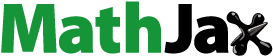
Abstract
The Financial Analysts Journal is a leading forum for sharing knowledge about investment management. It often features academic research, but its focus has consistently been on practice and how new knowledge can support one of society’s most important endeavors: preserving and growing assets for our collective economic future. In this article, I review some key contributions about portfolio management published in this journal. The lively debates demonstrate how asset management has evolved through give-and-take discussion of innovation versus established practice. The Financial Analysts Journal has consistently introduced its readers to new ideas and methods. In doing so, it has greatly improved professional practice.
Disclosure: The author reports no conflicts of interest.
Editor’s Note
Submitted 8 April 2020
Accepted 29 April 2020 by Stephen J. Brown
The Financial Analysts Journal has long played an important role in the professionalization of investment management. During its distinguished history, it has been a leading outlet, together with the Journal of Portfolio Management, for shared knowledge about the critical issue of portfolio choice. The Financial Analysts Journal regularly brings academic finance theory into practice, but just as often, it opens new challenges for scholars to explore. This article traces the development of modern portfolio management through a tour of some of the Financial Analysts Journal’s most important contributions to that field. The selection is personal. Over the course of my academic career, the Financial Analysts Journal has introduced me to ideas and techniques that lay parallel to, and yet were distinctly different from, the concurrent topics in academic finance journals. It was—and still is—full of big ideas, interesting puzzles, and new insights into such topics as asset allocation, diversification, performance measurement, hedging, and investor behavior. Academia has introduced a number of key models and methods to practice over the past several decades, but translating, adapting, and using these models has been the central concern of practice. Contributors to the Financial Analysts Journal have been and continue to be at the vanguard of this effort.
Bottom Up vs. Top Down
When the Financial Analysts Journal was founded in 1945, practitioners had no comprehensive financial model of portfolio management. For the first two decades of its life, the Financial Analysts Journal focused squarely on security analysis and valuation methods. The informed analyst was interested in such questions as which variables best forecast stock and bond performance, the economic outlook for various industries and commodities, and how the macroeconomy affected markets. Important issues in the pages of the Financial Analysts Journal in this early era included the forecasting power of the price-to-earnings ratio (Molodovsky 1953), the challenge of growth stock valuation (Jenks 1947), and the professionalization of security analysis (Graham 1946, 1952). As Irving Kahn (2005, p. 6), a founding editorial board member, put it:
Many of us were interested in hands-on, fundamental analysis of actual companies and actual industries. The approach we pursued was to give how-to advice for day-to-day practice on analyzing company, industry, and national statistics and facts.
Diversification was certainly recognized, however, as beneficial. A smattering of articles considered the broader structure of the portfolio. In an important contribution, Carter (1950) argued that in a mutual fund context, analyzing and appraising a security on its individual merits is not enough; rather, stocks must be considered within the context of the overall portfolio. Several Financial Analysts Journal contributors highlighted portfolio diversification within US markets. A study by Robert Milne (1958) used a panel of a decade of earnings by industry to argue that institutions should hold a mix of stocks mirroring the multiple sources of corporate profits. He closed by posing the question to readers, “What constitutes sound diversification?” (p. 54). Economists Edward Renshaw and Paul Feldstein contributed one of the most prescient of the early Financial Analysts Journal articles about diversified portfolios (Renshaw and Feldstein 1960). Their proposal for an “unmanaged” index fund is discussed in detail by Stephen Brown in his recent article in this journal (Brown 2020).
The “New Research.”
G. H. Fisher (1953) introduced mean–variance optimization to Financial Analysts Journal readers. His article focused on the problem of estimating inputs to the model developed by Harry Markowitz. Fisher’s time overlapped with Markowitz’s time at the Rand Corporation, and Fisher was intimately familiar with Markowitz’s (1952) landmark paper “Portfolio Selection” that appeared in the Journal of Finance less than a year earlier than Fisher’s article. In fairly technical terms, Fisher argued that diversification derived from the structure of the economy and that a stochastic version of Leontief’s input–output model could help estimate the covariance matrix.Footnote1 Fisher’s article is evidence that, on the one hand, the Financial Analysts Journal did not shy away from highly mathematical but potentially useful contributions.
On the other hand, the profession did not entirely embrace new methods and tools from operations research. The Markowitz model was lumped together with other “computer programs” that, to some extent, posed a challenge to the bottom-up security analysis that prevailed. In 1963, when Benjamin Graham reflected in the Financial Analysts Journal on the future of financial analysis, much of his article focused on the traditional role of valuation—in which he was the reigning expert. Graham did see the future in applying statistical and computational models, however, and he nicely summarized the approach as follows:
I believe there is theoretical merit in the original Markowitz concept of “efficient portfolios.” This seeks to find by a computer program the portfolio that offers the largest expected return compatible with a given acceptable risk, or, conversely, the least risk associated with a required or expected return. Under this approach it would be up to the Analyst to estimate the degree of risk as well as the expectable return for each issue in the large group from which the portfolio would be drawn. (Graham 1963, p. 70)
In the following decades, the flow of articles in the Financial Analysts Journal reflected the evolution of investment analysis from a bottom-up, fundamental quest for value to a top-down, holistic approach that relied on new quantitative methods. By 1966, mean–variance analysis, once a shock to the profession, was hailed as “the new research” (Shelton 1966). Mathematics and statistics became forever part of the toolkit of readers of the Financial Analysts Journal.
The Markowitz Revolution
Markowitz’s mean–variance model represented a revolutionary approach to portfolio construction, but practitioners quickly realized that implementation presented challenges:
What securities or asset classes should be used?
What inputs should be used?
What should be done when the mathematical model generated patently absurd portfolios?
What time horizon is appropriate?
When should the model be updated?
How does one estimate investor risk aversion, preferences, and goals?
How does one integrate investment objectives?
The Problem of Inputs.
Applying mean–variance optimization to the universe of investable securities requires the estimation of a huge covariance matrix. William Baumol’s (1966) article “Mathematical Analysis of Portfolio Selection” is an early, highly readable assessment of this problem. Despite the industry having moved into the computer age, covariance estimation was evidently extremely costly. Citing the pathbreaking paper of William Sharpe (1963), Baumol (1966, p. 98) wrote:
The cost of a simplified portfolio calculation involving 1500 securities has been estimated to lie between $150 and $350 for a single run in computer time alone, and it has been suggested that a single run of the complete Markowitz calculations might come to as much as 50 times these orders of magnitude.
Baumol articulated the Achilles heel of applying the model at the individual-security level—namely, that inverting a covariance matrix of 2,000 security returns was empirically impossible. Forecasting expected returns security by security was also problematic—not only because of efficient market theory but also because companies change over time: “For even if the individual data were all known, the portfolio selection calculation in which they were utilized is hardly simply and intuitively obvious” (Baumol 1966, p. 99).
Sharpe’s 1963 article was arguably more important to the practice of portfolio management than his famous CAPM equilibrium theory. In the 1963 work, he introduced the “diagonal model” showing how the vast dimensionality of the covariance matrix of individual security returns could be reduced to a manageable size by the assumption of a single factor representation. In his words:
The major characteristic of the diagonal model is the assumption that the returns of various securities are related only through common relationships with some basic underlying factor. The return from any security is determined solely by random factors and this single outside element. . . . More explicitly:
where Ai and Bi are parameters, Ci is a random variable with an expected value of zero and variance Qi, and I is the level of some index. The index, I, may be the level of the stock market as a whole, the Gross National Product, some price index or any other factor thought to be the most important single influence on the returns from securities. The future level of I is determined in part by random factors:
where An+1 is a parameter and
Cn+1 is a random variable with an expected
value of zero and a variance of Qn+1. It is
assumed that the covariance between Ci
and Cj is zero for all values of i
and j (i
j).
(Sharpe 1963, p. 281)
Sharpe noted later in the article, “The model’s extreme simplicity enables the investigator to perform a portfolio analysis at a very small cost” (p. 291).
I quoted Sharpe (1963) at length because his method radically changed the application of mean–variance optimization to portfolio management. It also provided the intuition behind the capital asset pricing model (CAPM). If the simplifying assumption of a single-factor model delivers a close approximation to the efficient frontier, then the loading on that single factor is perhaps a sufficient metric, in practice, for risk. For Sharpe, evidently the notion of an equilibrium was closely tied to the practical challenge of implementation of the Markowitz model. His 18 contributions to the Financial Analysts Journal over his career testify to a lifelong interest in the practice of asset management, not simply in its theory.
The diagonal model—single-factor representation of security returns—was an important contribution to portfolio optimization. Not only did it lead to a significant reduction in the dimensionality of the portfolio optimization problem by reducing the number of input parameters to be estimated, but it also led to simplified algorithms for portfolio selection.Footnote2 In a reappraisal of factor model representations of security returns in the context of large-scale portfolio optimization, Fan, Fan, and Lv (2008) argued that because portfolio optimization algorithms are based on the inverse of the return covariance matrix, reducing covariance matrixes to a factor structure is not only less computationally complex but also provides more reliable estimates—and, therefore, more reliable optimization output—than does inverting the full covariance matrix.
The late Lawrence Fisher was a long-time member of the University of Chicago faculty and co-founder, with James Lorie, of the database from which all modern research in equities has sprung: CRSP (the Center for Research in Security Prices). He also contributed to the theory of optimization. Fisher’s 1975 Financial Analysts Journal article was motivated by the problem of parameter estimation errors and resulting nonsensical portfolios. He reconciled potentially flawed parameter inputs with capital market theory by working backwards from the investor’s current portfolio. Taking current portfolio variance as a measure of risk tolerance and using Sharpe’s diagonal model, he showed that the dual of the portfolio optimization problem identifies a set of expected asset returns that support the current allocation.Footnote3 These results can be compared with theory—for example, with the CAPM—or with an investor’s own fundamental views. Rather than imposing priors on the outputs to the optimization, he used the outputs to adjust priors on the inputs. He showed how to use these expected returns iteratively to tune the portfolio. His work appears to have paralleled Sharpe (1974), who also proposed an iterative allocation process through inspection of implied expected returns derived from the dual problem.
