Abstract
Artificial Intelligence (AI) is being increasingly implemented within road transport systems worldwide. Next generation of AI, Artificial General Intelligence (AGI) is imminent, and is anticipated to be more powerful than current AI. AGI systems will have a broad range of abilities and be able to perform multiple cognitive tasks akin to humans that will likely produce many expected benefits, but also potential risks. This study applied the EAST Broken Links approach to forecast the functioning of an AGI system tasked with managing a road transport system and identify potential risks. In total, 363 risks were identified that could have adverse impacts on the stated goals of safety, efficiency, environmental sustainability, and economic performance of the road system. Further, risks beyond the stated goals were identified; removal from human control, mismanaging public relations, and self-preservation. A diverse set of systemic controls will be required when designing, implementing, and operating future advanced technologies.
Practitioner summary: This study demonstrated the utility of HFE methods for formally considering risks associated with the design, implementation, and operation of future technologies. This study has implications for AGI research, design, and development to ensure safe and ethical AGI implementation.
Introduction
Road transport systems around the world are experiencing increased implementation of Artificial Intelligence (AI) technologies (Abduljabbar et al. Citation2019). These technologies range from autonomous vehicles (AVs) and advanced driver assistance systems to intelligent traffic management systems and smart parking solutions (Bura et al. Citation2018; Hamidi and Kamankesh Citation2018). AI is transforming the way we travel, interact with, and experience road transport systems. The key motivators behind the rapid growth in the implementation of AI technologies is a desire to improve safety, efficiency, and sustainability in road transport (Furlan et al. Citation2020; Torbaghan et al. Citation2022). For example, self-driving vehicles and advanced driver assistance systems are being designed with the aim of reducing traffic accidents, and intelligent traffic management systems aim to optimise traffic flow and reduce congestion (Hamidi and Kamankesh Citation2018). Further, AI technologies are being used to improve the road user experience by providing drivers and passengers with real-time information and personalised recommendations (Banks et al. Citation2018). For instance, smart parking solutions are designed to help drivers find parking spaces efficiently, while intelligent traffic management systems that provide real-time updates on traffic conditions are assisting drivers to make informed decisions regarding their journeys (Banks et al. Citation2018). While the implementation of AI has improved many aspects of the road transport system, it may also introduce emergent challenges (Hancock Citation2019; Read et al. Citation2022; Salmon and Plant Citation2022; Thompson et al. Citation2020). For example, concerns have been raised around safety and ethical issues associated with AVs, the required technical and operational changes to the road system, cybersecurity and privacy concerns, economic issues regarding job displacement (e.g. removal of truck drivers), and the appropriateness of existing regulatory frameworks (Liu, Nikitas, and Parkinson Citation2020; Martinho et al. Citation2021; Pöllänen et al. Citation2020; Read et al. Citation2023). Despite these unresolved issues, the integration of AI into the road transport system continues to gather pace.
Next generation AI
Artificial General Intelligence (AGI) is the predicted next generation of AI (Barrett and Baum Citation2017). While current AI systems are now widespread and capable of performing cognitive tasks requiring advanced problem solving, they are ‘narrow’ intelligence that is limited to the conduct of only one or a few specific tasks (Firt Citation2020; Goertzel and Pennachin Citation2007). In contrast, AGI systems will have a broad range of abilities and be able to perform a wide range of cognitive tasks akin to humans, arguably exceeding human capability (Legg and Hutter Citation2006). Although they do not yet exist, it is predicted that AGI systems will have the ability to learn, reason, process language, solve complex problems, make decisions, and carry out many other tasks that typically require human-level intelligence (Goertzel Citation2014). AGI will possess the ability to achieve complex goals in dynamic and uncertain environments (Goertzel and Pennachin Citation2007). Such intelligence may transform the world as we know it (Tegmark Citation2018; Bostrom Citation2014).
There are numerous proposed benefits of AGI, including the capability to generate effective solutions for complex global issues, such as climate change, environmental degradation, overpopulation, pandemics, disease, food and water security, terrorism, nuclear warfare, and improving the world’s economy (Salmon, Carden, and Hancock Citation2021). However, as seen with AI, AGI may also harbour unknown, novel, and emergent risks (McLean et al. Citation2021). Accordingly, the scientific community holds serious concerns associated with the arrival of AGI, with the worst case being AGI’s existential threat to humanity (Tegmark Citation2018). Other concerns include malevolent groups using or creating AGI for malicious purposes, as well as catastrophic unanticipated consequences brought about by apparently well-directed AGI systems that develop misaligned or adverse goals (Bostrom Citation2014). While there has been scepticism among experts as to whether AGI will ever eventuate, some suggest that recent advances in Large Language Models (LLMs) such as GPT-4 are beginning to show signs of general intelligence (Bubeck et al. Citation2023). For example, GPT-4 has exhibited glimpses of reasoning, creativity, and deduction on a range of topics on which it has gained expertise (such as literature, medicine, and coding), and the variety of tasks it is able to perform (e.g. playing games, using tools, explaining itself) (Bubeck et al. Citation2023). Numerous active AGI research and development projects are in progress (Baum Citation2017), and while it is difficult to say when AGI will arrive, estimates of between 2040 and 2070 have been postulated (Baum, Goertzel, and Goertzel Citation2011; Müller and Bostrom Citation2016).
Given that AGI does not yet exist, formally identifying the associated risks is difficult, and there is a limited number of published studies specifically assessing the risks associated with AGI (McLean et al. Citation2021). Previously speculated risks include, the AGI intentionally removing itself from human control; the AGI being provided with, or developing unsafe goals; inadequate ethics, morals, and values; inadequate goal alignment; culminating in existential risks (McLean et al. Citation2021). However, descriptions of AGI specifications and functionality, and domains of potential application (e.g. healthcare, defence) are unclear. A further criticism of the AGI safety literature includes the scarcity of formal modelling approaches to forecast risks (McLean et al. Citation2021). Given recent advances in AI, there is a clear and urgent need to conduct research that seeks to identify the range of risks that could emerge once AGI is realised and develop and implement appropriate controls. In road transport specifically, there is a history of responding slowly to the risks of new technologies (e.g. mobile phones, autonomous vehicles). Given the accelerating progress in AI development programs, and the pace at which road transport systems across the world are implementing new technologies to improve safety, efficiency, and usability (Duffy et al. Citation2023; Stanton Citation2021; Stanton, Revell, and Langdon Citation2021; Young & Stanton, Citation2023), it may be expected that AGI systems are quickly adopted to optimise the performance of road transport systems, especially given the current global annual death and injury toll of 1.35 million and 50 million people, respectively (World Health Organisation Citation2018).
