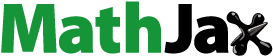
Abstract
This study identifies the indicators of sector-level time-series predictability. The results show that investors can expect higher predictability in the more volatile sectors. In the developed markets, price downtrends, lower trading volume, and higher dividend yields indicate stronger predictability. The cyclical and sensitive super-sectors become more predictable as liquidity goes down. Particularly in the cyclical super-sectors, smaller market capitalization and larger term spread also indicate predictability. Sector selection based on the indicators can generate economic benefits.
PL Credits: 2.0:
Introduction
In the investment process, identifying economic sectors and industries that are expected to gain more than the overall markets is of great importance (Beller, Kling, and Levinson Citation1998). Investors may go further by choosing individual securities but may simply hold industry portfolios, funds, or sector exchange-traded funds. This investing strategy, generally known as “sector investing,” seeks a balance between passive index investing and active stock selection (Alexiou and Tyagi Citation2020). Sector investing is not only about selecting the best industry in which to invest but also about rotating sectors based on geographical locations or business cycles. It requires industry analysis, which has many aspects, such as examining fundamentals and the industry’s environment. Often ignored, however, is how well investors can predict future stock returns in a specific industry sector.
One of the predictors, which has been widely tested and is among the simplest, is stock price itself—specifically, past and current returns. The existence of this time-series predictability has been strongly supported by studies of financial markets in the past few decades (Yen and Lee Citation2008; Lim and Brooks Citation2011) as has rejection of the weak form of market efficiency (e.g., Narayan and Smyth Citation2015; Fama Citation1991). Time-series predictability fits many behavioral and rational pricing theories that are based on a single risky asset (Moskowitz, Ooi, and Pedersen Citation2012). Predictability that uses all nonprice public information is often denoted “cross-sectional predictability” (McLean and Pontiff Citation2016). Several factors have been tested for their predictive power, with financial ratios, such as dividend yield and the price-to-earnings ratio, being among the most commonly tested (Rapach, Strauss, and Zhou Citation2010; Campbell and Thompson Citation2008; Welch and Goyal Citation2008; Campbell and Shiller Citation1988). And some researchers have tested combinations of predictors to extract more information than is available from only a single predictor (Dai and Zhu Citation2020; Zhang et al. Citation2019; Jordan, Vivian, and Wohar Citation2014; Timmermann Citation2006).
Sector-level analysis of predictability, however, is often overlooked even in the more common studies of cross-sectional predictability. Yet, sector-level predictability can be stronger than market-level predictability (Bannigidadmath and Narayan Citation2016) and can differ across industry sectors; that is, some sectors are more predictable than others (Phan, Sharma, and Narayan Citation2015). The reason could be that investors receive more valuable pricing information in certain industries or that information possession and processing are heterogeneous across industries (Bannigidadmath and Narayan Citation2016; Westerlund and Narayan Citation2015a).
Sector-level predictability is important because, in practice, investors do not hold well-diversified portfolios. Household investors tend to hold undiversified portfolios that target a few industries, not only because of behavioral biases but also because of financial constraints and limited borrowing opportunities (Roche, Tompaidis, and Yang Citation2013). Mutual funds also hold portfolios concentrated in industries when they believe certain sectors will outperform or the funds have superior information about specific industries (Kacperczyk, Sialm, and Zheng Citation2005). Narayan, Ahmed, and Narayan (Citation2017) show that investors will benefit greatly from investing in certain sectors—such as technology and financials in the United States. Also, fund managers’ industry selection skills are known to drive their relative performance (Busse and Tong Citation2012), and funds that are more industry-concentrated perform better than less concentrated ones (Kacperczyk, Sialm, and Zheng Citation2005). As for portfolio diversification, seeking diversification across global sectors is important when global markets tend to be integrated (Cavaglia, Brightman, and Aked Citation2000). Industry-wide categorization is also known to influence the investment decisions of retail investors and thus affect stock prices (Jame and Tong Citation2014).
If investors who pursue sector investing want to identify sectors where they can best predict future returns, they need to understand how certain industry factors or characteristics are associated with sector-level predictability. For example, Westerlund and Narayan (Citation2015a) show that relative valuation measures, such as the book-to-market ratio, can explain sector-level predictability. Risk premiums can also vary at the sector level, as seen in the studies using the Fama–French three-factor model (Rapach et al. Citation2011) and the capital asset pricing model (Narayan and Bannigidadmath Citation2015).
Understanding sector-level predictability is not an easy task, however, when return predictability is expected to be time-varying. Finding predictors and models that can improve on even the most naive benchmark forecasting models in out-of-sample periods is difficult (Welch and Goyal Citation2008) because the degree of market efficiency is known to change over time (Lim and Brooks Citation2011). Investors’ behavior responds to varying market conditions too (Lo Citation2004). Risk factors in pricing models could vary with the business cycle because risk-averse investors could demand higher risk premiums during crisis periods (Guiso, Sapienza, and Zingales Citation2018; Fama and French Citation1989; Campbell and Cochrane Citation1999).
Most past studies of time-varying predictability examine cross-sectional predictability and determinants, such as financial ratios (Bannigidadmath and Narayan Citation2016; Kim, Shamsuddin, and Lim Citation2011; Timmermann Citation2008; Paye and Timmermann Citation2006). The time variability of time-series predictability, however, is rarely investigated. Time-series predictability is usually assumed to be unchanged in the weak-form efficiency literature, but Moskowitz, Ooi, and Pedersen (Citation2012) emphasize the importance of time-series predictability. They also highlight that cross-sectional and time-series predictability are related and that the underlying driver of the changes is time-series predictability. Some studies trace the time variability of time-series predictability to market-level factors (Lim and Brooks Citation2011; Lagoarde-Segot Citation2009) but not to sector-level factors.
Therefore, our study has three main objectives. First, we investigate whether time-series predictability exists and how it differs across industry sectors and over time. Next, we examine which sector-level characteristics can indicate the degree of time-series predictability. Note that the indicators of time-series predictability are not the same as the predictors of returns. Indicators of time-series predictability signify the degree to which future stock returns can be forecasted by past and current returns, but predictors of returns attempt to predict future stock returns. Finally, we evaluate the economic significance of time-series predictability for investment management, particularly for sector investing, in the form of gains in predictability and investment returns.
Many studies have not considered the type of investors who benefit from predictability. Investors are commonly assumed to have access to a large amount of public information and thus to be able to exploit cross-sectional predictability. In reality, few investors seem to be capable of taking advantage of this predictability (Cooper and Gulen Citation2006) despite academic evidence of exploitation (McLean and Pontiff Citation2016). Also, certain types of investor may need to, or be willing to, pursue time-series predictability instead of cross-sectional predictability.
In this regard, our study focuses on the type of investor who, unlike the rational investor of classical finance, does not use full information. Following Barberis and Thaler (Citation2005), we call these investors “less rational investors,” but because Barberis and Thaler find many possible departures from rationality, a further simplifying choice is required to make the subsequent analysis manageable. Therefore, we assume that less rational investors use only past price history for forecasting but know certain contemporaneous sector fundamentals for sector selection. In addition, they do their best by combining the forecasts from univariate models and updating their models under changing market circumstances, similar to those in Lo’s (Citation2004) adaptive markets hypothesis. The benefit of adopting less rational investors for this study is that the predictability of their forecasting models will tend to be conservative and not be overstated because of the limited information. Also, our findings can be generalized to more rational or “able” investors.
In essence, this study aims to identify the indicators of sector-level time-series predictability for less rational investors’ sector investing. We use a two-step approach inspired by the multistep methods of Phan, Sharma, and Narayan (Citation2015), Wang et al. (Citation2018), and Devpura, Narayan, and Sharma (Citation2018). We measure time-series predictability in each sector and then identify the indicators of the times-series predictability by a panel regression model. Our technical approach is distinct from these authors’ approaches. The main difference in the first step is that we measure predictability by less rational investors’ best forecasting accuracy in terms of out-of-sample forecasting errors, instead of examining the direct link between returns and one-step backward predictors commonly found in the studies of cross-sectional predictability. Therefore, we essentially are studying out-of-sample predictability, an idea shared with Kong et al. (Citation2011). We can more easily propose a practical approach that would help investors where in-sample evidence is irrelevant (Rapach et al. Citation2011; Welch and Goyal Citation2008). In the second step, we analyze the dynamic panels of sector-level indicators and predictivity rather than testing each predictor in a time-series setup (Devpura, Narayan, and Sharma Citation2018; Phan, Sharma, and Narayan Citation2015) or building component portfolios on indicators (Kong et al. Citation2011). Specifically, we adopt the dynamic common correlated effects estimator. It controls for cross-sectional dependence, which potentially exists across sectors and markets, while fully using long time series and large cross-sections.