Fisher (1975) described how the idea originated in discussions of the dual approach with Markowitz in 1964, and he credits Treynor and Black (1973) with a Bayesian approach related to the CAPM. Sharpe’s 1974 article might have prompted Fisher to finally write the idea up and place it in an influential practitioner publication. Regardless of precedent, this notion of using the optimization framework as a structure for assessing the logic of the inputs as well as the reasonableness of the output emerged early in practice. Both works prefigure Black and Litterman (1991), a highly influential model for applied portfolio management. Black and Litterman proposed that the initial expected return inputs should be whatever are required to ensure that the implied default asset allocation is equal to what we observe in the markets.
The practical problem of statistical inputs to mean–variance optimization continued to be a focus of the Financial Analysts Journal. A straightforward tack was taken by Ibbotson and Sinquefield (1979), who updated their 1977 study of historical estimates of the risk and return of major US asset classes. The firm Ibbotson Associates grew, in part, to meet the demand in practice for reliable statistical evidence about risk and return by asset class. In a stationary world, the longer the time series, the more accurate the estimate of the mean. In 1977, the CRSP data provided 52 years of returns—a reasonable framework for making projections about the long-term future of US capital markets. Jeremy Siegel (1992) extended the data for US stock and bond returns back to 1802, providing even more data on risk, return, and correlation.
Dimson, Marsh, and Staunton (2004) presented an international version of the Ibbotson data based on more than a century of annual returns to global financial markets—essentially doubling the length of the Ibbotson and Sinquefield study. They demonstrated that the risk-and-return characteristics documented in US markets—particularly the equity risk premium—were consistent with the international evidence.
Of course, Financial Analysts Journal contributors also recognized problems with relying on historical inputs to mean–variance optimization. Carleton and Lakonishok (1985) showed how using historical estimates may be misleading because of estimation error.
Despite the optimizer revolution, not all Financial Analysts Journal contributors advocated its use. The critique of mean–variance optimization launched by Richard Michaud (1989) was particularly influential. He coined the phrase “estimation-error maximizers” for the Markowitz model. Michaud observed that investment professionals were abandoning mean–variance optimizers when they found their portfolios to be “unintuitive and without obvious investment value.” He also highlighted research to reduce estimation error through such tools as Bayes–Stein shrinkage (Philippe Jorion 1986). Michaud’s message is that the most attractive assets—those with the highest mean—are also those most likely to have the highest estimation error. This caution is important to those chasing historical asset class returns. Philippe Jorion (1992), whose Bayes–Stein work Michaud cited, published his own take on input estimation problems in a Financial Analysts Journal article. Studying how best to construct an international bond portfolio, he pioneered the use of simulation to quantify the range of portfolio weights one would obtain for each country.
Rebalancing and Risk Parity.
Another big issue that emerged in applying the mean–variance model was portfolio rebalancing. In “Adaptive Asset Allocation Policies” in the Financial Analysts Journal, Sharpe (2010) summarized why rebalancing matters and why it necessarily reflects investor beliefs. He pointed out that rebalancing is contrarian, so it must reflect a view that the equity market will mean-revert—a theoretically unsatisfying axiom with marginal empirical support. In contrast, a buy-and-hold portfolio presumes a static supply of assets. He proposed an adaptive approach that would, taking into account both growth in values and net security issuance, rebalance toward the composition of the world wealth portfolio. He relied, again, on the dual of the portfolio optimization problem and a conviction that equilibrium asset pricing is a reasonable prior. In Sharpe’s view, assessing the asset mix of the world wealth portfolio is easier than confidently estimating expected returns.
An interesting model that contradicts Sharpe’s equilibrium proposition emerged in financial practice in the mid-2000s—namely, risk parity. In simple form, the model derives weights from the risks of the asset classes, not their weights in the world wealth portfolio or from implied expected returns. An example, which is illustrated in Asness, Frazzini, and Pedersen (2012), weights the equity and debt components according to the inverse of their rolling three-year excess volatilities. Without leverage, this process would result in something like a 25%/75% stock/bond portfolio and, in standard capital market equilibrium, an expected return consistent with a beta of 0.25. Levering the fixed-income part of the portfolio to the same risk as the equity, however, brings the investor to risk parity. The backtest carried out by Asness et al. from 1926 shows the risk-parity portfolio handily beating a 60/40 long-only portfolio and the value-weighted market portfolio on a raw and a risk-adjusted basis.
Proponents of risk parity recognize that it is inconsistent with standard capital market theory because it implies that low-risk debt lies above the capital market line. Asness et al. (2012) and Anderson, Bianchi, and Goldberg (2012) proposed an institutional/behavioral explanation: leverage aversion. Leverage itself presents a risk for which the marginal investor demands compensation. Given a target return of, say, 8% annually, investors (either by choice or because of institutional constraints) prefer to hold more equities rather than lever a portfolio of low-yield fixed-income assets. If the marginal investor is leverage averse, the result could compress the spread in expected returns between low-risk assets and equity and generate a high Sharpe ratio for the portfolio. The resulting premium for low-beta assets is consistent with Frazzini and Pedersen (2010), who pointed out that it is consistent with a constrained version of capital market theory investigated by Fischer Black (1972).
Skeptics of risk parity expressed concern that leverage is a seductive sinecure for underfunded pension liabilities (Sullivan 2010) and that belief in a higher-than-merited Sharpe ratio for levered bonds is not necessary to support a capital market line with a higher slope than the equity risk premium as commonly measured. Some analysts (e.g., Siegel 2010) noted that the market portfolio should contain a mix of all asset classes, not just equities. In fact, with a broad set of inputs, an analyst might be able to estimate the market portfolio in better ways than with the risk-parity heuristic.
How Much Does Asset Allocation Matter Anyway?
A question that encapsulates the age-old contrast between top-down and bottom-up portfolio management is the importance of active management versus asset allocation. To address this issue, Brinson, Hood, and Beebower (1986) decomposed the variance of returns to 91 large pension plans over the period 1974–1983 into three parts: returns resulting from passive allocation, from timing, and from selection. They attributed 94% of the variation in the average fund’s performance to its passive asset allocation. Timing and security selection, once the original bread and butter of financial analysts (and Financial Analysts Journal readers in its early years), made up less than 7% of the performance differential. These results were updated in 1991 (Brinson, Singer, Beebower 1991) with another 10 years of a larger, out-of-sample dataset, and the results were the same: The marginal impact of getting the allocation right swamped the focus on how it was executed.
Brinson et al. (1986) sparked a 20-year debate about the importance of asset allocation, the subtext of which was whether active management mattered (e.g., Hood 2005; Ellis 2015). Ibbotson and Kaplan (2000) pointed out that Brinson, Hood, and Beebower’s analysis was not as straightforward as it seemed. Brinson et al.’s (1986) method did not account for most funds having roughly the same market exposure, or beta, which would necessarily explain the lion’s share of time-series variation in portfolio performance. In their study, Ibbotson and Kaplan asked how much of the variation in returns among funds is explained by differences in policy. They studied 10 years of returns and allocations for 94 balanced mutual funds for 1989–1998 and a similar sample of pension funds. Essentially, they verified Brinson et al.’s (1986) result: A regression on the market could explain 80%–90% of time-series variation in returns. They found, however, that performance differences among funds, estimated by cross-sectional regressions of individual funds on their passive policy benchmarks, explained 35%–40% of differences in fund performance. In short, when the common exposure was accounted for, Ibbotson and Kaplan found that security selection explains more of the return variation among funds than asset allocation explains. The authors made a simple econometric point: Cross-sectional regressions remove the time-series effect of common movement via the intercept term in the regression. This approach is useful in studying why one fund’s return differs from another, whereas the Brinson et al. (1986) time-series approach is useful for understanding how well a passive proxy explains the average performance of a population of funds.
The controversy was joined by several other Financial Analysts Journal authors. For example, Vardharaj and Fabozzi (2007) found a similar difference between time-series and cross-sectional variation in their study of US stock portfolios. Xiong, Ibbotson, Idzorek, and Chen (2010) followed up with larger fund samples and a slightly different benchmark of industry average performance. In their Financial Analysts Journal comment, Sénéchal and Singer (2010) argued that asset allocation during the crash mattered a lot but the Xiong et al. approach masked this effect. Thus, asset allocation remains an important issue. Evidently, pointing out methodological differences in measuring the effects of asset allocation did not settle differences in interpretation. Regardless, the debate was useful for Financial Analysts Journal readers in choosing what approach to take to answer various kinds of questions.
Risk
Some of the biggest financial innovations of the twentieth century were derivatives and dynamic models of portfolio choice. The Black–Scholes model was a watershed in the theory of asset pricing, but its mathematical complexity provided limited intuition (Black and Scholes 1972, 1973; Merton 1973b). A year after the model’s publication in academic outlets, Black (1975) introduced option pricing to Financial Analysts Journal readers. He straightforwardly explained how the Black–Scholes option valuation model worked and provided a schedule of hedge ratios for those daunted by the mathematics. Others also pressed to bridge the gap between the complex formula and the needs of practice. For example, Dimson (1977a, 1977b) developed a graphical method for rapidly calculating call options.
