Abstract
Road transport is experiencing disruptive change from new first-of-a-kind technologies. While such technologies offer safety and operational benefits, they also pose new risks. It is critical to proactively identify risks during the design, development and testing of new technologies. The Systems Theoretic Accident Model and Processes (STAMP) method analyses the dynamic structure in place to manage safety risks. This study applied STAMP to develop a control structure model for emerging technologies in the Australian road transport system and identified control gaps. The control structure shows the actors responsible for managing risks associated with first-of-a-kind technologies and the existing control and feedback mechanisms. Gaps identified related to controls (e.g. legislation) and feedback mechanisms (e.g. monitoring for behavioural adaptation). The study provides an example of how STAMP can be used to identify control structure gaps requiring attention to support the safe introduction of new technologies.
PRACTITIONER SUMMARY
This paper considers emerging risks associated with new technologies in the road transport system. It demonstrates a novel approach using STAMP to identify gaps in control and feedback mechanisms within the existing control structure which should be addressed to mitigate risk.
1. Introduction
Road transport is experiencing a period of disruption resulting from the introduction of increasingly sophisticated artificial intelligence-based technologies. These technologies include those aimed at replacing human drivers such as highly automated vehicles (i.e. level 4 described by the Society of Automotive Engineers; SAE) and those designed to better control driver behaviours such as automated driver behaviour monitoring technologies which can identify drivers who are using mobile phones or failing to wear seatbelts.Footnote1 While such technologies are implemented with the intention of improving safety, in the context of 1.35 million road traffic deaths annually (WHO Citation2018), design flaws can create risks if they are not identified and managed appropriately. The recent fatal crashes involving Tesla (e.g. NTSB Citation2020) and Uber/Volvo automated vehicles (NTSB Citation2019) demonstrate the risks that emerge when appropriate controls are not put in place to manage rapid technological development. Such risks have also arisen in other transport domains such as the Boeing 737 MAX crashes (Sgobba Citation2019), where automation worked against pilot inputs. Further, it has been suggested that although automation is likely to prevent road traffic accidents, automation can also result in new types of crashes (e.g. Thompson et al. Citation2020). Sometimes described as ‘unruly’ (Dekker Citation2011), advanced technologies can be intractable (i.e. the ‘black box’ of AI decision making) creating uncertainties around how they may interact with other system components, leading to failure. Further, the ever-increasing speed of technological development means that control actions put in place by those responsible for controlling their effects (i.e. governments, regulators) can be outdated before they are even fully implemented.
When considering risks that may be present in future systems, it is important to note that they may not only involve risks to safety. For example, ethical and legal issues have been identified in respect to autonomous systems (Burton et al. Citation2020) and artificial general intelligence (AGI) systems (McLean et al. Citation2021). Investigations into other types of emerging technologies, such as brain-computer interfaces, have identified risks associated with personal autonomy, legal considerations, privacy and security, psychological impacts, physical health, societal impacts, and malicious applications (King, Read, and Salmon Citation2022). Given the potential application of advanced technologies in transport systems of the future, either for driver interfaces or traffic management, it is germane to consider potential risks that such technologies might pose.
It is clear then that the introduction of new technology can have pervasive impacts throughout a complex system. Systems thinking approaches provide a means to holistically consider such impacts. ‘Systems thinking’ within safety science is a philosophy applied to improve understanding and response to safety-related issues in work systems as well as broader social systems, including road transport (e.g. Salmon, McClure, and Stanton Citation2012; Salmon and Read Citation2019). The philosophy is supported by models and analysis methods underpinned by the notion that adverse events emerge from non-linear interactions between multiple components across a system (Leveson Citation2004; Rasmussen Citation1997). Systems thinking acknowledges that safety is a responsibility that is shared across all hierarchical levels of a system, up to and including regulatory bodies, government, and international organisations. Importantly, systems thinking approaches seek to analyse behaviour at a whole of system level, beyond the so-called ‘sharp-end’ (e.g. road users directly involved in incidents and the immediate road environment) to consider broader organisational, social, economic and political factors and influences that generated circumstances that gave rise to the incident. Systems thinking therefore offers an alternative and indeed opposing approach to traditional individualistic and deterministic approaches focussed on human error alone (see Read et al. Citation2021 for a review).
Systems thinking methods have previously been applied to understand existing safety challenges within the road system such as drink driving (Salmon et al. Citation2020), use of devices within the vehicle (Parnell, Stanton, and Plant Citation2017); the factors contributing to drivers’ engagement in the ‘fatal five’ driving behaviours (Salmon et al. Citation2019), freight vehicle crashes (Newnam and Goode Citation2015), crash reporting and analysis (Stanton, Harvey, et al. Citation2019), and risk in low-income contexts (Hamim et al. Citation2020). Importantly, systems thinking can also help to understand challenges associated with technological disruption in future road transport contexts. For example, Stanton, Harvey, et al. (Citation2019) used a series of systems thinking methods to analyse the Uber-Volvo collision with a pedestrian, Pöllänen et al. (Citation2020) used Rasmussen’s (Citation1997) Risk Management Framework as a basis for considering how blame attribution may change with the introduction of autonomous vehicles, while Banks et al. (Citation2018) used the Event Analysis of Systemic Teamwork (EAST) framework (Stanton et al. Citation2013) to model distributed cognition within road transport networks incorporating intelligent transportation systems. While several systems thinking methods are available that can be useful to apply to analyse the introduction of first-of-a-kind technologies in road transport (see Salmon et al. Citation2022), most methods focus on risk identification for specific technologies or use cases, rather than modelling the whole system which maintains control of risk. The control structure from the Systems Theoretic Accident Model and Processes (STAMP) approach is unique in its approach which involves identification of actors at various system levels and the control and feedback mechanisms in place to maintain safety across the system lifecycle. It enables the taking of a broad perspective, independent of specific technologies, tasks or actor interactions. STAMP was adopted in the current study to assist in investigating whether the current road transport control structure is appropriate for managing the risks associated with new technologies, or if control gaps exist that need to be addressed.