We explore two more dimensions—market development and super-sectors. On the one hand, the emerging markets are expected to be more predictable than the developed markets (Harvey Citation1995) because of lower liquidity (Acharya and Pedersen Citation2005) and less established market microstructures, such as a lack of trading system automation, insider trading regulations, and accounting standardization (Lagoarde-Segot Citation2009). On the other hand, they are guided more by heterogeneous local information (Harvey Citation1995), are less correlated with the global markets (Berger, Pukthuanthong, and Yang Citation2011; Zaremba et al. Citation2021), and are often subject to financial reforms. Therefore, predictability in the emerging markets is likely to be more time-varying than in the established markets. Super-sectors, which Morningstar (Citation2010) defines as cyclical, defensive, and sensitive, are classified according to their sensitivity to the business cycle. Return predictability may vary across the super-sectors as the markets ride the business cycle.
Our findings show that sector-level time-series predictability is not strong and was not affected by the COVID-19 pandemic but varies across sectors and market development. Certain indicators can imply stronger time-series predictability for less rational investors. For example, in general, more volatile sectors are more predictable. In the developed markets, price downtrends and lower trading volume but higher dividend yield indicate stronger predictability. The emerging markets do not have such relationships despite their higher level of predictability. Super-sectors, which are sensitive to the business cycles, become more predictable as liquidity decreases. In the cyclical super-sectors, price downtrends, small market size, and larger term spreads also indicate predictability.
Our indicators successfully indicate the existence of sectors with stronger time-series predictability in out-of-sample periods. Exploiting higher predictability for higher profits, however, is not easy. Among the sector-level buy-and-hold strategies we test, we find that those based on market size, illiquidity, and term spread lead to consistently higher returns than the market.
This study makes several important contributions. First, it provides practical and forward-looking indicators for investors who seek predictable sectors. In those sectors, they can potentially generate more profits from their trading strategies than in the other sectors. Second, this study demonstrates a new perspective on measuring predictability. We first theoretically define a type of investor and then adopt their forecasting performance as predictability. Our approach bypasses the potential issue of specifying a certain structure between returns and predictors. Third, we show sector-level heterogeneity in time-series predictability by investigating multiple dimensions: sectors, super-sectors, and stage of market development. As a result, more applications can be devised in sector investing than with market-level investing. Finally, our study examines the impact of the COVID-19 pandemic on time-series predictability. It complements the recent strand of literature on cross-sectional predictability (see, among others, Ciner Citation2021; Hasan Citation2022; Ma et al. Citation2022; Naidu and Ranjeeni Citation2021; Rahman, Amin, and Al Mamun Citation2021; Salisu and Vo Citation2020).
Methods
In this section, we discuss how we measure time-series predictability, identify the indicators of such predictability, select candidate indicators, and test for the economic significance of sector-level predictability.
Measuring Time-Series Predictability
We measure time-series predictability by the forecasting performance of less rational investors on a rolling-window basis. The rolling-window method is a popular method of examining the evolution of market efficiency. According to a survey paper by Lim and Brooks (Citation2011), there are three research frameworks for dealing with time-varying market efficiency or predictability: subperiod analysis, time-varying parameter models, and rolling estimation windows. First, we do not use subperiod analysis because it assumes that the model parameters change at only a few predetermined points. In other words, it cannot provide a sufficient number of observations to model the time variability of predictability. In contrast, the time-varying parameter model allows the model parameters to change through time and thus captures the changes in predictability. However, it requires a strong assumption about how returns are generated or related to the predictors, such as state–space or autoregressive models (see, e.g., Dangl and Halling Citation2012). Our approach of measuring predictability by forecasting accuracy via rolling windows does not require either return generation processes or forecasting models to be always correct.
In our method, each rolling window is approximately one year long: 250 trading days. The investors observe daily stock returns in the previous 200 days (the estimation period) and then produce daily forecasts by using multiple forecasting models. They use the intermediate 25 days (the training period) to calculate the weights for combining forecasts where necessary and then forecast future returns for the next 25 days. These last 25 days (the evaluation period) are used to evaluate forecasting errors and performance. The accuracy of the best forecasts in each rolling window is converted to one corresponding value of time-series predictability. The sizes of these periods are inspired by Moskowitz, Ooi, and Pedersen (Citation2012), who find that a relationship between lagged one-year returns and the following month’s returns is the dominant force behind time-series predictability.
Then, we move the rolling window by 25 days, about one calendar month, and repeat the process until we obtain a monthly series of time-series predictability. We repeat the process for each sample sector and market. Eventually, we have panel data on monthly predictability.
We prefer the rolling-window method to the alternative expanding (or recursive) window method because the rolling-window method better represents less rational investors’ use of limited information.
Note that estimation periods partially overlap. Overlapping observations can incorporate more information and lower the variance of estimates but potentially incur autocorrelation, which we later control for in a dynamic panel model.
The less rational traders are assumed to choose three forecasting models: naive, exponential smoothing, and autoregressive moving average (ARMA). Rather than testing a large number of forecasting models, we adopt these three simple models, which are widely used but incorporate only past prices. Therefore, if we measure their forecasting accuracy as predictability, the level of predictability ought to be conservative and not overstated. Also, this level can be considered a minimum level of predictability for more rational or able investors.
In detail, first, the naive model uses the historical average of returns in the estimation period as the future forecast. It is also used as a benchmark for calculating forecasting performance. Second, the simple exponential smoothing model (Gardner and Mckenzie Citation1985) predicts the future returns as the weighted average of the current return and the immediate-past forecast. The weights are estimated to minimize the Schwarz information criterion (SIC). Finally, the ARMA models use the past values of returns and the contemporaneous and past errors to predict future returns. Specifically, we choose the best AR and MA orders based on the SIC after testing up to three lags.
Single forecasts from these three models are additionally merged into combined forecasts, which are known to perform better and be less variable than single forecasts (Hibon and Evgeniou Citation2005). We use six combination methods. Except for the median method, they calculate the weighted average of three single forecasts (), where wi is the weight of single forecast i (
). The mean method (the first method) and the median methods (second) calculate the average and the median of three forecasts, respectively. They often outperform more complicated methods (Clemen Citation1989). The least-squares method (third), which was popularized by Granger and Ramanathan (Citation1984), finds the wi that minimizes the forecast errors in the training period. The rank method (fourth) of Aiolfi and Timmermann (Citation2006) evaluates the performance of single forecasts in the training period and calculates wi by the performance rank (ρi) as
The Akaike (fifth) and the Bayesian (sixth) information criterion methods calculate wi from the values of information criterion (C) as
The predictability in each rolling window τ (PDτ) is measured as the largest reduction of forecasting errors relative to those by the naive model. In other words, PD represents the best forecasting accuracy among the single and combined forecasting models:
(1)
(1)
where M is the root mean squared error of forecasted returns over actual returns and m is single or combined forecasting models except the naive (v) model. This measure is essentially similar to finding the model with the largest out-of-sample R2 (Campbell and Thompson Citation2008; Henkel, Martin, and Nardari Citation2011) among candidate forecasting models. In addition, the statistical significance of predictability is evaluated against the naive model following the tests by Clark and West (Citation2007) and Zhang et al. (Citation2019). Note that we do not adopt Westerlund and Narayan’s (Citation2015a, Citation2015b) method or its extension (Devpura, Narayan, and Sharma Citation2018) despite the methods’ growing popularity in testing cross-sectional predictability. Our study uses a different definition of predictability based on out-of-sample forecasting. Also, their methods essentially use a bivariate model between a return and a nonprice predictor and thus are not easily used to test the time-series predictability of return, which is of a univariate nature.