The potential application of option-pricing theory to portfolio management was quickly recognized. University of California, Berkeley, professor Nils Hakansson (1976) floated a visionary proposal in his Financial Analysts Journal article “The Purchasing Power Fund: A New Kind of Financial Intermediary.” He described a means by which investors could buy “supershares” that paid out in specific market outcomes. These conditional contracts could serve as building blocks for an investor to construct payoffs of all sorts—including downside risk protection in case of a market decline. With the solution to option pricing at hand, investors could finely tailor payoffs to suit their preferences, and Hakansson envisioned new institutions to deliver these tradable baskets of securities.
Later, Cox, Ross, and Rubinstein (1979) published their famous article on binomial option pricing. It was revolutionary because it showed how to dynamically construct an option even when it did not exist in the marketplace. Shortly thereafter, Mark Rubinstein founded Leland, O’Brien, and Rubinstein (LOR) and began using the binomial model to offer capital preservation to institutional investors. In the Financial Analysts Journal in 1981, Rubinstein and Leland (p. 69) explained the mechanism of a dynamic hedge that lay at the heart of the binomial model:
If options on a particular stock or on a portfolio do not exist, we can create them by using the appropriate strategy for the underlying asset and cash. For example, we can effectively create an at-the-money protective put option on our equity portfolio. We would begin by placing part of our capital in the equity portfolio and part in cash and then, without changing the composition of the equity portfolio, shift between the portfolio and cash as the equity portfolio value changes and as the “expiration date” approaches. Such an investment strategy would be tantamount to insuring the equity portfolio against losses by paying a fixed premium to an insurance company.
Rubinstein’s (1985) more detailed account, “Alternative Paths to Portfolio Insurance,” having won first prize in the Institute for Quantitative Research in Finance competition in 1984, appeared in the July/August 1985 issue of the Financial Analysts Journal. He showed how the binomial model could be used to construct a “synthetic put,” a dynamic hedge between equity and debt positions that theoretically would maintain a floor value for the portfolio. LOR’s portfolio insurance was a mathematically derived stop-loss strategy that would sell equities as their value declined—reaching a zero stock position before hitting the floor. The article briefly touched on one potential weakness: “Stop-loss strategies may be threatened by jumps in security prices” (Rubinstein 1985, p. 49). How LOR would provide insurance against security price jumps was not clear in the article.
Other contributors to the Financial Analysts Journal filled in some of the details about the effects of portfolio insurance. For example, Clarke and Arnott (1987), in an article accepted prior to the 1987 crash, illustrated the trade-offs and costs associated with holding or constructing a put on the portfolio. They showed how the put created a spike in the return distribution near the floor and that the cost of the put was high compared with the expected return of an all-equity portfolio. Bookstaber and Clarke (1985) pointed out that a portfolio with options was no longer lognormally distributed; a third moment had to be considered. Therefore, standard portfolio metrics, such as the capital market line, security market line, and the Sharpe and Treynor measures, were not valid tools for risk adjustment in such a portfolio. Mean–variance optimization could not be used for portfolio choice when options were a significant component of the portfolio. Modern financial tools had once again changed the paradigm of portfolio management. Just when Financial Analysts Journal readers figured they had mastered the new finance, the game changed once again.
The binomial model, and the LOR application of it to portfolio insurance, provided a key insight for investment managers. The put was no magic. It was an intuitively appealing variation of a more traditional stop-loss strategy where, instead of a dichotomous choice between risky and safe assets, the choice was based on a schedule of continuous rebalancing between stocks and bonds over a fixed time horizon. Other researchers soon showed how to relax the time horizon constraint. Estep and Kritzman (1988) proposed TIPP—that is, “time-invariant portfolio protection.” And Perold (1986) proposed CPPI—that is, “constant proportion portfolio insurance.” Both were based on concepts introduced in Merton (1971) and discussed in Perold and Sharpe’s 1988 Financial Analysts Journal review article on varieties of dynamic allocation strategies. Dybvig (1999) extended the idea of CPPI to endowment spending policies that use a dynamic cash cushion to cover liabilities; this Financial Analysts Journal article won the Commonfund Prize that year.
The Crash of 1987.
The market crash of 1987 was, at that time, the biggest one-day decline in the history of the US stock market. It was a sudden free fall that called into question not only the legitimacy of the efficient market theory but also the reliability of quantitative investment models. In the January/February issue of 1988, Rubinstein assessed the connection between portfolio insurance and this market crash. True to his 1985 observation, market jumps made continuous rebalancing impossible. When the market opened down several percentage points, so-called portfolio insurance (a misnomer to begin with because it was not a guarantee) did not perform as expected. It failed at precisely the moment when it was most needed.
Rubinstein (1988) also reflected on the question of whether portfolio insurance caused the crash itself. He estimated that on Black Monday (19 October 1987), portfolio insurers accounted for about 11.5% of the volume and that the amount of equities committed to portfolio insurance strategies was $60 billion–$80 billion before the crash. He believed neither of these estimates seemed dispositive of guilt. After all, investors had always used stop-loss strategies, albeit not all based on a common quantitative model. More relevant, in his view, was a sudden fear about the structural integrity of the market itself. Investors on Black Monday discovered they could not execute their trades and had no clarity about when and how they could. Market failure may have provoked the nine standard deviation plunge of 22.6% in the Dow Jones Industrial Average. Or not: Richard Roll’s (1988) widely cited study of the crash found only marginal evidence that market arrangements played a role.
Paradoxically, Rubinstein (1988) also predicted that the crash of 1987 would increase the demand for protective dynamic strategies. A couple of generations after the extreme volatility of the 1930s, investors had, overnight, relearned the lessons of downside risk the hard way. He guessed they might be willing to forgo some return to avoid another such disaster. A search of books published over the period 1980–2012 on the term “portfolio insurance” (using the Google N-gram viewer) showed a peak in 1990 and a decline to near zero by 2012, which is consistent with a half-life of interest in extreme risk mitigation of about 10 years. Rubinstein, now deceased, was a true financial visionary. After portfolio insurance, he created the exchange-traded fund (ETF)—an innovation likely to be with us for a long time. The concept of the ETF had its origins in Hakansson’s (1976) supershares idea; the challenge lay in getting regulatory approval (McLaughlin 2007). In Rubinstein’s 1989 Financial Analysts Journal article, he proposed a tradable, large, diversified basket of stocks, which his firm launched and began trading on the Philadelphia Stock Exchange a few months before the article was published. Although Rubinstein planned to build out Hakansson’s entire “superfund” concept, only the first step—the ETF—was realized.
Hedging Liabilities
Every investment portfolio has an objective—namely, to meet a set of future expenditures. Pension funds, for example, want to provide for an expected stream of future liabilities with particular characteristics, including duration and sensitivity to inflation. Liabilities should drive portfolio choice, but integrating them into mean–variance optimization is not trivial. Markowitz’s original model used indifference curves derived from quadratic utility to proxy for the investor’s objective function. He also realized, however, that this abstraction made application difficult. In “Markowitz Revisited” (Markowitz 1976, p. 51), he suggested simply reporting the risk-and-return pairs from the frontier and leaving the choice up to the investor:
Real investors these days usually seem more comfortable with the idea of examining risk–return tradeoffs than with psychoanalyzing their utility function and letting the computer pick a portfolio that maximizes its expected value.
A Liability Asset.
In the 1980s, Martin Leibowitz, then research director at Salomon Brothers, began to publish studies on the importance of matching the duration of assets and liabilities. Leibowitz and Henriksson (1988) used Roy’s (1952) linearization to express the notion of a shortfall constraint—a disaster being the failure to meet pension liabilities. They proposed that the shortfall target should be a portfolio proxy for portfolio liabilities rather than a simple numeric value.
Leibowitz (1987) and Ezra (1991) applied this idea to pension plan sponsors and argued that defined benefit plan sponsors cared about the expected surplus of assets minus liabilities and thus should seek to maximize that value. The notion is simple. A pension fund holds a short position in a series of promised future cash payments and requires a long position to meet the liabilities. The present value of the liabilities is interest-rate sensitive and varies with their duration. Despite the trend in the 1980s to embrace the equity risk premium as a source of long-term growth, Leibowitz (1986) argued that bonds in the pension fund portfolio offer an important hedge against interest-rate shocks. He believed that chief investment officers should look at total portfolio duration to understand the pension’s exposure to underfunding.
But what about stocks? Their dividend stream is presumably infinite and growing. Leibowitz and Kogelman (1993) attributed the puzzlingly low empirical estimate of the duration of corporate equities to the observation that over the long term, equities are a hedge against inflation, which is correlated with interest rates. Delving deeply into the role equities play in a portfolio with bondlike liabilities, Bodie (1995) showed that an all-equity portfolio poses serious shortfall risk, even over a long horizon, and that not only does equity risk not diminish with the holding period but it may even grow.
The extremely practical technique of surplus optimization emerged from this and related research in the Financial Analysts Journal and the Journal of Portfolio Management. Researchers have added interesting extensions. For example, Bookstaber and Gold (1988), integrating the “liability asset” approach with portfolio insurance, argued that the liability can be properly proxied only by a dynamic portfolio. They showed that pension liabilities contain, at least conceptually, some equities with low R2 values, which provides the theoretical justification for pensions to hold equities, not just duration-matched bonds. In summary, the idea that liabilities can be modeled and hedged with a “shadow portfolio” of high interest-rate sensitivity is a fundamental insight. It almost certainly helps us understand—if not address—the current funding crisis in public pension funds and has continued to highlight the future consequences of a sustained low-interest-rate environment.
Value at Risk.