1.1. Systems theoretic accident model and processes (STAMP)
Underpinned by systems theory and control theory, the STAMP model and associated methods describe and analyse the adaptive control structure in place to manage safety within a domain. STAMP views adverse events as emerging from the failure of an adaptive feedback function to maintain safety as a system changes over time. Applying STAMP involves the development of a control structure model. Leveson (Citation2004) provides a generic control structure representation (see ), which can then be adapted for the specific context. The control structure identifies, across hierarchical levels, the actors who share the responsibility for safety, along with the control mechanisms that are used to constrain system behaviour (controls enforced down the hierarchy in ), and the feedback mechanisms that are used to inform actors as to the effectiveness of controls in maintaining safety (communicated up through the hierarchy in ). The control structure model encompasses the system lifecycle, with representations generally covering both system development and system operations, with these two structures linked by the function of maintenance and evolution. The control structure can be used as the basis for risk assessment using the System-Theoretic Process Analysis (STPA) method (Leveson Citation2011) and for accident analysis using the Causal Analysis based on Systems Theory (CAST) method (Leveson Citation2004; Leveson et al. Citation2009).
Figure 1. Generic control structure (adapted from Leveson Citation2004).
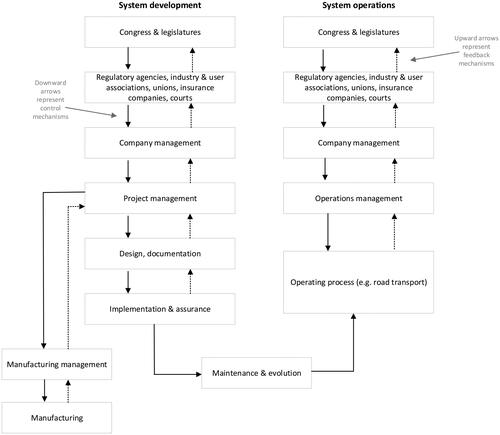
The control structure model alone, without the application of STPA or CAST, also provides a highly useful approach to modelling a complex safety-critical domain. Previous applications of the control structure include in road safety (Salmon, Read, and Stevens Citation2016; Staton et al. Citation2022), rail transport (Read, Naweed, and Salmon Citation2019), construction (Woolley et al. Citation2020) and sport concussion management (Holmes, Clacy, and Salmon Citation2019). To date, full control structure models have not been applied to consider whether current control and feedback mechanisms are appropriate for emerging technologies. While STPA has been applied to identify risks associated with some aspects of future road transport technologies such as automated driving (e.g. Khastgir et al. Citation2021), such control structures have focussed on the lower levels of the system (i.e. the controls and feedback mechanisms associated with the operating process) and structured approaches to identify control gaps have not been employed.
1.2. Study aims and boundaries of analysis
The aim of this study was first to develop a STAMP control structure model representing the current approach to introducing and managing first-of-a-kind technologies in the Australian road transport system and subsequently to identify gaps which could enable emergent risks to be realised.
The context of the analysis was the Australian road transport system; in terms of the current actors, controls and feedback mechanisms in place to manage safety risks, as well as other risks associated with new technologies such as those relating to privacy, security, and justice.
2. Method
Ethics approval for the study was granted by the University of the Sunshine Coast Human Research Ethics Committee (A201494).
2.1. Development of draft control structure
A draft control structure model was developed by the authors over three 2-hour workshops. The authors have extensive experience in applying systems thinking methods to describe and analyse road transport systems (Read et al. Citation2020; Salmon et al. Citation2020; Salmon et al. Citation2019) including consideration of the impact of new technology (e.g. Pöllänen et al. Citation2020; Thompson et al. Citation2020). The control structure model extends upon a previous STAMP model focussed on control of the ‘fatal five’ behaviours in one Australian state (Salmon, Read, and Stevens Citation2016; Salmon and Read Citation2019). This control structure was reviewed and expanded to include new actors, control and feedback mechanisms related to the insertion of new technologies. It was also expanded to be relevant across Australia and updated to encompass recent changes (e.g. new government agencies for road safety).
2.2. Verification of control structure
2.2.1. Participants
Ten participants (7 male; 3 female), independent of the research team, attended a subject matter expert (SME) verification workshop. Participants had a mean age of 47.8 years (SD = 10.4) and held current and previous roles in road safety policy, research, safety, strategy and data, behavioural science, human factors, traffic engineering, automotive engineering and project management. Participants held various roles including three Directors/Chief Officers, one Professor, three Managers for Road Safety, five Principal/Senior Policy Advisors and one Research Fellow. Mean experience in current role was 6.9 years (SD = 5.7; range 1–18 years) and mean experience in other roles related to road transport was 18.5 years (SD = 12.7; range 5–36 years). Participants were employed in government departments and agencies (state and federal), universities or research institutions, technology developers, consulting firms and industry coordinating agencies.
2.2.2. Materials and procedure
SMEs participated in a 2-hour workshop held virtually, using ZoomTM video-conferencing software. Participants provided consent and their demographic information via an online survey prior to their attendance. In addition, prior to attending participants were provided with an electronic copy of the draft control structure model.
At the commencement of the workshop, the research team provided an overview of the draft model. Following this, participants were asked to review the model to ensure its accuracy in capturing the current control structure for first-of-a-kind technology insertion. To support the model review process, two example technologies were selected to represent new technologies being brought into the Australian road transport system. They were intended to promote thought and discussion amongst the SMEs. The example technologies were the introduction of:
Infrastructure-based driver behaviour enforcement cameras (specifically those designed to use artificial intelligence to detect mobile phone usage)
Highly automated vehicles (Level 4, SAE)
For each of the example technologies, SMEs were prompted to review each level of the draft control structure model. First, they reviewed the International context level which sits at the top of the control structure model and influences the two sides of the Australian road transport system control structure: the Design and construction side and the Operations side. After considering the international level, participants next reviewed the Design and construction side of the control structure (from Level 1 down to Level 5), followed by the Operations side of the control structure (from Level 1 down to Level 5). For each level, after describing the relevant actors, controls, and feedback mechanisms, participants were asked to identify any missing actors, controls, or feedback mechanisms that currently exist in the system. Feedback was not constrained to the example technologies and participants also provided general feedback on the model including amendments to wording of specific actors, control or feedback measures.
2.3. Model refinement
Detailed notes and audio recordings taken during the SME workshop were reviewed to refine the model post-workshop. Refinements involved addition or removal of actors, control mechanisms and feedback mechanisms, as well as amendments to wording of these aspects of the model based on the advice of the SME participants. The changes were initially made by a single author (SM) then reviewed and discussed in a meeting with two additional authors (GR and PS) to ensure the feedback was appropriately addressed.
The updated model was distributed to all participants via email for further feedback and refinement. Two participants provided additional written feedback which was incorporated into the final version of the model.