Identifying the Indicators of Time-Series Predictability
Finally, we bring together the individual series of PD generated for each sector into a dynamic panel. Then, we identify the indicators for sector-level time-series predictability. Technically, we estimate how the values of individual indicators in the estimation periods are associated with the predictability in the evaluation periods of the rolling windows. We adopt two dynamic panel models: the dynamic fixed effects (FE) model and the dynamic common correlated effects (DCCE) model.
Our first model is the dynamic FE model with autoregressive terms of PD:
(2)
(2)
where s is a sector, α is a cross-section fixed effect, x is an indicator of predictability, β is its coefficient, γ is a vector of coefficients for lagged PDs, and e is the error term.
We add eight lagged values of PD (l = 1, …, 8) to account for the correlation that may be caused by overlapping estimation periods in the rolling-window method. The values of the indicators for each rolling window τ in sector s (xs,τ) are calculated as the average values within the corresponding estimation period and the sector. We test each indicator individually, which is a common approach in studies of return predictors.
Despite their wide use, FE models allow only the intercepts to differ across the cross-section groups (Blackburne and Frank Citation2007) and assume all other coefficients and error variances are the same (Pesaran, Shin, and Smith Citation1999). Furthermore, the dynamic FE estimator with the lagged dependent variable is known to be subject to a downward bias, particularly in samples with a small number of observations (T) over time (Pesaran, Shin, and Smith Citation1999), although the impact of that characteristic should be minimal in our sample because it has a fairly large T.
Therefore, we use the DCCE estimator, which allows for heterogeneous parameters across cross-sections. The DCCE estimators (Chudik and Pesaran Citation2015) further account for unobserved common factors between cross-sections, which can generate cross-sectional dependence, by including both contemporaneous and lagged cross-section averages. This characteristic is especially important in sector-level international studies, where cross-sectional dependence can occur within local markets as well as across the same sectors and the related super-sectors in the global markets. The DCCE estimator is given by
(3)
(3)
where f is a vector of unobserved common factors, δ is a vector of heterogeneous factor-loading, and Ω is the variance–covariance matrix. In particular, the DCCE specifies
where
The sample estimates of β and γ are obtained as cross-sectional averages. The number of cross-sectional lags is calculated as T(1/3), following Chudik and Pesaran (Citation2015) and Ditzen (Citation2018). We additionally adopt recursive mean adjustment (see So and Shin Citation1999) for potentially autocorrelated data.
Selecting Candidate Indicators
The selection of the indicator variables is driven by the literature on cross-sectional predictability. If time-series and cross-sectional predictabilities are related (Moskowitz, Ooi, and Pedersen Citation2012), those variables may affect time-series predictability. On the one hand, the earlier literature (e.g., Fama and French Citation1988) applied the dividend discount model and tested dividend yield for cross-sectional predictability. Wang et al. (Citation2018) tested 12 macroeconomic variables and confirmed that dividend yield has particularly strong predictive power, whereas most of the others have little predictive power. Recent studies by Zhu (Citation2015) and Hammami and Zhu (Citation2020) also emphasize the importance of dividend yield.
On the other hand, several studies have adopted Campbell and Thompson’s (Citation2008) framework to examine a variety of single predictors (e.g., Westerlund, Narayan, and Zheng Citation2015; Westerlund and Narayan Citation2012; Phan, Sharma, and Narayan Citation2015; Rapach, Strauss, and Zhou Citation2010). Their results show that company fundamentals, market valuation, and trading activities can affect return predictability. For example, Phan, Sharma, and Narayan (Citation2015) find that a higher book-to-market ratio (B/M) and larger trading volume increase return predictability while a higher price-to-earnings ratio (P/E) and larger firm size decrease it. Similarly, Kong et al. (Citation2011) show that return predictability is stronger for small-cap stocks or those with a higher B/M. Chen, Firth, and Rui (Citation2001) highlight the role of trading volume. Liquidity and transaction costs can influence investment decisions too because they can obstruct the execution of any investment strategies. Similarly, wide bid–ask spreads increase stock predictability (see Chordia, Roll, and Subrahmanyam Citation2008; Chung and Hrazdil Citation2010a, Citation2010b). The return–illiquidity relationship (or illiquidity premium) can also be linked to predictability (see Amihud Citation2002; Amihud et al. Citation2015). Built on Welch and Goyal (Citation2008), Devpura, Narayan, and Sharma (Citation2018) conclude that P/E and stock volatility can explain the predictability. Government bond yields and term spreads are also often tested for a relationship with return predictability (see, e.g., Rapach, Ringgenberg, and Zhou Citation2016).
We, therefore, use the following sector-level factors as the indicators of predictability: dividend yield, P/E, book-to-price ratio (B/P), return on equity (ROE), dollar trading volume, market capitalization, illiquidity, and market/sector excess returns. In addition, we include a dummy for negative price trends and standard deviation for the general risk level. A dummy for business cycles is also adopted. As macroeconomic variables, we use short-term government bond yields (short rates) and term spreads. However, we do not use the factors related to corporate bonds and corporate issuing activity as in Wang et al. (Citation2018) and Welch and Goyal (Citation2008) because of a lack of consistent data for the study of international markets and sector-level analysis.
Our expectation is as follows. The valuation of stocks relative to fundamentals is known to influence predictability (Kong et al. Citation2011; Phan, Sharma, and Narayan Citation2015). On the one hand, lower valuation increases predictability (Kong et al. Citation2011) because highly valued stocks may be subject to sudden correction. We expect dividend yield, P/E, and B/P to behave similarly. We also test ROE, as operating performance, for comparison. On the other hand, trading volume can represent the magnitude of information flow (Chen, Firth, and Rui Citation2001). Large volumes may be a consequence of either excessive noise or relevant information, which can hinder or support predictability (Phan, Sharma, and Narayan Citation2015). Market capitalization may work similarly but can also represent market development, in the sense of markets becoming more efficient and less predictable.
Stock volatility may reflect excessive information arrival and make traders less successful in information processing and model estimation (Chung and Hrazdil Citation2010b; Timmermann Citation1993), but this uncertainty can create underpricing (Timmermann Citation1993, Citation1996), which may generate predictable price movement. Illiquidity hinders arbitrageurs’ ability to correct mispricing quickly (Chung and Hrazdil Citation2010a; Chordia, Roll, and Subrahmanyam Citation2008) and thus may increase predictability.
Sectoral excess return as industry stock price performance is linked to business cycles; stock return predictability is known to be higher in an economic downturn (Henkel, Martin, and Nardari Citation2011; Kim, Shamsuddin, and Lim Citation2011). This effect could be even stronger during negative sector performance and contraction in the business cycle (Guiso, Sapienza, and Zingales Citation2018; Fama and French Citation1989; Campbell and Cochrane Citation1999). Short-term government bond yields (or short rates), a common proxy for risk-free rates, may reflect monetary policies reacting to the business cycle. Similarly, term spreads are expected to work as an indicator of the business cycle. That is, low short rates and negative or low term spreads may be connected to higher predictability.
These indicators are roughly categorized as follows: price and trade originated (negative price trend, volatility, trading volume, market capitalization, illiquidity, and excess return), relative valuation (dividend yield, P/E, and B/P), operating performance (ROE), or macroeconomic (business cycle, short rates, and term spreads).
Testing Economic Significance
Knowing the indicators of sector-level predictability can help investors make decisions, but demonstrating that this knowledge can indeed provide economic significance is imperative. For example, regarding cross-sectional predictability, Narayan, Ahmed, and Narayan (Citation2017) show that the gains in investing in certain sectors based on nonprice predictors are substantial. The authors pay little attention, however, to the indicators of time-series predictability. Therefore, this study, first of all, investigates whether the sectors selected on the basis of the identified indicators provide stronger time-series predictability than the other sectors, both in the in-sample and the out-of-sample periods. In addition, stronger predictability needs to eventually turn into stronger economic benefits for investors. Therefore, we also examine whether economic significance is easily achievable from trading strategies built on our indicators. Like Balvers, Wu, and Gilliland (Citation2000) and Narayan, Liu, et al. (Citation2016), a common framework is to compare the returns from the trading strategies with the return of a buy-and-hold benchmark strategy. However, even though investors know the indicators of strong sector-level predictability, they can adopt numerous trading strategies. Therefore, as demonstrations, we test a small number of simple time-series–based strategies: strategies that require minimal effort and thus are suitable for less rational traders. If these simple strategies can outperform benchmarks, more-capable investors could do better with sophisticated strategies. Note that we do not adopt utility gains, as in Narayan, Hoang et al. (Citation2016), for example, because this measure cannot easily capture gains when investors choose more volatile sector investing over market portfolios.