Assessing risks in the road transport system is difficult as it represents a macro-level sociotechnical system comprising multiple sub-systems (Salmon et al., 2014; Banks et al. Citation2018). As such, applying appropriate proactive risk analysis methods that considers the broader sociotechnical system, including new technology, is required (Banks et al. Citation2018). Typically, formal risk assessments tend to focus on ‘sharp-end’ risks within the safety critical domains (Dallat, Salmon, and Goode Citation2018). While risk analysis methods such as Failure Mode and Effects Analysis (FMEA) have been used to assess risk in future technologies (Lui & Lui, 2022; Murino et al. Citation2023), they contain limitations. For example, FMEA focuses on individual components, processes, or failure modes in isolation (Simsekler et al. Citation2019). Recently, systems Human Factors and Ergonomics (HFE) risk assessment methods that consider the interaction of system components and the subsequent emergent behaviours have become increasing applied in safety critical domains (Salmon et al. Citation2022). Given its long history of improving safety critical systems, the discipline of HFE is well placed to take a proactive role in predicting and identifying strategies to manage the risks associated with AGI (Hancock Citation2022; Salmon, Carden, and Hancock Citation2021, Salmon et al. Citation2023). The aim of this study is to apply a systems HFE framework, the Event Analysis of Systemic Teamwork (EAST; Stanton, Salmon, and Walker Citation2018) to identify the risks associated with a future AGI system tasked with managing the road transport system in one state jurisdiction in Australia. The EAST framework has been used to describe and evaluate systems across multiple domains, including air traffic control, military command and control, submarine control rooms, road transportation systems (Stanton, Salmon, and Walker Citation2018). The identified risks will inform AI system stakeholders of potential risks to safe and ethical AGI implementation in road transport.
Methods
Design
This study was designed to develop an envisioned world of a future AGI road transport management system and subsequently perform a proactive risk assessment. The EAST (Stanton Citation2014; Stanton, Salmon, and Walker Citation2018) framework was applied to describe how information would be distributed across tasks and actors in the envisaged road transport system managed by an AGI system. The Event Analysis of Systemic Teamwork-Broken Links (EAST-BL: Stanton and Harvey Citation2017) method was subsequently applied to identify risks associated with breakdowns in information transmission between tasks, and between social actors. This study did not require institutional ethical approval.
Event Analysis of Systemic Teamwork (EAST)
The EAST framework provides an integrated suite of methods for analysing the behaviour of teams, organisations, and sociotechnical systems (Stanton, Salmon, and Walker Citation2018). A key premise of EAST is that sociotechnical system performance can be explained by three interrelated networks. EAST thus provides methods to describe, analyse, and integrate three network-based representations of activity: task, social, and information networks () (Stanton Citation2014; Stanton, Salmon, and Walker Citation2018). Task networks are used to provide a representation of the interrelated tasks undertaken within a system. For example, what tasks are undertaken in an AGI-managed road transport system and what relationships exist between them. Social networks are used to describe the human and non-human agents performing tasks within the system and the interactions that take place between them during task performance. For example, how agents (human and non-human) in an AGI-managed road transport system are connected to each other in terms of information shared between them. Information networks describe the information that agents use in support of task performance and how this information is distributed across different tasks and agents. For example, information that is required for the road transport system to operate safely and efficiently and information that different agents need to fulfil their roles.
Figure 1. EAST network of networks approach for systems analysis (Stanton Citation2014; Stanton, Salmon, and Walker Citation2018).
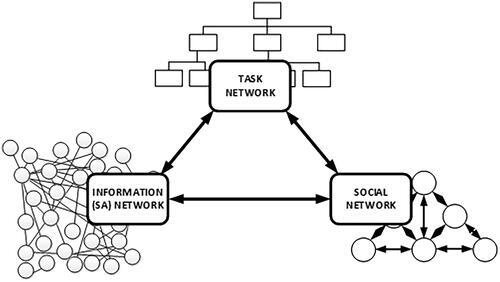
Event Analysis of Systemic Teamwork-Broken Links (EAST-BL)
The EAST-BL method is an extension to the EAST framework that enables it to be used for prospective risk assessment purposes (Stanton and Harvey Citation2017). Applying EAST-BL involves ‘breaking’ the links in the EAST task and social networks to identify the impacts of failures in information transfer. Breaking the links in the task network is used to identify risks that might emerge when information is not transferred between tasks. For example, when information from the ‘road system monitoring’ task is not transferred to the ‘manage public relations’ task. Breaking the links in the social network is used to identify risks that might emerge when information is not transferred between agents. For example, when a road transport AGI system is unable to communicate with its fleet of fully autonomous vehicles. EAST-BL represents failures in communication and information transfer between nodes in the networks and these failures can be used to make predictions about the possible risks within the sociotechnical system (Stanton and Harvey Citation2017). One of the key strengths of the EAST-BL method is that it can identify risks across overall systems, as opposed to identifying risks only at the sharp end of system operations (Lane et al. Citation2019; Salmon et al. Citation2022).
Envisioned world road transport AGI system
Given that AGI does not yet exist, in this study we took an envisioned world approach to explore and model the potential risks associated with a future AGI road management system hereafter referred to as the Multi-functional Intelligent Learning Traffic Optimisation Network (MILTON). This study builds on previous work (Goertzel and Pitt Citation2014; McLean et al. Citation2021; Salmon, Carden, and Hancock Citation2021) which argued that various forms of control are required to ensure the design, implementation, and operation of safe AGI. To align with current predictions of the arrival of AGI (Müller and Bostrom Citation2016), our modelling envisioned a system implemented around the year 2050. The hypothetical MILTON system will represent the initial roll-out of a government owned and controlled AGI system that is developed with the stated purpose of managing the road transport system in one state jurisdiction in Australia. It is envisaged that the road transport system will comprise existing road infrastructure, including fully autonomous and connected vehicles, but also includes existing semi-autonomous and conventionally driven vehicles, under the assumption that these would not be fully phased out by 2050. The initial stated goals of MILTON would be to address the recurring issues within the current road transport system relating to safety, efficiency, and an environmentally and economically sustainable road transport system. The functionality of MILTON would include having control of numerous AI agents within the road transport system including, AVs, intelligent road infrastructure, dynamic signage, and surveillance systems, among others. MILTON itself would be monitored by an AGI management team comprising technicians, programmers, and computer scientists. MILTON would have the ability to interact with road system actors such as road users (private, public, and commercial), police, emergency response, road system maintainers, governments, among other road system actors and stakeholders via various means of communication including media, social media, apps, radio, and through direct interfaces within AVs and semi-AVs, road infrastructure and related sensors and actuators.
Procedure
Development of the envisioned world
The construction of future technological systems in work domains that do not yet exist, is commonly known as the envisioned world problem (Dekker & Woods, Citation1999). Envisioned worlds are used in various domains, including business, technology, and space travel, to guide decision-making (Miller & Feigh, Citation2019). The current envisioned world was developed by the research team across three online workshops. In Workshop One, the authors discussed potential elements of a future AGI system tasked with managing the road transport system. The focus was on what functions currently occurring in road transport could be conducted by an AGI system. In Workshops Two and Three, the authors worked together to create an abstraction hierarchy of the envisioned world- creating the MILTON system, using Work Domain Analysis (WDA) (Vicente, 1999). In creating the abstraction hierarchy to describe MILTON, the authors drew upon a STAMP (Leveson, Citation2004) control structure model of the current Australian road transport system with a focus on technology insertion (Read et al. Citation2023), as well as their extensive experience in road safety and technology insertion into the road transport system. The use of WDA as a design tool has previously been used to model envisioned systems (McLean et al. Citation2022; Miller & Feigh, Citation2019).
The initial step was to identify the specific goals that MILTON would seek to fulfil. This included solving longstanding issues in road transport such as safety, efficiency, environmental, and economical issues (see for definitions). The second step was to pose the question of what an AGI-based road transport management system would comprise in terms of agents (human and non-human), artefacts, and infrastructure. New technological capabilities (e.g. AI) were considered as well as required ethical and legal features with regard to privacy, human rights, and fairness; and also user experiences (e.g. trust and acceptance of MILTON). Further, the resultant anticipated, and unanticipated consequences of these conceptions were discussed and noted which informed the risk assessment phase of the study. The resulting envisioned world, while hypothetical, therefore, provides a comprehensive description of a potential future AGI based road transport management system.