The goal of measuring the probability of a shortfall—and the sensitivity of that probability to factor exposures—motivated the widespread adoption in the 1990s of the concept of value at risk (VAR). Facing highly visible portfolio failures (such as the 1994 bankruptcy of Orange County, California, because of its use of derivatives), the investment profession adopted a constellation of metrics and methods for assessing the probability of Roy’s d—the disaster point. In a simple world that obeys lognormality and Sharpe’s diagonal model, calculating the probability of a disaster of a given magnitude over a given horizon is straightforward: It is the area under the left-most piece of the lognormal curve. Derivatives make this calculation vastly more complicated, and VAR estimation suffers from the familiar problem of parameter uncertainty as well as the curse in financial markets of fat-tailed distributions.
Linsmeier and Pearson (2000) provided Financial Analysts Journal readers a general overview of VAR methods. Some early red flags about the methodology include the study by Beder (1995), who found that, in practice, no two VAR measurements were alike. Her opinion was particularly valuable in light of her central role as a risk consultant attempting to prevent the Orange County bankruptcy. She also pointed out the dangerous but common misapprehension that a VAR loss estimate is a worst-case scenario; in fact, one should expect a loss at least as bad as the VAR for a given fraction of the time.
Philippe Jorion, whose 2006 book Value at Risk became the bible of VAR methods, also cautioned early in its application (Jorion 1996) that higher-order uncertainty about parameters was an important issue in VAR calculations. The seemingly precise quantification of expected minimum losses for given probability levels provided by VAR belies their inherent nature as estimates—indeed, estimates built on estimates, for which there is precious little empirical validation conditional on rare events. He pointed out that precise estimates of the left tail of a future distribution may be simply impossible. These and other articles demonstrate that, well before the financial crisis of 2008, the Financial Analysts Journal had become a major forum for discussion about (and critique of) VAR approaches.Footnote4
Beta and Risk: Factor Investing
The Financial Analysts Journal has also been an important forum for the topic of factor investing since virtually the inception of the concept. Sharpe and Cooper (1972) published a test of the CAPM risk–return relationship in the Financial Analysts Journal soon after Black, Jensen, and Scholes (1972) was published. Using CRSP data for the period 1931–1967, Sharpe and Cooper found that the top 10% of portfolios with high sensitivity to the market index (measured over 60 prior months) had high out-of-sample returns. This horizon was long enough to presume that realized returns reflected ex ante expected returns. This early test of the CAPM was particularly useful for investment managers because it provided an empirical foundation for using a single-factor model to build high-return portfolios.
The research on multifactor models also has a long history in the Financial Analysts Journal. Barr Rosenberg was an early innovator in the use of multifactor models of stock returns (Rosenberg 1982). He wrote presciently in the Financial Analysts Journal in 1982 about the challenges of estimating risk premiums associated with macroeconomic factors:
Various studies of factors in security returns—that is, common elements that underlie the returns of similar securities, such as shares in the same industry or bonds in the same quality group—have led to substantial progress in developing models for investment risk. These models allow prediction of the uncertainty of returns on securities and on portfolios of securities. Much less success has attended our efforts to establish the normal rewards for these factors, which are properties of general equilibrium. (p. 47)
In prior work, Rosenberg (1974) pointed out that, particularly in a multifactor framework, returns-based factor-loading estimates are subject to estimation error. He proposed using, instead, individual-security characteristics as the basis for factor construction. These traits are not regression based and can change as the company’s business changes. He argued that they can capture the systematic structure of residual risks that is otherwise difficult to estimate. This idea—the use of security characteristics as opposed to time-series estimates of factor loadings—has become an important approach to factor investing.Footnote5 Rosenberg found that after the market factor is extracted, company-level characteristics explain a significant amount of the residual covariance matrix. Interestingly, he also noted a problem—namely, unclear economic interpretation of the resulting factors.
Some 20 years after Sharpe and Cooper (1972) and 18 years after Rosenberg (1974), Fama and French (1992) revisited CAPM beta as a major determinant of expected stock returns. They raced the market factor against factor portfolios sorted on size, leverage, book‐to‐market equity, and the earnings-to-price ratio. The portfolio characteristics crowded out the market factor as the source of explanatory power for returns.
Research on these and related predictors of returns had a long prior history, but Fama and French (1992) invigorated an active modern interest in using characteristic-based factors to generate positive risk-adjusted returns.
The quest for powerful factors to explain stock returns continues apace. Academia and practice together have made significant progress since 1972 in modeling the cross-sectional differences in security returns.
Factors and the Macroeconomy.
Sharpe (1963) opened the door to much more than tests of the CAPM. Recall from his description of the diagonal model that it does not specify what the factor is. His CAPM theory identified it as the market portfolio, but the diagonal model allows it to be anything the analyst believes to be “the most important single influence on the returns from securities” (p. 281). So, the factor need not be the sole influence on returns, only the single most important one. It could even be a macroeconomic factor, such as the GNP. The general framework of Sharpe (1963) has proven to be extremely fruitful for theory and for practice. His diagonal model, devised for expediently addressing the dimensionality problem, opened the door for researchers to propose a variety of candidates for the most important factor—for example, consumption (Breeden 1979)—or to consider the relevance of multiple factors (Merton 1973a; Ross 1976a, 1976b).
Stephen Ross’s arbitrage pricing theory (APT) was a key innovation in factor investing. It proposed a link between asset returns and macroeconomic risks (Ross 1972, Ross 1976a, 1976b). The theory derived from the same concept as diagonality—that risk can be reduced to a few important dimensions defined by factors, each of which has an associated risk premium. Investors can hold a riskless asset with no factor covariance or take risk along factor dimensions in exchange for higher expected returns.
APT’s intuition is analogous to insurance companies choosing to underwrite a mix of property, casualty, and life depending on their specialization. A competitive, efficient marketplace for insurance determines the premium rates for each type of risk. An investor’s expected portfolio return (or a stock’s expected return) is simply a linear combination of the amount of exposure to each factor times the market-determined premium for that factor.
APT is useful for practice because it relaxes the CAPM implication that everyone would want to hold shares in the same market portfolio. The CAPM might tell investors to hold shares in one big index fund, but APT showed investors how to design portfolios that, instead, fit idiosyncratic preferences. Roll and Ross (1984) proposed just such an approach in their Financial Analysts Journal article. In it, they showed how to construct portfolios for investors who differ in their sensitivities to risks captured by macroeconomic variables—namely, inflation, industrial production, the default spread, and the term structure. Of course, most investors want to hedge against negative shocks to all of these. However, certain investors—for example, sovereign funds endowed with natural resources—might accept exposure to one risk, such as term structure shocks, in exchange for hedging crashes in global industrial production.
Chen, Roll, and Ross (1986) empirically identified important influences on stock returns that had a macroeconomic logic to them. These influences must be pervasive factors to command an insurance premium. Thus, a higher exposure to them should be compensated with a higher expected return. Chen et al. showed that betas on macro-factors explained cross-sectional differences in returns to US equities.
The APT framework is general enough to accommodate some of the other anomalies that have been systematized into investable factors. For example, the “betting against beta” puzzle discussed previously relies on the notion of leverage aversion. In APT terms, it can be interpreted as a theory about the risk premium on the yield-curve factor. Similarly, liquidity is an important systemic risk that is probably priced (Ibbotson, Chen, Kim, and Hu 2013). Less obvious, however, is momentum, which does not seem to easily fit known or postulated economic risk factors.Footnote6
A key question for the application of factor investing is how to meaningfully assess whether a client has a greater-than-average or less-than-average sensitivity to shocks to these factors. On the one hand, history can reveal certain statistical norms, but large shocks—for example “momentum crashes”—are rare and may not be representative. On the other hand, if everyone invested in factors that had a history of positive excess return but no special risk, then—as Sharpe would predict—prices for these factors should adjust.
The Financial Analysts Journal article written by Gregory Connor (1995) does an excellent job of comparing the explanatory power of three ways to construct factors: via loadings on macroeconomic variables (as in Chen et al. 1986), with statistical estimates of factors latent in returns (as in Roll and Ross 1980 and Connor and Korajczyk 1988), and on the basis of fundamental, characteristic-based groupings estimated by BARRA (the firm founded by Rosenberg). A comparison by Conner of these three methods is extremely useful. Using macroeconomic variables as risk factors, which makes the most sense economically, did the worst job of explaining differences in returns. The statistical model worked best (in sample), and using the fundamental factors did well—but mostly because of differences in the performance of industries—as opposed to identifying truly fundamental risk sources. Despite the economic logic of macroeconomic variables, study after study has confirmed that the Fama and French (1992) factors (and the periodic updates that Fama and French have provided as they continue to delve into the mystery of the cross-section of stock returns) are difficult to beat in terms of explanatory value.
Factor investing by various names, including “smart beta,” had become pervasive in asset management by the mid-2010s. It is not difficult to see why. Factors composed of securities with particular characteristics or past behavior were found to have performed well over long stretches of time and to have attractive covariance profiles. APT provided a theoretical framework about why the factors should work and an agenda for how to assess whether past performance will replicate out of sample. At the same time, factor investing provides a framework for incorporating the liability structure of the portfolio into the asset allocation process.
The continued challenge of modern asset pricing research is to convincingly explain the nature of the factors. Do the factors that best spread returns capture some pervasive fundamental risk, or are they driven by systematic behavioral biases and tendencies—which themselves may be sources of systematic risk? With many creative researchers looking for new factors, a “factor zoo” has not been difficult to identify. The problem for investors is whether these factors will deliver out-of-sample returns as risk premiums (if you believe in the APT) or alpha (if you do not).