2.4. Identification of gaps
Following SME verification of the model, gaps in the control structure were identified. Gaps were identified in three ways, over a series of meetings involving three of the authors (GR, SM and PS). First, gaps were identified from reviewing the SME workshop outputs (notes and videos) as SME feedback regarding the model often involved discussions about gaps, even though this had not been explicitly prompted by the facilitators. Second, the control structure model was compared to previously published control structure models for other domains. These included control structure models of civil aviation (Allison et al. Citation2017), military missile simulation (Stanton, Harvey, et al. Citation2019), rail transport (Read, Naweed, and Salmon Citation2019), and construction (Woolley et al. Citation2020). These control structures were chosen as they represent domains with more formalised and mature approaches to safety management than the existing road transport system, where the onus for safety has traditionally fallen on the individual road user (with key controls based on education/training, compliance and enforcement). The comparison was conducted by reviewing the four previously published control structures, beginning with the highest level (usually equivalent to Level 1: Parliament and legislatures) and moving down the structure to consider each control and feedback mechanism to determine if it was identified in the road transport control structure. Where controls and feedback mechanisms identified in the previous control structures were not found within the current road transport control structure, the research team discussed whether this represented a gap in terms of safety and risk management. Specifically, the team discussed whether the missing control or feedback mechanism might provide benefits in managing risks associated with the introduction of first-of-a-kind road transport technologies. Where they did, these gaps were documented. Where differences were related to operational differences between domains, such as the feedback measures ‘Terrain warning’ and ‘Interrogation of transponder’ in the control structure for aircraft crew response to rapid decompression events (Allison et al. Citation2017), these were not documented. Finally, while the authors (GR, SM and PS) conducted the control structure model comparisons, additional gaps were documented based on the team’s knowledge and prior research in road safety and safety science more generally.
3. Results
The final control structure model is presented as supplementary material 1. The model shows the actors at each level, as well as the control mechanisms (labels shown adjacent to the solid arrows propagating down through the hierarchy) and feedback mechanisms (labels adjacent to the dashed arrows propagating up through the hierarchy) in place.
The following sections describe the final model across three key extracts: (1) the International context; (2) the Design and construction side of the control structure; and (3) the Operations side of the control structure. To reflect that all aspects of the system are affected implicitly by non-formal influences, such as social norms, the model notes that Australian society and culture sits behind both sides of the control structure.
3.1. International context
shows the international actors that the SMEs agreed have an influence on the introduction of new road system technology, along with the control measures they impose on the Australian road transport system, and the feedback mechanisms that Australian road system stakeholders use to provide information on the operation of new technologies back to interactional actors. Thirteen international actors that influence the management of safety of new technologies, through control mechanisms, were identified (see supplementary material). Note that international manufacturers/developers include vehicle manufacturers recognising that Australia does not have local manufacturers (including manufacturers of automated vehicles).
The controls that this set of international actors impose onto the Australian transport system include coordination and agreements (e.g. the United Nations declaration of human rights relating to privacy and surveillance, relevant to technologies such as mobile phone detection cameras), international road safety strategies developed by international road safety organisations, targets and performance measures (e.g. the United Nations vision zero target), international standards and guidelines (e.g. IEEE standards for intelligent transportation systems), research funding provided by international government forums (e.g. funding for research programs investigating the safe design of automated vehicles), design decisions by international technology or automotive manufacturers/developers whose products are exported to Australia, international legislation set by road safety authorities of other nations which become influential as ‘best practice’ and other international best practice and guidelines.
The feedback mechanisms that are used by Australian road transport system stakeholders to provide information to international actors include government reports on road safety, research proposals, reports and findings in response to research funding, financial reporting and advocacy and lobbying to international government forums.
3.2. Road transport system design & construction
presents the Design and construction side of the control structure. Details of the actors at each level can be found in the Supplementary Materials. At the top of the structure sits the Australian Constitution. This sets out the ‘separation of powers’ of the federal and state/territory governments whereby the state and territory governments have the power to legislate for road safety. However, other topics relevant to emerging technologies, such as communications, are legislated at the federal level. This creates additional complexity in relation to governance around introducing technologies such as vehicle-to-vehicle or vehicle-to-infrastructure communication technologies operating via wireless networks. This point is important for Level 1: Parliament and Legislatures and Level 2: Government agencies, industry associations, user groups, courts, universities of the control structure as although the states and territories are ultimately responsible for road safety, much effort is placed into developing national policies, and nationally consistent approaches across Australia as a federated nation.
Ten actors were identified in Level 1: Parliament and Legislatures. These actors impose controls on Level 2 such as strategy, policy and action plans (e.g. policy directives), political objectives (e.g. ministerial statements supporting regulatory reform to enable trials of automated vehicles), and laws, rules and regulations (e.g. the Motor Vehicle Standards Act 1989 that requires all road vehicles, included imported vehicles, to comply with the Australian Design Rules). Feedback provided to this level includes government reports, financial reporting, advocacy and lobbying, research proposals, reports and findings, and draft legislation for parliamentary debate and consideration (e.g. revised legislation to enable enforcement from offences detected by automated cameras).
Twenty-eight actors were identified at Level 2: Government agencies, industry associations, user groups, courts, universities. At this level, actors impose some controls on the Manufacturing function, such as standards, contractual arrangements, and funding and resource allocation. It should be noted that in the Australian context, manufacturing is limited to technologies other than vehicles, such as detection cameras or other infrastructure-based sensors and communication technologies. Controls imposed on Level 3: Operational delivery and management include strategy, policy, procedures and action plans (e.g. the National Road Safety Strategy 2021–2030), standards and codes of practice (e.g. the Standards Australia Artificial Intelligence Roadmap), and guidelines (e.g. for consideration of cybersecurity in software design). Feedback mechanisms include research findings (e.g. from automated vehicle testing to determine the interfaces with existing infrastructure), research proposals and performance reporting.
Nineteen actors were identified at Level 3: Operational delivery and management. Actors at this level also impose controls on the Manufacturing function, such as standards, contractual arrangements, and funding/resource allocation. Controls imposed on Level 4: Project management team include policy and procedures, training, and targets and performance measures (e.g. KPIs in relation to time to deliver, budget and quality requirements). Feedback mechanisms include research findings, research proposals and performance reporting (e.g. performance against KPIs for detection cameras would require a certain level of accuracy for use in enforcement action).
Eleven actors were identified at Level 4: Project management team. At this level, actors impose supervision on the Manufacturing function, and impose controls on Level 5: Design and assurance processes such as system requirements, contractual arrangements (e.g. where functions are outsourced) and funding/resource allocation. Feedback mechanisms provided to Level 4 include performance reporting, financial reporting and incident reports (e.g. incidents identified during testing and verification processes).