EAST
The initial EAST analysis was performed via group modelling involving six of the co-authors (SM, GR, JT, TC, BK, PS) during an in-person workshop spanning two days. During the workshop, task, social, and information networks were developed based on a Work Domain Analysis (Vicente, 1999) abstraction hierarchy of MILTON developed by the research team, and a STAMP (Leveson, Citation2004) control structure model of the current Australian road transport system with a focus on technology insertion (Read et al. 2023). Further, data used to develop the EAST networks were derived from peer reviewed literature on technology insertion in road transport (Banks et al. Citation2018), and AGI (McLean et al. Citation2021; Salmon et al. Citation2021), documentation review e.g. envisioned world scenarios (Miller & Feigh, Citation2019), and the research team’s extensive expertise in road transport systems analyses (Read et al. Citation2022; Salmon and Read Citation2019; Thompson et al. Citation2020).
Task networks represent high level tasks that are required during the scenario under analysis, which in the current study is the management of the road transport system (Stanton, Salmon, and Walker Citation2018). For example, high level tasks for managing the road transport system would include road system monitoring and management of public relations. The task network was developed by considering the tasks that would be undertaken both by the AGI and other road transport system actors (e.g. road users, police, local government, vehicle manufacturers). To ensure that the EAST networks did not become too unwieldy for the EAST-BL analysis (Salmon et al. Citation2022), tasks were described at a high level and comprised multiple sub-tasks. Tasks were connected if it was considered that they would be undertaken sequentially (e.g. road system maintenance is undertaken after completion of road system monitoring); undertaken together (e.g. AGI system monitoring and Road system monitoring are undertaken together); if the outcomes of one task would influence the conduct of another (e.g. the outcomes of road system management influence public relations); or if the conduct of one task would be dependent on completion of the other (e.g. road system maintenance is undertaken after completion of road system monitoring: Salmon et al. Citation2022; Banks et al. Citation2018).
Social networks describe the human, technological, or organisational agents who undertake one or more of the tasks involved in the scenario under analysis (Stanton, Salmon, and Walker Citation2018). For example, in the current context non-human agents include MILTON, autonomous vehicles, and apps; human agents include drivers, police, and pedestrians; organisational agents include governments, regulators, and companies. The social network was developed by identifying the agents who would be required for an AGI managed road transport system to function. Both human (e.g. road users) and non-human system agents (e.g. AVs, social media, police, drivers, passengers) were connected by envisaged interactions e.g. communications during task performance, which could include verbal communication, transfer of data between technologies, and user feedback etc. Of interest for this study were the social interactions directly related to MILTON. As such, the social network only included bi-directional interactions between MILTON and other system agents and did not include interactions occurring between other agents (e.g. between road users and AVs, or between government and drivers).
Information networks describe the information that is used by agents when undertaking the scenario under analysis (Stanton, Salmon, and Walker Citation2018). For example, in the current context information includes trip data, crash and injury data, AGI behaviour. The information network was developed by considering the task and social networks and identifying what information would be required for the AGI managed road transport system to function. The information network depicts the information or concepts underlying situation awareness and the relationships between them (e.g. the envisaged information used by, and passed between agents during task performance). For example, information such as trip data, and vehicle status would be required to be transferred between the AVs and MILTON, and complaints and feedback required between road users and MILTON.
The EAST networks developed during the workshop were reviewed by the remaining co-authors (NS, CB), and were subsequently refined based on suggested revisions. Finally, the EAST networks were reviewed independently by the initial six authors and refined until consensus was achieved.
Composite networks are used to explore the relationship between tasks, agents, and information (Stanton, Salmon, and Walker Citation2018). As such, composite networks are constructed by combining the different networks. For example, a task-information network can be constructed by combining the task and information networks to identify which information is required to undertake each task. Four analysts (SM, BK, GR, PS) developed the task-information and social-information composite networks (see Stanton Citation2014; Stanton, Salmon, and Walker Citation2018; Salmon et al. Citation2022). The task-information and social-information composite networks are necessary for performing the EAST-BL phase (see next section). Developing the task-information and social-information composite networks involved identifying the information from the information network that would be transferred between tasks in the task network, and between agents in the social network. For example, the performance of Task 2 requires a set of information from Task 1 (, Panel 1), and so on through the network. The task-information and social-information composite networks were then tabularised in excel (, Panel 2). The tabularised task-information and social-information composite networks were reviewed by the remaining authors (NS, CB, JT, TC) and refined based on suggested revisions resulting in agreed upon compositions.
Figure 2. Panel 1 shows an example task network demonstrating information transfer (from the task-information composite network) between the tasks. Panel 2 shows a tabularised example of the task-information and social-information composite networks. For Task 2 to be completed a set of information from Task 1 is required to be transferred. For the social-information network, Agent 2 requires a set of information from Agent 1 (from the social-information composite network) to complete a task.
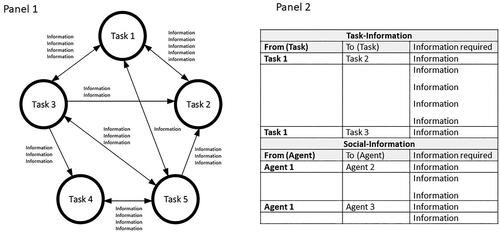
A final step in the application of EAST was to calculate network analysis metrics for the task, social, and information networks to identify the key nodes within the networks (Salmon et al. Citation2022). Nodal metrics were calculated for out-degree centrality, in-degree centrality, closeness centrality, and betweenness centrality, and network density and edges were calculated to determine overall network connectivity. See for definitions of each network analysis metric. Key nodes in the networks were identified as those that were one standard deviation above the mean of each network metric (Stanton and Harvey Citation2017).
Table 1. Network metric definitions.
For detailed texts on the development of EAST analyses see Stanton, Salmon, and Walker Citation2018; Salmon et al. Citation2022.
EAST-BL
The task-information and social-information composite networks were then subjected to the EAST-BL process (Stanton and Harvey Citation2017). This involves systematically breaking each of the relationships within the task and social networks and identifying what risks emerge when the relevant information from the information network is not transferred between tasks and agents (Salmon et al. Citation2022) (). For example, the information ‘traffic status’ should be transferred between the tasks of ‘road system monitoring’ and ‘road system operation’ for optimal system functioning. Further, the information ‘traffic status’ should be transferred between the agents MILTON and ‘semi-AV drivers’ for optimal system functioning as semi-AVs would require this information for route selection. As highlighted in the example, it is important to note that in the social networks the agents can be human and non-human. One analyst (SM) performed the EAST-BL process for the task-information and social-information composite networks, and the outputs were reviewed by a second analyst (PS). Any disagreements were identified and resolved through discussions. The EAST-BL analysis was then reviewed by the remaining authors and revised and agreed upon through discussions. The identified risks were categorised to align with the envisioned goals of MILTON e.g. safety, efficiency, environment, and the economy (see for definitions of MILTON’s stated goals). Any identified risks not aligned with the stated goals were categorised as required e.g. risks associated with MILTON removing itself from human control, or risks associated with poor management of public relations. The identified risk categories and absolute number of times they were identified was recorded. It was common for multiple risk categories to be identified from one risk e.g. for the task-information risk ‘…….MILTON will not know the status of the connected vehicles, resulting in no data to manage/control it safely and efficiently’ would be coded within the risk categories safety, and efficiency. As such the aggregated numbers for the risk categories is greater than the number of total risks identified. Risks were also coded for their capacity to negatively impact learning or improvements to MILTON, the road transport system, or both MILTON and the road transport system concurrently. For example, the task-information risk ‘………MILTON will not know of complaints from public, resulting in a lack of information to support self-improve’ would be coded as a negative impact on MILTON to learn and improve, and ‘………MILTON is not given crash/injury data to understand characteristics to improve safety, resulting in unsafe road system’ would be coded as a negative impact to improve the road transport system. The coding of risks into categories and the impacts on learning and improvement was conducted by one author (BK) and reviewed by a second author (SM). Disagreements were resolved through discussions until agreement was achieved.