We assume the investors know which sectors they need to choose following the indicators identified in this study. Their investment horizon is also rolling-window–based as specified previously. Specifically, after the estimation period of each rolling window, the investors select the top 10 sectors based on each indicator—for example, the top 10 least liquid sectors in cyclical super-sectors. Then, they use one of the three sector-level buy-and-hold strategies and hold the portfolios over the evaluation period. We calculate their investment returns and compare them with the market buy-and-hold strategy. The trading costs involving the indicator-based buy-and-hold strategies should be comparable to the market buy-and-hold strategies.
The investors’ trading strategies are as follows. First, the investors find which forecasting model works best in the previous rolling window and go long or short the stocks on the basis of its prediction for the current evaluation period. They trade only in the selected top 10 sectors in the expectation that their forecasting model will perform better in those sectors. Second, the investors adopt the naive forecasting model and go long or short the stocks on the basis of its prediction—that is, the average historical return in the estimation window. In this approach, the strategy is also a momentum strategy. Again, expecting higher predictability for those sectors, the investors trade those top 10 sectors. Finally, even more naively believing that predictability will turn into profitability in the top 10 sectors, the investors simply buy and hold those sectors. This last approach can be said to be a buy-and-hold strategy in sector investing, and the earlier two strategies are its variants with forecasting elements.
Data
The sample data cover 47 international stock markets from 2 January 1999 to 30 June 2022 (see ). For each market, one market-wide index and 11 industry-sector indices are used. The number of daily observations is 6,310 for each return series and 3,273,203 in total. Each daily return series is eventually converted to the predictability series by the rolling-window method as explained in the section on methodology. The number of windows is 236 for each sector and market and 84,692 in total. The first 185 windows in each series, ending on 22 September 2016, are used as the in-sample period (IS) to estimate the model for the indicators of time-series predictability. Then, the remaining 50 windows, between 23 September 2016 and 30 June 2022, are used as the out-of-sample period (OOS). They are equally divided into the pre-pandemic out-of-sample period (OOS1) and the pandemic period (OOS2). Periods OOS1 and OOS2 are divided by the date on which the World Health Organization declared a public health emergency: 31 January 2020. Our main data source for stock prices is the Thomson Datastream Global Equity Indices, which provides a standardized way for comparative studies of international stock markets. The corresponding financial and accounting variables are from Datastream Worldscope and Eikon.
Table 1. Summary Statistics I: Market Averages
The sector classification follows the Industry Classification Benchmark: energy (ENEG), basic materials (BMAT), industrials (INDU), consumer discretionary (CODI), consumer staples (COST), telecommunication (TELC), technology (TECN), financials (FINA), utilities (UTIL), health care (HLTH), and real estate (RLES). The industry sectors are further categorized into three super-sectors on the basis of their implications for investment strategies (Morningstar Citation2010): (1) cyclical, which contains BMAT, CODI, FINA, and RLES; (2) sensitive, which contains ENEG, INDU, TELC, and TECN; and (3) defensive, which contains COST, UTIL, and HLTH. Cyclical super-sectors are the most pro-cyclical in terms of following business cycles and having high beta risk. Sensitive super-sectors are moderately related to business cycles. Defensive super-sectors are counter-cyclical and characterized by low beta risk because the stock prices are generally not affected by economic fluctuations (Singh Citation2020; Makarov and Papanikolaou Citation2008). The firms in the defensive super-sectors generate revenue during recessions as well as other parts of the business cycle and thus exhibit low volatility.
Using the MSCI Country Classification Standard, we also classify the sample sectors into three subgroups based on market development, namely, developed (DEV), emerging (EMG), and frontier (FRT). The frontier markets are only included in the full sample analysis, not the subsample analysis, because of their small sample size.
The indicators of predictability are measured as their average values in the estimation period in each rolling window. Specifically, a general risk level is measured by the standard deviation of return. Market capitalization is represented by the total value of ordinary shares of all index constituents, in millions of US dollars. Dollar trading volume or turnover by volume (TV) is the aggregation of the number of shares traded multiplied by the closing price, in thousands of US dollars. Illiquidity (IL) is measured following Amihud’s (Citation2002) illiquidity measure: the absolute return divided by dollar trading volume plus 1. Stock performance (PF) is proxied by stock index returns less risk-free rates. DY, P/E, ROE, and B/P are weighted by the market values of the constituents. Following the business cycle dating of the National Bureau of Economic Research, the business cycle (BC) indicator has a value of 1 in expansion and 0 in contraction. Short rates representing risk-free rates are proxied by short-term (3-month) government bond yields. For the countries without these data, we adopt the next shortest term government bond yield (1 or 2 years). Term spreads (TS) are the gap in yields between long-term (10-year) government bonds and RF. The correlations of these indicators are presented in . The variables are winsorized at 1 and 99% to minimize the impact of outliers and normalized for regression.
Table 2. Summary Statistics II: Correlations
Results and Discussion
Time-series predictability exists in about 14% of the rolling windows of all tested sectors (, Panel A). Considering that the predictability can arise in about 5% of them by chance, this finding confirms a significant level of predictability despite earlier findings that once variability is considered, predictability might appear only sporadically (Timmermann Citation2008). The average value of measured predictability (PD) is around 0.013 (1.3%), which essentially quantifies the less rational investors’ forecasting performance over naive forecasting (, Panel B).
Table 3. Time-Series Predictability
A certain level of cross-sectional variation of time-series predictability is observed across sectors (as shown in Panels A and B of ). For example, the cyclical super-sectors are relatively more predictable than the other super-sectors. The financial, real estate, industrial, and technology sectors exhibit strong predictability. This sector-level heterogeneity is consistent with Kong et al. (Citation2011), but its size is small. The emerging and frontier markets are more predictable than the developed markets (Panel B of ). Panel C of clearly shows that time-series predictability is stronger in economic downturns (BC = 0) than in expansion (BC = 1) as is cross-sectional predictability (Henkel, Martin, and Nardari Citation2011). Panel C also supports the previous finding from the statistical tests of time-series predictability (Kim, Shamsuddin, and Lim Citation2011). Cyclical super-sectors show a stronger variation across business cycles than other super-sectors. Their predictability may have a cyclical nature too.
summarizes the indicators influencing time-series predictability in all sectors and markets. Clearly, the dynamic FE results tend to overstate the significance of the indicators of predictability. Therefore, we focus on the DCCE results because their estimates are unbiased under dynamic structure and cross-sectional dependence. In the DCCE results, illiquidity (IL), price downtrend, and performance (NT and PF) significantly affect time-series predictability. The indicators linked to relative valuation, operating performance, and macroeconomy do not show significance either at the sector or market level.
Table 4. Time-Series Predictability and Its Indicators
First, illiquidity is positively related to time-series predictability at a sector level. On the one hand, this finding verifies earlier findings that less liquid sectors deter arbitrageurs and thus have higher predictability (Chung and Hrazdil Citation2010a; Chordia, Roll, and Subrahmanyam Citation2008). Therefore, less rational investors can enjoy more success from their forecasting methods in such sectors. On the other hand, this positive relationship may partly reflect the cross-sectional link between stock returns and the illiquidity premium. It is known that investors require a premium for holding illiquid stocks (Amihud and Mendelson Citation1986; Amihud et al. Citation2015). If the illiquidity premium changes over time, it may subsequently affect time-series predictability.
