Abstract
Extensive evaluations exist concerning the linkage between objective task demands and subsequent effects on user performance. However, the human user also experiences a range of emotions related to external task demands. Problematically, little is known about the associations between emotional valence, and arousal associated with the task demand–performance axis. In this paper, we advance a theoretical model concerning such interactive influences using three dimensions: (1) emotional valence, (2) arousal, and (3) task demand. The model evaluates the impact of these dimensions on user performance. It also identifies critical emotional user states, particularly those resulting in negative performance effects, as well as non-critical emotional states that can positively impact performance. Finally, we discuss the implications for affect-adaptive systems that can mitigate the impact of critical emotional states while leveraging the benefits of non-critical ones.
Practitioner summary
To effectively model performance and prevent errors in safety-critical human-machine systems, it is crucial to consider user states of emotional valence, arousal, and the current task demand. The proposed model enables the classification of critical and non-critical states within affect-adaptive systems. States characterised by negative valence, high arousal, and overload should be avoided to foster high performance, especially in safety-critical environments. Additionally, the present work offers recommendations for preserving and restoring non-critical states to ensure optimal performance and provides implications for training.
Introduction
With the expansion of automation in human–machine systems, productivity has increased commensurately. This trend has been especially evident in recent decades (Acemoglu and Restrepo Citation2019; Autor Citation2015; Hancock Citation2014). In highly automated safety-critical systems, such as advanced aircraft and nuclear power plants, the task requirements imposed on human operators are rapidly evolving. Users are now tasked with monitoring the automation for increasingly rare and exceptional events (Hancock Citation2023). This is a role in which human capacity proves rather limited (Hancock Citation2014; Kaber and Endsley Citation1997; Mackworth Citation1945, Citation1950). In these situations, operators are expected to take corrective action whenever and wherever the automated system functionality fails or exhibits only minor shortfalls. In aviation, for instance, the pilot must assume manual control if the autopilot malfunctions. This transition can be particularly challenging, as the actions of the automated system, or the reasoning behind those actions, may not be immediately apparent or fully understood by the flight crew. Bainbridge (Citation1983) refers to these and allied phenomena as the “ironies of automation” (p. 1). Increasing levels of automation in human–machine systems can thus have detrimental effects on overall system performance. Hence, its advantages must be carefully weighed against its drawbacks (Hancock Citation2014). These drawbacks are especially associated with systems that employ only fixed levels of automation (Parasuraman and Riley Citation1997).
Unlike fixed automated systems, adaptive human–machine systems assess and adjust the level of automation needed, thus avoiding the pitfalls of static automation (Hancock et al. Citation1985). Adaptive systems dynamically adapt task allocations or alter interface characteristics to meet the operator’s evolving needs without requiring explicit user instruction (Feigh et al. Citation2012). Adjusting the level of task demand is one of the most common and well-studied methods of adaptation known to improve user performance. For instance, Whitlow et al. (Citation2005) achieved a 155% increase in performance by adapting task demands within a command and control paradigm. Similarly, Parasuraman et al. (Citation1996) demonstrated performance enhancement through task demand adaptation in a multitask flight simulation. They temporarily increased task demands by transferring tasks from automation to the user. After tasks were returned to the automation, user performance surpassed pre-adjustment levels. Prinzel et al. (Citation2003) also investigated the impact of changing task demands on user performance within an adaptive system. The system alternated between manual and automatic task modes depending on the participant’s level of engagement, as measured by electroencephalographic (EEG) signals. Participants exhibited improved performance in response to the adaptation. These studies demonstrate how adapting task demands is an effective strategy for optimising user performance in automated systems.
Adaptation can arise from a variety of factors within the system. For example, adaptive processes can use objective task properties, environmental characteristics, the user’s performance, fatigue, and/or stress levels (Marras and Hancock Citation2014; Parasuraman and Hancock Citation2008). The user’s mental state significantly impacts performance, and unfavourable states can lead to errors and intentional violations of rules and regulations (Wiegmann and Shappell Citation2001). The primary aim of an adaptive system is to manage fluctuations in user states, such as attention or fatigue, to minimise adverse outcomes. Additionally, adaptive systems can also seek to maximise positive emotional states to fully optimise the joint human-machine system (Hancock et al. Citation2005; Parasuraman and Hancock Citation2008).
The operator’s emotional state can also influence performance within a human–machine system (Cai and Lin Citation2011). Emotions impact the operators’ attention, motivation, and response behavior (Gaillard Citation2008). Moreover, emotional state can consume cognitive resources, thereby reducing the resources available for task processing (Plass and Kalyuga Citation2019). For example, anger in road rage situations can result in risky driving behavior (Li et al. Citation2019; Sanghavi et al. Citation2023). Similarly, both sadness and happiness can impair driving efficiency (Zimasa et al. Citation2017). The impact of emotional states on performance is evident in other safety-critical domains, such as in flying tasks (Dai et al. Citation2022; Li and Lajoie Citation2021). Therefore, adaptive systems should incorporate real-time emotional state monitoring to detect these critical emotional influences. For instance, physiological data can be analysed and inform future system responses. When conceptualising and developing an affect-adaptive system—where affect is synonymous with emotion (Meyer et al. Citation2001)—a fundamental question arises: Which emotional states are critical and which are non-critical for optimising performance? To our knowledge, there is currently no theoretical model capable of delineating these pertinent states, which impedes the development of an effective affect-adaptive system. This manuscript seeks to address this research gap.
The present work introduces a theoretical framework that classifies vital as well as non-vital emotional states and arousal levels to facilitate optimal performance. Furthermore, existing research suggests that task demand interacts with both arousal and valence dimensions of emotion on behavioral and neurological levels (see Delaney-Busch et al. Citation2016; Zsidó et al. Citation2022). Therefore, integrating task demand into the framework sheds further light on the interplay between arousal, valence, and task performance.
The P-EAT model
Three dimensions are considered relevant for defining critical emotional user states for performance (P): Emotional Valence (E), Arousal (A), and Task Demand (T). Hence, our construct is referred to as the P-EAT model.
Emotional valence and arousal
In an adaptive human–machine system, accounting for emotional states requires quantifiable measures for emotions. Numerous suitable measurement methods are grounded in dimensional emotion models (see, e.g., Bradley and Lang Citation1994; Betella and Verschure Citation2016; Cai and Lin Citation2011; Toet and van Erp Citation2019). One of the most frequently used dimensional models (Yang et al. Citation2019) is the Circumplex Model of Affect (Russell Citation1980, see ). This model assumes that all emotional states arise from two fundamental neurophysiological systems: valence (i.e., a continuum from pleasure to displeasure) and arousal (i.e., a continuum between activation and deactivation). The orbitofrontal cortex is one of the neural correlates for valence, while the amygdala functions as a neural correlate for arousal (Lewis et al. Citation2007). Consequently, emotions can be characterised by varying degrees of valence and arousal. Being afraid, for example, arises from strong activation of the neural systems associated with both arousal and negative valence. Conversely, happiness results from moderate activation of the arousal neural systems and strong activation of positive valence.