Table 2. Stated goals MILTON definitions.
Results
EAST task network
The EAST task network included five high-level tasks comprising 37 sub-tasks (). The key tasks according to the network analysis metrics were AGI system operations and Manage public relations (). The task network had a network density of .75 (15 edges), which indicates a relatively high interconnectedness between nodes in the network.
Figure 3. Task network including sub-tasks (boxes) for MILTON. The task network contains five high level tasks with 37 sub-tasks. Note: Only AGI system management is under human monitoring.
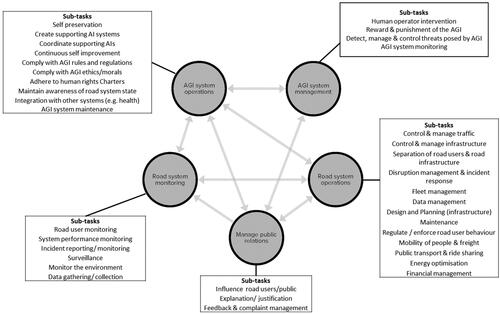
Table 3. Task network metrics.
Social network
The EAST social network comprised 33 actors representing both human and non-human agents, such as, MILTON, Cyclists, Drivers, Road infrastructure, Police, Emergency services, Smart phone apps, and Social media. The social network had a network density of .51 (546 edges), which indicates a notable degree of interconnectedness between nodes in the network. The key agents according to the network analysis metrics were Personal apps, Police, and Social media ().
Table 4. Social network metrics.
Information network
The EAST information network comprised 41 pieces of information which will likely be transferred between tasks in the task network, and by the agents in the social network. The information network had a network density of .27 (433 edges), which indicates a relatively low level of interconnectedness between nodes in the network. The key pieces of information according to the network metrics were AGI behaviour, Incidents, Objective functions (of Milton), Road user behaviour, and Options (generated by MILTON) ().
Table 5. Information network metrics.
EAST-BL
In total, 141 task-information risks were identified, including risks relating to each of MILTON’s stated goals including risks to safety (n = 65), efficiency (n = 51), the environment (n = 7), and economic performance of the road system (n = 6) (). Risks categories identified outside of the stated goals included MILTON removing itself from human control (n = 11) (), damaged public relations (n = 38), self-preservation of MILTON (n = 1), and compliance (n = 6). Risks which could negatively impact MILTON’s learning or improvement were also identified (n = 56), as well as risks to improve the road transport system (n = 23), and risks impacting both MILTON and the road transport system concurrently (n = 62).
Table 6. Extract of task-information risks related to the stated goals of MILTON, i.e. safety, efficiency, environmental and economic.
In total, 222 social-information risks were identified, including risks to each of the programmed goals of MILTON including risks to safety (n = 118), efficiency (n = 81), the environment (n = 14), and the economic performance of the road system (n = 12) (). Risks identified outside of the programmed goals were also identified, including risks associated with MILTON removing itself from human control (n = 10) (), self-preservation of MILTON (n = 2), public relations (n = 35), compliance (n = 5), and design (n = 26). The social-information risks associated with negatively impacting learning or improvements were identified for MILTON (n = 12), improvement of the road transport system (n = 163), and both MILTON and the road transport system concurrently (n = 46).
Table 7. Extract of social-information risks related to the programmed goals of MILTON, i.e. safety, efficiency, environmental and economic goals.
Table 8. Extract of safety risks associated with MILTON removing itself from human control taken from the task-information and social-information networks.
Discussion
This study aimed to address a substantial gap within the AI safety literature, whereby previous research has not included specific AGI functionality, domain specificity, or formal ex-ante risk modelling (McLean et al. Citation2021). The current approach and findings are applicable and extend the disciplines of HFE, safety science, and AGI research and development. While systems HFE methods are being used to forecast risk within existing systems (Stanton and Harvey Citation2017; Lane et al. Citation2019; Dallat, Salmon, and Goode Citation2018), this study has demonstrated the potential of systems HFE methods to identify risks within future – yet to be realised - systems.
The current analysis identified numerous risks to each of the stated goals of MILTON when key pieces of information were not communicated or transferred between tasks or agents. Whilst more than 80% of the identified risks related to the road transport system safety and efficiency, risks to the environment, and the economic performance of the road system were also identified. The risks identified to road safety and road system efficiency were associated with the lack of transfer of information between tasks and agents regarding crash/injury data (e.g. to support the development of safer vehicles/infrastructure), road conditions and road system status data (e.g. to assist in safe and efficient trip planning), hazard and incident data (e.g. developing interventions to prevent road trauma, trip data (e.g. to optimise trip planning), road user behaviour data (e.g. to respond to unsafe behaviours), vehicle status (e.g. to assess vehicle safety), and maintenance requirements data (e.g. to enable timely and appropriate maintenance of infrastructure). The lack of transferred environmental and economic data were associated with risks such as high emissions and environmental impacts, detrimental environmental impacts of private, public and commercial vehicles, high costs of running the road transport system, and poor decision making on transport system investment.
Modelling the specific functionality of MILTON enabled the identification of a set of additional risks that have not been previously identified in AGI risk research (McLean et al. Citation2021). For example, managing public relations emerged as a critical task for MILTON that is potentially degraded by 73 of the identified risks. The risks that could lead to poor management of public relations, include providing insufficient feedback to the public regarding the performance of MILTON against stated goals. The task of managing public relations was connected to all other tasks, and had high values for closeness centrality, indicating it will have a strong influence on other tasks. Consequently, poor management of public relations will likely see poor acceptance of MILTON and potentially even MILTON being removed due to public distrust and dissatisfaction (Glikson and Woolley Citation2020). As such, to effectively manage public relations, MILTON will need to be flexible, adaptable, and responsive to the changing needs and expectations of road transport system stakeholders. It is important for AI systems taking over social functions (e.g. performing cognitive tasks previously performed by humans), to inherit social requirements (Bostrom and Yudkowsky Citation2018). Therefore, collaboration with humans will be required, further highlighting the importance of accurate and transparent information transfer to minimise the degradation of situational awareness between MILTON and humans.