Alternatives
A fundamental question about factor investing is whether all priced factors trade in public securities markets. There is no reason they should. Some assets may not be amenable to public ownership and control. Others may have information asymmetries that institutional investors cannot easily access and monitor. The fact that the public capital markets are limited in scope was long understood as a nuisance to asset pricing tests of factors. Roll (1977) pointed out that an investment could look like it had positive alpha but only because the market was not on the efficient frontier, which theory says is formed from all assets. Serious attempts to identify the global wealth portfolio have appeared in the Financial Analysts Journal. Doeswijk, Lam, and Swinkels (2014) estimated the components of the invested world asset portfolio over the period 1990–2012, an important update of Ibbotson and Siegel (1983).
The Endowment Model.
What is a problem to academia can be an opportunity for enterprising portfolio managers. In the 1990s, David Swensen, chief investment officer of the Yale Investments Office, pioneered an approach that relied on the aggressive use of extended diversification across a variety of asset classes: international markets, hedge funds, venture capital, private equity, real estate, and natural resources. Loosely termed “alternative investments,” these assets are all things that should be in the world wealth portfolio (Swensen 2000). At the time Swensen arrived at Yale in 1985, alternative investments were extremely understudied but held prospects of high returns to astute investors. He noted that within each of these asset classes, the performance spread between winning and losing managers was huge. To him, that phenomenon implied inefficient markets and the potential to add alpha by selecting good managers.
The Yale endowment model seemed, at first, both risky and revolutionary. For example, the risks of limited partnership investment in private capital and hedge funds were difficult to assess. No reliable return metrics were available for private capital, and extant hedge fund databases in the early 1990s suffered from significant biases.Footnote7 VAR did not capture the uncertainties of opaque strategies and structures that relied fundamentally on human skill and integrity.
Swensen and his team developed a risk management process that took into account statistical metrics but focused on personnel assessment and the possibility of operational risk. My co-authors and I found this perspective to be of first-order importance to fund survival (Brown, Goetzmann, Liang, and Schwarz 2009).
The Yale endowment approach, with its focus on alternative investments and the use of outside managers, has been widely emulated.Footnote8 Barber and Wang (2013) studied the performance of university endowments over a 21-year period and found that many followed strategies heavily emphasizing alternative investments. They found that Yale and other endowments of large universities did well by adopting this approach. The reason may be their capacity for maintaining a long-term perspective, which allows for purchasing and holding the less liquid investments.
Over its lifetime, the Financial Analysts Journal has been an important forum for research and discussion about alternative investments. For example, in the post–World War II period, non-US equities were a novelty, but in the 1950s, investment adviser Roman Gorski argued for international portfolio diversification to hedge geopolitical uncertainties (Gorski 1954) and Standard Oil’s investment adviser, T. R. Lilley, championed European stocks as diversifiers in the institutional portfolio (Lilley 1959). Later, Bruno Solnick (1974) pointed out the benefits of international diversification—a topic he had long studied academically.
Another example of an alternative asset discussed by contributors to the Financial Analysts Journal is commodities. Bodie and Rosansky (1980) provided one of the first reports of a rigorous study of commodity futures’ rates of return as an investment. Gorton and Rouwenhorst (2006) documented a premium similar to the equity premium over more than a half-century of commodity returns, and commodity returns had low correlations with equities. Their follow-up Bhardwaj, Gorton, and Rouwenhorst (2015) tracked the out-of-sample performance over a decade.
Similarly for private capital as an investment, the Financial Analysts Journal published a remarkable early empirical study of venture capital investing. Rotch (1968) documented the highly successful performance of a few major funds but noted that a “high degree of risk is inherent in the route they take to financial gain” (p. 147).
Further afield from financial assets but plainly relevant for such institutions as university endowments and museums are collectibles. Dimson and Spaenjers (2014) aggregated the evidence about the risk and return to investing in collectibles of many kinds—gold, stamps, art, and even violins. None beat stocks as to return, but all beat bills and bonds, albeit with considerable volatility. Moreover, holding and transactions costs were difficult to evaluate.
Hedge Funds.
Hedge funds are a key component of the endowment model. The Yale endowment has regularly allocated more than 20% to “absolute-return” strategies, the commonly used category for hedge funds. Conceptually, absolute-return strategies are the opposite of factor strategies. Their purpose is not to profit from exposure to systematic risk premiums but to capture mispricing; they focus on alpha, not beta. In 1966, hedge fund manager Martin Sosnoff provided Financial Analysts Journal readers with an excellent introduction to hedge funds (Sosnoff 1966):
A HEDGE FUND is a securities fund which not only buys stocks for long-term price appreciation but also sells stocks short. The concept of short selling is injected to reduce risk during periods of market decline. The emphasis is on maximizing stock market selection, i.e., buying stocks with above average prospects and selling short stocks which appear over-priced based upon investment judgment. The leverage of borrowed money is used to maximize capital gains. As the fund continually will be short a certain percentage of invested capital, a fully invested investment posture generally is maintained. Hopefully, the elimination of market risk will be attained by being long and short the market in varying proportions over a period of time. . . . (p. 105) The hedge fund manager either lives by the sword or dies by the sword. (p. 105)
Michael Steinhardt’s speech about hedge fund styles to the Financial Analysts Federation’s Investment Management Workshop at Princeton University on 19 July 1982 was reprinted in the Financial Analysts Journal (Steinhardt 1982). With the usual disclaimers, he touted Steinhardt Partners’ impressive track record: From 1967 to 1982, his fund had generated after-fee gains of 26.3%; most notably, the earnings were 27.8% in 1974, when the S&P 500 Index was down 38.1%.
When the Yale endowment model popularized the use of hedge funds, it reintroduced the original DNA of the Financial Analysts Journal to portfolio management: fundamental security analysis focused on valuation and identification of mispriced securities. Although some investors were astute enough to hire top hedge fund talent (like Michael Steinhardt) early on, not until the 1990s did hedge funds truly become an asset class. At that point, the trajectory from bottom-up investing to top-down asset allocation had come full circle. Steinhardt himself closed his fund in 1995 after huge losses in 1994—more than a decade after his speech at Princeton. Ironically, he went on to become chair of WisdomTree Investments, a “fundamental indexing” provider that focused on ETF factor investing.
The Financial Analysts Journal, together with the Journal of Portfolio Management, has continued to explore the merits and role of hedge funds in investment portfolios and in markets. A thorough review of the hedge fund literature—even just within the Financial Analysts Journal—would require an article of its own, but a few key points can be highlighted. As data quality improved, researchers began to provide useful quantitative metrics to investors. Liang (1999, 2001) was among the earliest empirical studies of hedge funds. Taking into account the crucial survivorship bias problem, he documented high Sharpe ratios for hedge funds over a five-year period to 1996. Malkiel and Saha (2005), using a different database and methodology, found less positive results. They argued that the survivorship problem was even bigger than formerly believed. The question of risk-adjusted hedge fund performance has continued and each year brings more data and a chance to study collective and individual performance (e.g., Ibbotson, Chen, and Zhu 2011).
Survivorship has been a central issue in measuring hedge fund performance, but another problem involves the proper measurement of absolute return. The first issue is how to adjust for exposure to systematic risk factors when a hedge fund has the freedom to pursue fleeting opportunities across a broad spectrum of securities and markets. The second issue is how to measure return when the expectation is that skill will derive from successful timing of investments. Fung and Hsieh (2002, 2004) addressed these issues by introducing dynamic risk factors estimated from the space of hedge fund returns themselves and represented by marketable assets. Their model included factors to capture hedge fund–specific behavior, such as trend following and merger arbitrage.
Since Fung and Hsieh’s landmark work, Financial Analysts Journal contributors have continued active debate about whether and how to control for systematic factor exposures. At issue is the question of whether returns come from skill or exposure to known risk premiums: If from skill, then hedge fund managers earn their 2% management and 20% incentive fees. If from exposure to known premiums, then presumably, such exposures could—and perhaps should—be replicated less expensively (Waring and Siegel 2006).
Conclusion
Asset management is one of society’s most important activities. It is a huge fiduciary responsibility to manage the assets on which the future livelihood of households and vital organizations rely. Without question, therefore, the science of portfolio management has made an important contribution to society. Asset management in the twenty-first century is an improvement over asset management of 100 years ago. More people all over the world have greater access to diversified, regulated, and thoughtfully constructed portfolios than ever before—and often, at a lower cost than before. The Financial Analysts Journal and related publications have played no small role in this development.
Society makes progress through institutions. Professional societies and focused journals are vital to this progress. The professional approach to asset management adopted by CFA Institute embraces the idea that fiduciary duty demands not only a mastery of established concepts and methods but also constant learning and sharing of knowledge. Although the adoption of new ideas and tools is difficult, the vehicle by which they are introduced, tested, and adapted makes a huge difference in the ease or difficulty of their spread. The editorial process sets the tone for the discourse around new ideas. To read through the contributions, letters, debates, and editors’ comments in the Financial Analysts Journal over the past 75 years is to trace the evolving technology of investment management—to see how it absorbs new knowledge, challenges it, tests it through application, and feeds these experiences and views back. This process has been well curated by the succession of Financial Analysts Journal editors, and the profession is the better for it.
Acknowledgments
I thank Stephen J. Brown, Elroy Dimson, Myra Drucker, Luis García-Feijóo, CFA, CIPM, Martin Gruber, Roger Ibbotson, Martin Liebowitz, Ranji Nagaswami, CFA, Geert Rouwenhorst, William Sharpe, Larry Siegel, Sandra Urie, CFA, and Paula Volent, CFA, for helpful comments.
Notes
1 Wassily Leontief (1906–1999) was awarded the Nobel Memorial Prize in Economic Sciences in 1973 for developing comprehensive input–output models of the US and the world economy. His approach has been widely used to understand the systemic propagation of demand and supply shocks through economies and the complex network of global production and trade that emerged in the 20th century.