Figure 1. Circumplex model of affect adapted from Russell (Citation1980).
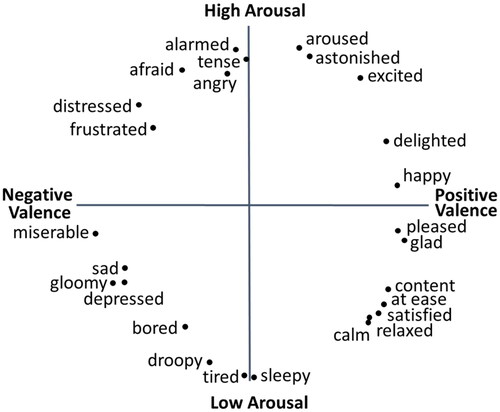
Task demand
The third dimension of our model is task demand. Despite extensive research on the influence of task demand on performance, to our knowledge, there is a lack of concrete definitions for this construct. Moreover, inconsistencies in the use of related constructs, such as task load, lead to further confusion (Staal Citation2004). Wickens (Citation2002) describes task demand as the resources demanded by a task necessary to achieve a given level of performance. However, we consider task demand strictly as an external and objectively quantifiable influence. Similarly, Hancock et al. (Citation1995) argued that to make assertions about an individual’s behavior under differing demand conditions, the absolute level of task demand should be changed between conditions, allowing for quantitative comparison of performance between trials. Following this approach, we use task demand as an objective measure encompassing characteristics such as, but not limited to, task format, task complexity, use of multimedia, time pressure, and pacing of instruction (Paas et al. Citation2003).
Although arousal is strongly influenced by task demand (Hancock and Warm Citation1989), it is necessary to separate these two dimensions. For example, arousal can be particularly high when performing a task for the first time, even though objective task demand is low (Belyusar et al. Citation2015). shows our proposed model’s structure with its three dimensions: emotional valence, arousal, and task demand.
User states in the P-EAT model
Different tasks and conditions can induce various states within the model. provides examples of these states for all eight quadrants of the cube along with the circumstances that can evoke them.
Table 1. Exemplary user states.
While user states can potentially exist in all eight quadrants of the cube-shaped model, the probability of occurrence may not be equally distributed. Given that task demand significantly influences arousal (Hancock and Warm Citation1989), low task demand might often coincide with states of low arousal, whereas high task demand might be more often associated with states of high arousal. Therefore, we propose a diagonal plane representing the more probable states along the task demand-arousal axis within the model (see ). This diagonal plane has no direct influence on critical or non-critical states; its purpose is simply to illustrate a frequency distribution of user states, aiming to enhance comprehension of the P-EAT model.
In the present work, we examine performance across various emotional user states resulting from combinations derived from the three dimensions: emotional valence, arousal, and task demand.
Performance in the P-EAT model
To identify the critical and non-critical states for high performance in the model, we reviewed the relevant literature. First, we evaluated theoretical models that connect the three individual axes to performance. Second, we examined empirical findings from other research groups. We focused primarily on task performance as opposed to subjective reports of performance, as these latter measures can vary within an individual and across different situations (Hancock and Matthews Citation2019).
Theoretical models
Emotion and cognition are intricately linked, and emotional valence can influence performance through various mechanisms, including information processing, attention, and motivation (Gaillard Citation2008). The affect-as-information hypothesis (Clore et al. Citation2001) posits that emotions serve as sources of information about objects, tasks, and decision alternatives, thereby influencing cognitive functions such as memory, judgement, decision-making, and information processing (Clore et al. Citation2001). According to this hypothesis, negative emotions narrow attentional focus and lead to local, item-specific, and stimulus-based information processing, while positive emotions encourage a broad attentional focus with associated top-down and theory-based processing (Clore et al. Citation2001).
Following the affect-as-information hypothesis, an individual’s valence state may either positively or negatively performance, depending on the specific demands of the task. Furthermore, positive and negative emotions may consume cognitive resources by requiring the processing of task-irrelevant information (Pekrun and Linnenbrink-Garcia Citation2012). Consistent with this assumption, Weiss and Cropanzano (Citation1996) argue that all emotions, regardless of their valence, could lead to performance decrements in work-related tasks, as they require resources to manage and may induce job-incompatible behaviors. Negatively-valenced emotions may require greater regulative control due to their unpleasant character, further straining cognitive resources (Hofstee et al. Citation2021). Finally, emotions can influence motivation, thereby also altering performance (Pekrun and Linnenbrink-Garcia Citation2012). According to these theoretical models, performance levels are lowest at the extreme ends of the valence axis, especially in cases of negative valence, and highest around its centre with a possible tendency towards more positive valence.
A classical approach to describe the relationship between arousal and performance is the Yerkes-Dodson law, which depicts an inverted U-shaped relationship. At extremely high and low levels of arousal, performance tends to be poor, while at moderate levels of arousal, it is at its peak (Yerkes and Dodson Citation1908). Despite being extensively cited, this law has been heavily criticised because of methodological limitations in its development (Hancock and Ganey Citation2003). Although the Yerkes-Dodson law is often used to explain human performance in stressful conditions, it was originally created to model animal learning under states of electronic shock (see Hancock and Ganey Citation2003). In response to these limitations, Hancock and Warm (Citation1989) developed a more accurate and sophisticated model that provides an alternative to the inverted U-shaped pattern. The Hancock and Warm (Citation1989) model is consistent with empirically observed physiological, psychological, and behavioral effects. Consequently, we employ this framework as a foundation for our proposed model despite Hancock and Warm’s (1989) focus on stress rather than arousal.
illustrates an operator’s physiological and psychological adaptive capability as a function of stress levels (Hancock and Warm Citation1989). Input stress, represented on the x-axis, ranges from states of underload (hypostress) to overload (hyperstress). Adaptability is represented on the y-axis. The dashed lines depict psychological adaptive capability, for instance, when facing external stressors like noise. Solid lines represent physiological adaptive capability, such as when coping with temperature changes. The resulting output is reflected in behavioral changes, such as performance efficiency.
Figure 4. Functional adaptability expressed as a function of stress. Illustration from Hancock and Warm (Citation1989, p.21).
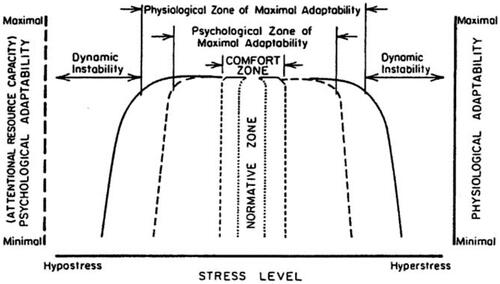
Furthermore, indicates the zone of maximal adaptability for both physiological and psychological stressors. While adaptive capability can manage minor levels of hypo- and hyperstress, higher levels eventually affect the output. However, medium stress levels result in a comfort region that requires minimal compensatory action to maintain performance efficiency. According to the Hancock and Warm (Citation1989) model, performance in P-EAT would be lowest at the extreme ends of the arousal axis and highest around the centre.