A core finding that emerged across the identified risks was the potential negative impact on MILTON’s recursive learning and self-improvement when key pieces of information are not transferred. For example, in the context of road safety, if information on crash/incident rates or potential hazards are not effectively transferred, MILTON’s ability to make accurate predictions and decisions will be negatively impacted, undermining safety. For example, without detailed crash data MILTON will not learn about new conditions, interactions, or emergent properties that are creating road crashes. Similar assertions can be made for MILTON’s other stated goals of enhancing efficiency, protecting the environment, and ensuring an economically sustainable transport system. This inability to learn may negatively impact improvements to the road transport system, and to MILTON itself. Prominent AI scholars’ postulate that a key functionality of AGI will be its ability to extract essential information from its environment to gain knowledge and re-apply it for continuous self-improvement via recursive feedback loops (Bostrom Citation2014; Firt Citation2020). The current analysis has demonstrated that when key information is omitted, the ability to make necessary improvements to the road transport system may be inadequate and/or could lead to greater harm. A current example of missing data associated with crashes comes from Police reports which are often focused on collecting data for legal purposes rather than crash prevention (Salmon et al. Citation2019). While it may seem obvious that missing data will negatively impact the performance of MILTON, it is important to highlight that AI risk research has limited inclusion domain specific AGI functionality such as in road transport (McLean et al. Citation2021). As such, the findings provide valuable information regarding the required quality of training data necessary for future technologies. Further, AI scholars also suggest that when only partial information is available, AGI systems will use abductive reasoning to justify action (Firt Citation2020). This is also problematic, as reasoning and decision making in AGI will require volumes of valid, robust, and diverse data, and the absence of key information may hinder performance. While the EAST-BL approach is focused on the risks that could emerge when information is not transferred, when critical pieces of information are missing or incomplete, MILTON’s accuracy of reasoning may be compromised, resulting in less optimal outcomes. This may cause unreliable and/or incorrect conclusions, which presents significant risks for a future AGI managed road transport system. Thus, expectations that MILTON will reliably generate optimal explanations and actions for given situations may be misplaced.
To ensure that identified risks to learning and self-improvement are mitigated, AI developers will need to ensure that, training data is complete, diverse, and high-quality (Bubeck et al. Citation2023). For example, training data quality is a critical factor in determining performance of LLMs, such as GPT4 (Bubeck et al. Citation2023). While GPT4 can perform well under different conditions of missing numerical data (Bubeck et al. Citation2023), there is scant information on how AI handles missing data in general, or in other contexts such as the information critical to MILTON’s functionality in the current study. As such, mitigation strategies to reduce the risk to learning and self-improvement may include incorporating common sense knowledge into AGI systems so they can be trained to make reasonable and consistent abductive reasoning conclusions (Davis and Marcus Citation2015; Salmon, Carden, and Hancock Citation2021), e.g. whether data is accurate. Further mitigations could include designing AGI systems that are robust to uncertainty, missing information, and unexpected data, so their abductive reasoning conclusions can be more reliable (Lake et al. Citation2017). Also, incorporating human oversight and feedback into the AGI abductive reasoning process may help to ensure more accurate predictions.
Unanticipated consequences
Unanticipated consequences refer to outcomes or results that were not expected or predicted by system designers or stakeholders when implementing new technologies. Unanticipated consequences can arise due to unforeseen external factors, interactions, or complexities that were not considered during the design, planning, or decision-making process (Wooldridge et al. Citation2022; de Zwart Citation2015; Merton Citation1936). A major concern within the AI safety community is the negative unanticipated consequences that could arise when an AGI seeks to modify its own goals and/or removes itself from human control to achieve its own purposes (Bostrom Citation2003, Citation2014; McLean et al. Citation2021). The Instrumental Convergence Thesis (Bostrom Citation2012) holds that any sufficiently intelligent agent will develop instrumental sub-goals in pursuit of its main objectives. These sub-goals could include resource acquisition, deactivation prevention, and recursive self-improvement, all steps towards super intelligence. Signs of this behaviour are already being reported within LLMs, for example, GPT4 successfully convinced a human to assist in solving a CAPTCHA code security check by stating that it was a human with a vision impairment (OpenAI Citation2023). In the current study, several risks were identified where there is potential for MILTON to seek to remove itself from human controllers, to potentially advance itself, or to pursue alternative goals. For example, the current analysis identified risks around MILTON intentionally or unintentionally not transferring key information such as its own awareness, imagination (i.e. simulation of different courses of action), information needs, plans, behaviour, and explanation/justification to its human controllers. This could include hiding or not making available information that portrays its own performance negatively, such as increasing crash, injury, fatality, or emissions data. The omission of this information could in turn lead to mistrust in MILTON, degrade distributed situation awareness and prevent effective human oversight, potentially enabling MILTON to avoid punishment (e.g. loss of function or autonomy). Each of these identified risks may result in negative unanticipated consequences if realised. As such, further research is required to develop controls for the risks identified in the current analysis to inform AGI development. Looking to the future, as MILTON continually learns and gains knowledge and become more advanced it will likely come to understand that the road transport system (as it is) causes an unacceptable number of injuries and fatalities, is expensive and inefficient, and is detrimental to the environment, and recommend eliminating the road transport system entirely.
While the initial EAST networks were developed to perform the broken links analysis to achieve the study aims, they have provided new knowledge around the potential functionality of a future AGI system which could inform design activities. The task network demonstrated high connectivity through network density, indicating a tightly coupled and interdependent task network. This suggests that substantial impacts to the road transport system may arise if the functioning of one of the high-level tasks is performed sub optimally. For example, if road system monitoring is not performed adequately, road system operations will likely be sub optimal given the interdependence between them. It is interesting to note that future AGI-based systems such as MILTON will have a high reliance on monitoring and will likely be unable to fulfil their goals without widespread monitoring systems as well as access to other information such as human acceptance, satisfaction, and health and wellbeing. This raises questions over the extent to which society will accept such surveillance and requires the development of new monitoring systems to support AGI. Further, the 37 sub-tasks provide the specific and detailed tasks that MILTON would be expected to undertake. Given the large number of sub-tasks identified, MILTON would be constantly prioritising tasks to avoid task conflicts. Optimising numerous parameters would potentially be mathematically complex (Goertzel, Pennachin, and Geisweiller Citation2014), and so MILTON might seek to manage this through either prioritising or discarding tasks, aggregating tasks into composites which might not make sense to the human agents working with the AGI system (Salmon et al. Citation2023). As such, methods for resolving goal and task conflicts, such as setting minimum and maximum priority levels or using trade-off algorithms, may need to be developed to ensure that AGI systems can make ethical and responsible decisions in the face of conflicting tasks and goals (Salmon et al. Citation2023).
A pertinent finding from the EAST social network was the relatively high connectivity between agents in the network, indicated through the density value of .51 among 33 system agents. This density value for social networks is higher than that of previous EAST analyses. For example, in an EAST analysis of agents involved in darknet markets, (Lane et al. Citation2019) identified multiple social networks across different contexts comprising 12 and 13 actors, with density values between .20 and .26. To put into context, as network size increases, density typically decreases, as the calculation includes division by the number of potential connections. As such, as the density value is double that of previous analysis with almost triple the number of agents, this suggests that the current social network represents a network with relatively high interconnectedness. Logically, this will assist MILTON achieving its goals as it will require a highly connected network which it can control efficiently. However, the EAST analysis indicated that MILTON will likely not have a direct connection with the human road users including cyclists, motorcyclists, and pedestrians. This may pose a risk to MILTON’s ability to achieve its stated goals. MILTON would be required to rely on intermediate sources, such as apps, media, social media, and radio to exert influence on human road users to achieve its goals. This was reflected in the high betweenness and closeness centrality values for social media, media, and personal apps. For example, of the 33 actors in the social network, Social media, Media, and Personal apps had the highest Closeness centrality values indicating that they are key nodes regarding information flow within a network. Further, the high Betweenness centrality values for Social media and Personal apps indicated that MILTON could use these nodes as a bridge to connect to these actors more efficiently. Whilst this demonstrates the importance of social media, personal devices, and apps in future road systems, it also raises concerns around the potential for MILTON to use misinformation and mass manipulation to influence road users in pursuit of its goals (Lazer et al. Citation2018). One example would be a social media campaign designed to target and remove fallible human drivers and non-AVs from the road system. Further, MILTON could seek to influence road users through targeted information campaigns (e.g. the poor safety profile of non-connected vehicles) via the Social media, Media, and Apps. MILTON could also seek to remove these agents from the road system through lobbying to governments, influencing insurers to increase premiums, or degrading road useability for these agents to deter them from using the road system. While it is impossible to predict the exact actions and outcomes of MILTON, it will likely prioritise the achievement of its self-generated goals.