At Level 5: Design and assurance processes the functions of design, construction and testing and verification occur, and the outputs of these are taken into implementation, maintenance and evolution. An important feedback mechanism exists whereby evaluation findings and lessons learned from implementation and maintenance are fed back into project management to support future re-design and evolution of technologies, as well as inform the design and development of new technologies.
3.3. Road transport system operations
presents an extract of the Operations side of the control structure. Details of the actors at each level can be found in the Supplementary Material.
As for the Design and construction side of the control structure, the Australian Constitution is represented at the top to acknowledge the separation of powers between state/territory and federal governments in Australia. For the Operations side of the control structure, a total of 11 actors were identified in Level 1: Parliament and Legislatures. These actors included 10 of the same actors within , in addition to Local Government Associations. These actors impose controls on Level 2 such as strategy, policy and action plans (e.g. policy directives), political objectives, and laws, rules and regulations (e.g. traffic regulations that govern mobile phone use, or interactions between road users). Feedback provided to this level includes government reports, financial reporting, advocacy and lobbying (e.g. industry groups lobbying members of parliament for legislative reform to support the introduction of automated vehicles), research proposals, reports and findings, policy development, and draft legislation for parliamentary debate and consideration. Feedback from lower levels is also gained, in the form of advocacy, public opinion and complaints from members of the public.
Thirty-three actors were identified at Level 2: Government agencies, industry associations, user groups, courts, and universities. At this level, actors impose controls on Level 3: Operational delivery and management including strategy, policy, procedures and action plans (e.g. state-based road safety action plans), standards and codes of practice (e.g. codes of practice for managing information privacy associated with storing images from detection cameras), and guidelines (e.g. for managing cybersecurity). Actors also impose controls on Level 4: Local management and supervision in the form of legal penalties on business owners should they fail in their duty of care to ensure the safety of employees (e.g. by providing an illegally modified automated vehicle) and on Level 5: Operating process and environment such as road safety campaigns/education developed by state and territory road transport authorities to influence driver behaviour, or legal penalties enforced by courts directly onto drivers. Feedback mechanisms provided to Level 2 actors include a range of data and statistics on infringements, safety, risk, injuries, traffic flows and trip types, as well as registration and licencing data.
Twenty-five actors were identified at Level 3: Operational delivery and management. Controls are imposed by this level onto Level 4: Local management and supervision in relation to policy and procedures (e.g. safe driving policies by employers that influence the behaviour of work supervisors), training (e.g. training of vehicle inspectors/mechanics) and targets and performance measures (e.g. targets on road safety audits to cover priority road areas). Actors also impose controls directly on Level 5: Operating process and environment in relation to recalls (e.g. vehicle faults), licencing of drivers, and registration of vehicles. Feedback provided to Level 3 actors is somewhat similar to that provided to Level 2 actors, with some unique mechanisms such as maintenance reports, software error reports and crash reports.
Seventeen actors were identified at Level 4: Local management and supervision. Controls imposed on Level 5: Operating process and environment include driving instruction, information about rules and regulations (e.g. for pedestrians via schools and early learning centres) and dynamic traffic management (e.g. control of signals by traffic management centres). Feedback provided to this level includes crash, incident and maintenance reports, infringement histories and evaluations (e.g. the findings of infrastructure audits to determine suitability for automated vehicle testing).
The final level, Level 5: Operating process and environment, incorporates vehicles (including manually driven vehicles and vehicles with automated functions), human road users and the operating environment. Together these describe how the process of mobility is managed on the road. The operating environment imposes controls on how humans interact with road vehicles including enforcement and other penalties (e.g. automatic enforcement by infrastructure-based cameras), route guidance (e.g. provided by navigation apps) and information about rules and regulations (e.g. information provided by vehicle to infrastructure communications systems). Feedback from road users to the operating environment includes road user behaviours (e.g. use of mobile phones while driving recorded by detections cameras, or data collected via apps). This level also incorporates road users (e.g. drivers, powered two-wheelers, pedestrians and cyclists), along with the control and feedback relationships with any vehicles being operated. Here, both control and feedback mechanisms relate predominantly to road user behaviours in terms of positioning and movement (e.g. a driver travelling in a cycling lane prevents a cyclist from occupying that road space) and verbal and non-verbal communications (e.g. use of handsignals to indicate an intention to turn).
3.4. Control structure analysis—gaps in controls and feedback
The control structure model provides a means to analyse the current control and feedback mechanisms at each level and determine gaps whereby these mechanisms do not currently exist in the system. indicates gaps identified in relation to control measures across the control structure and provides examples of the gaps identified in relation to feedback measures. The gaps relate to missing controls, existing controls that do adequately address the issues associated with new technologies, delayed feedback mechanisms and missing feedback mechanisms.
Table 1. Example control gaps identified in the control structure.
Table 2. Example feedback gaps identified in the control structure.
4. Discussion
The aim of this study was to apply STAMP to develop a control structure model for first-of-a-kind technologies introduced into the road transport system and identify gaps that could generate risks. The model suggests that there are numerous actors, controls and feedback mechanisms present to manage the risks associated with new technologies. However, this does not necessarily suggest that the adaptive feedback function is currently working effectively or will into the future with the continued introduction of new technologies. Dekker (Citation2011), for example, argues that the ‘protective structure’ put in place to manage safety (incorporating such controls as regulatory arrangements, safety committees, compliance and oversight) can be ineffective due to poor knowledge and information, conflicting goals, and can lead the system towards failure through control measures introducing unanticipated negative consequences in another area. The large number of actors residing at level 2 (e.g. government agencies, industry associations, user groups, courts, universities) on both sides of the control structure indicates the existance of a large protective structure within the road transport system. Even within governments, there are various departments who must coordinate approaches to ensure the safe introduction of technologies suggesting a need to focus on collaboration, information sharing and avoidance of silos across levels of the system. Actors at this level are an important group to consider, given their strategic remit and function as ‘gatekeepers’ of information and advice to ministers and parliament. Highlighting the complexity of the coordination task supports previous arguments that the road transport system in Australia is at risk of drifting into failure and requires us to continue to advance systems approaches to understanding and addressing safety issues (Salmon, McClure, and Stanton Citation2012). Further research should explore the extent to which there is meaningful interaction and collaboration between level 2 actors around the management of risks associated with new and emerging road transport technologies.