Task demand significantly influences task performance (Epps Citation2018). Unsurprisingly, increasing task demand strains the user’s limited cognitive resources which, in turn, leads to a decrease in performance (e.g., Hancock and Caird Citation1993). However, even tasks with low task demand can result in performance decrements, such as when the task is very monotonous and unengaging (Epps Citation2018). Both extremes on the task demand axis, overload, and underload, are associated with lower performance compared to moderate levels of task demand.
From a theoretical standpoint, we hypothesise that non-critical states lie within a comfort zone at the centre of the model, while critical states are in the outer edges. However, Yoon (Citation2009) argues that an individual’s abilities may influence the relationship between task demand and performance, suggesting that skill development can enhance performance. In P-EAT, we anticipate that the position of the comfort zone will shift backward along the task demand axis as the operator’s skills improve.
Empirical evidence
After establishing the location of critical states within our three-dimensional model on a theoretical basis, we reviewed existing empirical evidence. We searched for keywords such as valence, emotion, affect, feeling, mood, sentiment, stress, arousal, activation, task load, task difficulty, task complexity, task demand, and performance across many databases (PsycINFO, PsycArticles, ACM Digital Library, IEEE, Science Direct, and ProQuest Dissertations and Theses) and Google Scholar. In the present paper, we discuss studies that have investigated at least two of the three dimensions (see ).
Table 2. Results of literature search on the effects on performance.
For the emotional valence dimension, we identified five studies (Cai and Lin Citation2011; Gupta et al. Citation2016; Jeon et al. Citation2014; Pêcher et al. Citation2009; Stickel et al. Citation2009) supporting our theory-based assumption that states of positive and negative valence impair performance, as all intense emotions impose additional demands on cognitive resources (Pekrun and Linnenbrink-Garcia Citation2012). Di Stasi et al. (Citation2022) and Abdu, Shinar, and Meiran (Citation2012) demonstrated that eliciting negative valence as opposed to neutral valence leads to a performance decrement. However, these studies did not investigate the impact of positive valence. All other studies that used valence reported improved performance with positive valence as opposed to negative valence, one limitation being that valence was only considered as a binary dimension (positive vs. negative). In order to provide evidence for the suggested comfort zone, a ternary dimension (positive vs. neutral vs. negative) would be necessary. Overall, this research suggests that eliciting positive valence and avoiding negative valence is an effective means of maintaining optimal performance.
Research on the arousal and task demand dimensions has produced mixed findings. Several studies have demonstrated that moderate arousal is associated with high performance (Cai and Lin Citation2011; Stickel et al. Citation2009), aligning with the Hancock and Warm (Citation1989) model. It is worth noting that these studies investigated arousal as a continuum rather than a dichotomy. Li and Lajoie (Citation2021) found that when task demand was low, high levels of arousal improved performance. However, this effect was not found when task demand was high. In support of our hypothesised model, the low-demand condition induced overall low arousal. Thus, proportionally increasing arousal improved performance. Although Bargh and Cohen (Citation1978) failed to find a relationship between arousal and performance under conditions of low task demand, they did find that high demand was associated with impaired performance, providing further support for the Hancock and Warm model (Citation1989) and our proposed model.
In general, research suggests that high task demand is associated with impaired performance. Theoretically, low task demand should also impair performance (Hancock and Warm Citation1989). However, this effect has not been consistently observed in the literature presented in . This may be due to an underload-induced performance decrement only occurring during monotonous tasks (Loukidou et al. Citation2009). Emotion research has yet to employ this paradigm. Also, a longer task duration might be necessary to observe a performance decrement related to low task demands (Szalma and Teo Citation2012). Finally, high task demands could be associated with both high arousal and low performance due to a greater number or complexity of tasks.
Critical and non-critical states in the P-EAT model
Drawing from the previously discussed theoretical models and the empirical evidence, we established a comfort zone of non-critical states for high performance within the P-EAT model. Hertzberg (Citation1972) and Branton (Citation1969) suggest that comfort can be defined as the absence of discomfort. States lying outside this comfort zone are considered critical, potentially leading to performance decrements. The cubic shape with rounded edges was derived from the comfort zone in the Hancock and Warm (Citation1989) model.
Prior theoretical models such as Weiss and Cropanzano (Citation1996), Hancock and Warm (Citation1989), and Hancock and Caird (Citation1993) suggest a central comfort zone encompassing all three dimensions. This would place our comfort zone in the centre of the cube. However, empirical evidence, as presented in , indicates a slightly shifted comforted zone towards more positive valence, lower arousal, and lower task demand (see grey lines in ).
Practitioner takeaways
Practitioners can derive three main takeaways from the proposed P-EAT Model:
Definition of critical and non-critical states in affect-adaptive systems.
Strategies for preserving and restoring non-critical states and for avoiding critical states to maintain high performance.
Implications for training.
1. Definition of critical and non-critical states in affect-adaptive systems
The P-EAT model provides a foundation for delineating critical and non-critical emotional states for optimal performance within human-machine systems. With this foundation, user-state diagnosis can be developed to detect emotional states and evaluate them considering task requirements within the human-machine system. Real-time assessment of critical and non-critical emotional states, for instance, using physiological data, enables adaptive systems to execute appropriate and timely adaptation strategies. In such a system, there are three stages of data processing within a feedback loop: collection, analysis, and translation (Fairclough Citation2017).
Data are typically collected by physiological sensors such as heart rate monitors (Fairclough Citation2017). However, less intrusive methods such as facial expressions or pupil diameter are more suitable for safety-critical systems (Schmitz-Hübsch and Fuchs Citation2022). Whenever possible, data analysis should also be conducted in real-time and involve cleaning the data to remove artefacts (Fairclough Citation2017). Translation involves converting physiological units of measurement into computer commands for execution within the human-computer interface (Fairclough Citation2017). For example, positive emotions could be induced via positive feedback or task demand reduced using a higher level of automation. The effects of the translation are then re-evaluated, resulting in a feedback loop (Fairclough Citation2017).
2. Preservation and restoration of non-critical states for high performance
With the P-EAT model and the proposed comfort zone, critical states can be identified and avoided to enhance performance. Adaptation strategies can be tailored for each of the three dimensions. For instance, if valence is especially negative, adaptations could be implemented to shift valence towards the positive direction. This could be achieved with mood-enhancing lighting (Pail et al. Citation2011), color (Plass et al. Citation2014), or shapes (Berry and McArthur Citation1985). Additionally, an affect-adaptive system could improve valence by playing music that the user enjoys (Kushnir et al. Citation2012) or by providing hot beverages (Quinlan et al., Citation1997).