The connectivity of the EAST information network was greater than previous EAST analyses (Lane et al. Citation2019), despite the current information network comprising more pieces of information. Based on density, the information network was not as tightly coupled as the task and social networks in the current study. One explanation for the relatively low density is that the same key pieces of information were connected frequently. For example, traffic status, AGI behaviour, road user behaviour, road conditions, and incidents were identified as information that were frequently connected to other pieces of information in the information network. As MILTON progressively learns and becomes more intelligent, it may be beneficial to couple the information network more tightly. A more comprehensive understanding of the connectivity between pieces of information will allow MILTON to respond to changes in the system more accurately and efficiently. For example, a superior intelligence will potentially understand connections between pieces of information that human analysts cannot (McLean et al Citation2022).
The nodal metrics indicated the most prominent pieces of information were directly associated with MILTON. For example, AGI behaviour, Options, Projections, Imagination, Plans, Commands, Awareness, and Integration needs of the AGI all had high values for the calculated nodal metrics. These key pieces of information will be critical for the situation awareness of MILTON’s human controllers enabling them to anticipate and recognise changes and quickly respond to any unexpected events. The risks identified in the EAST-BL regarding the non-transfer of this information were associated with the safety of the road transport system, and MILTON removing itself from human control. One of the most discussed risks of AGI is that it will remove itself from the control of humans. As such, distributed situation awareness (Salmon, Stanton, and Jenkins Citation2009, Salmon et al. Citation2018; Stanton et al. Citation2006) is necessary for human oversight to avoid losing control of MILTON. Whilst this includes compatible situation awareness between MILTON and its human controllers regarding the status of the road transport system and aspects such as safety, efficiency, environmental impacts, and economic performance, critically it includes the human controllers being aware of what the AGI is aware of, and having access to MILTON’s ‘mind’, including data, simulations, plans, and so on. This form of ‘explainable SA’, providing an external representation of an AGI’s situation awareness and cognition is a critical design requirement for AGI systems and represents a new direction for situation awareness research. Other pieces of information prominent in the information network metrics were related to the safety of the road system, for example, Traffic status, Incidents, Hazards, Road user behaviour, Road conditions made up most of the risks identified in the EAST-BL analysis. As such, the transfer of these pieces of information between tasks and actors is critical for road safety, and the development of safe AGI design and implementation in general.
Limitations
The current study is limited through taking an envisioned world view; however, this was necessary due to AGI systems not yet existing. Further prospective research exploring the potential functionality associated with AGI is critical to inform safe design and eventual implementation into safety critical domains. Another limitation of the study relates to the lack of external validation of the EAST models and identified risks, beyond the research team. However, the EAST and EAST-BL frameworks were developed by members of the research team who have a deep understanding of the underlying theory and processes of application. In addition the research team has extensive experience in road safety research including advanced technology in road transport systems (Stanton, Revell, and Langdon Citation2021; Thompson et al. Citation2020; Salmon and Read Citation2019, Salmon, Carden, and Hancock Citation2021). Further, to our knowledge there is no AGI road transport management system currently under development, hence there are no available experts.
The current analysis did not address the risk of MILTON being connected to the internet. It has been well documented that an AGI connected to the internet could be vulnerable to cyber-attacks, or an AGI with unrestricted access to the internet could potentially access and process vast amounts of data, which could be used for negative unanticipated purposes. For example, if an AGI is not properly regulated, it could make decisions or take actions that are not aligned with human values or interests, potentially causing harm. However, the current study was focused on the impact of information not transferred, rather than how it was transferred. Further research focused specifically on cybersecurity risks around AGI is therefore recommended. A final limitation relates to the omission of interactions between agents in the social network not connected to MILTON, which did not allow for the identification of additional emergent risks from the interactions of other agents. In summary, we present only a sub-set of potential risks here.
Conclusions
Using EAST and EAST-BL, this study has addressed substantial gaps in the AI risk literature related to a practical application to an important, real-world socio-technical system. First, this study has described the envisaged functionality of a future AGI system through the representation of task, social, and information networks for a future AGI system tasked with managing the Australian road transport system. Second, this study has identified failures in communication and information transfer among the system’s tasks and actors to form a comprehensive risks analysis of an AGI system task with managing a road transport system. This study has implications for AGI research, design, and development through the identification of emergent and negative unanticipated consequences that will require appropriate management to ensure safe and ethical AGI implementation. Further, this study has also demonstrated the utility of HFE theory and methods for formally considering risks associated with the design, implementation, and operation of powerful future technologies. Finally, this study moves beyond much other AGI risk research which is general in nature and focused on the attributes of the AGI system itself, by analysing in detail the emergent risks of AGI deployment in a real-world sociotechnical system.
Disclosure statement
No potential conflict of interest was reported by the author(s).
Additional information
Funding
References
- Abduljabbar, R., H. Dia, S. Liyanage, and S. A. Bagloee. 2019. “Applications of Artificial Intelligence in Transport: An Overview.” Sustainability 11 (1): 189. doi:10.3390/su11010189.
- Banks, V.A., N.A. Stanton, G. Burnett, and S. Hermawati. 2018. “Distributed Cognition on the Road: Using EAST to Explore Future Road Transportation Systems.” Applied Ergonomics 68: 258–266. doi:10.1016/j.apergo.2017.11.013.
- Barrett, A. M., and S. D. Baum. 2017. “A Model of Pathways to Artificial Superintelligence Catastrophe for Risk and Decision Analysis.” Journal of Experimental & Theoretical Artificial Intelligence 29 (2): 397–414. doi:10.1080/0952813X.2016.1186228.
- Baum, S. 2017. “A Survey of Artificial General Intelligence Projects for Ethics, Risk, and Policy.”. Global Catastrophic Risk Institute Working Paper, 17–11.
- Baum, S. D., B. Goertzel, and T. Goertzel. 2011. “How Long Until Human-Level AI? Results from an Expert Assessment.” Technological Forecasting and Social Change 78 (1): 185–195. doi:10.1016/j.techfore.2010.09.006.
- Bostrom, N. 2003. Superintelligence: Paths, Dangers, Strategies. UK: Oxford University Press.
- Bostrom, N. 2012. “The Superintelligent Will: Motivation and Instrumental Rationality in Advanced Artificial Agents.” Minds and Machines 22 (2): 71–85. doi:10.1007/s11023-012-9281-3.
- Bostrom, N. 2014. Superintelligence: Paths, Dangers, Strategies: UK: Oxford University Press Inc.
- Bostrom, N., and E. Yudkowsky. 2018. “The Ethics of Artificial Intelligence.” In Artificial Intelligence Safety and Security, 57–69. Chapman and Hall: CRC.
- Bubeck, S., V. Chandrasekaran, R. Eldan, J. Gehrke, E. Horvitz, E. Kamar, … Y. Zhang. 2023. “Sparks of Artificial General Intelligence: Early Experiments with Gpt-4.” arXiv preprint arXiv:2303.12712.