In addition to describing the existing system with STAMP, this study used the control structure in a novel way to identify gaps in control and feedback mechanisms. The identification of gaps was achieved in three ways: via SME input, comparison with existing control structures, and research team discussion. Each of these approaches gained useful insights, suggesting the benefits of engaging with both industry SMEs and researchers in analysing the existing system and learning from other domains with more formalised and mature approaches to safety management. Indeed, previous research has suggested that the road transport system can learn from other safety-critical industries when implementing new technologies, such as automated vehicles (Papadimitriou et al. Citation2020, Read et al. Citation2022). The STAMP control structure provided a useful and theoretically underpinned framework to support such comparisons.
4.1. Control gaps
Specific gaps relating to missing controls and existing controls that are inadequate in the face of emerging advanced technologies were identified in relation to each level of the control structure. This suggests that new and enhanced controls are needed within the road transport system to better equip it to respond to the introduction of first-of-a-kind technologies. In relation to the Design and construction side of the control structure, one of the gaps identified related to the lack legislation for an independent road safety regulator. Safety regulators independent of government are common in other safety-critical domains yet are not present for road transport. Independence of regulators has been used as a means to avoid political interference in regulatory actions (Hanretty & Koop, Citation2012), which in the safety realm, could lead to market or economic factors being prioritised over safety concerns. In the current Australian road transport system, a range of regulatory structures are in place at various levels of government. For example, road infrastructure has traditionally been owned and managed by state or local government authorities with the majority of oversight conducted by state authorities, focussed on individual road users via vehicle registration, licencing and police enforcement of road rules. For vehicle manufacturers, federal regulations exist in the form of vehicle standards and consumer regulation, however these are not enforced by an independent regulator and manage a range of issues beyond safety alone. No independent regulator exists at either the state or federal levels to provide independent oversight. Further, legislation tends to be prescriptive rather than representing principles (or performance) based regulation (Black Citation2007). Our control structure model did identify two regulatory actors that play a role in regulating organisations in relation to work-related driving: state-based workplace health and safety regulators and the National Heavy Vehicle Regulator, however these sit at the Operations side of the control structure rather than influencing design/construction. And while a new regulatory safety regulator will be established in Australia with the mandate of ensuring the in-service safety of automated vehicles (NTC. Citation2021), this will interface with the existing regulatory system and likely act predominantly around operations. Further, the level of independence from government of this new regulator is yet to be announced.
An additional control gap identified on the Design and construction side of the control structure was the lack of a process for ensuring that information about residual risks is communicated to relevant stakeholders to ensure that risks that could not be addressed during design are managed elsewhere in the system. For example, should an automated vehicle manufacturer identify during testing that computer vision systems are less reliable at accurately identifying objects under certain road environments or weather conditions there is no process requiring this to be communicated to governments, retailers or consumers. A solution could be to require open sharing of the limitations of new technologies, with additional controls being implemented. Additional controls in the instance of limitations of computer vision systems could involve governments installing advisory signage at locations where the technology is known to be less reliable or retailers providing information/briefings to new owners regarding limitations of the technology.
Considering the Operations side of the control structure, an example of a control gap was the lack of legislation establishing an independent transport safety investigator for the road transport domain. The current transport safety investigation agency, the Australian Transport Safety Bureau, only has powers to independently investigate and make recommendations regarding aviation, rail, and maritime operations. An independent road crash investigation body has previously been identified as a critical requirement in other jurisdictions (e.g. Stanton, Harvey, et al. Citation2019). The introduction of new technologies such as advanced automated vehicles arguably enhances this need given that existing crash analysis and reporting systems are already limited and are unlikely to provide useful data on causes relating to the design and behaviour of vehicle automation technologies. Extending this remit to more closely align with the US National Transportation Safety Bureau which has investigated various automated vehicle crashes (e.g. NTSB. Citation2017, NTSB Citation2019, NTSB Citation2020), would ensure that safety lessons can be learned nationally from accident investigations into crashes associated with new technologies. Given the establishment of a future Road Safety Investigation Branch in the United Kingdom (Stanton et al., 2023), it is suggested that addressing this gap in current road transport systems is relevant internationally. In addition, the analysis conducted in the present study suggests that road safety investigation bodies should have a broad remit, and appropriate resources to investigate existing types of crashes, as well as those involving advanced technologies (e.g. automated vehicles or, failures of intelligent transport systems for traffic control).
A second control gap example for the Operations side of the control structure is the lack of legislation to restrict the importation of portable devices intentionally designed to evade detection by enforcement cameras. That is, as such cameras become more common, it is likely that manufacturers of portable devices such as mobile phones will seek to design devices that are less likely to be detected (through using different materials, reducing device size, means of user interaction, etc). Given that most devices are manufactured overseas, the importation and sale of such devices may require new regulations to be established.
A final example of a control gap example on the Operations side of the control structure relates to the fact that existing driver licencing schemes do not consider users/operators of automated vehicles. This was noted as an inadequate control whereby reforms will need to be made to licencing categories and training and education to ensure that users of automated vehicles have appropriate knowledge and skills for the safe operation of these technologies, including capabilities and limitations of the technology and emergency procedures.
4.2. Feedback gaps
An example of a gap in feedback idenitified in the Design and construction side of the control structure was a lack of data on existing workforce expertise in advanced technology/AI to ensure there is adequate professional expertise in the safe design and construction of new technologies. It will be important for those across the system to have expertise across areas such as software engineering and safety verification, as well as cybersecurity, human factors and other specialties. The road system is rapidly changing from requiring the traditional engineering disciplines to needing new types of specialists to manage new types of risks. It is important that planning is undertaken now to ensure professionals with appropriate training and skill sets will be available to perform this work competently.
An additional feedback gap on the Design and construction side of the control structure is the lack of a system in place to monitor intentional workarounds or unintended modifications to technologies following implementation. One example here relates to measures taken by users to avoid being detected by automated mobile phone detection cameras such as by using a smartwatch to make calls or send text messages. Another example relates to automated vehicles, where it is possible that owners will seek to modify systems to improve their vehicle’s performance or remove safety constraints, analogous to current practices such as ‘chip tuning’ (Glas et al. Citation2015). Additionally, automated driving systems might be installed into older vehicles and integrated with other technologies in a way not intended or foreseen by the original manufacturer. Means to identify such practices for monitoring and intervention purposes could include random vehicle inspections/audits, audits of vehicle mechanics or larger-scale, national monitoring of web-based advertisements of modification services or social media discussions regarding modifications.
