019)
The vehicles are operated and monitored through HMIs (Liu, Aydin, et al. Citation2022; Zhang, Feltner, et al. Citation2021)
At higher levels of automation, operators will be in a supervisory role (Man, Lundh, and MacKinnon Citation2018; Zhang, Guo, et al. Citation2021)
However, there are also differences between the aviation and maritime domains such as:
Slower speeds in the maritime domain versus aviation, so decisions are less time critical (Praetorius et al. Citation2012)
Maritime navigational decisions need to be made earlier due to the high inertia of ships and because they are underactuated as they have fewer inputs than degrees of freedom (Reyhanoglu Citation1997; Veitch and Andreas Alsos Citation2022)
The aviation domain navigation is in the three dimensions and in maritime only in two dimensions (Praetorius et al. Citation2012)
These differences may limit the applicability of insights from UAV operations for MASS. Even though there are differences in operating MASS and UAVs from RCCs, the similarities in their operation mean that it is useful to consider the remote operating problems already experienced with UAVs and understand what lessons could be learnt for MASS.
It is expected that there will be multiple operator roles in the RCC, such as an operator monitoring the ship, an engineer and other team members, however, this would depend on the type of mission being carried out and the complexity of the MASS (Burmeister et al. Citation2014b; Mackinnon et al. Citation2015; Man et al. Citation2015; Saha Citation2021). Multiple operator teams are also used in the operation of UAVs, such as multiple pilots, sensor/payload operators, an operation commander and other support personnel depending on the type of missions and complexity of the systems in use (Armstrong, Izzetoglu, and Richards Citation2020; Giese, Carr, and Chahl Citation2013; Gregorio et al. Citation2021; Man et al. Citation2015; Ruiz et al. Citation2015). For example, for the operation of surveillance UAVs teams of two are often used, a pilot and a video feed operator (Helton et al. Citation2015). It has also been suggested that there are three main roles in UAV operation, an operations director (for planning and coordination), a pilot and a mission specialist (in charge of operating the onboard sensors) (Peschel and Murphy Citation2015). As technological capabilities advance, it is expected that single operators will be responsible for monitoring multiple uncrewed MASS (Andersson et al. Citation2021; Fan et al. Citation2020; Ramos, Utne, and Mosleh Citation2019). Similarly, the use of a single operator to control multiple UAVs is being investigated as the automated systems become more advanced leaving operators to supervise the UAVs (Jessee et al. Citation2017; Lim et al. Citation2021; Silva et al. Citation2017). Currently, the use of UAV swarms monitored by a single operator is being explored (Hocraffer and Nam Citation2017).
In aviation, there have been numerous accidents involving UAVs (Giese, Carr, and Chahl Citation2013). A study reviewed 68 US military UAV accidents between 2011 and 2014, it was found that 38% of the casual factors were categorised as unsafe acts and 53% of those unsafe acts were due to judgement and decision errors (Oncu and Yildiz Citation2014). It is important to note that human error should not be used to explain the cause of an accident it should be the starting point for an investigation into why the error occurred (Dekker Citation2006). Due to the similarities in the operation of both UAVs and MASS, similar decision errors could likely be seen in the operation of MASS from RCC. It shows the importance of investigating the decision-making process in the operation of uncrewed systems, to understand why these decision errors were made and what can be done in terms of system design to better support operator decision-making. It has been highlighted that operators will still play an important part in the performance of the whole system, as they will be a backup if the automated systems fail and will need to be able to make appropriate decisions in potentially safety-critical situations (Kari and Steinert Citation2021; Man et al. Citation2015).
This paper aims to investigate decision-making in uncrewed vehicle operation to understand the issues associated with operating uncrewed vehicles from RCCs. This will be achieved by using a UAV accident as a case study to develop a Perceptual Cycle Model (PCM; Neisser Citation1976) of the operator’s decision-making process. This will enable us to explore how the operators came to their decisions in the context of the system and environment, rather than just looking at their erroneous decisions. It is important to consider why these decisions made sense to the operators at the time they were made and what were the underlying mechanisms supporting their decisions. The analysis of the decision-making model developed will then be used to infer potential design recommendations for MASS systems, to better support the decision-making of the human operators. It will also be used to investigate what lessons could be learnt from operating UAVs from ground-based RCCs and how their design influenced the human-machine team decision-making process, to gain insights for the design of MASS RCCs.
2. The perceptual cycle model
The PCM is a naturalistic decision-making model developed by Neisser (Citation1976), which links the decision-maker’s thoughts with information available within the world (or environment), which goes on to influence their behaviour and actions. The concept of ‘schema’ is used to describe organised mental templates of a decision maker’s thoughts or behaviours based on their past experience and world knowledge (Plant and Stanton Citation2012). The PCM is a cyclical model (see ) in which world information that is available to the decision-maker modifies their perception of the environment and as a result may trigger their schema (Plant and Stanton Citation2012). This then directs the actions they take and then more information about the situation may be available to them as a result of their actions or due to the dynamic situation and the cycle begins again (Plant and Stanton Citation2012). Their schema is activated by bottom-up processing as the decision-maker uses the world information to match their past experience, which then modifies their perception of the situation and leads them to potential actions they may take (top-down processing) (Banks, Plant, and Stanton Citation2018). One feature of naturalistic decision-making is that decisions are constantly being made due to the developing dynamic situation, the decision-maker will go round the PCM multiple times as the situation evolves. The decision-maker’s schema may be adapted, or an alternative schema may be triggered. As the situation develops, the decision-maker’s potential actions may also be altered (Revell et al. Citation2020).
Figure 1. The perceptual cycle model (Neisser 1976; Plant and Stanton Citation2012).
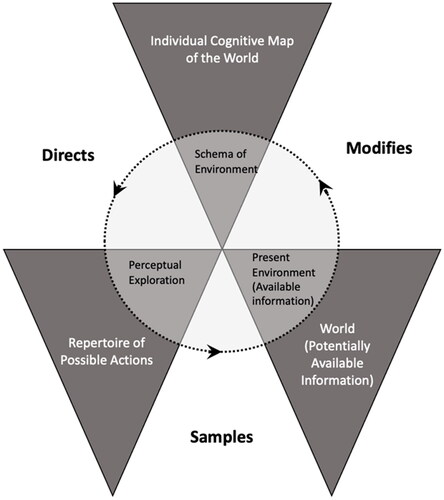
The PCM will be used to explore decision-making in a UAV accident to investigate human-system relationships in the operation of uncrewed vehicles. To understand what can affect operators’ decision-making processes when operating uncrewed vehicles from RCCs and gain insights into how MASS operators’ decision-making could be supported. Even though at higher levels of autonomy the MASS operator’s main responsibilities will be to monitor the ship’s automated systems, their decision-making will still be important in the safe operation of MASS, as they will need to handle alerts given by the automated systems and intervene when the systems cannot handle a situation (Man et al. Citation2015). For example, the operator may need to be involved in navigation decisions due to the complexity of a situation or in the event of a component or system failure (Burmeister et al. Citation2014a; Liu, Chu, et al. Citation2022; Ramos et al. Citation2020). Therefore, it will be necessary to investigate how the decision-making process of MASS operators can be supported to promote the safe operation of MASS.
This decision-making model has been chosen as it has been previously used to investigate incidents involving human-system interactions using case studies such as the Kegworth Plane crash (Plant and Stanton Citation2012), the Kerang rail crash (Salmon et al. Citation2013) and the Ladbroke Grove rail crash (Stanton and Walker Citation2011). Also, studies such as Banks et al. (Citation2018) have used the PCM framework to investigate an accident involving other another automated system, an automated vehicle accident. The accident which occurred on the 7th May 2016 involved a Telsa Model S which was being operated in Auto-pilot mode that crashed into a trailer that was crossing an intersection on a highway west of Williston, Florida (Banks, Plant, and Stanton Citation2018). In this case, the PCM framework was used to show how internally held schema can influence a decision-maker’s actions and how potentially inappropriate schema of automated systems and their reliability can lead to fatal accidents (Banks, Plant, and Stanton Citation2018). This accident highlighted the issue of responsibility when humans use automated systems and how task allocation between the human and the system needs to be clear so humans are aware of their responsibility (Banks, Plant, and Stanton Citation2018). However, Banks et al., (Citation2018) also demonstrated that vehicle manufacturers also have a degree of responsibility to support and facilitate the development of appropriate schema, so that appropriate levels of trust are developed for the automated systems they design.
As shown by others (e.g. Plant and Stanton Citation2012; Banks et al. Citation2018), the PCM framework can be used to explore system error in the human-system relationships, as it puts the human decision-makers thought process into the system context. The PCM framework shows how their decisions made sense at the time based on the information available and their interactions with the automated system (Plant and Stanton Citation2012). Plant and Stanton (Citation2015) proved the validity and re-test reliability of the PCM by showing that it is valid for representing real-life data using the example of rotary wing pilots. By exploring system errors in this way, it can show what led the decision-makers to their decisions, to understand how their decision-making can be further supported in the context of the system they are operating. The application of a UAV case study to the PCM will be used to investigate the system errors that occurred in this case, to understand how similar issues might be prevented in the operation of MASS from RCCs and how MASS operator decision-making could be supported.