Second, sectoral stock performance (PF) has a strong negative relationship with time-series predictability at the market level. In other words, stock markets with stronger (weaker) price performance have lower (higher) predictability. Downward trending markets (NT) have even higher predictability. This finding implies that less rational investors’ forecasting will be more accurate in weakly performing markets. This evidence is consistent with the macroeconomic-level link between business cycles and market predictability. Stock markets are known to be more predictable during economic downturns (Henkel, Martin, and Nardari Citation2011) but less predictable in economic expansions (Kim, Shamsuddin, and Lim Citation2011). The reason could be that investors asking for a higher premium in economic downturns increase predictability (Fama and French Citation1989; Campbell and Cochrane Citation1999; Guiso, Sapienza, and Zingales Citation2018). However, the BC indicator does not capture this effect in the DCCE results. The implication is that the nature of this relationship is more dynamic than what a simple dummy can reflect and is overshadowed by other factors.
Third, the indicators related to company fundamentals—such as dividend yield and operating performance—have insignificant relationships with time-series predictability. Although fundamentals are important theoretical building blocks in stock-pricing models, their predictive power for stock returns is inconclusive (Paye and Timmermann Citation2006). For example, dividend yield shows no strong evidence of predictability in Hjalmarsson (Citation2010). Its link to time-series predictability could be similarly weak. Finally, other indicators related to the relative valuation of stocks, such as P/E and B/P, are not linked to time-series predictability despite their power as predictors (Kong et al. Citation2011; Phan, Sharma, and Narayan Citation2015).
Unlike the DCCE indicators, the FE estimators overstate the significance of the indicators. It could be a consequence of the limitations of FE models in ignoring cross-sectional dependence and causing a bias in dynamic models (Nickell Citation1981). These indicators include volatility, trading volume, and market capitalization. Similarly, the business cycle indicator (BC) is significant only in the FE models, although its sign and significance match previous studies (Guiso, Sapienza, and Zingales Citation2018; Fama and French Citation1989; Campbell and Cochrane Citation1999). However, the FE and the DCCE results are not essentially different in terms of the signs of estimated coefficients. Therefore, those insignificant indicators in the DCCE results deserve further investigation by subsample analysis, that is, where their cross-sectional averages are calculated within each subsample.
The first subgroup analysis compares the industry sectors of developed and emerging markets (as shown in the left panels of ). The first difference between the subsample results and the whole sample’s results (shown in ) is the significant positive influence of volatility in both markets. In other words, less rational investors’ forecasting performance is stronger in the more volatile sectors. This rather unintuitive result can happen when higher estimation uncertainty creates overall underpricing (Timmermann Citation1993, Citation1996) and the subsequent correction generates predictability over time. Therefore, even less rational investors can benefit from easy-to-detect patterns. Similar empirical evidence is shown by Kim, Shamsuddin, and Lim (Citation2011). Another difference is the loss of significance in illiquidity. Illiquidity and the illiquidity premium are known to be larger in the emerging markets (Amihud et al. Citation2015) together with higher structural risk (Donadelli and Persha Citation2014), but they may not be linked to time-series predictability. Sector-level price performance (PF) maintains the same significant negative effect in both markets.
Table 5. Predictability and Its Indicators: Subgroup Analysis
A notable difference between the developed and the emerging markets is that a larger trading volume reduces time-series predictability only in the developed markets. Trading volume may reflect excessive noise in the market, which reduces predictability (Phan, Sharma, and Narayan Citation2015). The investors in the developed markets, however, are likely to be more able, so their trading increases market efficiency while decreasing predictability. Similarly, the subgroup analysis can reveal which specific indicator is more dominant between the two types of markets. For example, the sectors in the developed market become more predictable with price downtrends (NT) or larger dividend payments (DY). Therefore, the less rational investors ought to look for information on prices and dividends in the developed markets.
Macroeconomic factors, such as business cycles, short rates, and term spreads do not show any difference between the developed and the emerging markets and they are all insignificant. This finding does not match those of earlier studies on the predictors of returns. For example, both RF and TS are strong predictors of returns (Hjalmarsson Citation2010), and BC affects the power of TS as a predictor in the developed markets (Henkel, Martin, and Nardari Citation2011; Kim, Shamsuddin, and Lim Citation2011). Our results show that the indicators of predictability behave differently from the predictors of returns.
The second subgroup analysis examines three super-sectors separately: cyclical, sensitive, and defensive (as shown in the right panels of ). Across all three super-sectors, volatility (SD) remains as strongly relevant as in the whole sample. However, each super-sector has distinctive indicators. First, the cyclical super-sectors are associated with the largest number of indicators: NT, SD, MC, IL, and TS. The high sensitivity of cyclical super-sectors to business cycles may be behind the significance of these indicators if the indicators share this sensitivity, which can increase return predictability (Henkel, Martin, and Nardari Citation2011; Kim, Shamsuddin, and Lim Citation2011). However, the link to the business cycle indicator is not directly observable here as in the all-sample results. Next, the sensitive super-sectors have three identified indicators—SD, IL, and PF—and the defensive super-sectors have only one—SD. This finding shows that volatility is a universal indicator of predictability across super-sectors whereas the other indicators are likely to be related to the business cycle to some degree.
In summary, stronger time-series predictability can be found from eight indicators in specific sectors as follows: (1) price downtrends, specifically in the developed markets and cyclical super-sectors; (2) higher volatility in general; (3) higher illiquidity, particularly in cyclical and sensitive super-sectors; (4) lower price performance in the sensitive super-sectors; (5) lower trading volume in the developed markets; (6) smaller market capitalization in the cyclical super-sectors; (7) higher dividend yields in the developed markets; and (8) larger term spreads in the cyclical super-sectors.
In terms of subgroups, first, in all sectors, the more volatile sectors are more predictable. Second, in the developed markets, stronger predictability is expected with price downtrends, lower trading volume, and higher dividend yields. Third, the emerging markets do not have such relationships. Fourth, in the sensitive super-sectors, predictability increases when liquidity and price performance decrease. Finally, in the cyclical super-sectors, higher predictability is linked to down-trending price, smaller market size, and larger term spread. By investing in these sectors, the less rational trader can obtain higher predictability while enjoying better diversification than investing in individual stocks.
Whether these findings deliver economic significance to investors is another question. First of all, we need to examine whether the sectors selected by the eight indicators just listed do indeed provide higher predictability and whether such predictability is persistent in the out-of-sample periods. summarizes the net predictability gains in the selected eight groups of sectors over the rest of the sectors. Clearly, the selected sectors can provide persistently higher predictability than the other sectors in all eight groups, although the absolute size of the predictability gain is not large. The gain is not only positive in the in-sample period (IS) but also positive in both out-of-sample periods (OOS1 and OOS2). The impact of the COVID-19 pandemic on predictability is not obvious.
Table 6. Predictability Gains
Therefore, trading strategies can be devised to generate economic significance—for example, higher returns—from stronger predictability. presents the net return gains in sector investing when building trading strategies based on the six indicators in our study. We exclude two indicators, NT and PF, because they are directly linked to sector price performance and thus are not ideal when calculating gains in sector-level returns. Specifically, we test the simple buy-and-hold strategy in sector investing (B&H) and two variants with forecasting elements—buy or sell following momentum (MOM) and the previous best forecasting method (BST).
Table 7. Return Gains
The results are summarized in terms of the net gains over the nonselected sectors (left panel) and the net gains over the market buy-and-hold strategy (right panel). shows that the return gains are limited to certain indicators and trading strategies. For example, the B&H strategy generates consistently higher return gains against the market and the rest of the sectors when tested in the sectors with the larger market cap, higher illiquidity, and larger term spreads. The magnitude of the return gains from profitable trading strategies in these sectors reaches, on average, about 0.6% over 25 trading days (or 6% over 250 trading days). The MOM strategy is also profitable in large or highly illiquid sectors. These gains were not affected by the COVID-19 pandemic (OOS2). We assume that the trading costs when adopting the market B&H strategy and the three tested trading strategies are similar for the same amount of investment since the tested strategies are also buy-and-hold approaches at a sector level. BST, however, which requires certain time-series forecasting skills, does not beat the market. This finding implies that any other sophisticated strategies, which need more complex forecasting or frequent trading than B&H and MOM, may find it difficult to generate sufficient gains to be usable because they are likely to be even more costly. However, it is also fair to note that we did not test a large number of the available trading strategies.