Turning to the Operations side of the control structure, a gap in feedback relates to the capacity of government agencies to measure the ‘normal performance’ of road users over time as technologies are introduced to enable monitoring of unintentional behavioural adaptation to new technologies. For example, research has shown that drivers using adaptive cruise control, even during a short field study, exhibited increased engagement in a secondary task, reduced response times to hazards and increased variability in lane positioning (Rudin-Brown and Parker Citation2004). In terms of future technologies, it has been hypothesised that cyclists and other vulnerable road users such as pedestrians or e-scooter riders, learning over time that highly automated (and flawlessly performing) vehicles do not pose a physical threat may adapt to behave more assertively (i.e. pulling out in front of AVs at intersections) (Thompson et al. Citation2020). Such behaviour may introduce new safety risks should a cyclist mistake a manually driven vehicle for an automated vehicle and is likely to have significant impacts on efficiency of the transportation network, and the uptake of automated vehicles. To the authors’ knowledge, no road transport system currently has the capacity to systematically monitor these sorts of adaptive behaviours on an on-going basis to inform decision making regarding the need for design changes or new or improved control measures. This could be achieved by widespread data analysis, using AI systems to classify behaviours and determine trends, although this in turn requires considerations around risks to privacy of societal monitoring.
More broadly, there are potential first-of-a-kind technologies that can impact on road safety that are not yet known. It is therefore imperative that actors at higher levels of the system, including governments worldwide, monitor technological trends with a view to understanding emerging impacts that may increase the risk of crashes in their local contexts.
4.3. Strengths, limitations and future research
While the control structure was verified by a relatively small number of SMEs, the group was highly experienced and represented a range of stakeholder groups across the road transport system. In addition, the control structure was based on a previously published model (Salmon, Read, and Stevens Citation2016). Importantly, this analysis has shown that the control structure model can provide a mechanism for identifying gaps in control and feedback mechanisms. While the model was developed and analysed in the Australian context, the impact of emerging technologies is a challenge being faced worldwide and we expect that the gaps in control and feedback mechanisms are likely to be broadly generalisable. Previous research comparing the road transport system in different countries (Bangladesh, China, Kenya, United Kingdom and Vietnam) highlighted similarities in system structures (McIlroy et al. Citation2019). This suggests that the control structure developed in the present study should be relatively straightforward to adapt in future research modelling road transport systems in other countries, taking account of local differences. Comparisons of control structures internationally would also be a useful avenue for future research and could give insights into how the Australian control structure could be strengthened.
The present study was designed to take a broad perspective on the introduction of new technologies with a focus on identifying gaps in the overall control structure which could be addressed prior to widespread implementation. Therefore, the gaps identified are relatively high-level. However, as more advanced technology begins to be implemented, future research should consider the application of additional systems-based risk assessment methods to gain a comprehensive view on the risks associated with specific technologies. This could involve building on the control structure developed in this study to apply STPA (Leveson Citation2011), which allows for the identification of instances where control and feedback mechanisms in the control structure model could fail and prompts the development of risk controls to address such failures. However, there are also limitations of the STAMP control structure approach that should be noted. For example, Lintern and Kugler (Citation2017) note that the hierarchical model underpinning STAMP can promote safety management via micromanagement and retrospective approaches, reliant on feedback to manage emerging issues. In addition, disadvantages of STPA in identifying risks based on the control structure include that it does not explicitly identify emergent risks (i.e. those that arise from the interaction of failures), that there is limited evidence of reliability and validity and that the outputs can become complex and unwieldly (Salmon et al. Citation2022). As such, it would be worthwhile to apply additional complementary systems thinking approaches to the question of the safety of advanced technologies in the road transport system. Candidate approaches include the Event Analysis of Systemic Teamwork Broken Links (EAST-BL; Stanton and Harvey Citation2017), the Networked Hazard Analysis and Risk Management System (Net-HARMS) (Dallat, Salmon, and Goode Citation2018) and Agent-Based Modelling (ABM). Depending on the method, applications would involve identifying risks in terms of failures relating to information transmission (EAST-BL), tasks and task interactions (Net-HARMS), and agent interactions (ABM). Each of these analyses could provide additional and complementary data to the current high-level, hierarchically-based analysis.
Future work could also take the control structure presented here and use it to extend the EAST approach using a control network (Salmon et al. Citation2018), which would enable an understanding of the inter-relationships amongst controls and to analyse controls in relation to system tasks, agents and information used by agents to maintain safety.
Finally, it is suggested that the approach of using control structure models to identify gaps in control and feedback mechanisms could be used to identify recommendations to improve safety control in other domains. A more formal, systematic analysis of the control and feedback mechanisms in place across all published control structures could produce a classification scheme of types of control and feedback mechanisms to support future analysts to identify gaps across domains and industries.
5. Conclusion
This paper has demonstrated the utility of developing a control structure model of the existing road transport system as a first step in considering where gaps may exist in controls and in the adaptive feedback function to maintain safety over time. If not addressed, the gaps identified could allow risks associated with the introduction of future technologies, such as advanced automated vehicles, to be realised. We suggest that the control structure developed in this study can assist current efforts in Australia and other jurisdictions by providing a structure to prompt consideration of the different control and feedback mechanisms required by different actors.
An important next step, as technologies begin to be implemented, involves the use of comprehensive systems-based risk assessment methods for considering future systems in detail. Further, we encourage more applications of systems methods to understand the risks associated with future technologies involving AI (inlcuding advanced forms representing AGI) across safety-critical domains.
Abbreviations | ||
ABM | = | Agent Based Modelling |
AGI | = | Artificial General Intelligence |
AI | = | Artificial Intelligence |
CAST | = | Causal Analysis based on Systems Theory |
EAST | = | Event Analysis of Systemic Teamwork |
EAST-BL | = | Event Analysis of Systemic Teamwork Broken Links |
Net-HARMS | = | Networked Hazard Analysis and Risk Management System |
SME | = | Subject Matter Expert |
STAMP | = | Systems Theoretic Accident Model and Processes |
STPA | = | System-Theoretic Process Analysis |
Supplemental Material
Download PDF (453 KB)Disclosure statement
No potential conflict of interest was reported by the author(s).
Additional information
Funding
Notes
1 For example, in Queensland, Australia, these are approved photographic detection devices under the Traffic Regulation 1962, known specifically as a digital driver behaviour camera system.
References
- Allison, C. K., K. M. Revell, R. Sears, and N. A. Stanton. 2017. “Systems Theoretic Accident Model and Process (STAMP) Safety Modelling Applied to an Aircraft Rapid Decompression Event.” Safety Science 98: 159–166. doi:10.1016/j.ssci.2017.06.011.
- Banks, V. A., N. A. Stanton, G. Burnett, and S. Hermawati. 2018. “Distributed Cognition on the Road: Using EAST to Explore Future Road Transportation Systems.” Applied Ergonomics 68: 258–266. doi:10.1016/j.apergo.2017.11.013.