3. Case study of an unmanned aerial vehicle accident
3.1. WK050 accident synopsis
The Watchkeeper (WK) is a UAV which is used for intelligence surveillance reconnaissance and is operated from a Ground Control Station (GCS; Defence Safety Authority Citation2019). On 13th June 2018, a team of pilots were completing their Captaincy Development training at West Wales Airport. Each pilot was required to complete three flights in the first pilot role (pilot 1; who’s in charge of monitoring the UAV’s systems) and three in the second pilot role (pilot 2; who focuses on the reconnaissance mission). Six test flights were planned, however on the fourth flight, an accident occurred during the landing attempt. A comprehensive accident investigation was led by the Defence Safety Authority to establish the root cause of the accident, culminating in the publication of an accident report in February 2019 (Defence Safety Authority Citation2019). Whilst the training exercise took place there were five team members in the GCS, the two pilots, an aircrew instructor, a spare pilot and a flight execution log author, see for the control room layout.
Figure 2. Line drawing of the Ground Control Station setup.
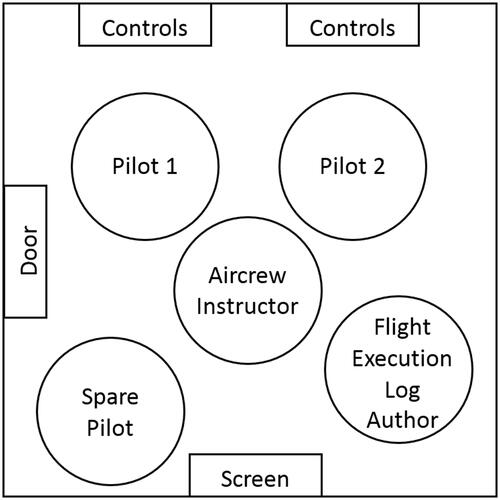
According to the accident report, the first pilot (pilot 1) had decided to land the UAV earlier than planned due to high crosswind gusts, as it was anticipated the high crosswinds may lead to several go-arounds before the UAV landed successfully. The accident report outlines that during the UAV’s landing, the UAV’s Automatic Take-Off and Landing System (ATOLS) did not sense ground contact when it touched down on the runway. As it had not sensed ground contact, the ATOLS decided to auto-abort the landing attempt and perform a go-around. However, during the landing attempt, the UAV had deviated from the runway’s centreline and after the UAV touched down it continued to deviate onto the grass. The pilots had observed this deviation onto the grass from the UAV video feed and tried to manually abort the landing. This was four seconds after the UAV’s ATOLS had made the decision to abort the landing (see ), so when the pilot tried to manually abort the landing there was no response from the UAV. Two seconds later the pilot decided to cut the UAV’s engine. The cause of the accident was found to be the pilot cutting the engine and the GCS crew losing situational awareness was found to be one of the causal factors in the accident (Defence Safety Authority Citation2019). shows a summary of the UAV accident showing the path of the UAV during the landing attempt, the landing stages and the points at which the system and manual commands were given. shows a summarised timeline of the events leading to the accident, which has been broken down into four phases: landing initiated, landing approach, ground touch window and emergency handling (Defence Safety Authority Citation2019). It is important to understand the limited time sampling window the GCS crew had at each stage during the landing attempt. shows that pilot 1 decided to manually abort the landing four seconds after the ATOLS had already made the decision to abort the landing, then decided to cut the engine two seconds later. The decision to cut the engine had only been made nine seconds after the UAV had been seen deviating from the runway. The emergency handling stage was only six seconds long, showing the short time in which, the decision was made.
Figure 3. UAV’s path on the runway, the system alerts and manual commands given during the landing attempts. See figure for the timeline of each of the landing stages.
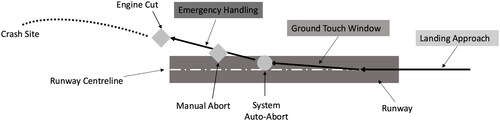
Figure 4. Timeline of the WK050 accident (Defence Safety Authority Citation2019). Note: Automatic Take-off and Landing System (ATOLS).
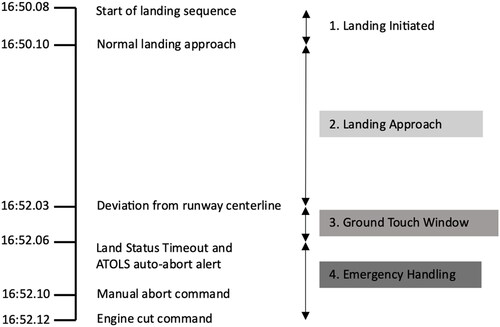
Often in the case of accidents like the WK050, the operators of these systems are found to be at fault and ‘human error’ is the cause of the accident (Dekker Citation2001; Woods Citation2010). However, it is important to consider the wider system context in these cases to understand why the accident occurred and why the operators made the decisions that they did, rather than just looking at the decisions they made (Dekker Citation2001). In the maritime domain ‘human error’ is often sighted as the cause of accidents but it is necessary to understand the root cause of why these errors were made (Chang et al. Citation2021; Kaptan et al. Citation2021; Luo and Shin Citation2019). Although MASS are expected to remove some avenues of human error due to the change in the role of the human operator there is potential for new errors to emerge and it will be necessary to consider how higher levels of automation could affect the safety of operating MASS (Chang et al. Citation2021).
In the WK050 accident, it is necessary to investigate the thought processes of the pilots to understand why the decision was made to cut the engine. By analysing the accident from the point of view of the decision-makers it can show why these decisions made sense to the operators in that situation (Dekker Citation2006; Plant and Stanton Citation2012). The decisions made by the operator are dependent on their understanding of the situation based on the information that was available to them at the time and their goals (Dekker Citation2006; Dekker Citation2011). Operators often experience competing goals in complex socio-technical systems, in which safety has to be weighed up against (Dekker Citation2006). When investigating the cause of accidents it is necessary to consider organisational norms, operational pressure and regulations to understand the conflicting goals of the environment the operator is making decisions within (Dekker Citation2006; Woods Citation2010). By analysing decision-making in accidents and incidents using a systemic approach, different contributing factors at each level of the system can be shown (Woods Citation2010). The risks identified using a systemic approach may then be used to investigate ways to mitigate these risks in the similar operation of future uncrewed MASS at RCCs.
3.2. Perceptual cycle model of the WK050 crash
3.2.1. Model development
The PCM was developed by a team of human factors practitioners and subject matter experts (SMEs) in an iterative cycle using the Defence Safety Authority (Citation2019) accident report as a basis for discussion. SMEs were defined as members of the uncrewed system community with experience in UAV operations including academic and industrial stakeholders, for further details of the four SMEs’ roles and their number of years of experience in the domain see . Information from the accident report was coded into one of three categories from the PCM framework: ‘Schema’ (based on their individual cognitive map of the world based on their experience); ‘World’ (the world information that was available to them) and; ‘Action’ (the actions they then took) and structured against the accident timeline. The data was then put into a model for analysis for uncrewed system operation recommendations.
Table 2. Subject Matter Expert roles and number of years’ experience in the domain.
3.2.2. What happened during the landing attempt – ‘work-as-done’
shows the analysis of the accident using the PCM framework. The PCM developed () predominantly follows an iterative cycle from ‘World’ to ‘Schema’ to ‘Action’ and can be followed using the step numbering shown in the model. There was a pre-mission briefing for the GCS crew before the training exercise, typically this would include allocation of roles, local weather conditions and routes. As part of this briefing, it may have also been discussed that in other landing attempts at West Wales Airport there had been deviations from the runway centreline, so this may have become an expected behaviour for that particular runway in certain weather conditions.
Figure 5. Analysis of the Watchkeeper accident using the PCM framework (Neisser 1976). Note: Pilot 1 (P1), Pilot 2 (P2), Unmanned Air Vehicle (UAV), Full Motion Video (FMV), Flight Reference Cards (FRCs), Warnings Cautions Advisories (WCA), Air Vehicle Display Computer (AVDC), Air Traffic Control (ATC) and Above Ground Level (AGL).