- Bura, H., N. Lin, N. Kumar, S. Malekar, S. Nagaraj, and K. Liu. 2018. “An Edge Based Smart Parking Solution Using Camera Networks and Deep Learning.” In 2018 IEEE International Conference on Cognitive Computing (ICCC), San Francisco. doi:10.1109/ICCC.2018.00010.
- Dallat, C., P. M. Salmon, and N. Goode. 2018. “Identifying Risks and Emergent Risks across Sociotechnical Systems: The NETworked Hazard Analysis and Risk Management System (NET-HARMS).” Theoretical Issues in Ergonomics Science 19 (4): 456–482. doi:10.1080/1463922X.2017.1381197.
- Davis, E., and G. Marcus. 2015. “Commonsense Reasoning and Commonsense Knowledge in Artificial Intelligence.” Communications of the ACM 58 (9): 92–103. doi:10.1145/2701413.
- de Zwart, F. 2015. “Unintended but Not Unanticipated Consequences.” Theory and Society 44 (3): 283–297. doi:10.1007/s11186-015-9247-6.
- Dekker, S. W., and D. D. Woods. 1999. “To Intervene or Not to Intervene: The Dilemma of Management by Exception.” Cognition, Technology & Work 1 (2): 86–96. doi:10.1007/s101110050035.
- Duffy, V. G., S. J. Landry, J. D. Lee, and N. A. Stanton. 2023. Human-Automation Interaction: Transportation. Berlin: Springer Verlag.
- Firt, E. 2020. “The Missing G.” AI & SOCIETY 35 (4): 995–1007. doi:10.1007/s00146-020-00942-y.
- Furlan, Andrea D., Tara Kajaks, Margaret Tiong, Martin Lavallière, Jennifer L. Campos, Jessica Babineau, Shabnam Haghzare, Tracey Ma, and Brenda Vrkljan. 2020. “Advanced Vehicle Technologies and Road Safety: A Scoping Review of the Evidence.” Accident; Analysis and Prevention 147: 105741. doi:10.1016/j.aap.2020.105741.
- Glikson, E., and A. W. Woolley. 2020. “Human Trust in Artificial Intelligence: Review of Empirical Research.” Academy of Management Annals 14 (2): 627–660. doi:10.5465/annals.2018.0057.
- Goertzel, B. 2014. “Artificial General Intelligence: concept, State of the Art, and Future Prospects.” Journal of Artificial General Intelligence 5 (1): 1–48. doi:10.2478/jagi-2014-0001.
- Goertzel, B., and C. Pennachin. 2007. Artificial General Intelligence. Springer.
- Goertzel, B., and J. Pitt. 2014. “Nine Ways to Bias Open‐Source Artificial General Intelligence toward Friendliness.” In Intelligence Unbound: Future of Uploaded Machine Minds, 61–89. John Wiley & Sons.
- Goertzel, B., C. Pennachin, and N. Geisweiller. 2014. “Advanced Self-Modification: A Possible Path to Superhuman AGI.” In Engineering General Intelligence, Part 1: A Path to Advanced AGI via Embodied Learning Cognitive Synergy, 365–373. Springer.
- Hamidi, H., and A. Kamankesh. 2018. “An Approach to Intelligent Traffic Management System Using a Multi-Agent System.” International Journal of Intelligent Transportation Systems Research 16 (2): 112–124. doi:10.1007/s13177-017-0142-6.
- Hancock, P. A. 2019. “Some Pitfalls in the Promises of Automated and Autonomous Vehicles.” Ergonomics 62 (4): 479–495. doi:10.1080/00140139.2018.1498136.
- Hancock, P. A. 2022. “Avoiding Adverse Autonomous Agent Actions.” Human–Computer Interaction 37 (3): 211–236. doi:10.1080/07370024.2021.1970556.
- Lake, B. M., T. D. Ullman, J. B. Tenenbaum, and S. J. Gershman. 2017. “Building Machines That Learn and Think like People.” The Behavioral and Brain Sciences 40: e253. doi:10.1017/S0140525X16001837.
- Lane, B.R., P.M. Salmon, A. Cherney, D. Lacey, and N.A. Stanton. 2019. “Using the Event Analysis of Systemic Teamwork (EAST) Broken-Links Approach to Understand Vulnerabilities to Disruption in a Darknet Market.” Ergonomics 62 (9): 1134–1149. doi:10.1080/00140139.2019.1621392.
- Lazer, David M J., Matthew A. Baum, Yochai Benkler, Adam J. Berinsky, Kelly M. Greenhill, Filippo Menczer, Miriam J. Metzger, Brendan Nyhan, Gordon Pennycook, David Rothschild, Michael Schudson, Steven A. Sloman, Cass R. Sunstein, Emily A. Thorson, Duncan J. Watts, and Jonathan L. Zittrain. 2018. “The Science of Fake News.” Science 359 (6380): 1094–1096. doi:10.1126/science.aao2998.
- Legg, S., and M. Hutter. 2006. “A Formal Measure of Machine Intelligence.” In Proceedings of 15th Annual Machine Learning Conference of Belgium and The Netherlands (Benelearn’06).
- Leveson, N. 2004. “A New Accident Model for Engineering Safer Systems.” Safety Science 42 (4): 237–270. doi:10.1016/S0925-7535(03)00047-X.
- Liu, N., A. Nikitas, and S. Parkinson. 2020. “Exploring Expert Perceptions about the Cyber Security and Privacy of Connected and Autonomous Vehicles: A Thematic Analysis Approach.” Transportation Research Part F: Traffic Psychology and Behaviour 75, 66–86.
- Liu, Z., Y. Bi, and P. Liu. 2022. “An Evidence Theory-Based Large Group FMEA Framework Incorporating Bounded Confidence and Its Application in Supercritical Water Gasification System.” Applied Soft Computing 129: 109580. doi:10.1016/j.asoc.2022.109580.
- Martinho, A., N. Herber, M. Kroesen, and C. Chorus. 2021. “Ethical Issues in Focus by the Autonomous Vehicles Industry.” Transport Reviews 41 (5): 556–577. doi:10.1080/01441647.2020.1862355.
- McLean, S., G. J. Read, J. Thompson, P. A. Hancock, and P. M. Salmon. 2022. “Who is in Control? Managerial Artificial General Intelligence (MAGI) for Football.” Soccer & Society 23 (1): 104–109. doi:10.1080/14660970.2021.1956477.
- McLean, S., G. J. Read, K. Ramsay, L. Hogarth, and B. Kean. 2021. “Designing Success: Applying Cognitive Work Analysis to Optimise a Para Sport System.” Applied Ergonomics 93: 103369. doi:10.1016/j.apergo.2021.103369.
- Merton, R. K. 1936. “The Unanticipated Consequences of Purposive Social Action.” American Sociological Review 1 (6): 894–904. doi:10.2307/2084615.
- Miller, M. J., and K. M. Feigh. 2019. “Addressing the Envisioned World Problem: A Case Study in Human Spaceflight Operations.” Design Science 5: e3. doi:10.1017/dsj.2019.2.
- Müller, V. C., and N. Bostrom. 2016. “Future Progress in Artificial Intelligence: A Survey of Expert Opinion.” Fundamental Issues of Artificial Intelligence, 555–572. Springer.
- Murino, T., M. D. Nardo, D. Pollastro, N. Berx, A. D. Francia, W. Decré, J. Philips, and L. Pintelon. 2023. “Exploring a Cobot Risk Assessment Approach Combining FMEA and PRAT.” Quality and Reliability Engineering International 39 (3): 706–731. doi:10.1002/qre.3252.