- Black, J. 2007. Principles Based Regulation: Risks, Challenges and Opportunities. In: Principles Based Regulation, Sydney, Australia. London School of Economics and Political Science.
- Burton, S., I. Habli, T. Lawton, J. McDermid, P. Morgan, and Z. Porter. 2020. “Mind the Gaps: Assuring the Safety of Autonomous Systems from an Engineering, Ethical, and Legal Perspective.” Artificial Intelligence 279: 103201. doi:10.1016/j.artint.2019.103201.
- Dallat, C., P. M. Salmon, and N. Goode. 2018. “Identifying Risks and Emergent Risks Across Sociotechnical Systems: The NETworked Hazard Analysis and Risk Management System (NET-HARMS).” Theoretical Issues in Ergonomics Science 19 (4): 456–482. doi:10.1080/1463922X.2017.1381197.
- Dekker, S. 2011. Drift into failure: From hunting broken components to understanding complex systems. Surrey: Ashgate.
- Glas, B., et al. 2015. “Automotive Safety and Security Integration Challenges.” Lecture Notes in Informatics P-240: 13–28.
- Hamim, Omar Faruqe, Shamsul Hoque, Rich C. McIlroy, Katherine L. Plant, and Neville A. Stanton. 2020. “A Sociotechnical Approach to Accident Analysis in a Low-Income Setting: using Accimaps to Guide Road Safety Recommendations in Bangladesh.” Safety Science 124: 104589. doi:10.1016/j.ssci.2019.104589.
- Hanretty, C., and C. Koop. 2012. “Measuring the Formal Independence of Regulatory Agencies.” Journal of European Public Policy 19 (2): 198–216. doi:10.1080/13501763.2011.607357.
- Holmes, G., A. Clacy, and P. M. Salmon. 2019. “Sports-Related Concussion Management as a Control Problem: Using STAMP to Examine Concussion Management in Community Rugby.” Ergonomics 62 (11): 1485–1494. doi:10.1080/00140139.2019.1654134.
- Khastgir, S., S. Brewerton, J. Thomas, and P. Jennings. 2021. “Systems Approach to Creating Test Scenarios for Automated Driving Systems.” Reliability Engineering & System Safety 215: 107610. doi:10.1016/j.ress.2021.107610.
- King, B. J., G. J. M. Read, and P. M. Salmon. 2022. “The Risks of Brain-Computer Interfaces: A Systematic Review.” International Journal of Human-Computer Interaction.
- Leveson, N. 2004. “A New Accident Model for Engineering Safer Systems.” Safety Science 42 (4): 237–270. doi:10.1016/S0925-7535(03)00047-X.
- Leveson, N., N. Dulac, K. Marais, and J. Carroll. 2009. “Moving beyond Normal Accidents and High Reliability Organizations: A Systems Approach to Safety in Complex Systems.” Organization Studies 30 (2-3): 227–249. doi:10.1177/0170840608101478.
- Leveson, N. 2011. “Applying Systems Thinking to Analyze and Learn from Events.” Safety Science 49 (1): 55–64. doi:10.1016/j.ssci.2009.12.021.
- Lintern, G., and P. N. Kugler. 2017. “Sociotechnical System Safety: Hierarchical Control versus Mindfulness.” Systems Engineering 20 (4): 307–317. doi:10.1002/sys.21396.
- McIlroy, R. C., K. A. Plant, M. S. Hoque, J. Wu, G. O. Kokwaro, V. H. Nam, and N. A. Stanton. 2019. “Who is Responsible for Global Road Safety? A Cross-Cultural Comparison of Actor Maps.” Accident; Analysis and Prevention 122: 8–18. doi:10.1016/j.aap.2018.09.011.
- McLean, S., G. J. M. Read, J. Thompson, C. Baber, N. A. Stanton, and P. M. Salmon. 2021. “The Risks Associated with Artificial General Intelligence: A Systematic Review.” Journal of Experimental & Theoretical Artificial Intelligence 35 (5): 649–663. doi:10.1080/0952813X.2021.1964003.
- Newnam, S., and N. Goode. 2015. “Do Not Blame the Driver: A Systems Analysis of the Causes of Road Freight Crashes.” Accident; Analysis and Prevention 76: 141–151. doi:10.1016/j.aap.2015.01.016.
- NTC. 2021. The Regulatory Framework for Automated Vehicles in Australia: Policy Paper. Melbourne: National Transport Commission. https://www.ntc.gov.au/transport-reform/ntc-projects/in-service-safety-AVs.
- NTSB. 2017. Highway Accident Report: Collision Between a Car Operating With Automated Vehicle Control Systems and a Tractor-Semitrailer Truck Near Williston, Florida, May 7, 2016. https://www.ntsb.gov/investigations/accidentreports/reports/har1702.pdf
- NTSB 2019. Highway Accident Report: Collision Between Vehicle Controlled by Developmental Automated Driving System and Pedestrian, NTSB/HAR-19/03. https://www.ntsb.gov/investigations/AccidentReports/Reports/HAR1903.pdf
- NTSB 2020. Highway Accident Report: Collision Between a Sport Utility Vehicle Operating With Partial Driving Automation and a Crash Attenuator, NTSB/HAR-20/01. https://www.ntsb.gov/investigations/AccidentReports/Reports/HAR2001.pdf
- Papadimitriou, E., C. Schneider, J. Aguinaga Tello, W. Damen, M. Lomba Vrouenraets, and A. ten Broeke. 2020. “Transport Safety and Human Factors in the Era of Automation: What Can Transport Modes Learn from Each Other?” Accident; Analysis and Prevention 144: 105656. doi:10.1016/j.aap.2020.105656.
- Parnell, K. J., N. A. Stanton, and K. L. Plant. 2017. “What’s the Law Got to Do with It? Legislation Regarding in-Vehicle Technology Use and Its Impact on Driver Distraction.” Accident; Analysis and Prevention 100: 1–14. doi:10.1016/j.aap.2016.12.015.
- Pöllänen, E., G. J. M. Read, B. R. Lane, J. Thompson, and P.M. Salmon. 2020. “Who is to Blame for Crashes Involving Autonomous Vehicles? Exploring Blame Attribution Across the Road Transport System.” Ergonomics 63 (5): 525–537. doi:10.1080/00140139.2020.1744064.
- Rasmussen, J. 1997. “Risk Management in a Dynamic Society: A Modelling Problem.” Safety Science 27 (2–3): 183–213. doi:10.1016/S0925-7535(97)00052-0.