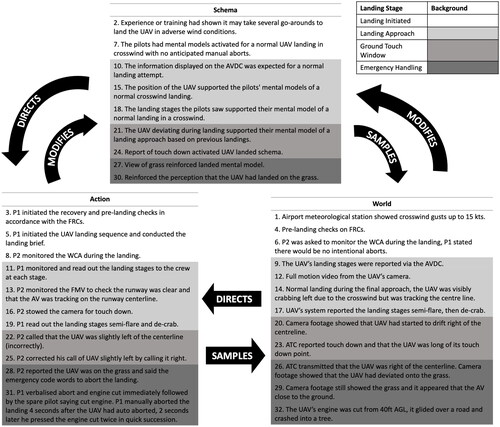
The landing-initiated stage began when pilot 1 saw the crosswind gusts of up to 15 kts and made the decision to land the UAV. Pilot 1 then initiated the recovery and pre-landing checks, which included locating the manual abort button for landing. Pilot 1 also briefed pilot 2 for the landing, informing him that there would be no intentional manual aborts during the landing. At this stage, both pilots would have activated schema based on their past experiences of landing a UAV during crosswinds with no expected manual aborts. This experience may have been from previous flights or simulator training. During the landing approach, the UAV’s system reported the landing stages on the Air Vehicle Display Computer (AVDC) as expected. This was being monitored by pilot 1 who then read out each landing stage. Pilot 2 was monitoring the warning, cautions and advisories as requested by pilot 1 during the pre-landing brief. Pilot 2 was also monitoring the full motion video from the UAV to check the runway was clear. The landing progressed as normal, and it could be seen from the video feed that the UAV was visibly crabbing left but was still tracking the centreline. This led the pilots to believe that the landing was still progressing as would be expected for a UAV landing in crosswinds. Pilot 2 then stowed away the camera for landing, turning it to face rearwards. This is a standard procedure used to protect the camera from any insects or debris during landing. The UAV system continued to report the landing stages (‘semi-flare’ and ‘de-crab’), and these were read out by pilot 1. These were as the GCS crew expected for a crosswind UAV landing.
The ground touch window then began, with the video feed showing that the UAV had started to drift right off the centreline. This was recognised by pilot 2 but was incorrectly reported to other members of the GCS crew (i.e., pilot 2 called out that the UAV had drifted left of the centreline). This may have been a perceptual mistake as the camera was now facing rearwards. The deviation at this point was not unusual during landing at West Wales airport and may have become expected behaviour. Air Traffic Control (ATC) then reported that the AV had touched down, but it was long from the touch down point. This message may have activated the GCS crew’s ‘landed’ schema, as touch down normally meant that the UAV had landed. Pilot 2 then corrected his previous call of UAV left of the centreline to UAV right of the centreline, which was then confirmed in a call from ATC. At this point, the deviation of the UAV from the runway centreline was not unexpected as the UAV had deviated in flights at that airport previously. This deviation may have supported the crew’s mental model of landing.
However, the video feed then showed that the UAV had left the runway and had deviated onto the grass, which was reported by pilot 2. The GCS crew then believed that the UAV had landed on the grass. At this point the emergency handling stage began, the mental model that the UAV had actually landed could have further been reinforced by the message from ATC that the UAV was right of the centreline. As the GCS crew now perceived the situation as UAV had landed on the grass, pilot 2 said the emergency code words to abort the landing. The video feed showed the grass beneath the UAV which appeared to the GCS crew as stationary. The GCS crew had the existing knowledge from the WK’s landing trials that the UAV lost speed much faster than on the runway, so the engine cut needed to be activated quickly. Pilot 1 verbalised the intention to abort and engine cut, immediately followed by the spare pilot saying, ‘engine cut’. Pilot 1 then manually aborted the landing attempt two seconds later than the UAV had auto aborted the landing. Pilot 1 pressed the engine cut button twice in quick succession. This then led to the UAV falling from 40 ft and gliding across a road into a tree. The GCS crew only had a six-second sampling window after ground contact had not been established and before the decision was made to cut the engine. This shows how compelling the video feed was to the GCS crew in deciding a course of action for their perceived situation.
3.2.3. What was supposed to happen during the landing attempt – ‘work-as-imagined’
The WK has an Automatic Take-off and Landing System (ATOLS), which controls take-off and landing. ATOLS reports the stages in the landing cycle to the pilots through an Air Vehicle Display Computer (AVDC) so its progress can be monitored. One of the stages of the landing cycle is called ‘Ground Contact’ referred to as the ground contact window in . If the ATOLS fails to detect ground contact during a specified window, it automatically aborts the landing attempt and begins to conduct a go-around so that another landing can be attempted. When the ATOLS decides to automatically abort the landing attempt, an auto-abort alert is shown to the pilots on the AVDC display. However, the system is designed so that the pilot can also step in and manually abort a landing attempt as a safety measure.
A PCM cycle has been developed to show what should have happened in this instance (see ). The ‘UAV had landed’ schema may have been activated by the message from ATC that the UAV had touched down (step 24 in ). This would have led to the expectation that the next landing mode shown on the AVDC would be ‘ground contact’ and then ‘free roll’. This would have meant that the UAV had established ground contact during the ground contact window, and it was now in free roll as would be expected during the landing phase. However, if the pilots had monitored the AVDC display they would have seen an auto-abort alert due to a land status time out and other indicators that the UAV was going around (e.g. the flight mode, climb rate, altitude, artificial horizon), rather than ‘ground contact’. This would have informed them that the UAV had not established ground contact and that the UAV was going to attempt another landing.
Figure 6. Analysis of what could have happened in the Watchkeeper accident using the PCM framework (Neisser 1976). Note: Pilot 1 (P1), Pilot 2 (P2), Unmanned Air Vehicle (UAV), Flight Reference Cards (FRCs), Warnings Cautions Advisories (WCA), Air Vehicle Display Computer (AVDC), Full Motion Video (FMV) and Air Traffic Control (ATC).
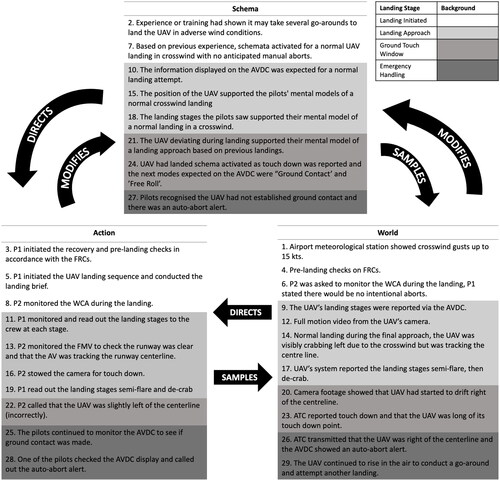
3.2.4. Comparison of the ‘work-as-done’ and ‘work-as-imagined’ PCMs
The PCM analysis indicates that the key difference between what actually happened in the WK050 accident () and what should have happened () is that the pilots’ attention was not on the AVDC and monitoring it for the expected landing stages ‘ground contact’ and ‘free roll’. It appears that the pilots were distracted by the video feed of the UAV on the grass. This distraction led to the misperception that the UAV had landed on the grass if the pilots’ attention was on the AVDC the erroneous decision to cut the UAV’s engine may have been prevented. If the pilots had seen the auto-abort alert on the AVDC, it may have prevented pilot 1 from cutting the engine. However, the auto-abort alert on the AVDC display was not seen due to the distraction of the video feed and the minimal visible indication of the alert (Defence Safety Authority Citation2019). It was found that the AVDC interface was a contributing factor in the accident report (Defence Safety Authority Citation2019).
The purpose of conducting a comparison between ‘work-as-imagined’ versus ‘work-as-done’ is that it enables us to highlight other potential weaknesses within the system. For instance, it may be that the auto-abort alert requires greater levels of saliency and pilots may need training on the use of the video feed in different scenarios. Also, pilots may need further training for unexpected situations to develop their emergency handling skills. It shows the importance of interface design in supporting pilots in maintaining their situation awareness, including their awareness of an automated system’s mode. For MASS this suggests that the design of MASS HMIs and their associated alerts will be important in helping operators maintain their situational awareness. It also suggests that the video feeds in RCCs should be considered carefully as their use could cause operator distraction in certain scenarios. Another issue highlighted was remote operator’s training for emergency scenarios to support their understanding and give them experience using the automated systems, to help them decide an appropriate course of action in the event of an alert or abnormal scenario.
4. Discussion
Automation is often used as a tool to try and overcome errors from occurring however, it simply changes the type and nature of the errors that can occur as there is still a human monitoring the automated system (Dekker Citation2006; Hoem Citation2020). For uncrewed systems, it is still important to consider the human who will be monitoring the automated systems to ensure their safe operation (Woods Citation2010). This is because whilst humans are no longer on board, they still play a key role in the safe operation of the system (Ahvenjärvi Citation2016; Hoem Citation2020). For instance, if there is a system failure or unexpected situation, operators at RCCs will have to decide a course of action and make appropriate decisions for the situation to mitigate any risks involved (Wahlström et al. Citation2015). It will therefore be important that human operators have the necessary information to maintain their situational awareness to make informed decisions to ensure system safety (Endsley and Kiris Citation1995; Onnasch et al. Citation2014; Porathe, Prison, and Man Citation2014). If the operator does not have situational awareness it can lead to erroneous decisions being made and potentially lead to incidents and accidents (Endsley Citation1995).