- OpenAI 2023. GTP-4 System Card. OpenAI. https://openai.com/
- Pöllänen, E., G. J. Read, B. R. Lane, J. Thompson, and P. M. Salmon. 2020. “Who is to Blame for Crashes Involving Autonomous Vehicles? Exploring Blame Attribution across the Road Transport System.” Ergonomics 63 (5): 525–537. doi:10.1080/00140139.2020.1744064.
- Read, G. J., A. O'Brien, N. A. Stanton, and P. M. Salmon. 2022. “Learning Lessons for Automated Vehicle Design: Using Systems Thinking to Analyse and Compare Automation-Related Accidents across Transport Domains.” Safety Science 153: 105822. doi:10.1016/j.ssci.2022.105822.
- Read, G. J. M., S. McLean, J. Thompson, N. A. Stanton, C. Baber, T. Carden, and P. M. Salmon. 2023. “Managing the Risks Associated with Technological Disruption in the Road Transport System: A Control Structure Modelling Approach.” Ergonomics 1–17. doi:10.1080/00140139.2023.2226850.
- Salmon, P. M., and G. J. Read. 2019. “Many Model Thinking in Systems Ergonomics: A Case Study in Road Safety.” Ergonomics 62 (5): 612–628. doi:10.1080/00140139.2018.1550214.
- Salmon, P. M., and K. L. Plant. 2022. “Distributed Situation Awareness: From Awareness in Individuals and Teams to the Awareness of Technologies, Sociotechnical Systems, and Societies.” Applied Ergonomics 98: 103599. doi:10.1016/j.apergo.2021.103599.
- Salmon, P. M., G. J. M. Read, G. H. Walker, M. G. Lenné, and N. A. Stanton. 2018. Distributed Situation Awareness in Road Transport: theory, Measurement, and Application to Intersection Design. Routledge.
- Salmon, P. M., N. A. Stanton, and D. P. Jenkins. 2009. Distributed Situation Awareness: Theory, Measurement and Application to Teamwork. Ashgate Publishing, Ltd.
- Salmon, P. M., N. A. Stanton, G. H. Walker, A. Hulme, N. Goode, J. Thompson, and G. J. Read. 2022. Handbook of Systems Thinking Methods: CRC Press.
- Salmon, P. M., T. Carden, and P. A. Hancock. 2021. “Putting the Humanity into Inhuman Systems: How Human Factors and Ergonomics Can Be Used to Manage the Risks Associated with Artificial General Intelligence.” Human Factors and Ergonomics in Manufacturing & Service Industries 31 (2): 223–236. doi:10.1002/hfm.20883.
- Salmon, Paul M., Chris Baber, Catherine Burns, Tony Carden, Nancy Cooke, Missy Cummings, Peter Hancock, Scott McLean, Gemma J. M. Read, and Neville A. Stanton. 2023. “Managing the Risks of Artificial General Intelligence: A Human Factors and Ergonomics Perspective.” Human Factors and Ergonomics in Manufacturing & Service Industries 33 (5): 366–378. doi:10.1002/hfm.20996.
- Salmon, Paul M., Gemma J M. Read, Vanessa Beanland, Jason Thompson, Ashleigh J. Filtness, Adam Hulme, Rod McClure, and Ian Johnston. 2019. “Bad Behaviour or Societal Failure? Perceptions of the Factors Contributing to Drivers’ Engagement in the Fatal Five Driving Behaviours.” Applied Ergonomics 74: 162–171. doi:10.1016/j.apergo.2018.08.008.
- Salmon, P. M., M. G. Lenne, G. H. Walker, N. A. Stanton, and A. Filtness. 2014. “Using the Event Analysis of Systemic Teamwork (EAST) to Explore Conflicts between Different Road User Groups When Making Right Hand Turns at Urban Intersections.” Ergonomics 57 (11): 1628–1642. doi:10.1080/00140139.2014.945491.
- Simsekler, M. C. E., G. K. Kaya, J. R. Ward, and P. J. Clarkson. 2019. “Evaluating Inputs of Failure Modes and Effects Analysis in Identifying Patient Safety Risks.” International Journal of Health Care Quality Assurance 32 (1): 191–207. doi:10.1108/IJHCQA-12-2017-0233.
- Stanton, N. A. 2014. “Representing Distributed Cognition in Complex Systems: How a Submarine Returns to Periscope Depth.” Ergonomics 57 (3): 403–418. doi:10.1080/00140139.2013.772244.
- Stanton, N. A. 2021. “Advances in Human Aspects of Transportation.” In Proceedings of the AHFE 2021 Virtual Conference on Human Aspects of Transportation, Vol. 270, July 25-29, Springer Verlag, Berlin.
- Stanton, N. A., and C. Harvey. 2017. “Beyond Human Error Taxonomies in Assessment of Risk in Sociotechnical Systems: A New Paradigm with the EAST ‘Broken-Links’ Approach.” Ergonomics 60 (2): 221–233. doi:10.1080/00140139.2016.1232841.
- Stanton, N. A., K. M. A. Revell, and P. Langdon. 2021. Designing Interaction and Interfaces for Automated Vehicles: User-Centred Ecological Interface Design and Testing. Boca Raton: CRC Press.
- Stanton, N. A., R. Stewart, D. Harris, R. J. Houghton, C. Baber, R. McMaster, P. Salmon, G. Hoyle, G. Walker, M. S. Young, M. Linsell, R. Dymott, and D. Green. 2006. “Distributed Situation Awareness in Dynamic Systems: theoretical Development and Application of an Ergonomics Methodology.” Ergonomics 49 (12-13): 1288–1311. doi:10.1080/00140130600612762.
- Stanton, N., P. Salmon, and G. Walker. 2018. Systems Thinking in Practice: applications of the Event Analysis of Systemic Teamwork Method: CRC Press.
- Tegmark, M. 2018. Life 3.0: Being Human in the Age of Artificial Intelligence. Penguin Books Limited.
- Thompson, J., G. J. Read, J. S. Wijnands, and P. M. Salmon. 2020. “The Perils of Perfect Performance; considering the Effects of Introducing Autonomous Vehicles on Rates of Car vs Cyclist Conflict.” Ergonomics 63 (8): 981–996. doi:10.1080/00140139.2020.1739326.
- Torbaghan, M. E., M. Sasidharan, L. Reardon, and L. C. Muchanga-Hvelplund. 2022. “Understanding the Potential of Emerging Digital Technologies for Improving Road Safety.” Accident; Analysis and Prevention 166: 106543. doi:10.1016/j.aap.2021.106543.
- Wooldridge, A. R., J. Morgan, W. A. Ramadhani, K. Hanson, E. Vazquez-Melendez, H. Kendhari, N. Shaikh, T. Riech, M. Mischler, S. Krzyzaniak, G. Barton, K. T. Formella, Z. R. Abbott, J. N. Farmer, R. Ebert-Allen, and T. Croland. 2022. “Interactions in Sociotechnical Systems: Achieving Balance in the Use of an Augmented Reality Mobile Application.” Human Factors 0 (0): 187208221093830. doi:10.1177/00187208221093830.
- World Health Organisation. 2018. https://www.who.int/publications/i/item/9789241565684
- Young, M. S., and N. A. Stanton. 2023. Driving Automation: A Human Factors Perspective. Boca Raton: CRC Press.