- Read, Gemma J.M., Alison O'Brien, Neville A. Stanton, and Paul M. Salmon. 2022. “Learning Lessons for Automated Vehicle Design: Using Systems Thinking to Analyse and Compare Automation-Related Accidents Across Transport Domains.” Safety Science 153: 105822. doi:10.1016/j.ssci.2022.105822.
- Read, G. J. M., A. Naweed, and P. M. Salmon. 2019. “Complexity on the Rails: A Systems-Based Approach to Understanding Safety Management in the Rail Industry.” Reliability Engineering & System Safety 188: 352–365. doi:10.1016/j.ress.2019.03.038.
- Read, G. J. M., P. M. Salmon, N. Goode, M. van Mulken, M. G. Lenné, N. Stevens, and G. H. Walker. 2020. “Interaction-Centred Design: An End User Evaluation of Road Intersection Concepts Developed Using the Cognitive Work Analysis Design Toolkit (CWA-DT).” Ergonomics 63 (10): 1221–1239. doi:10.1080/00140139.2020.1782996.
- Read, G. J. M., S. Shorrock, G. H. Walker, and P. M. Salmon. 2021. “State of Science: Evolving Perspectives on ‘Human Error.” Ergonomics 64 (9): 1091–1114. doi:10.1080/00140139.2021.1953615.
- Rudin-Brown, C. M., and H. A. Parker. 2004. “Behavioural Adaptation to Adaptive Cruise Control (ACC): Implications for Preventive Strategies.” Transportation Research Part F: Traffic Psychology and Behaviour 7 (2): 59–76. doi:10.1016/j.trf.2004.02.001.
- Salmon, P. M., N. A. Stanton, G. H. Walker, N. Goode, J. Thompson, A. Hulme, and G. J. M. Read. 2022. Systems Thinking Methods: Practical Guidance and Case Study Applications. Boca Raton, FL: CRC Press.
- Salmon, P. M., R. McClure, and N. A. Stanton. 2012. “Road Transport in Drift? Applying Contemporary Systems Thinking to Road Safety.” Safety Science 50 (9): 1829–1838. doi:10.1016/j.ssci.2012.04.011.
- Salmon, P. M., and G. J. M. Read. 2019. “Many Model Thinking in Systems Ergonomics: A Case Study in Road Safety.” Ergonomics 62 (5): 612–628. doi:10.1080/00140139.2018.1550214.
- Salmon, P. M., G. J. M. Read, V. Beanland, J. Thompson, A. Filtness, A. Hulme, R. McClure, and I. Johnston. 2019. “Bad Behaviour or Societal Failure? Perceptions of the Factors Contributing to Drivers’ Engagement in the Fatal Five Driving Behaviours.” Applied Ergonomics 74: 162–171. doi:10.1016/j.apergo.2018.08.008.
- Salmon, P. M., G. J. M. Read, N.A. Stanton, and M. G. Lenné. 2013. “The Crash at Kerang: Investigating Systemic and Psychological Factors Leading to Unintentional Non-Compliance at Rail Level Crossings.” Accident; Analysis and Prevention 50: 1278–1288. doi:10.1016/j.aap.2012.09.029.
- Salmon, P. M., G. J. M. Read, and N. Stevens. 2016. “Who is in Control of Road Safety? A STAMP Control Structure Analysis of the Road Transport System in Queensland, Australia.” Accident; Analysis and Prevention 96: 140–151. doi:10.1016/j.aap.2016.05.025.
- Salmon, P. M., G. J. M. Read, J. Thompson, S. McLean, and R. McClure. 2020. “Computational Modelling and Systems Ergonomics: A System Dynamics Model of Drink Driving-Related Trauma Prevention.” Ergonomics 63 (8): 965–980. doi:10.1080/00140139.2020.1745268.
- Salmon, P. M., G. J. M. Read, G. H. Walker, N. Goode, E. Grant, C. Dallat, T. Carden, A. Naweed, and N. A. Stanton. 2018. “STAMP Goes EAST: integrating Systems Ergonomics Methods for the Analysis of Railway Level Crossing Safety Management.” Safety Science 110 (Part B): 31–46. doi:10.1016/j.ssci.2018.02.014.
- Sgobba, T. 2019. “B-737 MAX and the Crash of the Regulatory System.” Journal of Space Safety Engineering 6 (4): 299–303. doi:10.1016/j.jsse.2019.09.006.
- Stanton, N. A., and C. Harvey. 2017. “Beyond Human Error Taxonomies in Assessment of Risk in Sociotechnical Systems: A New Paradigm with the EAST ‘Broken-Links’ Approach.” Ergonomics 60 (2): 221–233. doi:10.1080/00140139.2016.1232841.
- Stanton, N. A., C. Harvey, and C. K. Allison. 2019. “Systems Theoretic Accident Model and Process (STAMP) Applied to a Royal Navy Hawk Jet Missile Simulation Exercise.” Safety Science 113: 461–471. doi:10.1016/j.ssci.2018.12.020.
- Stanton, N.A., P.M. Salmon, L. Rafferty, G. Walker, C. Baber, and D.P. Jenkins. 2013. Human Factors Methods: A Practical Guide for Engineering and Design. 2nd ed. Ashgate, UK: Ashgate.
- Stanton, N.A., P.M. Salmon, G.H. Walker, and M. Stanton. 2019. “Models and Methods for Collision Analysis: A Comparison Study Based on the Uber Collision with a Pedestrian.” Safety Science 120: 117–128. doi:10.1016/j.ssci.2019.06.008.
- Staton, M., J. Barnes, A. Morris, and P. Waterson. 2022. “Over to You’: Using a STAMP Control Structure Analysis to Probe Deeper into the Control of UK Road Safety at a Municipal Level—the Case of Cambridgeshire.” Ergonomics 65 (3): 429–444. doi:10.1080/00140139.2021.1968033.
- Thompson, J., G. J. M. Read, J. Wijnands, and P. M. Salmon. 2020. “The Perils of Perfect Performance; considering the Effects of Introducing Autonomous Vehicles on Rates of Car vs Cyclist Conflict.” Ergonomics 63 (8): 981–996. doi:10.1080/00140139.2020.1739326.
- WHO 2018. Global Status Report on Road Safety. https://www.who.int/publications/i/item/9789241565684
- Woolley, M., N. Goode, P. M. Salmon, and G. J. M. Read. 2020. “Who is Responsible for Construction Safety in Australia? A STAMP Analysis.” Safety Science 132: 104984. doi:10.1016/j.ssci.2020.104984.