In the WK050 case, it was a loss of situational awareness of pilot 1 and the rest of the GCS crew, which was identified as a causal factor in the accident report (Defence Safety Authority Citation2019). This led to the formation of a mode error (Norman Citation1983; Sarter and Woods Citation1995), which can occur when an operator loses awareness of the system’s mode, leading them to make potentially inappropriate decisions as they may believe the system to be in a different mode than it is. In this case, the pilots were no longer aware of what mode the UAV was in and had believed it had landed. shows how the pilot’s mental models affected their view of the situation leading to their belief that the UAV had landed. It also shows how the world information, the video feed and the messages from ATC confirmed their belief that the UAV had landed on the grass. The pilots may have been distracted by the UAV’s video feed during the landing. However, the camera was not designed to be used as a decision support system. Instead, it was intended to be used for intelligence, surveillance, and reconnaissance only. It may be that the video feed needs to be switched off during landing or pilots may need to be trained when it is appropriate to use the video feed, as it may not always be clear what is actually happening. For instance, the camera is turned to face rearwards during landing to protect the lens, so pilots would only have a partial view of the situation.
This finding shows the importance of supporting operators to maintain their situational awareness when operating highly automated systems (Endsley and Kiris Citation1995; Onnasch et al. Citation2014; Porathe, Prison, and Man Citation2014). It also suggests that the types of feedback implemented for uncrewed systems need to be considered, as both visual and auditory feedback has been suggested for RCCs but the different types of feedback may distract operators from other important information in high workload and high-stress situations (Ahvenjärvi Citation2016; Mackinnon et al. Citation2015). The use of video feeds will also be relevant for MASS as their systems and systems designs include the use of onboard cameras providing feedback to operators at RCCs, which suggests that the video feed from the MASS could distract operators in high-stress and workload situations (Balbuena et al. Citation2017; Dobref et al. Citation2018; Giordano et al. Citation2015; Stateczny and Burdziakowski Citation2019; Wang et al. Citation2016; Wu et al. Citation2022). Osga and McWilliams (Citation2015) found that the addition of visual and audio feedback in the design of a MASS HMI improved task performance in a range of different tasks, such as collision avoidance, alarm response, system controls and waypoint reports tasks. Although initial investigations into the design of the MASS HMI highlighted the high risk of mode errors occurring due to the operators becoming distracted, suggesting a similar situation to this case study could occur in the operation of MASS (Osga and McWilliams Citation2015). It was also found that in the design of the MASS HMI that the rear camera view from the MASS could be distracting to operators whilst carrying out visual tasks (Osga and McWilliams Citation2015). This further suggests that the use of visual feedback will be an important factor in helping MASS operators maintain their situational awareness, however, there is a need to consider how this visual feedback is implemented to not cause distraction and it has also been suggested that as MASS will be operated at slower speeds than UAVs the video may not always be necessary (Wahlström et al. Citation2015).
The pilots missed the auto-abort given by the UAV’s ATOLS on the AVDC, this highlights the need to consider how interfaces and alert systems are designed for operation from an RCC. It has been found that deficiencies in the design of UAV HMIs have contributed to many UAV accidents (Friedrich and Lieb Citation2019; Hobbs and Lyall Citation2016; Wild et al. Citation2017). It may have been that the alert needs to be larger or its position on the interface needs to be more prominent (Phansalkar et al. Citation2010). An audio alert could also be added to the auto-abort alert to get the pilots’ attention, as their workload may be high during landing, and they could be distracted by the video feed and not looking directly at the AVDC (Morris and Montano Citation1996; Nakashima and Crébolder Citation2010; Olmos, Wickens, and Chudy Citation2000).
Due to the similarities in their operation, the HMI will also be an important factor in the operation of MASS as it connects the human operator to the uncrewed vehicle (Hobbs and Lyall Citation2016; Liu, Aydin, et al. Citation2022; Terwilliger et al. Citation2014). The design of interfaces at RCCs will affect the operator’s ability to maintain their situational awareness and therefore their ability to make appropriate decisions, for MASS novel ways of supporting the operator to maintain their situational awareness will be required (Chen, Haas, and Barnes Citation2007; Endsley Citation1995; Endsley Citation2003; Miller and Parasuraman Citation2007). It has been shown that for MASS collision avoidance the HMI will need to be designed appropriately to allow operators to interpret the information correctly, alert them to possible collisions and allow them to take the necessary actions (Ramos, Utne, and Mosleh Citation2019). The design of these alert systems for MASS will need to be carefully considered to ensure they are effective in gaining the operator’s attention and then that the HMI supports them to take the action to avoid collisions, as this case has shown it may be necessary to have audio alerts associated with visual alerts and ensure that any visual alerts are prominent on the HMI. Virtual Reality (VR) and 3D HMIs have also been suggested to improve an operator’s situational awareness at RCCs (Andersson et al. Citation2021; Karvonen and Martio Citation2019; Lager, Topp, and Malec Citation2019). It has been found that VR and 3D human-machine interfaces improved participants’ situational awareness and improved their ability to detect collisions (Lager, Topp, and Malec Citation2019). Also, the use of a virtual environment simulating the audio from an engine room has been suggested to allow engineers at RCCs to better diagnose engine failures (Michailidis et al. Citation2020).
Typically, PCM models follow an iterative cycle from ‘World’ to ‘Schema’ to ‘Action’ and then back to ‘World’ and so on. However, Revell et al. (Citation2020) recognised that it is possible decision-making can be bi-directional between model components (e.g. World-Action relationships). The analysis of the WK050 suggests such interactions were also present. World-Action relationships can be seen in step four to five and step twelve to thirteen in , where the world information directed the pilots’ behaviour. For example, pilot 1 initiated the pre-landing checks in accordance with the flight reference cards and pilot 2 monitored both the video feed to check the runway was clear and checked to see whether the UAV was following the centreline of the runway. These World-Action examples show skill-based behaviour whereby the decision maker recognises and understands information available within the world. This would immediately trigger a response by the decision maker (Plant and Stanton Citation2015; Revell et al. Citation2020). In the case of UAV flight, pilots follow set landing procedures, perform landing checks and monitor the video feed enabling the schema node to be skipped as pilots are already aware of the action, they need to take (Plant and Stanton Citation2015; Revell et al. Citation2020).
Pilot training and experience have also been highlighted as contributory factors to the WK050 incident (Defence Safety Authority Citation2019). Training has also been found to be a factor in many maritime accidents as well (Chauvin et al. Citation2013) and it has been highlighted as an important factor in the safe operation of MASS (Jo, D’agostini, and Kang Citation2020; Sharma and Kim Citation2022; Yoshida et al. Citation2020). In the case of WK050, the pilots had not experienced a UAV deviating off the runway and the flight simulator was not capable of generating a scenario depicting a land status time out combined with a UAV departing from the runway and a video feed (Defence Safety Authority Citation2019). This meant that the GCS crew faced a unique situation in which they were not equipped with the knowledge of the correct emergency procedure to follow or an accurate mental model of the situation. This suggests that training simulators need to be more representative of a larger number of emergency/system failure scenarios, to support pilots in developing accurate mental models. There are approximately 71 simulated emergencies on the WK simulators for the emergency procedure training (Defence Safety Authority Citation2019). However, there are 760 failures and alerts on the WK system (Defence Safety Authority Citation2019). Although it would not be feasible to expect all these scenarios to be covered, it might suggest that the pilots require more training scenarios to reduce the likelihood of another unique situation occurring.
This also has potential implications for MASS operators as they are expected to act as a backup to the automation and it has been suggested that simulator training may be used, so it will be necessary to consider the fidelity of those simulators and the range of scenarios that can be simulated (Hwang and Youn Citation2022; Yoshida et al. Citation2020). It was also found that in the WK050 accident, the GCS crew lacked knowledge about the ATOLS which would be an important part of the GCS crew’s expectations relating to each stage of the landing and the system’s auto-abort (Defence Safety Authority Citation2019). Simulator training has been found to give users a greater awareness of how a system works and could give remote operators greater experience working with the MASS’ automated systems (Koustanaï et al. Citation2012). Simulator training for abnormal situations has also been highlighted as a way to mitigate risks and allow operators to develop appropriate mental models in other domains such as rail (Tichon Citation2007), medicine (Baker et al. Citation2006), manual road vehicles (Roenker et al. Citation2003) and automated road vehicles (Ebnali et al. Citation2019; Krampell, Solís-Marcos, and Hjälmdahl Citation2020). For MASS it will be necessary that operators have the required knowledge of the ship’s automated system to allow them to make appropriate decisions, suggesting that appropriate simulator training may be a way to achieve this (Jo, D’agostini, and Kang Citation2020; Sharma and Kim Citation2022).