Conclusion
We have described our investigation of the relationship between time-series predictability and its sector-level indicators. We focused on investors who are searching for higher predictability for sector investing but are not as well informed as other investors. We examined 11 industry sectors across 47 international stock markets based on the dynamic fixed-effects and the dynamic common correlated effects models. The sample period covers two out-of-sample periods, including the COVID-19 pandemic period.
The main takeaway from this study is identification of the indicators of sector-level time-series predictability. Specifically, our key findings are as follows: Time-series predictability differs across sectors and is linked to the indicators of predictability at a sector level. Investors can expect stronger time-series predictability by carefully choosing sectors based on specific indicators. For example, in the developed markets, they can select sectors with down-trending prices, lower trading volumes, and higher dividend yields. To invest in the cyclical or sensitive super-sectors—that is, for business cycle investing—highly illiquid sectors or those in in which prices are underperforming are good destinations for investing based on higher predictability. For the cyclical super-sectors, investors can also choose small sectors in the markets where term spreads are large. They can also generally go for the more volatile sectors for higher predictability. The roles of company fundamentals and relative valuation are limited. Using the chosen indicators, investors could have consistently enjoyed strong predictability gains even in out-of-sample periods, including the COVID-19 pandemic period. They could have also obtained return gains by following certain indicators and using simple trading strategies.
This study offers comprehensive evidence of the indicators of time-series predictability and shows how they can be used to generate consistent predictability and economic gains for sector investing. Our study provides important implications along several dimensions that are of relevance to both academics and investors. First of all, we provide forward-looking indicators for investors who seek the more predictable sectors where they can attempt to generate more profits. We essentially show that time-series predictability displays sector-level heterogeneity identifiable by the indicators. These indicators can help even investors who are not able or willing to use all available information. Additionally, we provide the methods of measuring time-series predictability using investors’ forecasting as defined in our study. We find that the impact of the COVID-19 pandemic on time-series predictability and its indicators has been limited.
Some limitations of this study could be addressed by future research. A natural extension to sector investing could be the examination of individual company stocks and other investment portfolios, such as growth, value, large-cap, and small-cap portfolios. Other factors, such as investor sentiment and attitude, and industry specifics, such as competition, concentration, regulatory changes, and external events, could also be examined. Moreover, future extensions could include using an index like the one developed by Narayan, Iyke, and Sharma (Citation2021) for more comprehensive tests of the impact of the COVID-19 pandemic and tests of profitability for several active trading strategies.
Disclosure statement
No potential conflict of interest was reported by the author(s).
Additional information
Notes on contributors
Jin Suk Park
Jin Suk Park is an Associate Professor in Finance at Centre for Financial and Corporate Integrity, Coventry University, United Kingdom.
Mohammad Khaleq Newaz
Mohammad Khaleq Newaz is an Assistant Professor in Finance at School of Economics, Finance and Accounting, Coventry University, United Kingdom.
References
- Acharya, Viral V., and Lasse Heje Pedersen. 2005. “Asset Pricing with Liquidity Risk.” Journal of Financial Economics 77 (2): 375–410. doi:10.1016/j.jfineco.2004.06.007.
- Aiolfi, Marco, and Allan Timmermann. 2006. “Persistence in Forecasting Performance and Conditional Combination Strategies.” Journal of Econometrics 135 (1–2): 31–53. doi:10.1016/j.jeconom.2005.07.015.
- Alexiou, Constantinos, and Anshul Tyagi. 2020. “Gauging the Effectiveness of Sector Rotation Strategies: Evidence from the USA and Europe.” Journal of Asset Management 21 (3): 239–260. doi:10.1057/s41260-020-00161-6.
- Amihud, Yakov, Allaudeen Hameed, Wenjin Kang, and Huiping Zhang. 2015. “The Illiquidity Premium: International Evidence.” Journal of Financial Economics 117 (2): 350–368. doi:10.1016/j.jfineco.2015.04.005.
- Amihud, Yakov, and Haim Mendelson. 1986. “Asset Pricing and the Bid–Ask Spread.” Journal of Financial Economics 17 (2): 223–249. doi:10.1016/0304-405X(86)90065-6.
- Amihud, Yakov. 2002. “Illiquidity and Stock Returns: Cross-Section and Time-Series Effects.” Journal of Financial Markets 5 (1): 31–56. doi:10.1016/S1386-4181(01)00024-6.
- Balvers, Ronald, Yangru Wu, and Erik Gilliland. 2000. “Mean Reversion across National Stock Markets and Parametric Contrarian Investment Strategies.” The Journal of Finance 55 (2): 745–772. doi:10.1111/0022-1082.00225.
- Bannigidadmath, Deepa, and Paresh Kumar Narayan. 2016. “Stock Return Predictability and Determinants of Predictability and Profits.” Emerging Markets Review 26: 153–173. doi:10.1016/j.ememar.2015.12.003.
- Barberis, Nicholas, and Richard Thaler. 2005. “A Survey of Behavioral Finance.” In Advances in Behavioral Finance, edited by Richard H. Thaler, 1–24. Princeton, NJ: Princeton University Press.
- Beller, Kenneth R., John L. Kling, and Michael J. Levinson. 1998. “Are Industry Stock Returns Predictable?” Financial Analysts Journal 54 (5): 42–57. doi:10.2469/faj.v54.n5.2211.
- Berger, Dave, Kuntara Pukthuanthong, and J. Jimmy Yang. 2011. “International Diversification with Frontier Markets.” Journal of Financial Economics 101 (1): 227–242. doi:10.1016/j.jfineco.2011.02.009.
- Blackburne, Edward F., and Mark W. Frank. 2007. “Estimation of Nonstationary Heterogeneous Panels.” The Stata Journal: Promoting Communications on Statistics and Stata 7 (2): 197–208. doi:10.1177/1536867X0700700204.
- Busse, Jeffrey A., and Qing Tong. 2012. “Mutual Fund Industry Selection and Persistence.” Review of Asset Pricing Studies 2 (2): 245–274. doi:10.1093/rapstu/ras004.
- Campbell, John Y., and John H. Cochrane. 1999. “By Force of Habit: A Consumption-Based Explanation of Aggregate Stock Market Behavior.” Journal of Political Economy 107 (2): 205–251. doi:10.1086/250059.
- Campbell, John Y., and Robert J. Shiller. 1988. “Stock Prices, Earnings, and Expected Dividends.” The Journal of Finance 43 (3): 661–676. doi:10.1111/j.1540-6261.1988.tb04598.x.
- Campbell, John Y., and Samuel B. Thompson. 2008. “Predicting Excess Stock Returns out of Sample: Can Anything Beat the Historical Average?” Review of Financial Studies 21 (4): 1509–1531. doi:10.1093/rfs/hhm055.
- Cavaglia, Stefano, Christopher Brightman, and Michael Aked. 2000. “The Increasing Importance of Industry Factors.” Financial Analysts Journal 56 (5): 41–54. doi:10.2469/faj.v56.n5.2389.
- Chen, Gong Meng, Michael Firth, and Oliver M. Rui. 2001. “The Dynamic Relation between Stock Returns, Trading Volume, and Volatility.” The Financial Review 36 (3): 153–174. doi:10.1111/j.1540-6288.2001.tb00024.x.
- Chordia, Tarun, Richard Roll, and Avanidhar Subrahmanyam. 2008. “Liquidity and Market Efficiency.” Journal of Financial Economics 87 (2):249–268. doi:10.1016/j.jfineco.2007.03.005.
- Chudik, Alexander, and M. Hashem Pesaran. 2015. “Common Correlated Effects Estimation of Heterogeneous Dynamic Panel Data Models with Weakly Exogenous Regressors.” Journal of Econometrics 188 (2): 393–420. doi:10.1016/j.jeconom.2015.03.007.
- Chung, Dennis Y., and Karel Hrazdil. 2010a. “Liquidity and Market Efficiency: Analysis of NASDAQ Firms.” Global Finance Journal 21 (3): 262–274. doi:10.1016/j.gfj.2010.09.004.
- Chung, Dennis Y., and Karel Hrazdil. 2010b. “Liquidity and Market Efficiency: A Large Sample Study.” Journal of Banking & Finance 34 (10): 2346–2357. doi:10.1016/j.jbankfin.2010.02.021.