The affect-adaptive system can also reduce elevated arousal. In this case, it is crucial to distinguish whether the heightened arousal is caused by high task demand or other factors, so the system can either adjust the task demand or address other sources of concern, such as by playing soothing noises like ocean sounds (Cheng and Tsai Citation2016) tranquil music (Nilsson et al. Citation2005), or guided breathing exercises (Dhungel et al. Citation2008). Additional methods to decrease arousal include providing an anti-stress ball for kneading (Srivarsan et al. Citation2021), if the task can be performed with one hand, or equipping the operator’s seat with technologies that promote relaxation and mitigate discomfort, such as massage programs or a heating or cooling function.
Adaptation can also involve adjustments to task demand. Feigh et al. (Citation2012) created a taxonomy of adaptation strategies, and proposed four types of modification:
Modification of function allocation refers to who performs the task. For instance, tasks originally completed by the user can be transferred to the system to reduce task demand.
Modification of task scheduling addresses the temporal aspect of task processing. This can involve delaying less urgent tasks to reduce the cognitive load on the user.
Modification of interaction pertains to how information is presented in the user interface.
Modification of content refers to the quality and quantity of presented information. For instance, reducing the salience of non-critical information can help the operator focus on essential information.
Adaptations in an affect-adaptive system could mitigate the negative effects of each dimension individually or in combination given that the dimensions are not completely independent (Cai and Lin Citation2011; Hancock and Warm Citation1989). For instance, strong negative or positive valence can impact arousal levels (Cai and Lin Citation2011). Similarly, adaptation strategies like music could simultaneously affect both dimensions (Lazar and Pearlman-Avnion Citation2014).
In addition to implementing adaptation strategies during the task, the system could employ strategies during breaks. The affect-adaptive system could suggest activities like physical exercise, progressive muscle relaxation, or mindfulness exercises known to have calming effects (Jackson Citation2013; Kibler and Rider Citation1983; Morais et al. Citation2021). In extreme cases, when a user can no longer operate the system due to their emotional state, a replacement operator could be requested.
3. Implications for training
Another objective of an affect-adaptive system is to expand the comfort zone through targeted training (see ). Operators could be trained to manage high task demand, high arousal, and negative valence. This training would involve inducing critical states in simulations of common scenarios, as in Harris et al. (Citation2005). Cruit et al. (Citation2022) offer recommendations for training interventions designed to enhance pilot performance during unexpected events that may involve increased task demand and high arousal. Training for coping with negative valence could follow a similar approach. For example, in pilot training, a flight simulator scenario could introduce a conflict between crew members to induce negative valence.
This training would help users develop strategies and skills to effectively manage critical states. Furthermore, an affect-adaptive system could educate users about emotion regulation strategies and provide insight into their own emotional comfort zone. A deeper understanding would enable users to better comprehend their emotional states and regulate them more effectively (Millgram et al. Citation2023). Furthermore, Prinzel et al. (Citation2001) show that psychophysiological self-regulation in an adaptive system can improve performance. Even if training of emotion regulation itself does not change the comfort zone, the affect-adaptive system could anticipate critical user states, support the user in their self-regulation, and thereby aid them in returning to the comfort zone.
Limitations and future work
There are several limitations of our proposed model that must be considered. First, the model only accounts for the three dimensions of emotional valence, arousal, and task demand. However, there could be mediating factors that influence their impact on performance. For instance, emotional states can affect motivation and consequently influence performance (Linnenbrink-Garcia et al. Citation2016). Furthermore, attention could mediate emotion and performance, as emotional states influence attentional processes (Gasper and Clore Citation2002), which in turn affect performance (Wickens Citation2005). For example, negative emotions can narrow attentional focus, leading to failures in surveillance tasks (Clore et al. Citation2001). Additionally, the empirical evidence we used when developing the model may not be exhaustive and represents a limited snapshot of the empirical data. In particular, many of the studies listed in originate from the simulated driving field. Thus, their applicability to other domains may be limited, especially to those that do not involve safety-critical systems.
Moreover, it remains uncertain whether the P-EAT model is a universal solution or if the comfort zone varies among individuals. Schmitz-Hübsch et al. (Citation2022) and Vine et al. (Citation2015) emphasise interindividual differences in the valence-performance relationship within safety-critical environments, which any affect-adaptive system should take into account. Although the P-EAT framework can provide the basis for defining critical and non-critical emotional states in an adaptive system, practical implementation requires adjustments and refinements to customise the comfort zone for the operator. The model could also be customised based on the specific situation and task requirements, as suggested by Cohen-Lazry et al. (Citation2023).
Finally, implementing adaption strategies may present challenges, as they can cause confusion (Haarmann et al. Citation2009) and increase cognitive workload, thereby reducing performance (Lavie and Meyer Citation2010). When designing an affect-adaptive system, the advantages and disadvantages of each adaptation should be carefully weighed.
Future work should further empirically evaluate the model. For example, studies aiming at the validation of the comfort zone for optimal performance could consider valence as a continuous dimension rather than a binary one. It would also be beneficial to investigate potential interaction effects among the dimensions or explore more complex, nonlinear impacts on performance. Research should also consider which physiological methods are best suited for detecting emotional states (Jacucci et al. Citation2015). Commonly used metrics include heart rate, respiration rate, galvanic skin response, EEG, and electromyography (Egger et al. Citation2019). However, Schmitz-Hübsch and Fuchs (Citation2022) advocate for less intrusive measures such as pupil diameter, facial expressions, and voice recognition for affect-adaptive systems in operational environments.
Summary and conclusion
The present work proposes the P-EAT model, a theoretical framework describing how emotional valence, arousal, and task demand influence performance. Our review of relevant theoretical models and empirical evidence identifies that positive valence, low arousal, and low task demand are optimal for task performance. These findings resulted in a comfort zone for non-critical user states. Building on these insights, we offer methods for developing an affect-adaptive system to preserve and restore beneficial user states and foster efficiency and optimal performance, thereby contributing to safety in high-stakes environments.
Acknowledgments
The authors thank Ron Becker and the research assistants Hanya Irfan, Britney Raymond, Alana Cruz for their contribution to the literature search.
Disclosure statement
No potential conflict of interest was reported by the authors.
Additional information
Funding
References
- Abdu, R., D. Shinar, and N. Meiran. 2012. “Situational (State) Anger and Driving.” Transportation Research Part F: Traffic Psychology and Behaviour 15 (5): 575–580. doi:10.1016/j.trf.2012.05.007.
- Acemoglu, D., and P. Restrepo. 2019. “Automation and New Tasks: How Technology Displaces and Reinstates Labor.” Journal of Economic Perspectives 33 (2): 3–30. doi:10.1257/jep.33.2.3.
- Autor, D. H. 2015. “Why Are There Still so Many Jobs? The History and Future of Workplace Automation.” Journal of Economic Perspectives 29 (3): 3–30. doi:10.1257/jep.29.3.3.
- Bainbridge, L. 1983. “Ironies of Automation.” Automatica 19 (6): 775–779. doi:10.1016/B978-0-08-029348-6.50026-9.
- Bargh, J. A., and J. L. Cohen. 1978. “Mediating Factors in the Arousal-Performance Relationship.” Motivation and Emotion 2 (3): 243–257. doi:10.1007/BF00992589.