There were other factors involved in the accident, for example, the GCS was manned by five personnel in this case because it was part of a training exercise. However, the pilots were only used to three personnel (the two pilots and an aircrew instructor) being present in the small GCS unit. This caused communication confusion inside the GCS. At one point the spare pilot had a said engine cut but the pilots were unable to distinguish who had said this, as the other three personnel were behind them in the GCS (Defence Safety Authority Citation2019). As the flight was part of captaincy training there was not a clear command structure, as the aircrew instructor had said pilot 1 should take ownership and lead the flight, but it may have been that pilot 1 should have checked for agreement with the aircrew instructor before cutting the UAV’s engine (Defence Safety Authority Citation2019). This highlights the need for clear command structures in the operation of uncrewed systems, so the role and responsibilities of each team member are clear (Voshell, Tittle, and Roth Citation2016). There were also environmental factors, strong crosswinds and the slope of the runway at West Wales Airport which also contributed to the accident (Defence Safety Authority Citation2019).
For the operation of MASS, this accident highlights the need to carefully consider the training requirements for operators and ensure that operators are trained for abnormal scenarios as well as normal operations. The accident showed the importance of the RCC HMI requirements and a need to consider how warning systems are designed to inform operators. It also showed the need to consider how individual subsystem components may be used in MASS operation and to use human factors methods early in their design lifecycle to identify potential errors early on, so the risk of these errors can be mitigated. This example has shown the need for clearly defined roles of the operators working in an RCC, such as a shore-side control centre or host-ship for MASS operation and how the individual roles relate to the rest of the human-machine team. It will be necessary that MASS operators have appropriate pre-mission briefs so that operators are aware of any relevant information related to previous missions, such as the ship’s behaviour at a specific location or environmental conditions.
The analysis of the accident using the PCM showed how decision errors can occur due to the difficulties in operators maintaining their situational awareness when operating uncrewed vehicles from RCCs. Further, it demonstrates the potential for technology to be used in unanticipated ways that may go on to affect how decisions are made, showing that human factors methods need to be applied early in a system’s design lifecycle. In this case, the video feed appears to have had a distracting effect leading to the pilots losing situational awareness, which also could happen when operating MASS as operators will also have video feeds at RCCs and could potentially have a restricted view of the situation. It showed how learnt behaviours can influence expectations of uncrewed system operation, such as the pilots becoming used to the deviation during landing at an airport. This accident highlighted the importance of the operator’s mental models, it has been suggested that simulator training of different MASS system failures could be used to support operators in developing appropriate mental models, by giving them a greater awareness of what to expect in these situations. The accident also showed that the HMI is an important system component as it supports the operator in maintaining their situational awareness and making appropriate decisions. It will be necessary to consider each of these factors could influence the operation of MASS from RCCs, as similar difficulties in decision-making may be encountered due to the similarity’s operations between MASS and UAVs. Further work is needed to investigate decision-making specifically for MASS operation, to understand how it may be supported, what potential errors may occur and how these can be mitigated. Further work is also required to understand how MASS HMIs can be designed to support operator decision-making and to develop novel ways of supporting operators in maintaining their situational awareness at RCC. The use of simulator training for MASS operators should also be investigated further to understand how appropriate mental model development can be supported.
4.1. Evaluation
A limitation of using this approach is that it relied on the completeness and methods used in generating the Defence Safety Authority’s accident report, but this was also supplemented with the discussions with SMEs. There are also the effects of hindsight bias to consider when analysing accidents after they have occurred and the subjectivity of developing the decision-making models from the reports. However, the PCM has been used previously to investigate single case study accidents, such as an automated vehicle accident (Banks, Plant, and Stanton Citation2018) and an aviation accident (Plant and Stanton Citation2012), to understand why errors occurred in highly complex socio-technical systems and gain insights into what can be learnt from these accidents. Another limitation is the differences in the operation of UAVs and MASS from RCCs as MASS are operated at slower speeds and only navigate in two dimensions, which may limit the ability to generalise the findings to MASS (Praetorius et al. Citation2012). However, both uncrewed vehicles are operated from RCCs, so operators experience the same lack of proximity to the vehicles and difficulties in maintaining their situational awareness and both are safety-critical domains (Ahvenjärvi Citation2016; Gregorio et al. Citation2021; Kari and Steinert Citation2021; Man, Lundh, and MacKinnon Citation2018; Man et al. Citation2015; Skjervold Citation2018).
5. Conclusions
As automation technology is becoming more intelligent, uncrewed systems are now being used more widely such as MASS in the maritime domain (Ahvenjärvi Citation2016; Norris Citation2018; Porathe, Prison, and Man Citation2014). The introduction of these uncrewed systems is changing the role of the human operator to supervisors (Mallam, Nazir, and Sharma Citation2020; Nahavandi Citation2017), which has the potential to introduce new avenues of failure in the system (Ahvenjärvi Citation2016). This change brings certain challenges for decision-making as operators are now working with highly automated systems, which are also making decisions of their own, coupled with a reduction in situation awareness from working beyond their line of sight (Mackinnon et al. Citation2015; Man et al. Citation2018; Porathe, Prison, and Man Citation2014). The PCM has been used to investigate the decision-making process of pilots operating a UAV from a ground RCC, to understand how decision failures can occur during uncrewed vehicle operations at RCCs. Using the PCM in this way is beneficial as it shows the operator’s decision making process in the context of the system they are working with so that the breakdown in the human-machine relationship can be seen (Banks, Plant, and Stanton Citation2018; Plant and Stanton Citation2012). The PCM highlights how the schemata of the decision-makers (the pilots) and the environmental information they had from the world led to the decision to cut the engine (Plant and Stanton Citation2012). It is necessary to use such an approach to explore system failures in order to provide a causal explanation of why decisions were made, rather than just what decisions were made, to then be able to understand how accidents might be predicted or prevented in future (Banks, Plant, and Stanton Citation2018; Plant and Stanton Citation2012). By understanding why a decision was made, possible mitigation strategies can be suggested, to better support operators’ decision-making processes when operating uncrewed vehicles such as MASS.
Acknowledgements
This work was conducted as part of an Industrial Cooperative Awards in Science & Technology in collaboration with Thales UK Ltd. Any views expressed are those of the authors and do not necessarily represent those of the funding body.
Disclosure statement
No potential conflict of interest was reported by the author(s).
Additional information
Funding
References
- Ahvenjärvi, Sauli. 2016. “The Human Element and Autonomous Ships.” TransNav 10 (3): 517–521. doi:10.12716/1001.10.03.18.
- Andersson, Olov, Patrick Doherty, Mårten Lager, Jens-Olof Lindh, Linnea Persson, Elin A. Topp, Jesper Tordenlid, and Bo Wahlberg. 2021. “WARA-PS: A Research Arena for Public Safety Demonstrations and Autonomous Collaborative Rescue Robotics Experimentation.” Autonomous Intelligent Systems 1 (1): 9. doi:10.1007/s43684-021-00009-9.
- Armstrong, Jazsmine, Kurtulus Izzetoglu, and Dale Richards. 2020. “Using Functional Near Infrared Spectroscopy to Assess Cognitive Performance of UAV Sensor Operators during Route Scanning.” https://www.scitepress.org/papers/2018/67315/67315.pdf
- Baker, David, Sigrid Gustafson, Jeff Beaubien, Eduardo Salas, Paul Barach, and James Battles. 2006. “Medical Teamwork and Patient Safety: The Evidence-Based Relation.” https://archive.ahrq.gov/research/findings/final-reports/medteam/chapter5.html
- Balbuena, J., D. Quiroz, R. Song, R. Bucknall, and F. Cuellar. 2017. “Design and Implementation of an USV for Large Bodies of Fresh Waters at the Highlands of Peru.” Paper Presented at the OCEANS 2017 – Anchorage: 18–21 Sept 2017.
- Banks, V.A., K.L. Plant, and N.A. Stanton. 2018. “Driver Error or Designer Error: Using the Perceptual Cycle Model to Explore the Circumstances Surrounding the Fatal Tesla Crash on 7th May 2016.” Safety Science 108: 278–285. doi:10.1016/j.ssci.2017.12.023.
- Burmeister, Hans-Christoph, Wilko Bruhn, Ørnulf Jan Rødseth, and Thomas Porathe. 2014a. “Can Unmanned Ships Improve Navigational Safety?” Proceedings of the Transport Research Arena, 14–17.
- Burmeister, Hans-Christoph, Wilko Bruhn, Ørnulf Jan Rødseth, and Thomas Porathe. 2014b. “Autonomous Unmanned Merchant Vessel and Its Contribution towards the e-Navigation Implementation: The MUNIN Perspective.” International Journal of e-Navigation and Maritime Economy 1: 1–13. doi:10.1016/j.enavi.2014.12.002.
- Chang, Chia-Hsun, Christos Kontovas, Qing Yu, and Zaili Yang. 2021. “Risk Assessment of the Operations of Maritime Autonomous Surface Ships.” Reliability Engineering & System Safety 207: 107324. doi:10.1016/j.ress.2020.107324.