- Ciner, Cetin. 2021. “Stock Return Predictability in the Time of COVID-19.” Finance Research Letters 38 (January): 101705. doi:10.1016/j.frl.2020.101705.
- Clark, Todd E., and Kenneth D. West. 2007. “Approximately Normal Tests for Equal Predictive Accuracy in Nested Models.” Journal of Econometrics 138 (1): 291–311. doi:10.1016/j.jeconom.2006.05.023.
- Clemen, Robert T. 1989. “Combining Forecasts: A Review and Annotated Bibliography.” International Journal of Forecasting 5 (4): 559–583. doi:10.1016/0169-2070(89)90012-5.
- Cooper, Michael, and Huseyin Gulen. 2006. “Is Time-Series-Based Predictability Evident in Real Time?” The Journal of Business 79 (3): 1263–1292. doi:10.1086/500676.
- Dai, Zhifeng, and Huan Zhu. 2020. “Stock Return Predictability from a Mixed Model Perspective.” Pacific-Basin Finance Journal 60 (April): 101267. doi:10.1016/j.pacfin.2020.101267.
- Dangl, Thomas, and Michael Halling. 2012. “Predictive Regressions with Time-Varying Coefficients.” Journal of Financial Economics 106 (1): 157–81. doi:10.1016/j.jfineco.2012.04.003.
- Devpura, Neluka, Paresh Kumar Narayan, and Susan Sunila Sharma. 2018. “Is Stock Return Predictability Time-Varying?” Journal of International Financial Markets, Institutions and Money 52:152–72. doi:10.1016/j.intfin.2017.06.001.
- Ditzen, Jan. 2018. “Estimating Dynamic Common-Correlated Effects in Stata.” The Stata Journal: Promoting Communications on Statistics and Stata 18 (3): 585–617. doi:10.1177/1536867X1801800306.
- Donadelli, Michael, and Lauren Persha. 2014. “Understanding Emerging Market Equity Risk Premia: Industries, Governance and Macroeconomic Policy Uncertainty.” Research in International Business and Finance 30 (1): 284–309. doi:10.1016/j.ribaf.2013.09.008.
- Fama, Eugene F. 1991. “Efficient Capital Markets: II.” The Journal of Finance 46 (5): 1575–1617. doi:10.1111/j.1540-6261.1991.tb04636.x.
- Fama, Eugene F., and Kenneth R. French. 1988. “Dividend Yields and Expected Stock Returns.” Journal of Financial Economics 22 (1): 3–25. doi:10.1016/0304-405X(88)90020-7.
- Fama, Eugene F., and Kenneth R. French. 1989. “Business Conditions and Expected Returns on Stocks and Bonds.” Journal of Financial Economics 25 (1): 23–49. doi:10.1016/0304-405X(89)90095-0.
- Gardner, E. S., and Ed Mckenzie. 1985. “Forecasting Trends in Time Series.” Management Science 31 (10): 1237–1246. doi:10.1287/mnsc.31.10.1237.
- Granger, Clive W. J., and Ramu Ramanathan. 1984. “Improved Methods of Combining Forecasts.” Journal of Forecasting 3 (2): 197–204. doi:10.1002/for.3980030207.
- Guiso, Luigi, Paola Sapienza, and Luigi Zingales. 2018. “Time Varying Risk Aversion.” Journal of Financial Economics 128 (3): 403–421. doi:10.1016/j.jfineco.2018.02.007.
- Hammami, Yacine, and Jie Zhu. 2020. “Understanding Time-Varying Short-Horizon Predictability.” Finance Research Letters 32: 101097. doi:10.1016/j.frl.2019.01.009.
- Harvey, Campbell R. 1995. “Predictable Risk and Returns in Emerging Markets.” Review of Financial Studies 8 (3): 773–816. doi:10.1093/rfs/8.3.773.
- Hasan, Md Tanvir. 2022. “The Sum of All SCARES COVID-19 Sentiment and Asset Return.” The Quarterly Review of Economics and Finance 86: 332–346. doi:10.1016/j.qref.2022.08.005.
- Henkel, Sam James, J. Spencer Martin, and Federico Nardari. 2011. “Time-Varying Short-Horizon Predictability.” Journal of Financial Economics 99 (3): 560–580. doi:10.1016/j.jfineco.2010.09.008.
- Hibon, Michèle, and Theodoros Evgeniou. 2005. “To Combine or Not to Combine: Selecting among Forecasts and Their Combinations.” International Journal of Forecasting 21 (1): 15–24. doi:10.1016/j.ijforecast.2004.05.002.
- Hjalmarsson, Erik. 2010. “Predicting Global Stock Returns.” Journal of Financial and Quantitative Analysis 45 (1): 49–80. doi:10.1017/S0022109009990469.
- Jame, Russell, and Qing Tong. 2014. “Industry-Based Style Investing.” Journal of Financial Markets 19 (1): 110–30. doi:10.1016/j.finmar.2013.08.004.
- Jordan, Steven J., Andrew J. Vivian, and Mark E. Wohar. 2014. “Forecasting Returns: New European Evidence.” Journal of Empirical Finance 26: 76–95. doi:10.1016/j.jempfin.2014.02.001.
- Kacperczyk, Marcin, Clemens Sialm, and Lu Zheng. 2005. “On the Industry Concentration of Actively Managed Equity Mutual Funds.” The Journal of Finance 60 (4): 1983–2011. doi:10.1111/j.1540-6261.2005.00785.x.
- Kim, Jae H., Abul Shamsuddin, and Kian Ping Lim. 2011. “Stock Return Predictability and the Adaptive Markets Hypothesis: Evidence from Century-Long U.S. Data.” Journal of Empirical Finance 18 (5): 868–879. doi:10.1016/j.jempfin.2011.08.002.
- Kong, Aiguo, David E. Rapach, Jack K. Strauss, and Guofu Zhou. 2011. “Predicting Market Components out of Sample: Asset Allocation Implications.” The Journal of Portfolio Management 37 (4): 29–41. doi:10.3905/jpm.2011.37.4.029.
- Lagoarde-Segot, Thomas. 2009. “Financial Reforms and Time-Varying Microstructures in Emerging Equity Markets.” Journal of Banking & Finance 33 (10): 1755–1769. doi:10.1016/j.jbankfin.2009.01.007.
- Lim, Kian Ping, and Robert Brooks. 2011. “The Evolution of Stock Market Efficiency over Time: A Survey of the Empirical Literature.” Journal of Economic Surveys 25 (1): 69–108. doi:10.1111/j.1467-6419.2009.00611.x.
- Lo, Andrew. 2004. “The Adaptive Markets Hypothesis: Market Efficiency from an Evolutionary Perspective.” The Journal of Portfolio Management 30 (5): 15–29. doi:10.3905/jpm.2004.442611.
- Ma, Feng, Xinjie Lu, Jia Liu, and Dengshi Huang. 2022. “Macroeconomic Attention and Stock Market Return Predictability.” Journal of International Financial Markets, Institutions and Money 79 (April): 101603. doi:10.1016/j.intfin.2022.101603.
- Makarov, Igor, and Dimitris Papanikolaou. 2008. “Sources of Systematic Risk.” SSRN Electronic Journal (2008), 1–13. doi:10.2139/ssrn.968229.
- McLean, R. David, and Jeffrey Pontiff. 2016. “Does Academic Research Destroy Stock Return Predictability?” The Journal of Finance 71 (1): 5–32. doi:10.1111/jofi.12365.
- Morningstar. 2010. “Morningstar Global Equity Classification Structure.” https://indexes.morningstar.com/resources/PDF/Methodology%20Documents/SectorArticle.pdf.
- Moskowitz, Tobias J., Yao Hua Ooi, and Lasse Heje Pedersen. 2012. “Time Series Momentum.” Journal of Financial Economics 104 (2): 228–250. doi:10.1016/j.jfineco.2011.11.003.
- Naidu, Dharmendra, and Kumari Ranjeeni. 2021. “Effect of Coronavirus Fear on the Performance of Australian Stock Returns: Evidence from an Event Study.” Pacific-Basin Finance Journal 66 (February): 101520. doi:10.1016/j.pacfin.2021.101520.