2 See Elton, Gruber, and Padberg (1978); Latané and Tuttle (1967); and Sharpe (1972). These simplified algorithms are discussed at length in Chapter 6 of Elton, Gruber, Brown, and Goetzmann (2014).
3 The duality principle is the principle that optimization problems may be viewed from either the perspective of the primal problem or the dual problem. The solution to the dual problem provides a lower bound to the solution of the primal (minimization) problem.
4 See Fong and Vasicek (1997); Kritzman and Rich (2002); Duffie and Ziegler (2003).
5 “The results show that there are highly significant extra-market components of covariance among security returns; moreover, these risk components are such that the loadings of individual security returns on the factors are determined by observable characteristics of the firm: income statement and balance sheet data, industry membership, and historical behavior of returns on the security” (Rosenberg 1974), p. 263.
6 For Financial Analysts Journal articles on momentum, see Chan, Jegadeesh, and Lakonishok (1999). For value and momentum, see Asness (1997).
7 See Brown, Goetzmann, and Ibbotson (1999); Fung and Hsieh (2002); Aggarwal and Jorion (2010).
8 Yale’s endowment model may not have been the first of its kind. Chambers and Dimson (2015) showed that Swensen’s philosophy closely follows that of John Maynard Keynes—a visionary college endowment manager in his own right.
References
- Aggarwal, R., and P. Jorion. 2010. “Hidden Survivorship in Hedge Fund Returns.” Financial Analysts Journal, vol. 66, no. 2: 69–74 https://doi.org/10.2469/faj.v66.n2.1.
- Anderson, R. M., S. W. Bianchi, and L. R. Goldberg. 2012. “Will My Risk Parity Strategy Outperform?” Financial Analysts Journal, vol. 68, no. 6: 75–93 https://doi.org/10.2469/faj.v68.n6.7.
- Asness, C.. 1997. “The Interaction of Value and Momentum Strategies.” Financial Analysts Journal, vol. 53, no. 2: 29–36 https://doi.org/10.2469/faj.v53.n2.2069.
- Asness, C., A. Frazzini, and L. Pedersen. 2012. “Leverage Aversion and Risk Parity.” Financial Analysts Journal, vol. 68, no. 1: 47–59 https://doi.org/10.2469/faj.v68.n1.1.
- Barber, B. M., and G. Wang. 2013. “Do (Some) University Endowments Earn Alpha?” Financial Analysts Journal, vol. 69, no. 5: 26–44 https://doi.org/10.2469/faj.v69.n5.4.
- Baumol, W.. 1966. “Mathematical Analysis of Portfolio Selection: Principles and Application.” Financial Analysts Journal, vol. 22, no. 5: 95–99 https://doi.org/10.2469/faj.v22.n5.95.
- Beder, T. S.. 1995. “VAR: Seductive but Dangerous.” Financial Analysts Journal, vol. 51, no. 5: 12–24 https://doi.org/10.2469/faj.v51.n5.1932.
- Bhardwaj, G., G. Gorton, and G. Rouwenhorst. 2015. Facts and Fantasies about Commodity Futures Ten Years Later NBER Working Paper 21243 (June) https://doi.org/10.3386/w21243.
- Black, F.. 1972. “Capital Market Equilibrium with Restricted Borrowing.” Journal of Business, vol. 45, no. 3: 444–55 https://doi.org/10.1086/295472.
- Black, F.. 1975. “Fact and Fantasy in the Use of Options.” Financial Analysts Journal, vol. 31, no. 4: 36–41 https://doi.org/10.2469/faj.v31.n4.36.
- Black, F., M. C. Jensen, and M. Scholes. 1972. “The Capital Asset Pricing Model: Some Empirical Tests.” In Studies in the Theory of Capital Markets edited byJensen, M. C.: 79–121New York: Praeger.
- Black, F., and R. Litterman. 1991. “Asset Allocation: Combining Investor Views with Market Equilibrium.” Journal of Fixed Income, vol. 1, no. 2: 7–18.
- Black, F., and M. Scholes. 1972. “The Valuation of Option Contracts and a Test of Market Efficiency.” Journal of Finance, vol. 27, no. 2: 399–417 https://doi.org/10.2307/2978484.
- Black, F., and M. Scholes. 1973. “The Pricing of Options and Corporate Liabilities.” Journal of Political Economy, vol. 81, no. 3: 637–54 https://doi.org/10.1086/260062.
- Bodie, Z.. 1995. “On the Risk of Stocks in the Long Run.” Financial Analysts Journal, vol. 51, no. 3: 18–22 https://doi.org/10.2469/faj.v51.n3.1901.
- Bodie, Z., and V. I Rosansky. 1980. “Risk and Return in Commodity Futures.” Financial Analysts Journal, vol. 36, no. 3: 27–39.
- Bookstaber, R., and R. Clarke. 1985. “Problems in Evaluating the Performance of Portfolios with Options.” Financial Analysts Journal, vol. 41, no. 1: 48–62 https://doi.org/10.2469/faj.v41.n1.48.
- Bookstaber, R., and J. Gold. 1988. “In Search of the Liability Asset.” Financial Analysts Journal, vol. 44, no. 1: 70–80 https://doi.org/10.2469/faj.v44.n1.70.
- Breeden, D. T.. 1979. “An Intertemporal Asset Pricing Model with Stochastic Consumption and Investment Opportunities.” Journal of Financial Economics, vol. 7, no. 3: 133–38 https://doi.org/10.1016/0304-405X(79)90016-3.
- Brinson, G. P., L. R. Hood, and G. L. Beebower. 1986. “Determinants of Portfolio Performance.” Financial Analysts Journal, vol. 42, no. 4: 39–44.
- Brinson, G. P., B. D. Singer, and G. L. Beebower. 1991. “Determinants of Portfolio Performance II: An Update.” Financial Analysts Journal, vol. 47, no. 3: 40–48 https://doi.org/10.2469/faj.v47.n3.40.
- Brown, S. J.. 2020. “The Efficient Market Hypothesis, the Financial Analysts Journal, and the Professional Status of Investment Management.” Financial Analysts Journal, vol. 76, no. 2: 5–14.
- Brown, S. J., W. N. Goetzmann, and R. G. Ibbotson. 1999. “Offshore Hedge Funds: Survival and Performance, 1989–95.” Journal of Business, vol. 76, no. 2: 91–117 https://doi.org/10.1086/209603.
- Brown, S., W. Goetzmann, B. Liang, and C. Schwarz. 2009. “Estimating Operational Risk for Hedge Funds: The ω-Score.” Financial Analysts Journal, vol. 65, no. 1: 43–53 https://doi.org/10.2469/faj.v65.n1.8.
- Carleton, W., and J. Lakonishok. 1985. “Risk and Return on Equity: The Use and Misuse of Historical Estimates.” Financial Analysts Journal, vol. 41, no. 1: 38–4762 https://doi.org/10.2469/faj.v41.n1.38.
- Carter, W. D.. 1950. “The Quality of Mutual Fund Portfolio Supervision.” Financial Analysts Journal, vol. 6, no. 4: 32–36 https://doi.org/10.2469/faj.v6.n4.32.
- Chambers, D., and E. Dimson. 2015. “The British Origins of the US Endowment Model.” Financial Analysts Journal, vol. 71, no. 2: 10–14 https://doi.org/10.2469/faj.v71.n2.7.
- Chan, L. K. C., N. Jegadeesh, and J. Lakonishok. 1999. “The Profitability of Momentum Strategies.” Financial Analysts Journal, vol. 55, no. 6: 80–90.
- Chen, N. F., R. Roll, and S. A. Ross. 1986. “Economic Forces and the Stock Market.” Journal of Business, vol. 59, no. 3: 383–403 https://doi.org/10.1086/296344.
- Clarke, R., and Robert D. Arnott. 1987. “The Cost of Portfolio Insurance: Tradeoffs and Choices.” Financial Analysts Journal, vol. 43, no. 6: 35–4766 https://doi.org/10.2469/faj.v43.n6.35.
- Connor, G. 1995. “The Three Types of Factor Models: A Comparison of Their Explanatory Power.” Financial Analysts Journal, vol. 51, no. 3: 42–46 https://doi.org/10.2469/faj.v51.n3.1904.
- Connor, G., and R. A. Korajczyk. 1988. “Risk and Return in an Equilibrium APT: Application of a New Test Methodology.” Journal of Financial Economics, vol. 21, no. 2: 255–89 https://doi.org/10.1016/0304-405X(88)90062-1.
- Cox, J. C., S. A. Ross, and M. Rubinstein. 1979. “Option Pricing: A Simplified Approach.” Journal of Financial Economics, vol. 7, no. 3: 229–63 https://doi.org/10.1016/0304-405X(79)90015-1.
- Dimson, E.. 1977a. “Instant Option Valuation.” Financial Analysts Journal, vol. 33, no. 3: 62–69 https://doi.org/10.2469/faj.v33.n3.62.
- Dimson, E.. 1977b. “Option Valuation Nomograms.” Financial Analysts Journal, vol. 33, no. 6: 71–74 https://doi.org/10.2469/faj.v33.n6.71.
- Dimson, E., P. Marsh, and M. Staunton. 2004. “Irrational Optimism.” Financial Analysts Journal, vol. 60, no. 1: 15–25 https://doi.org/10.2469/faj.v60.n1.2589.
- Dimson, E., and C. Spaenjers. 2014. “Investing in Emotional Assets.” Financial Analysts Journal, vol. 70, no. 2: 20–25 https://doi.org/10.2469/faj.v70.n2.8.