- Chauvin, Christine, Salim Lardjane, Gaël Morel, Jean-Pierre Clostermann, and Benoît Langard. 2013. “Human and Organisational Factors in Maritime Accidents: Analysis of Collisions at Sea Using the HFACS.” Accident; Analysis and Prevention 59: 26–37. doi:10.1016/j.aap.2013.05.006.
- Chen, J.Y.C., E.C. Haas, and M.J. Barnes. 2007. “Human Performance Issues and User Interface Design for Teleoperated Robots.” IEEE Transactions on Systems, Man and Cybernetics, Part C (Applications and Reviews) 37 (6): 1231–1245. doi:10.1109/TSMCC.2007.905819.
- Defence Safety Authority. 2019. “Watchkeeper WK050, West Wales Airport - NSI.” https://assets.publishing.service.gov.uk/government/uploads/system/uploads/attachment_data/file/792953/20190402-WK043_SI_Final_Report-_Redacted__RT-OS.pdf
- Dekker, S. 2006. The Field Guide to Understanding Human Error. Farnham: Ashgate.
- Dekker, Sidney. 2001. “The Re-invention of Human Error I: Human Factors and Aerospace Safety.” Technical Report 2002-01. Lund University School of Aviation.
- Dekker, Sidney W.A. 2011. “What is Rational about Killing a Patient with an Overdose? Enlightenment, Continental Philosophy and the Role of the Human Subject in System Failure.” Ergonomics 54 (8): 679–683. doi:10.1080/00140139.2011.592607.
- Deng, H., R.Q. Wang, K.Y. Miao, Y. Zhao, J.M. Sun, and J.B. Du. 2018. “Design of Unmanned Ship Motion Control System Based on DSP and GPRS.” Paper Presented at the International Conference of Information Technology and Electrical Engineering (ICITEE), Guangzhou, Peoples R China, December 07–09.
- Dobref, Vasile, I. Popa, P. Popov, and I.C. Scurtu. 2018. “Unmanned Surface Vessel for Marine Data Acquisition.” IOP Conference Series: Earth and Environmental Science 172: 012034. doi:10.1088/1755-1315/172/1/012034.
- Ebnali, Mahdi, Kevin Hulme, Aliakbar Ebnali-Heidari, and Adel Mazloumi. 2019. “How Does Training Effect Users’ Attitudes and Skills Needed for Highly Automated Driving?” Transportation Research Part F: Traffic Psychology and Behaviour 66: 184–195. doi:10.1016/j.trf.2019.09.001.
- Endsley, Mica, and Esin Kiris. 1995. “The out-of-the-Loop Performance Problem and Level of Control in Automation.” Human Factors: The Journal of the Human Factors and Ergonomics Society 37 (2): 381–394. doi:10.1518/001872095779064555.
- Endsley, Mica R. 1995. “Toward a Theory of Situation Awareness in Dynamic Systems.” Human Factors: The Journal of the Human Factors and Ergonomics Society 37 (1): 32–64. doi:10.1518/001872095779049543.
- Endsley, Mica R., Betty Bolte, and Debra G. Jones. 2003. Designing for Situation Awareness: An Approach to User-Centered Design. Boca Raton, FL: CRC Press.
- Fan, Cunlong, Krzysztof Wróbel, Jakub Montewka, Mateusz Gil, Chengpeng Wan, and Di Zhang. 2020. “A Framework to Identify Factors Influencing Navigational Risk for Maritime Autonomous Surface Ships.” Ocean Engineering 202: 107188. doi:10.1016/j.oceaneng.2020.107188.
- Friedrich, Max, and Joonas Lieb. 2019. “A Novel Human Machine Interface to Support Supervision and Guidance of Multiple Highly Automated Unmanned Aircraft.” Paper Presented at the 2019 IEEE/AIAA 38th Digital Avionics Systems Conference (DASC). doi:10.1109/DASC43569.2019.9081645.
- Giese, S., D. Carr, and J. Chahl. 2013. “Implications for Unmanned Systems Research of Military UAV Mishap Statistics.” Paper presented at the 2013 IEEE Intelligent Vehicles Symposium (IV), 23–26 June 2013.
- Giordano, Francesco, Gaia Mattei, Claudio Parente, Francesco Peluso, and Raffaele Santamaria. 2015. “Integrating Sensors into a Marine Drone for Bathymetric 3D Surveys in Shallow Waters.” Sensors 16 (1): 41. doi:10.3390/s16010041.
- Gregorio, Marianna Di., Marco Romano, Monica Sebillo, Giuliana Vitiello, and Angela Vozella. 2021. “Improving Human Ground Control Performance in Unmanned Aerial Systems.” Future Internet 13 (8): 188. doi:10.3390/fi13080188.
- Helton, William S., Samantha Epling, Neil de Joux, Gregory J. Funke, and Benjamin A. Knott. 2015. “Judgments of Team Workload and Stress: A Simulated Unmanned Aerial Vehicle Case.” Proceedings of the Human Factors and Ergonomics Society Annual Meeting 59 (1): 736–740. doi:10.1177/1541931215591173.
- Hobbs, Alan, and Beth Lyall. 2016. “Human Factors Guidelines for Unmanned Aircraft Systems.” Ergonomics in Design: The Quarterly of Human Factors Applications 24 (3): 23–28. doi:10.1177/1064804616640632.
- Hocraffer, Amy, and Chang S. Nam. 2017. “A Meta-Analysis of Human-System Interfaces in Unmanned Aerial Vehicle (UAV) Swarm Management.” Applied Ergonomics 58: 66–80. doi:10.1016/j.apergo.2016.05.011.
- Hoem, Å S. 2020. “The Present and Future of Risk Assessment of MASS: A Literature Review. Paper Presented at the.” Proceedings of the 29th European Safety and Reliability Conference, ESREL 2019.
- Huang, Yamin, Linying Chen, R.R. Negenborn, and P.H.A.J.M. Gelder. 2020. “A Ship Collision Avoidance System for Human-Machine Cooperation during Collision Avoidance.” Ocean Engineering 217: 107913. doi:10.1016/j.oceaneng.2020.107913.
- Hwang, Taemin, and Ik-Hyun Youn. 2022. “Navigation Scenario Permutation Model for Training of Maritime Autonomous Surface Ship Remote Operators.” Applied Sciences 12 (3): 1651. doi:10.3390/app12031651.
- International Maritime Organisation. 2021. “Outcome of the Regulatory Scoping Exercise for the use of Maritime Autonomous Surface Ships (MASS).” Accessed 26 October 2021. https://wwwcdn.imo.org/localresources/en/MediaCentre/PressBriefings/Documents/MSC.1-Circ.1638%20-%20Outcome%20Of%20The%20Regulatory%20Scoping%20ExerciseFor%20The%20Use%20Of%20Maritime%20Autonomous%20Surface%20Ships…%20(Secretariat).pdf.
- Jessee, M.S., T. Chiou, A.S. Krepps, and B.R. Prengaman. 2017. “A Gaze Based Operator Instrumentation Approach for the Command of Multiple Autonomous Vehicles.” Paper Presented at the 2017 IEEE Conference on Control Technology and Applications (CCTA), 27–30 August 2017.
- Jo, Sohyun, Enrico D’agostini, and Jun Kang. 2020. “From Seafarers to E-Farers: Maritime Cadets’ Perceptions towards Seafaring Jobs in the Industry 4.0.” Sustainability 12 (19): 8077. doi:10.3390/su12198077.
- Kaptan, Mehmet, Songül Sarıali̇oğlu, Özkan Uğurlu, and Jin Wang. 2021. “The Evolution of the HFACS Method Used in Analysis of Marine Accidents: A Review.” International Journal of Industrial Ergonomics 86: 103225. doi:10.1016/j.ergon.2021.103225.
- Kari, Raheleh, and Martin Steinert. 2021. “Human Factor Issues in Remote Ship Operations: Lesson Learned by Studying Different Domains.” Journal of Marine Science and Engineering 9 (4): 385. doi:10.3390/jmse9040385.
- Karvonen, Hannu, and Jussi Martio. 2019. “Human Factors Issues in Maritime Autonomous Surface Ship Systems Development. Paper Presented at the.” Proceedings of the 1st International Conference on Maritime Autonomous Surface Ships.
- Koustanaï, Arnaud, Viola Cavallo, Patricia Delhomme, and Arnaud Mas. 2012. “Simulator Training with a Forward Collision Warning System: Effects on Driver-System Interactions and Driver Trust.” Human Factors 54 (5): 709–721. doi:10.1177/0018720812441796.
- Krampell, Martin, Ignacio Solís-Marcos, and Magnus Hjälmdahl. 2020. “Driving Automation State-of-Mind: Using Training to Instigate Rapid Mental Model Development.” Applied Ergonomics 83: 102986. doi:10.1016/j.apergo.2019.102986.
- Kretschmann, L., H.C. Burmeister, and C. Jahn. 2017. “Analyzing the Economic Benefit of Unmanned Autonomous Ships: An Exploratory Cost-Comparison between an Autonomous and a Conventional Bulk Carrier.” Research in Transportation Business & Management 25: 76–86. doi:10.1016/j.rtbm.2017.06.002.