- Belyusar, D., B. Mehler, E. Solovey, and B. Reimer. 2015. “Impact of Repeated Exposure to a Multilevel Working Memory Task on Physiological Arousal and Driving Performance.” Transportation Research Record: Journal of the Transportation Research Board 2518 (1): 46–53. doi:10.3141/2518-06.
- Berry, D. S., and L. Z. McArthur. 1985. “Some Components and Consequences of a Babyface.” Journal of Personality and Social Psychology 48 (2): 312–323. doi:10.1037/0022-3514.48.2.312.
- Betella, A., and P. Verschure. 2016. “The Affective Slider: A Digital Self-Assessment Scale for the Measurement of Human Emotions.” PLoS One 11 (2): e0148037. Article e0148037. doi:10.1371/journal.pone.0148037.
- Bradley, M., and P. Lang. 1994. “Measuring Emotion: The Self-Assessment Manikin and the Semantic Differential.” Journal of Behavior Therapy and Experimental Psychiatry 25 (1): 49–59. doi:10.1016/0005-7916(94)90063-9.
- Branton, P. 1969. “Behaviour, Body Mechanics and Discomfort.” Ergonomics 12 (2): 316–327. doi:10.1080/00140136908931055.
- Cai, H., and Y. Lin. 2011. “Modeling of Operators’ Emotion and Task Performance in a Virtual Driving Environment.” International Journal of Human-Computer Studies 69 (9): 571–586. doi:10.1016/j.ijhcs.2011.05.003.
- Cheng, T.‑H, and C.‑G. Tsai. 2016. “Female Listeners’ Autonomic Responses to Dramatic Shifts between Loud and Soft Music/Sound Passages: A Study of Heavy Metal Songs.” Frontiers in Psychology 7: 182. Article 182. doi:10.3389/fpsyg.2016.00182.
- Clore, G. L., K. Gasper, and E. Garvin. 2001. “Affect as Information.”. In Handbook of Affect and Social Cognition, edited by J. P. Forgas, 121–144. Mahwah, NJ: Lawrence Erlbaum.
- Cohen, I., N. den Braber, N. J. J. M. Smets, J. van Diggelen, W. P. Brinkman, and M. A. Neerincx. 2016. “Work Content Influences on Cognitive Task Load, Emotional State and Performance during a Simulated 520-days’ Mars Mission.” Computers in Human Behavior 55: 642–652. doi:10.1016/j.chb.2015.10.011.
- Cohen-Lazry, G., A. Degani, T. Oron-Gilad, and P. A. Hancock. 2023. “Discomfort: An Assessment and a Model.” Theoretical Issues in Ergonomics Science 24 (4): 480–503. doi:10.1080/1463922X.2022.2103201.
- Cruit, J. K., J. A. Kochan, M. Gruber, Y. Diaz, S. Pruchnicki, and C. Reed. 2022. Human-in-the-Loop Method to Test the Effectiveness of Training Pilot Responses to Unexpected Events. Task 4: Training Development Plan. United States. Department of Transportation. Federal Aviation Administration. https://rosap.ntl.bts.gov/view/dot/67401. doi:10.21949/1528626.
- Dai, Jing, Hang Wang, Lin Yang, Xinsheng Cao, Chunchen Wang, Zhijun Gao, Wendong Hu, and Zhihong Wen. 2022. “The Effects of Emotional Trait Factors on Simulated Flight Performance under an Acute Psychological Stress Situation.” International Journal of Occupational Safety and Ergonomics: JOSE 28 (4): 2411–2418. doi:10.1080/10803548.2021.1994750.
- Deffenbacher, J. L., D. M. Deffenbacher, R. S. Lynch, and T. L. Richards. 2003. “Anger, Aggression, and Risky Behavior: A Comparison of High and Low Anger Drivers.” Behaviour Research and Therapy 41 (6): 701–718. doi:10.1016/S0005-7967(02)00046-3.
- Deffenbacher, J. L., R. S. Lynch, E. R. Oetting, and D. A. Yingling. 2001. “Driving Anger: correlates and a Test of State-Trait Theory.” Personality and Individual Differences 31 (8): 1321–1331. doi:10.1016/S0191-8869(00)00226-9.
- Delaney-Busch, N., G. Wilkie, and G. Kuperberg. 2016. “Vivid: How Valence and Arousal Influence Word Processing under Different Task Demands.” Cognitive, Affective & Behavioral Neuroscience 16 (3): 415–432. doi:10.3758/s13415-016-0402-y.
- Dhungel, K. U., V. Malhotra, D. Sarkar, and R. Prajapati. 2008. “Effect of Alternate Nostril Breathing Exercise on Cardiorespiratory Functions.” Nepal Medical College Journal 10 (1): 25–27. doi:10.4103/aam.aam_114_20.
- Di Stasi, L. L., E. Gianfranchi, M. Pérez-García, and C. Diaz-Piedra. 2022. “The Influence of Unpleasant Emotional Arousal on Military Performance: An Experimental Study Using Auditory Stimuli during a Shooting Task.” International Journal of Industrial Ergonomics 89: 103295. doi:10.1016/j.ergon.2022.103295.
- Du, N., F. Zhou, E. M. Pulver, D. M. Tilbury, L. P. Robert, A. K. Pradhan, and X. J. Yang. 2020. “Examining the Effects of Emotional Valence and Arousal on Takeover Performance in Conditionally Automated Driving.” Transportation Research Part C: Emerging Technologies 112: 78–87. doi:10.1016/j.trc.2020.01.006.
- Egger, M., M. Ley, and S. Hanke. 2019. “Emotion Recognition from Physiological Signal Analysis: A Review.” Electronic Notes in Theoretical Computer Science 343: 35–55. doi:10.1016/j.entcs.2019.04.009.
- Epps, J. 2018. “Task Load and Stress.”. In The Wiley Handbook of Human Computer Interaction, edited by K. L. Norman and J. Kirakowski, Vol. 1, 1st ed. Hoboken, NJ: John Wiley & Sons Ltd.
- Fairclough, S. H. 2017. “Physiological Computing and Intelligent Adaptation.”. In Emotions and Affect in Human Factors and Human-Computer Interaction, edited by M. Jeon, 539–556. Cambridge, Massachusetts: Academic Press. doi:10.1016/B978-0-12-801851-4.00020-3.
- Feigh, K. M., M. C. Dorneich, and Caroline C. Hayes. 2012. “Toward a Characterization of Adaptive System.” Human Factors 54 (6): 1008–1024. doi:10.1177/0018720812443983.
- Gabana, D., L. Tokarchuk, E. Hannon, and H. Gunes. 2017. “Effects of Valence and Arousal on Working Memory Performance in Virtual Reality Gaming.”. 2017 Seventh International Conference on Affective Computing and Intelligent Interaction (ACII), 36–41. doi:10.1109/ACII.2017.8273576.
- Gaillard, A. W. K. 2008. “Concentration, Stress and Performance.”. In Performance Under Stress, edited by P. A. Hancock and J. L. Szalma, 59–76. Hampshire, UK: Ashgate Publishing.