- Narayan, Paresh Kumar, and Deepa Bannigidadmath. 2015. “Are Indian Stock Returns Predictable?” Journal of Banking & Finance 58: 506–531. doi:10.1016/j.jbankfin.2015.05.001.
- Narayan, Paresh Kumar, Bernard Njindan Iyke, and Susan Sunila Sharma. 2021. “New Measures of the COVID-19 Pandemic: A New Time-Series Dataset.” Asian Economics Letters 2 (2) doi:10.46557/001c.23491.
- Narayan, Paresh Kumar, Dinh Hoang, Bach Phan, Susan Sunila Sharma, and Joakim Westerlund. 2016. “Are Islamic Stock Returns Predictable? A Global Perspective.” Pacific-Basin Finance Journal 40 (A): 210–223. doi:10.1016/j.pacfin.2016.08.008.
- Narayan, Paresh Kumar, Huson Ali Ahmed, and Seema Narayan. 2017. “Can Investors Gain from Investing in Certain Sectors?” Journal of International Financial Markets, Institutions and Money 48: 160–177. doi:10.1016/j.intfin.2017.01.003.
- Narayan, Paresh Kumar, Ruipeng Liu, and Joakim Westerlund. 2016. “A GARCH Model for Testing Market Efficiency.” Journal of International Financial Markets, Institutions and Money 41: 121–138. doi:10.1016/j.intfin.2015.12.008.
- Narayan, Seema, and Russell Smyth. 2015. “The Financial Econometrics of Price Discovery and Predictability.” International Review of Financial Analysis 42: 380–393. doi:10.1016/j.irfa.2015.09.003.
- Nickell, Stephen. 1981. “Biases in Dynamic Models with Fixed Effects.” Econometrica 49 (6): 1417–1426. doi:10.2307/1911408.
- Paye, Bradley S., and Allan Timmermann. 2006. “Instability of Return Prediction Models.” Journal of Empirical Finance 13 (3): 274–315. doi:10.1016/j.jempfin.2005.11.001.
- Pesaran, M. Hashem, Yongcheol Shin, and Ron P. Smith. 1999. “Pooled Mean Group Estimation of Dynamic Heterogeneous Panels.” Journal of the American Statistical Association 94 (446): 621–634. doi:10.1080/01621459.1999.10474156.
- Phan, Dinh Hoang Bach, Susan Sunila Sharma, and Paresh Kumar Narayan. 2015. “Stock Return Forecasting: Some New Evidence.” International Review of Financial Analysis 40 (July): 38–51. doi:10.1016/j.irfa.2015.05.002.
- Rahman, Md Lutfur, Abu Amin, and Mohammed Abdullah Al Mamun. 2021. “The COVID-19 Outbreak and Stock Market Reactions: Evidence from Australia.” Finance Research Letters 38: 101832. doi:10.1016/j.frl.2020.101832.
- Rapach, David E., Jack K. Strauss, and Guofu Zhou. 2010. “Out-of-Sample Equity Premium Prediction: Consistently Beating the Historical Average.” Review of Financial Studies 23 (2): 821–862. doi:10.1093/rfs/hhp063.
- Rapach, David E., Jack K. Strauss, Jun Tu, and Guofu Zhou. 2011. “Out-of-Sample Industry Return Predictability : Evidence from a Large Number of Predictors.” SSRN Electronic Journal, 1–35. doi:10.2139/ssrn.1307420.
- Rapach, David E., Matthew C. Ringgenberg, and Guofu Zhou. 2016. “Short Interest and Aggregate Stock Returns.” Journal of Financial Economics 121 (1): 46–65. doi:10.1016/j.jfineco.2016.03.004.
- Roche, Hervé, Stathis Tompaidis, and Chunyu Yang. 2013. “Why Does Junior Put All His Eggs in One Basket? A Potential Rational Explanation for Holding Concentrated Portfolios.” Journal of Financial Economics 109 (3): 775–796. doi:10.1016/j.jfineco.2013.03.016.
- Salisu, Afees A., and Xuan Vinh Vo. 2020. “Predicting Stock Returns in the Presence of COVID-19 Pandemic: The Role of Health News.” International Review of Financial Analysis 71 (April): 101546. doi:10.1016/j.irfa.2020.101546.
- Singh, Amanjot. 2020. “COVID-19 and Safer Investment Bets.” Finance Research Letters 36 (October): 101729. doi:10.1016/j.frl.2020.101729.
- So, Beong Soo., and Dong Wan Shin. 1999. “Recursive Mean Adjustment in Time-Series Inferences.” Statistics & Probability Letters 43 (1): 65–73. doi:10.1016/S0167-7152(98)00247-8.
- Timmermann, Allan. 1993. “How Learning in Financial Markets Generates Excess Volatility and Predictability in Stock Prices.” The Quarterly Journal of Economics 108 (4): 1135–1145. doi:10.2307/2118462.
- Timmermann, Allan. 1996. “Excess Volatility and Predictability of Stock Prices in Autoregressive Dividend Models with Learning.” The Review of Economic Studies 63 (4): 523–557. doi:10.2307/2297792.
- Timmermann, Allan. 2006. “Forecast Combinations.” In Handbook of Economic Forecasting 2, edited by Graham Elliott, Clive W.J. Granger, and Allan Timmermann, 135–196. Amsterdam: Elsevier.
- Timmermann, Allan. 2008. “Elusive Return Predictability.” International Journal of Forecasting 24 (1): 1–18. doi:10.1016/j.ijforecast.2007.07.008.
- Wang, Yudong, Li Liu, Feng Ma, and Xundi Diao. 2018. “Momentum of Return Predictability.” Journal of Empirical Finance 45: 141–156. doi:10.1016/j.jempfin.2017.11.003.
- Welch, Ivo, and Amit Goyal. 2008. “A Comprehensive Look at the Empirical Performance of Equity Premium Prediction.” Review of Financial Studies 21 (4): 1455–1508. doi:10.1093/rfs/hhm014.
- Westerlund, Joakim, and Paresh Kumar Narayan. 2012. “Does the Choice of Estimator Matter When Forecasting Returns?” Journal of Banking & Finance 36 (9): 2632–2640. doi:10.1016/j.jbankfin.2012.06.005.
- Westerlund, Joakim, and Paresh Kumar Narayan. 2015a. “A Random Coefficient Approach to the Predictability of Stock Returns in Panels.” Journal of Financial Econometrics 13 (3): 605–664. doi:10.1093/jjfinec/nbu003.
- Westerlund, Joakim, and Paresh Kumar Narayan. 2015b. “Testing for Predictability in Conditionally Heteroskedastic Stock Returns.” Journal of Financial Econometrics 13 (2): 342–375. doi:10.1093/jjfinec/nbu001.
- Westerlund, Joakim, Paresh Kumar Narayan, and Xinwei Zheng. 2015. “Testing for Stock Return Predictability in a Large Chinese Panel.” Emerging Markets Review 24: 81–100. doi:10.1016/j.ememar.2015.05.004.
- Yen, Gili, and Cheng-few Lee. 2008. “Efficient Market Hypothesis (EMH): Past, Present and Future.” Review of Pacific Basin Financial Markets and Policies 11 (02): 305–329. doi:10.1142/S0219091508001362.
- Zaremba, Adam, Alina Maydybura, Anna Czapkiewicz, and Marina Arnaut. 2021. “Explaining Equity Anomalies in Frontier Markets: A Horserace of Factor Pricing Models.” Emerging Markets Finance and Trade 57 (13): 3604–3633. doi:10.1080/1540496X.2019.1612361.
- Zhang, Yaojie, Qing Zeng, Feng Ma, and Benshan Shi. 2019. “Forecasting Stock Returns: Do Less Powerful Predictors Help?” Economic Modelling 78: 32–39. doi:10.1016/j.econmod.2018.09.014.
- Zhu, Xiaoneng. 2015. “Tug-of-War: Time-Varying Predictability of Stock Returns and Dividend Growth.” Review of Finance 19 (6): 2317–2358. doi:10.1093/rof/rfu047.