- Lager, M., E.A. Topp, and J. Malec. 2019. “Remote Supervision of an Unmanned Surface Vessel – A Comparison of Interfaces.” Paper Presented at the 2019 14th ACM/IEEE International Conference on Human-Robot Interaction (HRI), 11–14 March 2019.
- Lim, Yixiang, Nichakorn Pongsakornsathien, Alessandro Gardi, Roberto Sabatini, Trevor Kistan, Neta Ezer, and Daniel J. Bursch. 2021. “Adaptive Human-Robot Interactions for Multiple Unmanned Aerial Vehicles.” Robotics 10 (1): 12. doi:10.3390/robotics10010012.
- Liu, Chenguang, Xiumin Chu, Wenxiang Wu, Songlong Li, Zhibo He, Mao Zheng, Haiming Zhou, and Zhixiong Li. 2022. “Human–Machine Cooperation Research for Navigation of Maritime Autonomous Surface Ships: A Review and Consideration.” Ocean Engineering 246: 110555. doi:10.1016/j.oceaneng.2022.110555.
- Liu, Jialun, Muhammet Aydin, Emre Akyuz, Ozcan Arslan, Esma Uflaz, Rafet Emek Kurt, and Osman Turan. 2022. “Prediction of Human–Machine Interface (HMI) Operational Errors for Maritime Autonomous Surface Ships (MASS).” Journal of Marine Science and Technology 27 (1): 293–306. doi:10.1007/s00773-021-00834-w.
- Luo, Meifeng, and Sung-Ho Shin. 2019. “Half-Century Research Developments in Maritime Accidents: Future Directions.” Accident; Analysis and Prevention 123: 448–460. doi:10.1016/j.aap.2016.04.010.
- Mackinnon, S.N., Y. Man, M. Lundh, and T. Porathe. 2015. “Command and Control of Unmanned Vessels: Keeping Shore Based Operators in-the-Loop.” Paper Presented at the 18th International Conference on Ships and Shipping Research, NAV 2015.
- Mallam, Steven C., Salman Nazir, and Amit Sharma. 2020. “The Human Element in Future Maritime Operations – Perceived Impact of Autonomous Shipping.” Ergonomics 63 (3): 334–345. doi:10.1080/00140139.2019.1659995.
- Man, Yemao, Monica Lundh, and Scott N. MacKinnon. 2018. “Facing the New Technology Landscape in the Maritime Domain: knowledge Mobilisation, Networks and Management in Human-Machine Collaboration.” Paper Presented at the International Conference on Applied Human Factors and Ergonomics.
- Man, Yemao, Monica Lundh, Thomas Porathe, and Scott MacKinnon. 2015. “From Desk to Field - Human Factor Issues in Remote Monitoring and Controlling of Autonomous Unmanned Vessels.” Procedia Manufacturing 3: 2674–2681. doi:10.1016/j.promfg.2015.07.635.
- Man, Yemao, Reto Weber, Johan Cimbritz, Monica Lundh, and Scott N. MacKinnon. 2018. “Human Factor Issues during Remote Ship Monitoring Tasks: An Ecological Lesson for System Design in a Distributed Context.” International Journal of Industrial Ergonomics 68: 231–244. doi:10.1016/j.ergon.2018.08.005.
- Maritime UK. 2021. “Maritime Autonomous Surface Ships UK Code of Practice.” Maritime UK. https://www.maritimeuk.org/media-centre/publications/maritime-autonomous-surface-ships-uk-code-practice/
- Mendonça, R., M.M. Marques, F. Marques, A. Lourenço, E. Pinto, P. Santana, F. Coito, V. Lobo, and J. Barata. 2016. “A Cooperative Multi-Robot Team for the Surveillance of Shipwreck Survivors at Sea.” Paper Presented at the OCEANS 2016 MTS/IEEE Monterey, 19–23 September 2016.
- Michailidis, T., G. Meadow, C. Barlow, and E. Rajabally. 2020. “Implementing Remote Audio as a Diagnostics Tool for Maritime Autonomous Surface Ships.” Paper Presented at the 2020 27th Conference of Open Innovations Association (FRUCT), 7–9 September 2020.
- Miller, Christopher, and Raja Parasuraman. 2007. “Designing for Flexible Interaction between Humans and Automation: Delegation Interfaces for Supervisory Control.” Human Factors 49 (1): 57–75. doi:10.1518/001872007779598037.
- Morris, R.W., and S.R. Montano. 1996. “Response Times to Visual and Auditory Alarms during Anaesthesia.” Anaesthesia and Intensive Care 24 (6): 682–684. doi:10.1177/0310057x9602400609.
- Nahavandi, S. 2017. “Trusted Autonomy between Humans and Robots: Toward Human-on-the-Loop in Robotics and Autonomous Systems.” IEEE Systems, Man, and Cybernetics Magazine 3 (1): 10–17. doi:10.1109/MSMC.2016.2623867.
- Nakashima, Ann, and Jacquelyn M. Crébolder. 2010. “Evaluation of Audio and Visual Alerts during a Divided Attention Task in Noise.” Canadian Acoustics 38 (4): 3–8.
- Neisser, U. 1976. Cognition and Reality. San Francisco: W.H. Freeman and Company.
- Norman, Donald A. 1983. “Design Rules Based on Analyses of Human Error.” Communications of the ACM 26 (4): 254–258. doi:10.1145/2163.358092.
- Norris, Jacob N. 2018. “Human Factors in Military Maritime and Expeditionary Settings: Opportunity for Autonomous Systems?” Paper Presented at the Advances in Human Factors in Robots and Unmanned Systems, Cham.
- Olmos, Oscar, Christopher D. Wickens, and Andrew Chudy. 2000. “Tactical Displays for Combat Awareness: An Examination of Dimensionality and Frame of Reference Concepts and the Application of Cognitive Engineering.” The International Journal of Aviation Psychology 10 (3): 247–271. doi:10.1207/S15327108IJAP1003_03.
- Oncu, Mehmet, and Suleyman Yildiz. 2014. “An Analysis of Human Causal Factors in Unmanned Aerial Vehicle (UAV) Accidents.” https://calhoun.nps.edu/handle/10945/44637.
- Onnasch, L., C.D. Wickens, H. Li, and D. Manzey. 2014. “Human Performance Consequences of Stages and Levels of Automation: An Integrated Meta-Analysis.” Human Factors 56 (3): 476–488. doi:10.1177/0018720813501549.
- Osga, G.A., and M.R. McWilliams. 2015. “Human-Computer Interface Studies for Semi-Autonomous Unmanned Surface Vessels.” Paper Presented at the 6th International Conference on Applied Human Factors and Ergonomics (AHFE), Las Vegas, NV, July 26–30.
- Parasuraman, R. 2000. “Designing Automation for Human Use: Empirical Studies and Quantitative Models.” Ergonomics 43 (7): 931–951. doi:10.1080/001401300409125.
- Parasuraman, Raja, and Victor Riley. 1997. “Humans and Automation: Use, Misuse, Disuse, Abuse.” Human Factors: The Journal of the Human Factors and Ergonomics Society 39 (2): 230–253. doi:10.1518/001872097778543886.
- Peschel, Joshua M., and Robin R. Murphy. 2015. “Human Interfaces in Micro and Small Unmanned Aerial Systems.” In Handbook of Unmanned Aerial Vehicles, edited by Kimon P. Valavanis and George J. Vachtsevanos, 2389–2403. Dordrecht: Springer Netherlands.
- Phansalkar, Shobha, Judy Edworthy, Elizabeth Hellier, Diane L. Seger, Angela Schedlbauer, Anthony J. Avery, and David W. Bates. 2010. “A Review of Human Factors Principles for the Design and Implementation of Medication Safety Alerts in Clinical Information Systems.” Journal of the American Medical Informatics Association 17 (5): 493–501. doi:10.1136/jamia.2010.005264.
- Pietrzykowski, J., Z. Pietrzykowski, and J. Hajduk. 2019. “Operations of Maritime Autonomous Surface Ships.” TransNav 13 (4): 725–733. doi:10.12716/1001.13.04.04.
- Plant, K.L., and N.A. Stanton. 2012. “Why Did the Pilots Shut down the Wrong Engine? Explaining Errors in Context Using Schema Theory and the Perceptual Cycle Model.” Safety Science 50 (2): 300–315. doi:10.1016/j.ssci.2011.09.005.
- Plant, K.L., and N.A. Stanton. 2015. “The Process of Processing: Exploring the Validity of Neisser’s Perceptual Cycle Model with Accounts from Critical Decision-Making in the Cockpit.” Ergonomics 58 (6): 909–923. doi:10.1080/00140139.2014.991765.
- Porathe, Thomas, Johannes Prison, and Yemao Man. 2014. “Situation Awareness in Remote Control Centres for Unmanned Ships.” https://core.ac.uk/download/pdf/70605914.pdf doi:10.3940/rina.hf.2014.12.