- Gasper, K., and G. L. Clore. 2002. “Attending to the Big Picture: Mood and Global versus Local Processing of Visual Information.” Psychological Science 13 (1): 34–40. doi:10.1111/1467-9280.00406.
- Gupta, R., Y.‑J. Hur, and N. Lavie. 2016. “Distracted by Pleasure: Effects of Positive versus Negative Valence on Emotional Capture under Load.” Emotion (Washington, D.C.) 16 (3): 328–337. doi:10.1037/emo0000112.
- Haarmann, A., W. Boucsein, and F. Schaefer. 2009. “Combining Electrodermal Responses and Cardiovascular Measures for Probing Adaptive Automation during Simulated Flight.” Applied Ergonomics 40 (6): 1026–1040. doi:10.1016/j.apergo.2009.04.011.
- Hancock, P. A. 2014. “Automation: How Much is Too Much?” Ergonomics 57 (3): 449–454. doi:10.1080/00140139.2013.816375.
- Hancock, P. A. 2023. “Reacting and Responding to Rare, Uncertain and Unprecedented Events.” Ergonomics 66 (4): 454–478. doi:10.1080/00140139.2022.2095443.
- Hancock, P. A., and J. K. Caird. 1993. “Experimental Evaluation of a Model of Mental Workload.” Human Factors 35 (3): 413–429. doi:10.1177/001872089303500303.
- Hancock, P. A., M. H. Chignell, and A. Lowenthal. 1985. “An Adaptive Human-Machine System.”. Proceedings of the IEEE Conference on Systems, 15, 627–629.
- Hancock, P. A., and H. C. N. Ganey. 2003. “From the Inverted-U to the Extended-U: The Evolution of a Law of Psychology.” Journal of Human Performance in Extreme Environments 7 (1): 5–14. doi:10.7771/2327-2937.1023.
- Hancock, P. A., A. D. Kaplan, J. K. Cruit, G. M. Hancock, K. R. MacArthur, and J. L. Szalma. 2019. “A Meta-Analysis of Flow Effects and the Perception of Time.” Acta Psychologica 198: 102836. doi:10.1016/j.actpsy.2019.04.007.
- Hancock, P. A., and G. Matthews. 2019. “Workload and Performance: Associations, Insensitivities, and Dissociations.” Human Factors 61 (3): 374–392. doi:10.1177/0018720818809590.
- Hancock, P. A., A. A. Pepe, and L. L. Murphy. 2005. “Hedonomics: The Power of Positive and Pleasurable Ergonomics.” Ergonomics in Design: The Quarterly of Human Factors Applications 13 (1): 8–14. doi:10.1177/106480460501300104.
- Hancock, P. A., and J. S. Warm. 1989. “A Dynamic Model of Stress and Sustained Attention.” Human Factors 31 (5): 519–537. doi:10.1177/001872088903100503.
- Hancock, P. A., G. Williams, C. M. Manning, and S. Miyake. 1995. “Influence of Task Demand Characteristics on Workload and Performance.” The International Journal of Aviation Psychology 5 (1): 63–86. doi:10.1207/s15327108ijap0501_5.
- Harris, W. C., P. A. Hancock, and S. C. Harris. 2005. “Information Processing Changes Following Extended Stress.” Military Psychology 17 (2): 115–128. doi:10.1207/s15327876mp1702_4.
- Hertzberg, H. T. E. 1972. “The Human Buttocks in Sitting: Pressures, Patterns, and Palliatives.” SAE Transactions 81 (1): 39–47. doi:10.4271/720005.
- Hofstee, G., P. G. W. Jansen, A. H. De Lange, B. R. Spisak, and M. Swinkels. 2021. “The Cognitive Costs of Managing Emotions: A Systematic Review of the Impact of Emotional Requirements on Cognitive Performance.” Work & Stress 35 (3): 301–326. doi:10.1080/02678373.2020.1832608.
- Jackson, E. M. 2013. “Stress Relief - the Role of Exercise in Stress Management.” ACSM’S Health & Fitness Journal 17 (3): 14–19. doi:10.1249/FIT.0b013e31828cb1c9.
- Jacucci, G., S. Fairclough, and E. T. Solovey. 2015. “Physiological Computing.” Computer Magazine. 48 (10): 12–16. doi:10.1109/MC.2015.291.
- Jeon, M., B. N. Walker, and J.‑B. Yim. 2014. “Effects of Specific Emotions on Subjective Judgment, Driving Performance, and Perceived Workload.” Transportation Research Part F: Traffic Psychology and Behaviour 24: 197–209. doi:10.1016/j.trf.2014.04.003.
- Kaber, D. B., and M. R. Endsley. 1997. “Out-of-the-Loop Performance Problems and the Use of Intermediate Levels of Automation for Improved Control System Functioning and Safety.” Process Safety Progress 16 (3): 126–131. doi:10.1002/prs.680160304.
- Kibler, V. E., and M. S. Rider. 1983. “Effects of Progressive Muscle Relaxation and Music on Stress as Measured by Finger Temperature Response.” Journal of Clinical Psychology 39 (2): 213–215. doi:10.1002/1097-4679(198303)39:2.
- Kushnir, J., A. Friedman, M. Ehrenfeld, and T. Kushnir. 2012. “Coping with Preoperative Anxiety in Cesarean Section: Physiological, Cognitive, and Emotional Effects of Listening to Favorite Music.” Birth (Berkeley, Calif.) 39 (2): 121–127. doi:10.1111/j.1523-536X.2012.00532.x.
- Lajunen, T., D. Parker, and S. G. Stradling. 1998. “Dimensions of Driver Anger, Aggressive and Highway Code Violations and Their Mediation by Safety Orientation in UK Drivers.” Transportation Research Part F: Traffic Psychology and Behaviour 1 (2): 107–121. doi:10.1016/S1369-8478(98)00009-6.
- Lavie, T., and J. Meyer. 2010. “Benefits and Costs of Adaptive User Interfaces.” International Journal of Human-Computer Studies 68 (8): 508–524. doi:10.1016/j.ijhcs.2010.01.004.
- Lazar, J. N., and S. Pearlman-Avnion. 2014. “Effect of Affect Induction Method on Emotional Valence and Arousal.” Psychology 05 (07): 595–601. doi:10.4236/psych.2014.57070.
- Lee, M., S. Lee, S. Hwang, S. Lim, and J. H. Yang. 2023. “Effect of Emotion on Galvanic Skin Response and Vehicle Control Data during Simulated Driving.” Transportation Research Part F: Traffic Psychology and Behaviour 93: 90–105. doi:10.1016/j.trf.2022.12.010.
- Lewis, P. A., H. D. Critchley, P. Rotshtein, and R. J. Dolan. 2007. “Neural Correlates of Processing Valence and Arousal in Affective Words.” Cerebral Cortex (New York, N.Y.: 1991) 17 (3): 742–748. doi:10.1093/cercor/bhk024.