- Doeswijk,, R., T. Lam, and L. Swinkels. 2014. “The Global Multi-Asset Market Portfolio, 1959–2012.” Financial Analysts Journal, vol. 70, no. 2: 26–41.
- Duffie, D., and A. Ziegler. 2003. “Liquidation Risk.” Financial Analysts Journal, vol. 59, no. 3: 42–51 https://doi.org/10.2469/faj.v59.n3.2530.
- Dybvig, P.. 1999. “Using Asset Allocation to Protect Spending.” Financial Analysts Journal, vol. 55, no. 1: 49–62 https://doi.org/10.2469/faj.v55.n1.2241.
- Ellis, C. D.. 2015. “In Defense of Active Investing.” Financial Analysts Journal, vol. 71, no. 4: 4–7 https://doi.org/10.2469/faj.v71.n4.5.
- Elton, E. J., M. J. Gruber, S. J. Brown, and W. N. Goetzmann. 2014. Modern Portfolio Theory and Investment Analysis9th ed.Hoboken, NJ: John Wiley and Sons.
- Elton, E. J., M. J. Gruber, and M. W. Padberg. 1978. “Optimal Portfolios from Simple Ranking Devices.” Journal of Portfolio Management, vol. 4, no. 3: 15–19.
- Estep, T., and M. Kritzman. 1988. “TIPP: Insurance without Complexity.” Journal of Portfolio Management, vol. 14, no. 4: 38–42 https://doi.org/10.3905/jpm.1988.409172.
- Ezra, D.. 1991. “Asset Allocation by Surplus Optimization.” Financial Analysts Journal, vol. 47, no. 1: 51–57 https://doi.org/10.2469/faj.v47.n1.51.
- Fama, E. F., and K. R. French. 1992. “The Cross‐Section of Expected Stock Returns.” Journal of Finance, vol. 47, no. 2: 427–65.
- Fan, J., Y. Fan, and J. Lv. 2008. “High Dimensional Covariance Matrix Estimation Using a Factor Model.” Journal of Econometrics, vol. 47, no. 1: 186–97 https://doi.org/10.1016/j.jeconom.2008.09.017.
- Fisher, G. H.. 1953. “The Input-Output Technique as an Aid in Security Analysis.” Financial Analysts Journal, vol. 9, no. 1: 85–92 https://doi.org/10.2469/faj.v9.n1.85.
- Fisher, L.. 1975. “Using Modern Portfolio Theory to Maintain an Efficiently Diversified Portfolio.” Financial Analysts Journal, vol. 31, no. 3: 73–85 https://doi.org/10.2469/faj.v31.n3.73.
- Fong, G., and O. A. Vasicek. 1997. “A Multidimensional Framework for Risk Analysis.” Financial Analysts Journal, vol. 53, no. 4: 51–57 https://doi.org/10.2469/faj.v53.n4.2099.
- Frazzini, A., and L. Pedersen. 2010. “Betting against Beta.” NBER Working Paper No. 16601 (December).
- Fung, W., and D. A. Hsieh. 2002. “Asset-Based Style Factors for Hedge Funds.” Financial Analysts Journal, vol. 58, no. 5: 16–27 https://doi.org/10.2469/faj.v58.n5.2465.
- Fung, W., and D. A. Hsieh. 2004. “Hedge Fund Benchmarks: A Risk-Based Approach.” Financial Analysts Journal, vol. 60, no. 5: 65–80 https://doi.org/10.2469/faj.v60.n5.2657.
- Gorski, R.. 1954. “Investment Security in an Uncertain World.” Financial Analysts Journal, vol. 10, no. 4: 33–36 https://doi.org/10.2469/faj.v10.n4.33.
- Gorton, G., and K. Rouwenhorst. 2006. “Facts and Fantasies about Commodity Futures.” Financial Analysts Journal, vol. 62, no. 2: 47–68 https://doi.org/10.2469/faj.v62.n2.4083.
- Graham, B.. 1946. “On Being Right in Security Analysis.” Analysts Journal, vol. 2, no. 1: 18–21 https://doi.org/10.2469/faj.v2.n1.18.2.
- Graham, B.. 1952. “Toward a Science of Security Analysis.” Analysts Journal, vol. 8, no. August: 97–99.
- Graham, B.. 1963. “The Future of Financial Analysis.” Financial Analysts Journal, vol. 19, no. 3: 65–70 https://doi.org/10.2469/faj.v19.n3.65.
- Hakansson, N.. 1976. “The Purchasing Power Fund: A New Kind of Financial Intermediary.” Financial Analysts Journal, vol. 32, no. 6: 49–59 https://doi.org/10.2469/faj.v32.n6.49.
- Hood, L.. 2005. “Determinants of Portfolio Performance: 20 Years Later.” Financial Analysts Journal, vol. 61, no. 5: 6–8 https://doi.org/10.2469/faj.v61.n5.2750.
- Ibbotson, R., Z. Chen, D. Kim, and W. Hu. 2013. “Liquidity as an Investment Style.” Financial Analysts Journal, vol. 69, no. 3: 30–44 https://doi.org/10.2469/faj.v69.n3.4.
- Ibbotson, R. G., P. Chen, and K. X. Zhu. 2011. “The ABCs of Hedge Funds: Alphas, Betas, and Costs.” Financial Analysts Journal, vol. 67, no. 1: 15–25 https://doi.org/10.2469/faj.v67.n1.6.
- Ibbotson, R. G., and P. D. Kaplan. 2000. “Does Asset Allocation Policy Explain 40, 90, or 100 Percent of Performance?” Financial Analysts Journal, vol. 56, no. 1: 26–33 https://doi.org/10.2469/faj.v56.n1.2327.
- Ibbotson, R., and L. B. Siegel. 1983. “The World Market Wealth Portfolio.” Journal of Portfolio Management, vol. 9, no. 2: 5–17.
- Ibbotson, R., and R. Sinquefield. 1979. “Stocks, Bonds, Bills and Inflation: Updates.” Financial Analysts Journal, vol. 35, no. 4: 40–44 https://doi.org/10.2469/faj.v35.n4.40.
- Jenks, J.. 1947. “Investing in Growth Stock.” Analysts Journal, vol. 3, no. 2: 38–53 https://doi.org/10.2469/faj.v3.n2.38.
- Jorion, P. 1986. “Bayes-Stein Estimation for Portfolio Analysis.” Journal of Financial and Quantitative analysis, vol. 21, no. 3: 279–292.
- Jorion, P.. 1992. “Portfolio Optimization in Practice.” Financial Analysts Journal, vol. 48, no. 1: 68–74 https://doi.org/10.2469/faj.v48.n1.68.
- Jorion, P.. 1996. “Risk2: Measuring the Risk in Value at Risk.” Financial Analysts Journal, vol. 52, no. 6: 47–56 https://doi.org/10.2469/faj.v52.n6.2039.
- Jorion, P.. 2006. Value at Risk: The New Benchmark for Managing Financial Risk3rd edNew York: McGraw-Hill.
- Kahn, I.. 2005. “Early Days at the Financial Analysts Journal.” Financial Analysts Journal, vol. 61, no. 2: 6–7 https://doi.org/10.2469/faj.v61.n2.2705.
- Kritzman, M., and D. Rich. 2002. “The Mismeasurement of Risk.” Financial Analysts Journal, vol. 58, no. 3: 91–99 https://doi.org/10.2469/faj.v58.n3.2541.
- Latané, H. A., and D. L. Tuttle. 1967. “Criteria for Portfolio Building.” Journal of Finance, vol. 22, no. 3: 359–73 https://doi.org/10.1111/j.1540-6261.1967. https://doi.org/10.1111/j.1540-6261.1967.tb02973.x.
- Leibowitz, M.. 1986. “Total Portfolio Duration: A New Perspective on Asset Allocation.” Financial Analysts Journal, vol. 42, no. 5: 18–29 https://doi.org/10.2469/faj.v42.n5.18.
- Leibowitz, M.. 1987. “Pension Asset Allocation through Surplus Management.” Financial Analysts Journal, vol. 43, no. 2: 29–40 https://doi.org/10.2469/faj.v43.n2.29.
- Leibowitz, M., and R. Henriksson. 1988. “Portfolio Optimization within a Surplus Framework.” Financial Analysts Journal, vol. 44, no. 2: 43–51 https://doi.org/10.2469/faj.v44.n2.43.
- Leibowitz, M., and S. Kogelman. 1993. “Resolving the Equity Duration Paradox.” Financial Analysts Journal, vol. 49, no. 1: 51–64 https://doi.org/10.2469/faj.v49.n1.51.
- Liang, B.. 1999. “On the Performance of Hedge Funds.” Financial Analysts Journal, vol. 55, no. 4: 72–85 https://doi.org/10.2469/faj.v55.n4.2287.
- Liang, B.. 2001. “Hedge Fund Performance: 1990–1999.” Financial Analysts Journal, vol. 57, no. 1: 11–18 https://doi.org/10.2469/faj.v57.n1.2415.
- Lilley, T. R.. 1959. “European Equities for the American Pension Fund.” Financial Analysts Journal, vol. 15, no. 3: 37–39 https://doi.org/10.2469/faj.v15.n3.37.
- Linsmeier, T., and N. Pearson. 2000. “Value at Risk.” Financial Analysts Journal, vol. 56, no. 2: 47–67 https://doi.org/10.2469/faj.v56.n2.2343.
- Malkiel, B. G., and A. Saha. 2005. “Hedge Funds: Risk and Return.” Financial Analysts Journal, vol. 61, no. 6: 80–88 https://doi.org/10.2469/faj.v61.n6.2775.