- Praetorius, Gesa, Fulko van Westrenen, Deborah L. Mitchell, and Erik Hollnagel. 2012. “Learning Lessons in Resilient Traffic Management: A Cross-Domain Study of Vessel Traffic Service and Air Traffic Control.” Paper Presented at the HFES Europe Chapter Conference Toulouse 2012.
- Ramos, M.A., Christoph A. Thieme, Ingrid B. Utne, and A. Mosleh. 2020. “Human-System Concurrent Task Analysis for Maritime Autonomous Surface Ship Operation and Safety.” Reliability Engineering & System Safety 195: 106697. doi:10.1016/j.ress.2019.106697.
- Ramos, Marilia, Ingrid Utne, and Ali Mosleh. 2019. “Collision Avoidance on Maritime Autonomous Surface Ships: Operators’ Tasks and Human Failure Events.” Safety Science 116: 33–44. doi:10.1016/j.ssci.2019.02.038.
- Revell, K., M. A. J. Richardson, P. Langdon, M. Bradley, I. Politis, S. Thompson, L. Skrypchuck, J. O'Donoghue, A. Mouzakitis, and N.A. Stanton. 2020. “Breaking the Cycle of Frustration: Applying Neisser’s Perceptual Cycle Model to Drivers of Semi-Autonomous Vehicles.” Applied Ergonomics 85: 103037. doi:10.1016/j.apergo.2019.103037.
- Reyhanoglu, Mahmut. 1997. “Exponential Stabilization of an Underactuated Autonomous Surface Vessel.” Automatica 33 (12): 2249–2254. doi:10.1016/S0005-1098(97)00141-6.
- Roenker, D.L., G.M. Cissell, K.K. Ball, V.G. Wadley, and J.D. Edwards. 2003. “Speed-of-Processing and Driving Simulator Training Result in Improved Driving Performance.” Human Factors 45 (2): 218–233. doi:10.1518/hfes.45.2.218.27241.
- Ruiz, J.J., M.A. Escalera, A. Viguria, and A. Ollero. 2015. “A Simulation Framework to Validate the Use of Head-Mounted Displays and Tablets for Information Exchange with the UAV Safety Pilot.” Paper Presented at the 2015 Workshop on Research, Education and Development of Unmanned Aerial Systems (RED-UAS), 23–25 November 2015.
- Saha, Rana. 2021. “Mapping Competence Requirements for Future Shore Control Center Operators.” Maritime Policy & Management : 1–13. doi:10.1080/03088839.2021.1930224.
- Salmon, P.M., G.J. Read, N.A. Stanton, and M.G. Lenné. 2013. “The Crash at Kerang: Investigating Systemic and Psychological Factors Leading to Unintentional Non-Compliance at Rail Level Crossings.” Accident; Analysis and Prevention 50: 1278–1288. doi:10.1016/j.aap.2012.09.029.
- Sandhåland, Hilde, Helle Oltedal, and Jarle Eid. 2015. “Situation Awareness in Bridge Operations – A Study of Collisions between Attendant Vessels and Offshore Facilities in the North Sea.” Safety Science 79: 277–285. doi:10.1016/j.ssci.2015.06.021.
- Sarter, Nadine, and David Woods. 1995. “How in the World Did We Ever Get into That Mode? Mode Error and Awareness in Supervisory Control.” Human Factors 37 (1): 5–19. doi:10.1518/001872095779049516.
- Scharre, P. The opportunity and challenge of autonomous systems. In Autonomous Systems: Issues for Defence Policymakers, edited by A. P. Williams and P. D. Scharre, 3–26). Norfolk, VA: NATO Allied Command Transformation, 2015.
- Sharma, Amit, and Tae-eun Kim. 2022. “Exploring Technical and Non-Technical Competencies of Navigators for Autonomous Shipping.” Maritime Policy & Management 49 (6): 831–849. doi:10.1080/03088839.2021.1914874.
- Silva, L.C. Batista da, R.M. Bernardo, H.A. de Oliveira, and P.F.F. Rosa. 2017. “Unmanned Aircraft System Coordination for Persistent Surveillance with Different Priorities.” Paper Presented at the 2017 IEEE 26th International Symposium on Industrial Electronics (ISIE), 19–21 June 2017.
- Skjervold, Espen. 2018. “Autonomous, Cooperative UAV Operations Using COTS Consumer Drones and Custom Ground Control Station.” Paper Presented at the MILCOM 2018-2018 IEEE Military Communications Conference (MILCOM).
- Stanton, Neville A., and Guy H. Walker. 2011. “Exploring the Psychological Factors Involved in the Ladbroke Grove Rail Accident.” Accident; Analysis and Prevention 43 (3): 1117–1127. doi:10.1016/j.aap.2010.12.020.
- Stateczny, Andrzej, and Pawel Burdziakowski. 2019. “Universal Autonomous Control and Management System for Multipurpose Unmanned Surface Vessel.” Polish Maritime Research 26 (1): 30–39. doi:10.2478/pomr-2019-0004.
- Terwilliger, Brent A., David C. Ison, Dennis A. Vincenzi, and Dahai Liu. 2014. “Advancement and Application of Unmanned Aerial System Human-Machine-Interface (HMI) Technology.” Paper Presented at the Human Interface and the Management of Information. Information and Knowledge in Applications and Services, Cham.
- Thieme, Christoph Alexander, and Ingrid Bouwer Utne. 2017. “A Risk Model for Autonomous Marine Systems and Operation Focusing on Human–Autonomy Collaboration.” Proceedings of the Institution of Mechanical Engineers, Part O: Journal of Risk and Reliability 231 (4): 446–464. doi:10.1177/1748006X17709377.
- Tichon, Jennifer G. 2007. “The Use of Expert Knowledge in the Development of Simulations for Train Driver Training.” Cognition, Technology & Work 9 (4): 177–187. doi:10.1007/s10111-006-0048-6.
- Vagia, Marialena, Aksel A. Transeth, and Sigurd A. Fjerdingen. 2016. “A Literature Review on the Levels of Automation during the Years. What Are the Different Taxonomies That Have Been Proposed?” Applied Ergonomics 53: 190–202. doi:10.1016/j.apergo.2015.09.013.
- Veitch, Erik, and Ole Andreas Alsos. 2022. “A Systematic Review of Human-AI Interaction in Autonomous Ship Systems.” Safety Science 152: 105778. doi:10.1016/j.ssci.2022.105778.
- Voshell, M., J. Tittle, and E. Roth. 2016. “Multi-Level Human-Autonomy Teams for Distributed Mission Management.” Paper Presented at the AAAI Spring Symposium – Technical Report.
- Wahlström, Mikael, Jaakko Hakulinen, Hannu Karvonen, and Iiro Lindborg. 2015. “Human Factors Challenges in Unmanned Ship Operations – Insights from Other Domains.” Procedia Manufacturing 3: 1038–1045. doi:10.1016/j.promfg.2015.07.167.
- Wang, J.H., F.X. Ren, Z.Y. Li, Z. Liu, X. Zheng, Y.S. Yang and Ieee. 2016. “Unmanned Surface Vessel for Monitoring and Recovering of Spilled Oil on Water.” Paper Presented at the OCEANS Conference, Shanghai, Peoples R China, April 10–13.
- Wild, Graham, Kellie Gavin, John Murray, Jose Silva, and Glenn Baxter. 2017. “A Post-Accident Analysis of Civil Remotely-Piloted Aircraft System Accidents and Incidents.” Journal of Aerospace Technology and Management 9 (2): 157–168. doi:10.5028/jatm.v9i2.701.
- Woods, D.D. 2010. Behind Human Error. Farnham: Ashgate.
- Wu, B., G. Li, L. Zhao, H.I.J. Aandahl, H.P. Hildre, and H. Zhang. 2022. “Navigating Patterns Analysis for Onboard Guidance Support in Crossing Collision-Avoidance Operations.” IEEE Intelligent Transportation Systems Magazine 14 (3): 62–77. doi:10.1109/MITS.2021.3108473.
- Yoshida, Masanori, Etsuro Shimizu, Masashi Sugomori, and Ayako Umeda. 2020. “Regulatory Requirements on the Competence of Remote Operator in Maritime Autonomous Surface Ship: Situation Awareness, Ship Sense and Goal-Based Gap Analysis.” Applied Sciences 10 (23): 8751. doi:10.3390/app10238751.
- Zhang, W.J., D. Feltner, D. Kaber, and J. Shirley. 2021. “Utility of Functional Transparency and Usability in UAV Supervisory Control Interface Design.” International Journal of Social Robotics 13 (7): 1761–1776. doi:10.1007/s12369-021-00757-x.
- Zhang, X., B. Guo, Y. Liu, and T. Huang. 2021. “Construction and Application of Applicability Evaluation Model of Human Factors Methods for Complex Human-Machine System.” Advances in Intelligent Systems and Computing 1213: 333–340.