- Li, S., T. Zhang, B. D. Sawyer, W. Zhang, and P. A. Hancock. 2019. “Angry Drivers Take Risky Decisions: Evidence from Neurophysiological Assessment.” International Journal of Environmental Research and Public Health 16 (10): 1–14. doi:10.3390/ijerph16101701.
- Li, T., and S. Lajoie. 2021. “Predicting Aviation Training Performance with Multimodal Affective Inferences.” International Journal of Training and Development 25 (3): 301–315. doi:10.1111/ijtd.12232.
- Linnenbrink-Garcia, L., E. Patall, and R. Pekrun. 2016. “Adaptive Motivation and Emotion in Education: Research and Principles for Instructional Design.” Policy Insights from the Behavioral and Brain Sciences 3 (2): 228–236.), doi:10.1177/2372732216644450.
- Loukidou, L., J. Loan-Clarke, and K. Daniels. 2009. “Boredom in the workplace: More than monotonous tasks.” International Journal of Management Reviews 11 (4): 381–405. doi:10.1111/j.1468-2370.2009.00267.x.
- Lu, Y., K. J. Jaquess, B. D. Hatfield, C. Zhou, and H. Li. 2017. “Valence and Arousal of Emotional Stimuli Impact Cognitive-Motor Performance in an Oddball Task.” Biological Psychology 125: 105–114. doi:10.1016/j.biopsycho.2017.02.010.
- Mackworth, N. H. 1945. Faulty perception caused by blank spells without signals during experiment on prolonged visual search. Air Ministry F.P.R.C. Report No. 568.
- Mackworth, N. H. 1950. Researches on the measurement of human performance. Med. Res. Council, Special Rep. Ser. No. 268. His Majesty’s Stationery Office.
- Marras, William S., and P. A. Hancock. 2014. “Putting Mind and Body Back Together: A Human-Systems Approach to the Integration of the Physical and Cognitive Dimensions of Task Design and Operations.” Applied Ergonomics 45 (1): 55–60. doi:10.1016/j.apergo.2013.03.025.
- Matthews, G., L. Dorn, T. W. Hoyes, D. R. Davies, A. I. Glendon, and R. G. Taylor. 1998. “Driver Stress and Performance on a Driving Simulator.” Human Factors 40 (1): 136–149, Article 1, doi:10.1518/001872098779480569.
- Meyer, W.‑U., R. Reisenzein, and A. Schützwohl. 2001. Einführung in die Emotionspsychologie, Bd.1, [Introduction to the Psychology of Emotion], 2nd ed. Bern, Switzerland: Hans Huber.
- Millgram, Y., M. K. Nock, D. D. Bailey, and A. Goldenberg. 2023. “Knowledge About the Source of Emotion Predicts Emotion-Regulation Attempts, Strategies, and Perceived Emotion-Regulation Success.” Psychological Science 34 (11): 1244–1255. doi:10.1177/09567976231199440.
- Morais, P., C. Quaresma, R. Vigário, and C. Quintão. 2021. “Electrophysiological Effects of Mindfulness Meditation in a Concentration Test.” Medical & Biological Engineering & Computing 59 (4): 759–773. doi:10.1007/s11517-021-02332-y.
- Nilsson, U., M. Unosson, and N. Rawal. 2005. “Stress Reduction and Analgesia in Patients Exposed to Calming Music Postoperatively: A Randomized Controlled Trial.” European Journal of Anaesthesiology 22 (2): 96–102. doi:10.1017/S0265021505000189.
- North, A. C., and D. J. Hargreaves. 1999. “Music and Driving Game Performance.” Scandinavian Journal of Psychology 40 (4): 285–292. doi:10.1111/1467-9450.404128.
- Paas, F., J. E. Tuovinen, H. Tabbers, and P. W. M. van Gerven. 2003. “Cognitive Load Measurement as a Means to Advance Cognitive Load Theory.” Educational Psychologist 38 (1): 63–71. doi:10.1207/S15326985EP3801_8.
- Pail, G., W. Huf, E. Pjrek, D. Winkler, M. Willeit, N. Praschak-Rieder, and S. Kasper. 2011. “Bright-Light Therapy in the Treatment of Mood Disorders.” Neuropsychobiology 64 (3): 152–162. doi:10.1159/000328950.
- Parasuraman, R., and P. A. Hancock. 2008. “Mitigating the Adverse Effects of Workload, Stress, and Fatigue with Adaptive Automation.”. In Performance Under Stress, edited by P. A. Hancock and J. L. Szalma, 45–58. Hampshire, UK: Ashgate Publishing.
- Parasuraman, R., M. Mouloua, and R. Molloy. 1996. “Effects of Adaptive Task Allocation on Monitoring of Automated Systems.” Human Factors 38 (4): 665–679. doi:10.1518/001872096778827279.
- Parasuraman, R., and V. Riley. 1997. “Humans and Automation: Use, Misuse, Disuse, Abuse.” Human Factors: The Journal of the Human Factors and Ergonomics Society 39 (2): 230–253. doi:10.1518/001872097778543886.
- Pêcher, C., C. Lemercier, and J. M. Cellier. 2009. “Emotions Drive Attention: Effects on Driver’s Behaviour.” Safety Science 47 (9): 1254–1259. doi:10.1016/j.ssci.2009.03.011.
- Pekrun, R., and L. Linnenbrink-Garcia. 2012. “Academic Emotions and Student Engagement.” In Handbook of Research on Student Engagement, edited by S. L. Christenson, A. L. Reschly, and C. Wylie, 259–282. Berlin, Germany: Springer.
- Pfaff, M. S. 2008. “Effects of Mood and Stress on Group Communication and Performance in a Simulated Task Environment.” [Unpublished dissertation]. The Pennsylvania State University.
- Plass, J. L., S. Heidig, E. O. Hayward, B. D. Homer, and E. Um. 2014. “Emotional Design in Multimedia Learning: Effects of Shape and Color on Affect and Learning.” Learning and Instruction 29: 128–140. doi:10.1016/j.learninstruc.2013.02.006.
- Plass, J. L., and S. Kalyuga. 2019. “Four Ways of Considering Emotion in Cognitive Load Theory.” Educational Psychology Review 31 (2): 339–359. doi:10.1007/s10648-019-09473-5.
- Prinzel, L. J. I., F. G. Freeman, M. W. Scerbo, P. J. Mikulka, and A. T. Pope. 2003. “Effects of a Psychophysiological System for Adaptive Automation on Performance, Workload, and the Event-Related Potential P300 Component.” Human Factors 45 (4): 601–613. doi:10.1518/hfes.45.4.601.27092.
- Prinzel, L. J. I., A. T. Pope, and F. G. Freeman. 2001. January 6). Application of Physiological Self-Regulation and Adaptive Task Allocation Techniques for Controlling Operator Hazardous States of Awareness (L-18075). https://ntrs.nasa.gov/citations/20010060397
- Quinlan, P., J. Lane, and L. Aspinall. 1997. “Effects of Hot Tea, Coffee and Water Ingestion on Physiological Responses and Mood: The Role of Caffeine, Water and Beverage Type.” Psychopharmacology 134 (2): 164–173. doi:10.1007/s002130050438.