- Markowitz, H.. 1952. “Portfolio Selection.” Journal of Finance, vol. 7, no. 1: 77–91.
- Markowitz, H.. 1976. “Markowitz Revisited.” Financial Analysts Journal, vol. 32, no. 5: 47–52 https://doi.org/10.2469/faj.v32.n5.47.
- Markowitz, H.. 1999. “The Early History of Portfolio Theory: 1600–1960.” Financial Analysts Journal, vol. 55, no. 4: 5–16 https://doi.org/10.2469/faj.v55.n4.2281.
- McLaughlin, T.. 2007. “Eyes Wide Shut: Exchange Traded Funds, Index Arbitrage, and the Need for Change.” Review of Banking and Financial Law, vol. 27, no. 2: 597–623.
- Merton, R. C.. 1971. “Optimum Consumption and Portfolio Rules in a Continuous-Time Model.” Journal of Economic Theory, vol. 3, no. 4: 373–413 https://doi.org/10.1016/0022-0531(71)90038-X.
- Merton, R. C.. 1973a. “An Intertemporal Capital Asset Pricing Model.” Econometrica, vol. 41, no. 5: 867–87 https://doi.org/10.2307/1913811.
- Merton, R. C.. 1973b. “Theory of Rational Option Pricing.” Bell Journal of Economics and Management Science, vol. 4, no. 1: 141–83 https://doi.org/10.2307/3003143.
- Michaud, R.. 1989. “The Markowitz Optimization Enigma: Is ‘Optimized’ Optimal?” Financial Analysts Journal, vol. 45, no. 1: 31–42 https://doi.org/10.2469/faj.v45.n1.31.
- Milne, R. D.. 1958. “Benchmarks for a Diversified Portfolio.” Financial Analysts Journal, vol. 14, no. 5: 51–54 https://doi.org/10.2469/faj.v14.n5.51.
- Molodovsky, N.. 1953. “Some Aspects of Price–Earnings Ratios.” Analysts Journal, vol. 9, no. 2: 65–78 https://doi.org/10.2469/faj.v9.n2.65.
- Perold, A.. 1986. Constant Proportion Portfolio Insurance Working paper (August).
- Perold, A., and W. Sharpe. 1988. “Dynamic Strategies for Asset Allocation.” Financial Analysts Journal, vol. 44, no. 1: 16–27 https://doi.org/10.2469/faj.v44.n1.16.
- Renshaw, E., and P. Feldstein. 1960. “The Case for an Unmanaged Investment Company.” Financial Analysts Journal, vol. 16, no. 1: 43–46 https://doi.org/10.2469/faj.v16.n1.43.
- Roll, R.. 1977. “A Critique of Asset Pricing Theory’s Tests, Part I: On Past and Potential Testability of the Theory.” Journal of Financial Economics, vol. 4, no. 2: 129–76.
- Roll, R.. 1988. “The International Crash of October 1987.” Financial Analysts Journal, vol. 44, no. 5: 19–35.
- Roll, R., and S. A. Ross. 1980. “An Empirical Investigation of the Arbitrage Pricing Theory.” Journal of Finance, vol. 35, no. 5: 1073–103 .
- Roll, R., and S. A. Ross. 1984. “The Arbitrage Pricing Theory Approach to Strategic Portfolio Planning.” Financial Analysts Journal, vol. 40, no. 3: 14–26.
- Rosenberg, B.. 1974. “Extra-Market Components of Covariance in Security Returns.” Journal of Financial and Quantitative Analysis, vol. 9, no. 2: 263–74 https://doi.org/10.2307/2330104.
- Rosenberg, B.. 1982. “The Current State and Future of Investment Research.” Financial Analysts Journal, vol. 38, no. 1: 43–50 https://doi.org/10.2469/faj.v38.n1.43.
- Ross, Stephen A.. 1972. “Portfolio and Capital Market Theory with Arbitrary Preferences and Distributions: The General Validity of the Mean-Variance Approach.” In Large Markets (No. 12-72). Philadelphia, PA: University of Pennsylvania, Wharton School Rodney L. White Center for Financial Research.
- Ross, S.. 1976a. “The Arbitrage Theory of Capital Asset Pricing.” Journal of Economic Theory, vol. 13, no. 3: 341–60 https://doi.org/10.1016/0022-0531(76)90046-6.
- Ross, S.. 1976b. “Return, Risk, and Arbitrage.” In Risk and Return in Finance edited byFriend, I., and J. BickslerCambridge, MA: Ballinger.
- Rotch, W.. 1968. “The Pattern of Success in Venture Capital Financing.” Financial Analysts Journal, vol. 24, no. 5: 141–47 https://doi.org/10.2469/faj.v24.n5.141.
- Roy, A.D.. 1952. “Safety First and the Holding of Assets.” Econometrica, vol. 20, no. 3: 431–449.
- Rubinstein, M.. 1985. “Alternative Paths to Portfolio Insurance.” Financial Analysts Journal, vol. 41, no. 4: 42–52 https://doi.org/10.2469/faj.v41.n4.42.
- Rubinstein, M.. 1988. “Portfolio Insurance and the Market Crash.” Financial Analysts Journal, vol. 44, no. 1: 38–47 https://doi.org/10.2469/faj.v44.n1.38.
- Rubinstein, M.. 1989. “Market Basket Alternatives.” Financial Analysts Journal, vol. 45, no. 5: 20–29 https://doi.org/10.2469/faj.v45.n5.20.
- Rubinstein, M., and H. Leland. 1981. “Replicating Options with Positions in Stock and Cash.” Financial Analysts Journal, vol. 37, no. 4: 63–72 https://doi.org/10.2469/faj.v37.n4.63.
- Sénéchal, E., and B. Singer. 2010. “‘The Equal Importance of Asset Allocation and Active Management’: A Comment.” Financial Analysts Journal, vol. 66, no. 4: 16–17 https://doi.org/10.2469/faj.v66.n4.12.
- Sharpe, W.. 1963. “A Simplified Model for Portfolio Analysis.” Management Science, vol. 9, no. 2: 277–93 https://doi.org/10.1287/mnsc.9.2.277.
- Sharpe, W. F.. 1972. “Simple Strategies for Portfolio Diversification: Comment.” Journal of Finance, vol. 27, no. 1: 127–29 https://doi.org/10.1111/j.1540-6261.1972.tb00628.x.
- Sharpe, W. F.. 1974. “Imputing Expected Security Returns from Portfolio Composition.” Journal of Financial and Quantitative Analysis, vol. 9, no. 3: 463–72 https://doi.org/10.2307/2329873.
- Sharpe, W. F.. 2010. “Adaptive Asset Allocation Policies.” Financial Analysts Journal, vol. 66, no. 3: 45–59 https://doi.org/10.2469/faj.v66.n3.3.
- Sharpe, W. F., and G. M. Cooper. 1972. “Risk–Return Classes of New York Stock Exchange Common Stocks, 1931–1967.” Financial Analysts Journal, vol. 28, no. 2: 46–5481 https://doi.org/10.2469/faj.v28.n2.46.
- Shelton, J. P.. 1966. “The New Research and the Investment Profession.” Financial Analysts Journal, vol. 22, no. 6: 65–67 https://doi.org/10.2469/faj.v22.n6.65.
- Siegel, J. J.. 1992. “The Equity Premium: Stock and Bond Returns since 1802.” Financial Analysts Journal, vol. 48, no. 1: 28–38.
- Siegel, L.. 2010. “Risk Parity: Classical Finance Properly Implemented, or Misunderstood?” Financial Analysts Journal, vol. 66, no. 5: 15–16 https://doi.org/10.2469/faj.v66.n5.12.
- Solnik, B.. 1974. “Why Not Diversify Internationally Rather than Domestically?” Financial Analysts Journal, vol. 30, no. 4: 48–54 https://doi.org/10.2469/faj.v30.n4.48.
- Sosnoff, M. T.. 1966. “Hedge Fund Management: A New Respectability for Short Selling.” Financial Analysts Journal, vol. 22, no. 4: 105–08 https://doi.org/10.2469/faj.v22.n4.105.
- Steinhardt, M. H.. 1982. “Investing, Hedge-Fund Style.” Financial Analysts Journal, vol. 38, no. 6: 33–35 https://doi.org/10.2469/faj.v38.n6.33.
- Sullivan, R.. 2010. “‘Speculative Leverage: A False Cure for Pension Woes’: Author Response.” Financial Analysts Journal, vol. 66, no. 4: 14–16 https://doi.org/10.2469/faj.v66.n4.11.
- Swensen, D. F.. 2000. Pioneering Portfolio Management: An Unconventional Approach to Institutional Investment. New York:: Simon and Schuster.
- Treynor, J., and F. Black. 1973. “How to Use Security Analysis to Improve Portfolio Selection.” Journal of Business, vol. 46, no. 1: 66–86 https://doi.org/10.1086/295508.
- Vardharaj, R., and F. Fabozzi. 2007. “Sector, Style, Region: Explaining Stock Allocation Performance.” Financial Analysts Journal, vol. 63, no. 3: 59–70 https://doi.org/10.2469/faj.v63.n3.4691.
- Waring, M. B., and L. B. Siegel. 2006. “The Myth of the Absolute-Return Investor.” Financial Analysts Journal, vol. 62, no. 2: 14–21 https://doi.org/10.2469/faj.v62.n2.4080.
- Xiong, J., R. Ibbotson, T. Idzorek, and P. Chen. 2010. “‘The Equal Importance of Asset Allocation and Active Management’: Author Response.” Financial Analysts Journal, vol. 66, no. 4: 17–18 https://doi.org/10.2469/faj.v66.n4.13.