- Reimer, B., B. Mehler, J. F. Coughlin, K. M. Godfrey, and C. Tan. 2009. “An on-Road Assessment of the Impact of Cognitive Workload on Physiological Arousal in Young Adult Drivers. In Proceedings of the First.” International Conference on Automotive User Interfaces and Interactive Vehicular Applications (pp. 115–118). doi:10.1145/1620509.1620531.
- Russell, J. A. 1980. “A Circumplex Model of Affect.” Journal of Personality and Social Psychology 39 (6): 1161–1178. doi:10.1037/h0077714.
- Sanghavi, H., Y. Zhang, and M. Jeon. 2023. “Exploring the Influence of Driver Affective State and Auditory Display Urgency on Takeover Performance in Semi-Automated Vehicles: Experiment and Modelling.” International Journal of Human-Computer Studies 171: 102979. doi:10.1016/j.ijhcs.2022.102979.
- Schmitz-Hübsch, A., and S. Fuchs. 2022. “Herausforderungen Bei Der Realisierung Affekt-Adaptiver Systeme in Sicherheitskritischen Umgebungen [Challenges in the Implementation of Affect-Adaptive Systems in Safety-Critical Environments].” In Mensch-Maschine-Interaktion. Konzeptionelle, soziale und ethische Implikationen neuer Mensch-Technik-Verhältnisse, edited by O. Friedrich, J. Seifert, and S. Schleidgen, 302–311, Paderborn, Germany: Brill, Mentis. doi:10.30965/9783969752609_023.
- Schmitz-Hübsch, A., S. M. Stasch, R. Becker, S. Fuchs, and M. Wirzberger. 2022. “Affective Response Categories - Toward Personalized Reactions in Affect-Adaptive Tutoring Systems.” Frontiers in Artificial Intelligence 5: 873056. Article 873056. doi:10.3389/frai.2022.873056.
- Schneider, S., M. Wirzberger, and G. D. Rey. 2019. “The Moderating Role of Arousal on the Seductive Detail Effect in a Multimedia Learning Setting.” Applied Cognitive Psychology 33 (1): 71–84. doi:10.1002/acp.3473.
- Srivarsan, R., G. Sridevi, and S. Preetha. 2021. “An Evaluation on Use of Stress Ball Exercise on Stress Management among Student Population – a Cross Section Study.” Journal of Pharmaceutical Research International 33: 506–514. doi:10.9734/jpri/2021/v33i47B33150.
- Staal, M. A. 2004. Technical Report. NASA/TM-2004-212824. Moffett Field, California: NASA Ames Research Center.
- Stickel, C., M. Ebner, S. Steinbach-Nordmann, G. Searle, and A. Holzinger. 2009. “Emotion Detection: application of the Valence Arousal Space for Rapid Biological Usability Testing to Enhance Universal Access.” Universal Access in Human-Computer Interaction. Addressing Diversity: 5th International Conference, UAHCI 2009, 615–624. doi:10.1007/978-3-642-02707-9_70.
- Szalma, J. L., and G. W. L. Teo. 2012. “Spatial and Temporal Task Characteristics as Stress: A Test of the Dynamic Adaptability Theory of Stress, Workload, and Performance.” Acta Psychologica 139 (3): 471–485. doi:10.1016/j.actpsy.2011.12.009.
- Toet, A., & van Erp, J. (2019). The EmojiGrid as a Tool to Assess Experienced and Perceived Emotions. Psych, 1, 1, 469–481. doi:10.3390/psych1010036.
- Underwood, G., P. Chapman, S. Wright, and D. Crundall. 1999. “Anger While Driving.” Transportation Research Part F: Traffic Psychology and Behaviour 2 (1): 55–68. doi:10.1016/S1369-8478(99)00006-6.
- Vine, Samuel J., Liis Uiga, Aureliu Lavric, Lee J. Moore, Krasimira Tsaneva-Atanasova, and Mark R. Wilson. 2015. “Individual Reactions to Stress Predict Performance during a Critical Aviation Incident.” Anxiety, Stress, and Coping 28 (4): 467–477, Article 4, doi:10.1080/10615806.2014.986722.
- Weiss, H. B., and R. Cropanzano. 1996. “Affective Events Theory.” Research in Organizational Behavior 18 (1): 1–74. https://web.mit.edu/curhan/www/docs/articles/15341_readings/affect/affectiveeventstheory_weisscropanzano.pdf.
- Whitlow, S. D., P. M. Ververs, B. Buchwald, and J. Pratt. 2005. “Scheduling Communications with an Adaptive System Driven by Real-Time Assessment of Cognitive State.”. In Human Factors and Ergonomics Series. Foundations of Augmented Cognition, edited by D. D. Schmorrow, 1st ed., 367–376, Boca Raton, Florida: CRC Press.
- Wickens, C. D. 2002. “Multiple Resources and Performance Prediction.” Theoretical Issues in Ergonomics Science 3 (2): 159–177. doi:10.1080/14639220210123806.
- Wickens, C. D. 2005. “Attentional Tunneling and Task Management.”. Proceedings of the 13th International Symposium on Aviation Psychology, 620–625. doi:10.1080/10508410902766549.
- Wiegmann, D. A., and S. A. Shappell. 2001. Human error analysis of commercial aviation accidents using the human factors analysis and classification system (HFACS) (DOT/FAA/AM-01/3). Office of Aviation Medicine. https://rosap.ntl.bts.gov/view/dot/15409
- Yang, H., J. Han, and K. Min. 2019. “Distinguishing Emotional Responses to Photographs and Artwork Using a Deep Learning-Based Approach.” Sensors (Basel, Switzerland) 19 (24): 5533. doi:10.3390/s19245533.
- Yerkes, R. M., and J. D. Dodson. 1908. “The Relation of Strength of Stimulus to Rapidity of Habit-Formation.” Journal of Comparative Neurology and Psychology 18 (5): 459–482. doi:10.1002/cne.920180503.
- Yoon, C. Y. 2009. “The Effect Factors of End-User Task Performance in a Business Environment: Focusing on Computing Competency.” Computers in Human Behavior 25 (6): 1207–1212. doi:10.1016/j.chb.2009.06.008.
- Zimasa, T., S. Jamson, and B. Henson. 2017. “Are Happy Drivers Safer Drivers? Evidence from Hazard Response Times and Eye Tracking Data.” Transportation Research Part F: Traffic Psychology and Behaviour 46: 14–23. doi:10.1016/j.trf.2016.12.005.
- Zsidó, A. N., D. T. Stecina, and M. C. Hout. 2022. “Task Demands Determine Whether Shape or Arousal of a Stimulus Modulates Competition for Visual Working Memory Resources.” Acta Psychologica 224: 103523. Article 103523. doi:10.1016/j.actpsy.2022.103523.